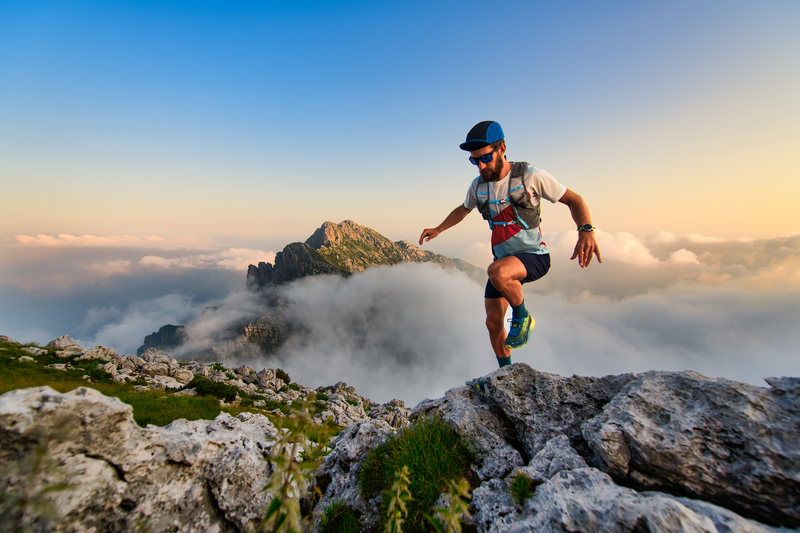
94% of researchers rate our articles as excellent or good
Learn more about the work of our research integrity team to safeguard the quality of each article we publish.
Find out more
REVIEW article
Front. Energy Res. , 22 July 2024
Sec. Smart Grids
Volume 12 - 2024 | https://doi.org/10.3389/fenrg.2024.1437614
This article is part of the Research Topic Advancements in Power System Condition Monitoring, Fault Diagnosis and Environmental Compatibility View all 14 articles
The transformer plays a critical role in maintaining the stability and smooth operation of the entire power system, particularly in power transmission and distribution. The paper begins by providing an overview of traditional fault diagnosis methods for transformers, including dissolved gas analysis and vibration analysis techniques, elucidating their developmental trajectory. Building upon these traditional methods, numerous researchers have aimed to enhance and optimize them through intelligent technologies such as neural networks, machine learning, and support vector machines. These researchers have addressed common issues in traditional fault diagnosis methods, such as the low correlation between characteristic parameters and faults, ambiguous fault descriptions, and the complexity of feature analysis. However, due to the complexity of transformer structures and the uncertainties in operating environments, the collection and analysis of characteristic parameters becomes highly intricate. Researchers have further refined algorithms and feature values based on intelligent diagnostic algorithms for transformers. The goal is to improve diagnostic speed, mitigate the impact of measurement noise, and further advance the adaptability of artificial intelligence technology in the field of transformers. On the other hand, the excellent multi-parameter analysis capability of artificial intelligence technology is more suitable for transformer diagnostic techniques that involve the fusion of multiple information sources. Through the powerful data acquisition, processing, and decision-making capabilities provided by intelligent algorithms, it can comprehensively analyze non-electrical parameters such as oil and gas characteristics, vibration signals, temperature, along with electrical parameters like short-circuit reactance and load ratio. Moreover, it can automatically analyze the inherent relationship between faults and characteristic quantities and provide decision-making suggestions. This technique plays a pivotal role in ensuring transformer safety and power network security, emerging as a prominent direction in transformer fault diagnosis research.
The power transformer stands as a vital component within transmission and distribution networks, serving as the linchpin for voltage and energy conversion (Barkas et al., 2022). Its operational integrity directly influences the safety and stability of the entire power system. However, due to manufacturing limitations, environmental complexities, and sporadic maintenance, transformers face relatively high failure rates. A malfunctioning transformer can trigger widespread power outages, significantly impacting people’s lives, industrial electricity usage, and causing substantial economic losses. Hence, timely and accurate fault diagnosis and prediction for transformers are imperative (Chakravorti et al., 2013). These proactive measures not only prevent potential hazards but also enhance the grid’s reliability and availability (Yu et al., 2016).
Early fault diagnosis methods for transformers, including preventive electrical tests, impulse voltage waveform tests, dissolved gas analysis (DGA), and vibration analysis (Roncero-Clemente and Roanes-Lozano, 2018; Lu et al., 2020; El-kenawy et al., 2022), have played a crucial role in preventing safety incidents and driving advancements in the power market. However, these traditional approaches often possess limitations in diagnostic feature quantity and precision under specific conditions, resulting in reduced diagnostic accuracy (Standard, 2000; Kim et al., 2013; Liu et al., 2015). Consequently, they are unable to fully meet the evolving diagnostic requirements for power transformers.
To overcome these limitations of traditional diagnostic methods, extensive research has delved into intelligent transformer diagnosis (Malik et al., 2020; Zhang et al., 2022). Artificial intelligence algorithms have excellent big data processing and learning ability (Divya et al., 2023). By processing a substantial volume of transformer fault data, these algorithms can conduct thorough analyses and predictions, effectively addressing challenges encountered in traditional diagnostic methods, including incomplete data analysis and the fuzzy relationship between features and faults. The outcome is a significant enhancement in the accuracy of diagnosis and detection, making it particularly valuable in transformer diagnosis. Various algorithms, including neural networks (Duraisamy et al., 2007), support vector machines (Zhu et al., 2019), machine learning (Zhuo and Ge, 2021) and others, have been employed for transformer fault diagnosis. However, due to the complexity of transformer internal structures and external environments, utilizing these intelligent algorithms in combination with traditional methods still presents challenges (Ma et al., 2021). Therefore, researchers have mitigated the impact of noise in measurements and improved the adaptability of intelligent diagnostic methods for various transformers by optimizing algorithms and enhancing feature extraction techniques (Jina et al., 2024; Wang et al., 2024), further propelling the development of intelligent diagnostic technologies. On the other hand, the concept of combining multiple diagnostic methods has emerged with the advancement of artificial intelligence technology. In the review of DGA, Taneja et al. (2016) proposed that intelligent diagnosis technology should not be confined to a singular diagnosis method but should encompass a comprehensive diagnosis involving multiple characteristic parameters. While establishing the health index for the transformer insulation system, Badawi et al. (2022) observed that the accuracy of verification using a single DGA is notably lower when compared to the comprehensive analysis of various characteristics, including DGA, winding resistance, insulation oil moisture content, acidity, etc. The estimation of health index results obtained through the use of a transformer detection system with multi-source information proves to be more accurate. Therefore, the development direction for intelligent transformer fault diagnosis is oriented towards integrating multi-source information, leveraging the data collection, analysis and processing capabilities of multi-source information integration technology. The transformer diagnosis technology based on multi-source data integration can identify various fault types efficiently and accurately, and provide a more comprehensive protection for the transformer (Zheng et al., 2018). This emphasizes the forward direction for ongoing research and development in transformer fault diagnosis technology, making a positive contribution to enhancing the reliability and stability of the power system.
Diagnosing transformer faults requires understanding the causes and types of different faults. With the development of the power market, the capacity of power transformers is constantly increasing to meet the needs of the market. To ensure uninterrupted operation, it is crucial to establish a detailed maintenance plan. In the process of analyzing the causes of faults, Kumar et al. (2015) classified the failure modes of transformers into electrical faults, mechanical faults, and thermal faults. These faults further break down into categories like external, ground, interphase short-circuit, and inter-turn faults (Soni and Mehta, 2021). These failures are often caused by winding deformation, insulation oil aging, overheating, system overload, design defects, and other factors (Hashemnia et al., 2016; Fu et al., 2017; Christina et al., 2018), as shown in Figure 1. By classifying transformer faults and analyzing their causes, one can clarify the objectives and approaches of transformer fault diagnosis.
External faults of transformers mainly refer to faults related to the external power grid or connection line of transformers. These problems arise from transmission line or transformer malfunctions, as well as faults from other devices connected to the external power transformer. Overloading the power system or overvoltage caused by lightning strikes are also categorized as external faults (Awadallah et al., 2015; Sun et al., 2021; Ounissi et al., 2023). For example, during power system switching, the transformer becomes susceptible to overvoltage generated within the system. Before a fault occurs, the power system is protected by measures such as instantaneous overcurrent protection and overcurrent protection (Florkowski et al., 2010). During the fault diagnosis process, techniques such as frequency analysis and preventive electrical tests are employed to monitor the voltage, current, and frequency characteristics of transformers. This facilitates fault detection and prediction, thereby prompting maintenance personnel to perform necessary repairs.
Grounding faults can occur in the high-voltage or low-voltage windings of a transformer, and the causes of such faults may include insulation aging, insulation material damage, equipment humidity, external damage, or operational errors. Grounding faults can potentially damage the equipment itself and the connected power grid. Currently, researchers often supplement traditional differential relays with Restricted Earth Fault (REF) relays to enhance transformer safety and reliability (Davarpanah et al., 2012; Ebadi et al., 2021). Due to variations in grounding locations, ground faults can give rise to issues such as localized discharge and overheating upon occurrence. Rapid detection of these faults can be achieved through methods such as dissolved gas analysis (DGA), infrared imaging, and electrical analysis (Sun et al., 2024; Vatsa and Hati, 2024).
A phase-to-phase short-circuit fault in a transformer can lead to significant issues such as temporary power interruptions and voltage instability. Therefore, it is crucial to promptly detect and rectify such faults upon occurrence. The primary causes of these faults include aging of the transformer’s insulation system, overcurrent, and mechanical deformation (Djufri and Yandra, 2020). Effective diagnosis of insulation failure and mechanical deformation in transformers can be achieved through methods such as dissolved gas analysis (DGA), vibration analysis, and sweep frequency analysis (Jiang et al., 2021; Kherif et al., 2021). Conducting rapid diagnosis before the occurrence of phase-to-phase short-circuit faults helps prevent major safety incidents and mitigate economic losses.
The power transformer is a vital component in the power system, where the iron core and winding play integral roles. Among transformer issues, turn-to-turn failures in the core and winding stand out as frequent causes of malfunctions. Out of 526 cases of transformer failures, Figure 2 highlights that winding-related failures were most common (Behjat and Vahedi, 2013). These failures often stem from inter-turn insulation aging, caused by various factors such as frequent overloading, mechanical vibration, high voltage stress, high current stress (especially during external short circuits), thermal overload, and contamination (Wang et al., 2002; PE, 2003). Hence, utilizing diagnostic techniques like vibration analysis, frequency response analysis, and polarization current analysis is essential for fault detection. These methods are capable of identifying critical issues such as mechanical deformation and insulation failure in transformers (Muhamad et al., 2013; Al-Ameri et al., 2021). Moreover, infrared imaging for detecting overheating also holds significant merit (Zhang and Li, 2021), given its non-contact nature for fault detection.
Power transformers are among the most critical components of power systems. Due to the high reliability requirements of power systems, transformers often need to operate continuously for extended periods, with limited opportunities for shutdown maintenance. Therefore, it is essential to continuously monitor the operational status of transformers throughout their service life to ensure reliable diagnosis and detection at the earliest sign of a fault. The aging and deterioration of transformer components are crucial aspects of fault detection, with these faults manifesting primarily through electrical and non-electrical characteristics. With advancements in technology, industrial standards have defined several diagnostic methods that employ chemical, electrical, and other testing techniques to provide a comprehensive diagnosis of transformers. Based on the concept of acquiring, analyzing, and processing partial discharge source characteristic parameters for fault diagnosis, various diagnostic methods have been developed. These methods include the analysis of polarization and depolarization currents, the analysis of dielectric breakdown voltage, and other diagnostic approaches based on the analysis of electric and non-electric gases, such as oil and gas analysis and vibration analysis (Eldery et al., 2006; Yang et al., 2009). The relationship between characteristic values and faults is thoroughly examined. Figure 3 provides a comprehensive overview of the partial discharge detection technique. Additionally, a rapid diagnosis of transformer faults is discussed (Soni and Mehta, 2021). The integration of these methods contributes to an effective understanding and identification of faults in transformers.
The polarization and depolarization current (PDC) technique stands as a cornerstone in electrical fault diagnosis. This method offers a non-destructive approach, capable of assessing the dielectric response of insulation systems in power transformers. Given its non-destructive nature, it excels in detecting insulation failure faults induced by factors like moisture, partial discharge, contamination, and overheating. Notably, it allows for the evaluation of oil-paper insulation and other supporting insulation joints even without opening the main tank (Mousavi et al., 2020). This feature presents a promising application prospect in assessing the water content of transformer insulating paper (Leibfried and Kachler, 2002; Hao et al., 2012). In PDC measurement, DC power is applied across the entire specimen for an extended duration. During this time, the current steadily increases as the polarization process begins. This occurs because various insulating components within the specimen react with distinct time constants. Through these observations, the specimen’s conductivity is measured, monitored, calculated, and characterized. The ultimate aim is to understand the insulation’s behavior and identify any abnormalities or partial discharges that might occur during the measurement process. The schematic diagram is shown in Figure 4.
Figure 4. Schematic diagram of PDC measuring device (A) and typical waveform (B) (Mousavi et al., 2020).
Based on the principle, researchers have commenced studying the correlation between PDC measurements and transformer faults. Talib et al. (2013) analyzed the dielectric response and conductivity of mineral insulation oil using the time-domain measurement technique of PDC. Their findings suggest that PDC measurements can detect overheating issues in transformer insulation oil. However, conducting PDC measurements comes with complexities due to limitations in equipment and methods. Factors such as oil conductivity, paper conductivity, water content, temperature, and insulation aging can significantly impact PDC test outcomes (Saha and Purkait, 2004; Liao et al., 2015). In practical fault diagnosis processes, it is essential to consider the influence of multiple factors. Flora et al. (2017) have conducted extensive experimental and case studies exploring these influencing factors. Their findings suggest that PDC could evolve into a powerful tool for long-term transformer insulation diagnosis if meticulous care is taken to mitigate the effects of these identified parameters. This study explores the feasibility of using PDC to detect the insulation condition of transformers and analyze specific fault types based on PDC results. On the other hand, PDC measurement and analysis is a time-consuming off-line process, and the longer processing time limits its application in transformer fault diagnosis to some extent (Mishra et al., 2018; Mishra et al., 2019). Dutta et al. (2020) addressed this issue by conducting research based on the mechanism of PDC measurements. They proposed estimating normalized depolarization charge by measuring shorter durations of polarization current, thereby reducing detection time. However, analyzing the faults that impact insulation anomalies through PDC requires highly demanding analytical models. Although estimation methods may enhance detection speed, they can adversely affect the precision and classification of fault diagnosis. Combining artificial intelligence algorithms with PDC is currently a significant direction in the advancement of this technology. By analyzing the impact of transformer parameters such as temperature, humidity, and conductivity using algorithms and training them accordingly, the efficiency and speed of PDC analysis can be effectively improved.
Transformer sweep frequency-response analysis (SFRA) is one of the important tests to determine the mechanical deformation of transformer windings. The method’s ability to discern subtle changes in the mechanical integrity of the transformer makes it a valuable tool for identifying issues related to winding conditions and facilitating proactive maintenance or corrective measures (Ludwikowski et al., 2012; Samimi et al., 2017). This method was originally proposed by Dick and Erven, (1978) where diagnostic results are derived by comparing the measured winding transfer function with reference measurements. However, this comparison introduces significant subjectivity, as the reference values are obtained from measurements on another transformer with a similar structure, making it difficult to assess the severity of the fault occurrence. It is evident that SFRA’s primary drawback lies in its subjectivity, as the diagnostic process heavily relies on engineers’ experience. To mitigate this, establishing commonly used criteria for interpretation becomes crucial. Bjelić et al. (2022) computed statistical fault indicators for various types of internal short-circuit faults in SFRA and introduced a new indicator based on reverberation time commonly utilized in acoustics. This allows for the utilization of low-cost measurement and signal processing equipment (Bjelić et al., 2022). Zhao et al.(2019) addressed the often-overlooked factors of capacitance and inductance in coil windings, establishing a comprehensive high-frequency circuit model for transformers, thereby enhancing SFRA analysis accuracy. Shamlou et al. (2021) introduced a fully automated technique for interpreting SFRA in power transformers, as depicted in Figure 5. Their approach combines digital image processing with evidence theory for analysis. Both simulation and experimental results indicate that this method achieves a high level of accuracy in fault reporting (Shamlou et al., 2021). Their approach circumvents the subjective diagnosis inherent in traditional methods, enhancing fault diagnosis efficiency by employing algorithmic techniques to analyze SFRA. Hence, within the context of leveraging efficient analysis methods like neural networks to optimize feature parameter analysis, the future trajectory of SFRA lies in employing artificial intelligence algorithms to interpret SFRA signals. This, combined with diverse comparison indicators, aims to mitigate the need for expert subjective judgment (Zhao et al., 2017; Abu-Siada and Aljohani, 2018).
Figure 5. The fully automated frequency signal analysis method (Shamlou et al., 2021).
When the mineral oil in the transformer is exposed to high heat and electrical stress, it breaks, and gas is produced as a result (Islam et al., 2018). Different forms of stress produce different gases, which can be divided into fault gases and non-fault gases. Fault gas is further divided into organic hydrogen (CH4, C2H6, C2H4, C2H2 and H2) and carbon oxide gas (CO2 and CO). The analysis of these fault gases and investigating their correlation with faults constitutes the Dissolved Gas Analysis (DGA) method used in transformer fault diagnosis. Its advantage lies in the ability to detect early occurrences of faults such as overheating, partial discharge, and breakdown in transformers. However, its disadvantage is the lack of accuracy and inability to detect and diagnose faults when the ratio codes fall outside the specified range (Rao et al., 2021). In early fault diagnosis, the amount of H2 and CO can be used to identify and diagnose early transformer faults according to temperature gas and key gas method (KGM) (Irwanto, 2021). However, this diagnostic method suffers from significant drawbacks due to the absence of clear indicators to confirm fault types. The type of gases detected depends on the severity of the specific transformer fault. With the progress of detection equipment and the development of DGA analysis, more mapping relationships between gas characteristic parameters and faults have been discovered, and more effective transformer fault analysis methods have been produced (Poonnoy et al., 2021), Such as Doernenburg Ratio Method (DRM) (Gouda et al., 2018), IEC Ratio Method (IRM) (Li et al., 2016) and Duval Triangle Method (DTM) (Barbosa et al., 2017). Bustamante et al. (2019) summarized and compared these methods, and summarized the characteristics of different gas analysis and diagnosis methods, as shown in Table 1.
Table 1. Comparison of dissolved gas analysis methods (Bustamante et al., 2019).
Compared to earlier methods like KGM, DTM offers a more precise fault diagnosis partitioning. It transitions from relying solely on the quantity and type of gases to utilizing the proportion of fault gases, thereby enhancing diagnostic accuracy. Rodríguez et al. (2021) assessed the gas production of three common transformer faults using different DGA diagnostic methods. The findings suggest that DTM is a more reliable approach for diagnosing faults in natural ester liquids. Lakehal and Tachi, (2017) developed a Bayesian model incorporating DTM, highlighting its advantages in fault detection, and successfully applied it to five power transformers. The utilization of DTM proves effective in identifying localized discharge, thermal faults, and electrical faults in transformers (Golarz, 2016; Wattakapaiboon and Pattanadech, 2016; Pattanadech et al., 2019; Pattanadech and Wattakapaiboon, 2019). However, DTM operates as a closed diagnostic system, providing results regardless of the presence of all gases. It resides at the boundary of two fracture zones, unable to pinpoint the actual fault zone, posing a limitation. Therefore, the Duval Pentagon Method (DPM) was developed to enhance the accuracy of DGA. By integrating two Duval pentagons without interference, DPM can identify different types of faults from the same set of DGA data, significantly improving the range of faults identified compared to DTM (Pattanadech et al., 2019; Pattanadech and Wattakapaiboon, 2019). Pattanadech and Wattakapaiboon, (2019) examined the performance of different DGA analysis methods, applying DTM and DPM to specific cases. The results showed that DPM exhibited the best consistency and could identify transformer insulation aging that DTM could not detect. Building upon DPM, Gouda et al. proposed the Seven Pentagon Method based on the seven fault gases produced in transformer faults, as illustrated in Figure 6. Their research added CO2 and CO to the five gases in DPM, enabling the differentiation between cellulose insulation faults caused by heating and transformer faults, thereby enhancing the accuracy of fault diagnosis for specific transformer types. However, excessive classification makes it challenging to determine subcategories of faults. Artificial intelligence algorithms have shown promising applications in analyzing data and correlations between faults, suggesting that combining artificial intelligence algorithms with DPM and other DGA analysis methods is the primary direction for current and future developments (Sutikno et al., 2024).
Figure 6. The fault type regions of the Seven Pentagon Method (Gouda et al., 2018).
Mechanical faults, insulation faults, and other issues can all affect the vibrations of the windings and core in a transformer. Hence, conducting vibration analysis enables the diagnosis of transformer faults (Granados-Lieberman et al., 2023). In comparison to traditional fault detection methods, vibration analysis offers distinct advantages in terms of electrical isolation. It stands out as an efficient and reliable non-invasive technique for detecting faults in transformer windings (Bagheri et al., 2018). Despite this, vibration-based transformer diagnostic technology has not gained widespread usage and discussion compared to other approaches. Part of the reason for this issue is the lack of unique interpretation methods for vibration signals. Another part is the challenging nature of noise removal from vibration signals and the complex effects various factors have on vibrations (Yang et al., 2004).
Vibration analysis emerged in early transformer fault diagnosis due to its non-electrical nature and non-invasive measurement approach (Borucki et al., 2015). Bartoletti et al. (2004) employed experiments and field tests to distinguish faulty transformers. They mitigated the impact of noise on fault analysis to some extent by constraining the frequency range of vibration sources and integrating them with voltage signals. However, the presence of considerable noise and various influencing factors affected the diagnosis. Subsequent researchers have sought different avenues to enhance diagnostic accuracy. Borucki, (2012) introduced an improved vibro-acoustic method known as Mechanical Vibration Measurement (MVM), utilizing transient operational conditions of transformers to assess the status of windings and cores. This approach circumvents the influence of the measured object’s current load on vibration acoustic signals, thereby enhancing diagnostic accuracy. Meanwhile, Zheng et al. (2015) employed laser Doppler vibrometry for non-contact measurement of transformer vibration signals and established a winding vibration analysis system based on laser measurement. Both Borucki’s and Zheng’s studies aimed to bolster vibration analysis accuracy by mitigating influencing factors in the measurement process, thereby reducing errors at the source. Shen et al. (2015) established and analyzed the relationship model between the surface vibration of the fuel tank and the insulation weakening of transformer windings. By monitoring the vibration signal of the tank and integrating information about the load current, as well as the normal and fault states of the transformer, the fundamental frequency signal of the transformer tank’s surface vibration is computed. His research focused on the impact of transformer current signals on vibration signals, aiming to enhance diagnostic accuracy through model analysis. Zhang et al. enhanced the vibration analysis method through mathematical statistical techniques. They analyzed by comparing the slope value of the cumulative probability distribution curve of transformer vibration signals, as illustrated in Figure 7. This analysis allows for the examination of the energy spectrum of the signals, enabling the rapid and accurate identification of the short-circuit fault degree in transformer windings (Zhang et al., 2019). Algorithmic optimization of vibration analysis itself has been conducted, resulting in improved diagnostic accuracy and efficiency.
Figure 7. Cumulative probability distribution curve of vibration signal under different working state of transformer (Zhang et al., 2019).
While the efforts of the researchers have propelled the advancement of vibration analysis, their focus has predominantly centered on dry-type transformers, rendering their findings potentially inapplicable to oil-immersed transformers. Addressing this gap, Miao et al. (2023) conducted a comprehensive study on the vibration signals of oil-immersed transformers. They established a three-dimensional electromagnetic-mechanical-acoustic coupling analysis model specifically tailored for oil-immersed power transformers. Their research primarily concentrated on scrutinizing the vibration characteristics unique to oil-immersed transformers, thereby further fostering the evolution of vibration analysis within the domain of transformer diagnostics.
As transformer technology progresses, there emerges a heightened demand for the precision of vibration analysis techniques. While many researchers have contributed to enhancing diagnostic accuracy to some extent in their respective studies, the varied sources of vibration signals and the diverse array of transformer models impose distinct impacts on vibration signals. Consequently, there remains a pressing need for further research and standardization within the realm of transformer vibration analysis. The integration of artificial intelligence algorithms with vibration analysis techniques represents a pivotal direction for the advancement of this technology. Such integration enables the swift analysis of diverse factors influencing vibrations, facilitates noise removal and signal delineation, and promises significant advancements in the underlying technology itself (Xu et al., 2010).
Chapter 3 provides an exposition on the evolution of different diagnostic techniques. As transformer technology advances and the electricity market develops, relying solely on diagnostic techniques proves insufficient for achieving rapid fault diagnosis and adapting to the complex realities of the modern transformer industry. A multitude of influencing factors, along with the increasingly intricate data analysis processes, have compromised the reliability of fault diagnosis, thereby impeding transformer progress (Wani et al., 2021). The development of artificial intelligence (AI) technology has simplified data analysis and prediction. Even in the absence of structural information, AI can derive relatively accurate models through training, addressing the challenging multifactorial analysis inherent in traditional methods. To further enhance the accuracy and efficiency of transformer fault diagnosis, the integration of AI technology into transformer fault diagnosis represents the primary direction of current development. This aims to achieve intelligent diagnosis of transformers, enabling them to adapt to the evolving demands of the industry. This chapter categorizes different AI technologies and combines intelligent analysis methods with traditional diagnostic techniques to discuss the development of intelligent transformer diagnosis technology.
Neural networks are artificial intelligence models inspired by the biological nervous system. Through training, neural networks learn the features and patterns of input data, enabling them to perform various tasks such as classification, regression, and clustering. Neural networks possess strengths such as strong adaptability, parallel processing capabilities, and robust generalization abilities, making them suitable for application in transformer fault diagnosis. Many researchers utilize neural network technology for the analysis and diagnosis of transformer faults, such as Radial Basis Function (RBF) neural networks (Mi et al., 2021), Probabilistic Neural Networks (PNN) (Yi et al., 2016), Elman neural networks (Li et al., 2022), and more. PNN demonstrates robustness, performing well in scenarios with limited sample data and high levels of noise interference. Consequently, it has been widely adopted by researchers in the field of transformer fault diagnosis. For instance, Bigdeli et al. (2013) applied vector fitting and PNN in transfer function analysis to identify winding faults in transformers, significantly improving diagnostic accuracy compared to traditional methods. Zhang et al. proposed a fault diagnosis method based on the vibration and noise characteristics of transformers, using PNN to predict transformer faults based on vibration and noise signals under different fault conditions (Zhou et al., 2023). Their approach can be applied to various types of transformers, enhancing the adaptability of vibration analysis techniques. However, the performance of PNN is significantly influenced by the smoothing factor of its hidden layer elements, which may reduce fault classification accuracy when applied in other diagnostic techniques.
To further enhance the accuracy of intelligent diagnostic methods, Yang et al. (2019) conducted research on intelligent diagnosis methods for Dissolved Gas Analysis (DGA). They employed the Bat Algorithm (BA) to optimize the smoothing factor, effectively mitigating the drawback of PNN’s susceptibility to local optima. The diagnostic method of BA-PNN is illustrated in Figure 8. When evaluated using real-world cases, BA-PNN demonstrated exceptional accuracy, achieving an accuracy rate of approximately 98%. Velásquez and Lara, (2020) proposed a new method with the lowest computational cost, utilizing a genetic algorithm to optimize an Artificial Neural Network (ANN) classifier for fault classification. They replaced the traditional Reinforcement Learning (RL) action selection process with a genetic algorithm-based optimizer. Their research, based on the combination of traditional diagnostic methods and neural network technology, further enhanced the accuracy of intelligent diagnosis through algorithm optimization and tailored design for transformer fault diagnosis scenarios. It is evident that different neural network training algorithms encounter various challenges. Therefore, it is necessary to employ appropriate optimization methods to address issues like local non-convergence and the significant impact of a small number of anomalous samples.
Figure 8. Transformer diagnosis method based on BA-PNN (Yang et al., 2019).
However, it is imperative to recognize that transformers exhibit diverse characteristics across various aspects following a fault occurrence. Integrating multiple diagnostic methods through artificial intelligence (AI) technology stands as a pivotal direction for the advancement of intelligent diagnostic techniques. Wang et al. (2014) proposed a transformer fault diagnosis method based on multi-source information fusion, incorporating SCADA data, dissolved gas sensor data, relevant electrical test data, operational maintenance records, and more. Employing a time-space weighted fusion method using BP neural networks, this intelligent approach significantly enhances the accuracy of transformer fault diagnosis. Xing and He, (2023) employed a one-dimensional convolutional neural network for extracting dissolved gas features and a deep residual compression activation neural network for extracting infrared image features, presenting a power transformer health assessment method based on multi-modal mutual neural networks. By amalgamating DGA with the infrared imaging method and utilizing multi-modal mutual neural networks for training, this method achieves high classification accuracy and precision, albeit with a longer training duration. In forthcoming developments, adopting intelligent diagnostic techniques that amalgamate multi-source information is imperative. Enhancing algorithm adaptability to complex sample data and real-world applications will be critical for advancing neural network technology in the field of intelligent diagnostics.
Deep learning, with its capability for performing more layers of nonlinear operations, has transcended the depth limitations of traditional neural networks. The features learned by deep learning models demonstrate a higher level of representativeness of raw data, significantly easing classification and visualization tasks. Consequently, deep learning has garnered increasing favor among researchers in the realm of transformer fault diagnosis (Liu et al., 2022; Jimenez-Navarro et al., 2023). Duan et al. (2019) employed deep learning to analyze inter-turn short-circuit faults in transformers, optimizing the input characteristics of three-phase transformer signals and thereby enhancing the robustness of inter-turn fault detection within the deep learning framework. Zhang et al. (2020a) proposed a transformer fault diagnosis method based on monitoring systems and integrated machine learning approaches. Liang et al. (2018) introduced a deep belief network (DBN) based on a deep genetic algorithm, which automatically establishes mapping relationships between feature gases and fault types, achieving accurate diagnosis.
Their studies have demonstrated that the integration of deep learning into transformer intelligent diagnostic methods results in enhanced accuracy. Nonetheless, they have overlooked the impact of noise and uncertainty factors on measurement data. In practical applications, sample data often exhibit issues such as imbalance, scarcity, and poor quality. Further research is required to improve the accuracy of intelligent diagnostic methods. De Andrade Lopes et al. (2021) employed the Borderline Synthetic Minority Over-Sampling Technique (SMOTE) for oversampling to balance the dataset. Their deep neural network, which integrates the Borderline SMOTE method with traditional DGA methods, artificial neural networks, and deep neural networks trained on imbalanced data, demonstrated the highest diagnostic accuracy. Zhang et al. (2020b) proposed a cost-sensitive learning-based one-dimensional convolutional neural network (1D CNN) model, optimizing its cost matrix using the particle swarm algorithm. This model places greater emphasis on minority fault types. Xing et al. (2023) introduced a deep noise-filtering diagnostic model based on noise vibration signals. They employed the balanced isolation forest method to detect abnormal data in the original vibration signals and used two deep noise filtering networks to suppress noise levels, effectively reducing the impact of abnormal sample data on diagnostic results. Vatsa et al. (2024) developed an intelligent diagnostic technique based on residual long short-term memory (LSTM) networks to analyze FDC. The structure of the system is illustrated in Figure 9. They utilized the Monte Carlo dropout prediction method to estimate uncertainty in polarizing current predictions. This approach not only addresses the inherent time-consuming nature of PDC measurements leading to vulnerable data but also further mitigates the impact of uncertainty factors on diagnostic results, significantly enhancing diagnostic accuracy.
Figure 9. The architecture of the residual LSTM model (Vatsa et al., 2024).
The researchers have effectively enhanced the precision of intelligent diagnostic techniques through the optimization of deep learning methods and denoising of measurement features, thus increasing the adaptability of intelligent diagnostic approaches to complex operational environments. Moreover, some researchers have further improved diagnostic accuracy and adaptability by integrating multiple sources of information. Zhang et al. (2020c) monitored and collected information on transformer faults, coupled with variations in transformer temperature and electrical signals, to establish a computational model for transformers based on multi-level faults and multiple feature parameters. By employing the Deep Belief Network Identification (DBNI) algorithm for fault detection and utilizing an optimal fusion algorithm to construct training samples for the transformer diagnostic model, they achieved improved diagnostic accuracy through joint analysis of two sets of feature information. Meanwhile, Liu et al. (2023) identified insufficient sample data and uneven distribution of collected data among faults as crucial factors limiting the application of machine learning in transformer fault detection. Therefore, they developed a power transformer fault warning system based on electrical quantities and vibration signals, incorporating multi-source information processing to simultaneously enhance cost-effectiveness and fault warning accuracy. Their research indicates that intelligent diagnostic methods integrating oil and gas features, electrical characteristics, vibration features, and other information can significantly improve data utilization efficiency and diagnostic accuracy, thereby showing promising development prospects in the field of deep learning technology capable of handling more complex feature sets.
SVM has also been widely applied in fault diagnosis to enhance the accuracy of fault classification. SVM is an effective method for handling high-dimensional independent variables without the need to recalculate from initial conditions to obtain new decision boundaries (Kim et al., 2019). However, the classification accuracy of an individual SVM may not reach optimal levels. Many researchers combine other intelligent methods with SVM to effectively improve classification performance and achieve convincing results. Illias and Zhaoliang, (2018) proposed a hybrid SVM algorithm based on an improved Evolutionary Particle Swarm Optimization (EPSO) algorithm to determine transformer fault types. EPSO combines particle swarm optimization with an evolutionary strategy, maintaining the superior characteristics of particles by introducing variation operations. To enhance the algorithm’s robustness, they introduced Time-Varying Acceleration Coefficients (TVAC) based on EPSO. Finally, the SVM-MEPSO-tvac method, a combination of MEPSO and SVM, not only reduced training time but also maintained high accuracy, providing a solution and insight for transformer fault type identification based on DGA data in practical applications. Hong et al. (2015) established a probability-based real-time detection and classification model for power transformer faults, combining SVM with vibration analysis. They categorized the transformer operating states into healthy, aging, and abnormal, then performed SVM classification associated with the sigmoid function. Through this method, they estimated the membership probability of each class of the binary decision tree.
However, SVM-based approaches also exhibit certain limitations, such as a tendency to misclassify samples near the decision boundary, which can reduce diagnostic accuracy (Zhang et al., 2020d). Therefore, many researchers have optimized the performance of intelligent transformer fault diagnosis methods by selecting the most relevant feature sets (Kari et al., 2018). They propose selecting feature subsets and employing intelligent algorithms to optimize the SVM method for transformer fault diagnosis. Hong et al. (2022) addressed the issue of fuzzy decision boundaries in SVM by proposing a Support Vector Feature-based Parameter Optimization Algorithm (SVFB). This not only shortened training time but also improved diagnostic accuracy. Zhu et al. (2022) optimized SVM using an Improved Seagull Optimization Algorithm (ISOA) and introduced the Henon chaotic map for population initialization. By combining Differential Evolution (DE) based on an adaptive formula, they improved the foraging formula of the Seagull Optimization Algorithm (SOA), enhancing algorithm diversity and the ability to find optimal SVM parameters. Hendel et al. (2023) applied Dempster-Shafer fusion to the results returned by Multi-class Support Vector Machine (M-SVM) to enhance accuracy and decision support. They also proposed an output post-processing method to address evidence conflict issues.
In the integration of SVM technology with transformer fault diagnosis, researchers have capitalized on the advantages of rapid training and minimal sample requirements. Simultaneously, they have addressed its drawbacks of low accuracy and subpar classification. Through algorithmic optimizations and other approaches, they have advanced the application of SVM technology in the domain of intelligent transformer fault diagnosis.
Incorporating intelligent algorithms for further analysis and optimization of traditional transformer fault diagnosis techniques significantly enhances both the applicability and diagnostic accuracy of conventional methods. However, faults often present in various forms, and detecting their abnormal characteristics from multiple perspectives yields superior diagnostic outcomes (Cao et al., 2020). Historically, utilizing features from different sources for fault diagnosis posed significant challenges due to their complex relationships and increased errors. Nevertheless, artificial intelligence algorithms excel in managing the intricate relationship between faults and features, as well as mitigating the negative impact of uncertainties inherent in measurements. Therefore, integrating the concept of multi-source information fusion into intelligent diagnosis technology stands as a key developmental trajectory.
Ni et al. combined Case-Based Reasoning (CBR) with Rule-Based Reasoning (RBR) and established a rapid transformer fault diagnosis system based on this approach (Ni et al., 2020). The system divides the structural characteristics and fault modes of equipment such as winding, core, bushing, on-load tap changer, cooling system, and non-electrical protection. Although multiple features are jointly analyzed for fault detection, there has been a lack of analysis regarding the relationship between faults and these features. Consequently, diagnostic outcomes rely heavily on the provided fault cases. Xiao et al. (2020) utilized Bayesian networks to establish the correlation between faults and various diagnostic tests. Expanding on this framework, they also considered factors such as insulation resistance, dielectric loss tangent, oil gas content, power frequency voltage, and leakage current. This approach notably improved the efficiency of on-site maintenance and fault identification. Their study comprehensively integrated multiple tests, employing Bayesian network diagnostic methods for the fusion of multi-source information, offering valuable guidance in this area. Luo et al. (2021) proposed an intelligent mining and early warning algorithm for transformer operation faults. This algorithm collects data from various dimensions, including electrical variables, non-electrical variables, and multiple source channels of the transformer. The framework of the intelligent mining algorithm is illustrated in Figure 10. The core of this framework lies in collecting a large amount of diverse, multi-dimensional, and heterogeneous data. By integrating the Pearson product-moment algorithm and Gran causality relationship algorithm, it ensures the inclusion of a substantial amount of electrical and non-electrical variable data. The correlation also ensures causality relationships. Therefore, it can analyze the intrinsic mechanisms between faults and feature quantities, significantly enhancing the reliability of fault warnings. His research effectively circumvented the drawbacks of the black-box nature of artificial intelligence algorithms, providing explanations for the fault diagnosis process under intelligent diagnostic technology.
Figure 10. Intelligent mining algorithm framework (Luo et al., 2021).
The transformer intelligent diagnosis method based on multi-source information fusion integrates multiple fault diagnosis approaches. While using diverse types of information for diagnosis mitigates the reliance on sample data inherent in traditional intelligent diagnosis algorithms and enhances diagnostic accuracy, the complexity of fault features and classification processes increases the difficulty of model training. Therefore, future development should emphasize the careful selection of fault features and the optimization of training models. Additionally, the scale of transformer intelligent diagnosis models should be tailored to different operational environments. In practical applications, the design of diagnosis models should account for the required accuracy and scale of fault diagnosis.
Transformers are a crucial component of power systems, and transformer fault diagnosis is essential for maintaining the stable operation of the power grid. This paper provides a comprehensive discussion on the development of transformer fault diagnosis techniques. Firstly, it introduces the types and causes of transformer faults. Then, it analyzes the evolution of traditional fault diagnosis methods from the perspectives of electrical and non-electrical detection. Given issues such as complex influencing factors and lengthy measurement analysis times, different researchers have advanced PDC development by reducing polarization current measurement time and integrating artificial intelligence algorithms to optimize the analysis process. Early DGA methods had problems like incomplete coding and incorrect fault identification. With the advancement of detection equipment and technology, new methods like DTM and DPM were proposed, and neural network model training was used to improve DGA analysis accuracy. Refining the relationship between transformer fault classification and characteristic gas ratios has become a major focus in DGA development. It is evident that traditional diagnostic methods are limited by increasingly complex fault characteristics, difficulty in improving detection accuracy, and complex fault analysis. Consequently, more researchers are combining neural networks, machine learning, and other AI technologies with traditional methods. These intelligent algorithms excel in data analysis and feature classification, addressing issues such as low correlation between characteristic parameters and faults, vague fault descriptions, and difficult feature analysis in traditional fault diagnosis. This significantly enhances the accuracy of transformer fault diagnosis and promotes the development of the transformer industry.
In the process of integrating AI into transformer fault diagnosis methods, intelligent algorithms also face challenges like difficulty in convergence, long training times, and high sensitivity to sample data. To solve these issues and further improve the accuracy and practicality of intelligent transformer diagnosis technology, researchers are combining multiple intelligent algorithms to leverage their strengths. Additionally, some researchers are using mathematical analysis to preprocess and denoise fault sample data, thereby reducing the negative impact of anomalous samples on the diagnostic model training. On the other hand, integrating various fault parameters such as electrical parameters, vibration parameters, and oil gas parameters, and using AI algorithms to establish models relating multi-source fault features to fault types, is also a significant direction of current development. This approach not only avoids diagnostic accuracy issues caused by inherent sample data errors but also provides a more comprehensive consideration, leading to more precise fault diagnosis. However, this also increases the complexity of the algorithms to some extent. Therefore, in the future development of transformer fault diagnosis, the optimization of algorithms and the enhancement and intelligentization of fault diagnosis technology will be crucial.
HC: Conceptualization, Data curation, Formal Analysis, Investigation, Methodology, Project administration, Software, Supervision, Validation, Writing–original draft, Writing–review and editing. CZ: Conceptualization, Data curation, Formal Analysis, Investigation, Methodology, Software, Supervision, Writing–original draft, Writing–review and editing. YM: Methodology, Project administration, Software, Supervision, Validation, Visualization, Writing–review and editing. JS: Project administration, Resources, Supervision, Validation, Visualization, Writing–review and editing. XX: Formal Analysis, Funding acquisition, Project administration, Resources, Validation, Visualization, Writing–review and editing.
The author(s) declare that financial support was received for the research, authorship, and/or publication of this article. This work was supported by Science and Technology Project of State Grid Corporation of China (J2023145).
The funder was not involved in the study design, collection, analysis, interpretation of data, the writing of this article, or the decision to submit it for publication.
Author HC was employed by State Grid Jiangsu Electric Power Company. Authors CZ, YM, JS, and XX were employed by State Grid Suzhou Power Supply Company.
All claims expressed in this article are solely those of the authors and do not necessarily represent those of their affiliated organizations, or those of the publisher, the editors and the reviewers. Any product that may be evaluated in this article, or claim that may be made by its manufacturer, is not guaranteed or endorsed by the publisher.
Abu-Siada, A., and Aljohani, O. (2018). Detecting incipient radial deformations of power transformer windings using polar plot and digital image processing. Iet Sci. Meas. Technol. 12 (4), 492–499. doi:10.1049/iet-smt.2017.0412
Al-Ameri, S. M., Kamarudin, M. S., Yousof, M. F. M., Salem, A. A., Siada, A. A., and Mosaad, M. I. (2021). Interpretation of frequency response analysis for fault detection in power transformers. Appl. Sci. 11 (7), 2923. doi:10.3390/app11072923
Awadallah, S. K. E., Milanovic, J. V., and Jarman, P. N. (2015). The influence of modeling transformer age related failures on system reliability. IEEE Trans. Power Syst. 30 (2), 970–979. doi:10.1109/TPWRS.2014.2331103
Badawi, M., Ibrahim, S. A., Mansour, D.-E. A., El-Faraskoury, A. A., Ward, S. A., Mahmoud, K., et al. (2022). Reliable estimation for health index of transformer oil based on novel combined predictive maintenance techniques. IEEE Access 10, 25954–25972. doi:10.1109/ACCESS.2022.3156102
Bagheri, M., Zollanvari, A., and Nezhivenko, S. (2018). Transformer fault condition prognosis using vibration signals over cloud environment. IEEE Access 6, 9862–9874. doi:10.1109/ACCESS.2018.2809436
Barbosa, T. M., Ferreira, J. G., Finocchio, M. a. F., and Endo, W. (2017). Development of an application based on the duval triangle method. IEEE Lat. Am. Trans. 15 (8), 1439–1446. doi:10.1109/TLA.2017.7994790
Barkas, D. A., Chronis, I., and Psomopoulos, C. (2022). Failure mapping and critical measurements for the operating condition assessment of power transformers. Energy Rep. 8, 527–547. doi:10.1016/j.egyr.2022.07.028
Bartoletti, C., Desiderio, M., Di Carlo, D., Fazio, G., Muzi, F., Sacerdoti, G., et al. (2004). Vibro-acoustic techniques to diagnose power transformers. IEEE Trans. Power Deliv. 19 (1), 221–229. doi:10.1109/TPWRD.2003.820177
Behjat, V., and Vahedi, A. (2013). An experimental approach for investigating low-level interturn winding faults in power transformers. Electr. Eng. 95 (2), 135–145. doi:10.1007/s00202-012-0248-4
Bigdeli, M., Vakilian, M., and Rahimpour, E. (2013). A probabilistic neural network classifier-based method for transformer winding fault identification through its transfer function measurement. Int. Trans. Electr. Energy Syst. 23 (3), 392–404. doi:10.1002/etep.668
Bjelić, M., Brković, B., Žarković, M., and Miljković, T. (2022). Fault detection in a power transformer based on reverberation time. Int. J. Electr. Power Energy Syst. 137, 107825. doi:10.1016/j.ijepes.2021.107825
Borucki, S. (2012). Diagnosis of technical condition of power transformers based on the analysis of vibroacoustic signals measured in transient operating conditions. IEEE Trans. Power Deliv. 27 (2), 670–676. doi:10.1109/TPWRD.2012.2185955
Borucki, S., Cichon, A., and Boczar, T. (2015). Diagnostics of the core mechanical construction based on vibroacoustic measurements and distribution of temperature field in transformer magnetic circuit. Acta Phys. Pol. A 128 (3), 306–310. doi:10.12693/aphyspola.128.306
Bustamante, S., Manana, M., Arroyo, A., Castro, P., Laso, A., and Martinez, R. (2019). Dissolved gas analysis equipment for online monitoring of transformer oil: a review. Sensors 19 (19), 4057. doi:10.3390/s19194057
Cao, C., Xu, B., and Li, X. (2020). Monitoring method on loosened state and deformational fault of transformer winding based on vibration and reactance information. IEEE Access 8, 215479–215492. doi:10.1109/ACCESS.2020.3039529
Chakravorti, S., Dey, D., and Chatterjee, B. (2013). Robust control in power systems. Germany: Springer Science and Business Media.
Christina, A., Salam, M., Rahman, Q., Wen, F., Ang, S., and Voon, W. (2018). Causes of transformer failures and diagnostic methods–A review. Renew. Sust. Energy Rev. 82, 1442–1456. doi:10.1016/j.rser.2017.05.165
Davarpanah, M., Sanaye-Pasand, M., and Iravani, R. (2012). Performance enhancement of the transformer restricted earth fault relay. IEEE Trans. Power Deliv. 28 (1), 467–474. doi:10.1109/TPWRD.2012.2208204
De Andrade Lopes, S. M., Flauzino, R. A., and Altafim, R. C. (2021). Incipient fault diagnosis in power transformers by data-driven models with over-sampled dataset. Electr. Power Syst. Res. 201, 107519. doi:10.1016/j.epsr.2021.107519
Dick, E., and Erven, C. (1978). Transformer diagnostic testing by frequuency response analysis. IEEE Trans. Power App. Syst. (6), 2144–2153. doi:10.1109/tpas.1978.354718
Divya, S., Panda, S., Hajra, S., Jeyaraj, R., Paul, A., Park, S. H., et al. (2023). Smart data processing for energy harvesting systems using artificial intelligence. Nano Energy 106, 108084. doi:10.1016/j.nanoen.2022.108084
Djufri, U., and Yandra, F. E. (2020). The design programming simulation as a reference for the adjustment of the relay GFR on the protection of the airway medium voltage 20 KV. England: IOP Publishing.
Duan, L., Hu, J., Zhao, G., Chen, K., Wang, S. X., and He, J. (2019). Method of inter-turn fault detection for next-generation smart transformers based on deep learning algorithm. High. Volt. 4 (4), 282–291. doi:10.1049/hve.2019.0067
Duraisamy, V., Devarajan, N., Somasundareswari, D., Vasanth, A. M., and Sivanandam, S. (2007). Neuro fuzzy schemes for fault detection in power transformer. Appl. Soft Comput. 7 (2), 534–539. doi:10.1016/j.asoc.2006.10.001
Dutta, S., Mishra, D., Baral, A., and Chakravorti, S. (2020). Estimation of de-trapped charge for diagnosis of transformer insulation using short-duration polarisation current employing detrended fluctuation analysis. High. Volt. 5 (5), 636–641. doi:10.1049/hve.2019.0348
Ebadi, A., Hosseini, S. M., and Abdoos, A. A. (2021). A new time-frequency analysis based supervision method for the transformer restricted ground fault relay. Int. J. Electr. Power Energy Syst. 129, 106858. doi:10.1016/j.ijepes.2021.106858
Eldery, M. A., Abdel-Galil, T. K., El-Saadany, E. F., and Salama, M. M. A. (2006). Identification of partial discharge locations in transformer winding using PSD estimation. IEEE Trans. Power Deliv. 21 (2), 1022–1023. doi:10.1109/TPWRD.2005.864067
El-Kenawy, E.-S. M., Albalawi, F., Ward, S. A., Ghoneim, S. S., Eid, M. M., Abdelhamid, A. A., et al. (2022). Feature selection and classification of transformer faults based on novel meta-heuristic algorithm. Mathematics 10 (17), 3144. doi:10.3390/math10173144
Flora, S. D., Divekar, M. S., and Rajan, J. S. (2017). Factors affecting polarization and depolarization current measurements on insulation of transformers. IEEE Trans. Dielectr. Electr. Insul. 24 (1), 619–629. doi:10.1109/TDEI.2016.005944
Florkowski, M., Furgal, J., and Pajak, P. (2010). Risks of transformers from switching overvoltages in electrical power systems. Prz. Elektrotechniczn 86 (5), 245–248.
Fu, Q., Zhu, J., Mao, Z.-H., Zhang, G., and Chen, T. (2017). Online condition monitoring of onboard traction transformer core based on core-loss calculation model. IEEE Trans. Ind. Electron. 65 (4), 3499–3508. doi:10.1109/TIE.2017.2758721
Golarz, J. (2016). Understanding dissolved gas analysis (DGA) techniques and interpretations. IEEE, 1–5. doi:10.1109/TDC.2016.7519852
Gouda, O. E., El-Hoshy, S. H., and El-Tamaly, H. H. (2018). Proposed heptagon graph for DGA interpretation of oil transformers. IET Gener. Transm. Dis. 12 (2), 490–498. doi:10.1049/iet-gtd.2017.0826
Gouda, O. E., El-Hoshy, S. H., and L-Tamaly, H. H. E. (2018). Proposed three ratios technique for the interpretation of mineral oil transformers based dissolved gas analysis. IET Gener. Transm. Dis. 12 (11), 2650–2661. doi:10.1049/iet-gtd.2017.1927
Granados-Lieberman, D., Huerta-Rosales, J. R., Gonzalez-Cordoba, J. L., Amezquita-Sanchez, J. P., Valtierra-Rodriguez, M., and Camarena-Martinez, D. (2023). Time-frequency analysis and neural networks for detecting short-circuited turns in transformers in both transient and steady-state regimes using vibration signals. Appl. Sci. 13 (22), 12218. doi:10.3390/app132212218
Hao, J., Liao, R., Chen, G., Ma, Z., and Yang, L. (2012). Quantitative analysis ageing status of natural ester-paper insulation and mineral oil-paper insulation by polarization/depolarization current. IEEE Trans. Dielectr. Electr. Insul. 19 (1), 188–199. doi:10.1109/tdei.2012.6148518
Hashemnia, N., Abu-Siada, A., and Islam, S. (2016). Detection of power transformer bushing faults and oil degradation using frequency response analysis. IEEE Trans. Dielectr. Electr. Insul. 23 (1), 222–229. doi:10.1109/TDEI.2015.005032
Hendel, M., Meghnefi, F., Senoussaoui, M. E. A., Fofana, I., and Brahami, M. (2023). Using generic direct M-SVM model improved by kohonen map and dempster–shafer theory to enhance power transformers diagnostic. Sustainability 15 (21), 15453. doi:10.3390/su152115453
Hong, K., Huang, H., Zhou, J., Shen, Y., and Li, Y. (2015). A method of real-time fault diagnosis for power transformers based on vibration analysis. Meas. Sci. Technol. 26 (11), 115011. doi:10.1088/0957-0233/26/11/115011
Hong, L., Chen, Z., Wang, Y., Shahidehpour, M., and Wu, M. (2022). A novel SVM-based decision framework considering feature distribution for Power Transformer Fault Diagnosis. Energy Rep. 8, 9392–9401. doi:10.1016/j.egyr.2022.07.062
Illias, H. A., and Zhaoliang, W. (2018). Identification of transformer fault based on dissolved gas analysis using hybrid support vector machine-modified evolutionary particle swarm optimisation. Plos ONE 13 (1), e0191366. doi:10.1371/journal.pone.0191366
Irwanto, I. (2021). Failure of 150 KV power transformer in indication of dissolved gas analysis test in total dissolved combustile gas (TDCG) method. Int. J. Eng. Educ. 6 (1). doi:10.30870/vanos.v6i1.10516
Islam, M. M., Lee, G., and Hettiwatte, S. N. (2018). A review of condition monitoring techniques and diagnostic tests for lifetime estimation of power transformers. Electr. Eng. 100, 581–605. doi:10.1007/s00202-017-0532-4
Jiang, P., Zhang, Z., Dong, Z., Wu, Y., Xiao, R., Deng, J., et al. (2021). Research on distribution characteristics of vibration signals of ±500 kV HVDC converter transformer winding based on load test. Int. J. Electr. Power Energy Syst. 132, 107200. doi:10.1016/j.ijepes.2021.107200
Jimenez-Navarro, M. J., Martinez-Ballesteros, M., Martinez-Alvarez, F., and Asencio-Cortes, G. (2023). A new deep learning architecture with inductive bias balance for transformer oil temperature forecasting. J. Big Data 10 (1), 80. doi:10.1186/s40537-023-00745-0
Jina, E., Zhang, Y., He, W., Zhang, W., Cao, Y., and Lv, G. (2024). A new method for oil-immersed transformers fault diagnosis based on evidential reasoning rule with optimized probabilistic distributed. IEEE Access 12, 34289–34305. doi:10.1109/ACCESS.2024.3370866
Kari, T., Gao, W., Zhao, D., Abiderexiti, K., Mo, W., Wang, Y., et al. (2018). Hybrid feature selection approach for power transformer fault diagnosis based on support vector machine and genetic algorithm. IET Gener. Transm. Dis. 12 (21), 5672–5680. doi:10.1049/iet-gtd.2018.5482
Kherif, O., Benmahamed, Y., Teguar, M., Boubakeur, A., and Ghoneim, S. S. (2021). Accuracy improvement of power transformer faults diagnostic using KNN classifier with decision tree principle. IEEE Access 9, 81693–81701. doi:10.1109/ACCESS.2021.3086135
Kim, S. W., Kim, S. J., Seo, H. D., Jung, J. R., Yang, H. J., and Duval, M. (2013). New methods of DGA diagnosis using IEC TC 10 and related databases Part 1: application of gas-ratio combinations. IEEE Trans. Dielectr. Electr. Insul. 20 (2), 685–690. doi:10.1109/tdei.2013.6508773
Kim, Y., Park, T., Kim, S., Kwak, N., and Kweon, D. (2019). Artificial intelligent fault diagnostic method for power transformers using a new classification system of faults. J. Electr. Eng. Technol. 14, 825–831. doi:10.1007/s42835-019-00105-0
Kumar, A., Singh, S. K., and Husain, Z. (2015). Root-cause analysis of transformer failure scenario at power sub-station. Adv. Environ. Agric. Sci., 265–270.
Lakehal, A., and Tachi, F. (2017). Bayesian duval triangle method for fault prediction and assessment of oil immersed transformers. Meas. Control 50 (4), 103–109. doi:10.1177/0020294017707461
Leibfried, T., and Kachler, A. (2002). Insulation diagnostics on power transformers using the polarisation and depolarisation current (PDC) analysis. USA: IEEE, 170–173. doi:10.1109/ELINSL.2002.995905
Li, B. L., Zhou, Q., Liu, Y. P., and Chen, J. X. (2022). A novel nondestructive testing method for dielectric loss factor of transformer oil based on multifrequency ultrasound. IEEE Trans. Dielectr. Electr. Insul. 29 (5), 1659–1665. doi:10.1109/TDEI.2022.3190821
Li, J. Z., Zhang, Q. G., Wang, K., Wang, J. Y., Zhou, T. C., and Zhang, Y. Y. (2016). Optimal dissolved gas ratios selected by genetic algorithm for power Transformer Fault Diagnosis based on support vector machine. IEEE Trans. Dielectr. Electr. Insul. 23 (2), 1198–1206. doi:10.1109/TDEI.2015.005277
Liang, Y., Xu, Y.-Y., Wan, X.-S., Li, Y., Liu, N., and Zhang, G.-J. (2018). Dissolved gas analysis of transformer oil based on Deep Belief Networks. IEEE, 825–828. doi:10.1109/ICPADM.2018.8401156
Liao, R., Liu, J., Yang, L., Zhang, Y., Gao, J., Ma, Z., et al. (2015). Extraction of frequency domain dielectric characteristic parameter of oil-paper insulation for transformer condition assessment. Electr. Pow. Compo. Sys. 43 (5), 578–587. doi:10.1080/15325008.2014.989377
Liu, B., Yu, A., Gao, K., Tan, X., Sun, Y., and Yu, X. (2022). DSS-TRM: deep spatial-spectral transformer for hyperspectral image classification. Eur. J. Remote Sens. 55 (1), 103–114. doi:10.1080/22797254.2021.2023910
Liu, H. L., Chen, J. C., Li, J., Shao, L., Ren, L., and Zhu, L. H. (2023). Transformer Fault warning based on spectral clustering and decision tree. Electronics 12 (2), 265. doi:10.3390/electronics12020265
Liu, Y., Ji, S. C., Yang, F., Cui, Y. J., Zhu, L. Y., Rao, Z. Q., et al. (2015). A study of the sweep frequency impedance method and its application in the detection of internal winding short circuit faults in power transformers. IEEE Trans. Dielectr. Electr. Insul. 22 (4), 2046–2056. doi:10.1109/TDEI.2015.004977
Lu, Y., Wang, X., and Li, Z. (2020). Calculation of fundamental frequency amplitude of transformer surface vibration based on ABC-ELM. England: IOP Publishing. doi:10.1088/1742-6596/1693/1/012221
Ludwikowski, K., Siodla, K., and Ziomek, W. (2012). Investigation of transformer model winding deformation using sweep frequency response analysis. IEEE Trans. Dielectr. Electr. Insul. 19 (6), 1957–1961. doi:10.1109/TDEI.2012.6396953
Luo, J., Yuan, L., Han, L., and Huang, W. (2021). Research on intelligent mining algorithm for distribution network Transformer Fault early warning. England: IOP Publishing. doi:10.1088/1755-1315/701/1/012014
Ma, X., Hu, H., and Shang, Y. (2021). A new method for transformer fault prediction based on multifeature enhancement and refined long short-term memory. IEEE Trans. Instrum. Meas. 70, 1–11. doi:10.1109/TIM.2021.3098383
Malik, H., Sharma, R., and Mishra, S. (2020). Fuzzy reinforcement learning based intelligent classifier for power transformer faults. ISA Trans. 101, 390–398. doi:10.1016/j.isatra.2020.01.016
Mi, X., Subramani, G., and Chan, M. (2021). The application of RBF neural network optimized by K-means and genetic-backpropagation in Fault Diagnosis of power transformer. EDP Sci. 8, 03002. doi:10.1051/e3sconf/202124203002
Miao, X., Jiang, P., Pang, F., Tang, Y., Li, H., Qu, G., et al. (2023). Numerical analysis and experimental research of vibration and noise characteristics of oil-immersed power transformers. Appl. Acoust. 203, 109189. doi:10.1016/j.apacoust.2022.109189
Mishra, D., Dutta, S., Baral, A., Haque, N., and Chakravorti, S. (2019). Use of interfacial charge for diagnosis and activation energy prediction of oil-paper insulation used in power transformer. IEEE Trans. Power Deliv. 34 (4), 1332–1340. doi:10.1109/TPWRD.2019.2892739
Mishra, D., Haque, N., Baral, A., and Chakravorti, S. (2018). Effect of charge accumulated at oil–paper interface on parameters considered for power transformer insulation diagnosis. Iet Sci. Meas. Technol. 12 (3), 411–417. doi:10.1049/iet-smt.2017.0354
Mousavi, S. A., Hekmati, A., Sedighizadeh, M., Bigdeli, M., and Bazargan, A. (2020). ANN based temperature compensation for variations in polarization and depolarization current measurements in transformer. Therm. Sci. Eng. Prog. 20, 100671. doi:10.1016/j.tsep.2020.100671
Muhamad, N., Suleiman, A., Phung, B., and Blackburn, T. (2013). Faults identification of biodegradable oil-filled transformers based on polarization and depolarization current measurement (PDC) method. IEEE Trans. Dielectr. Electr. Insul. 20 (6), 2299–2306. doi:10.1109/TDEI.2013.6678883
Ni, H., Xu, X., Gong, H., Luo, C., and Zhou, Z. (2020). Design of fast Fault Diagnosis system for transformer equipment based on CBR and RBR. England: IOP Publishing. doi:10.1088/1755-1315/546/5/052004
Ounissi, O., Ghodbane, H., Houassine, H., and Sellami, Y. (2023). Noninvasive transmission line diagnostic using FRA technique. Russ. J. Nondestr. Test. 59 (1), 62–72. doi:10.1134/S1061830922601076
Pattanadech, N., Sasomponsawatline, K., Siriworachanyadee, J., and Angsusatra, W. (2019). The conformity of DGA interpretation techniques: experience from transformer 132 units. IEEE, 1–4. doi:10.1109/ICDL.2019.8796588
Pattanadech, N., and Wattakapaiboon, W. (2019). Application of Duval pentagon compared with Other DGA interpretation techniques: case studies for actual transformer inspections including experience from power plants in Thailand. IEEE, 1–4. doi:10.1109/ICEAST.2019.8802523
Poonnoy, N., Suwanasri, C., and Suwanasri, T. (2021). Fuzzy logic approach to dissolved gas analysis for power transformer failure index and fault identification. Energies 14 (1), 36. doi:10.3390/en14010036
Rao, U. M., Fofana, I., Rajesh, K., and Picher, P. (2021). Identification and application of machine learning algorithms for transformer dissolved gas analysis. IEEE Trans. Dielectr. Electr. Insul. 28 (5), 1828–1835. doi:10.1109/TDEI.2021.009770
Rodríguez, J., Contreras, J., and Gaytán, C. (2021). Evaluation and interpretation of dissolved gas analysis of soybean-based natural ester insulating liquid. IEEE Trans. Dielectr. Electr. Insul. 28 (4), 1343–1348. doi:10.1109/TDEI.2021.009467
Roncero-Clemente, C., and Roanes-Lozano, E. (2018). A multi-criteria computer package for power transformer fault detection and diagnosis. Appl. Math. Comput. 319, 153–164. doi:10.1016/j.amc.2017.02.024
Saha, T. K., and Purkait, P. (2004). Investigation of polarization and depolarization current measurements for the assessment of oil-paper insulation of aged transformers. IEEE Trans. Dielectr. Electr. Insul. 11 (1), 144–154. doi:10.1109/TDEI.2004.1266329
Samimi, M. H., Tenbohlen, S., Akmal, A. a. S., and Mohseni, H. (2017). Effect of different connection schemes, terminating resistors and measurement impedances on the sensitivity of the FRA method. IEEE Trans. Power Deliv. 32 (4), 1713–1720. doi:10.1109/TPWRD.2016.2572160
Shamlou, A., Feyzi, M. R., and Behjat, V. (2021). Winding deformation classification in a power transformer based on the time-frequency image of frequency response analysis using Hilbert-Huang transform and evidence theory. Int. J. Electr. Power Energy Syst. 129, 106854. doi:10.1016/j.ijepes.2021.106854
Shen, P., Wang, C., Li, K., Li, Y., Ma, H., and Zhang, Z. (2015). Power and energy. China: CRC Press, 393–398.
Soni, R., and Mehta, B. (2021). Review on asset management of power transformer by diagnosing incipient faults and faults identification using various testing methodologies. Eng. Fail. Anal. 128, 105634. doi:10.1016/j.engfailanal.2021.105634
Standard, I. (2000). High-voltage test techniques: partial discharge measurements. IEC-60270: 13–31.
Sun, L., Xu, M., Ren, H., Hu, S., and Feng, G. (2024). Multi-point grounding fault diagnosis and temperature field coupling analysis of oil-immersed transformer core based on finite element simulation. Case Stud. Therm. Eng. 55, 104108. doi:10.1016/j.csite.2024.104108
Sun, Q. Q., Yang, L., Li, Q., Zhang, X. R., Wang, F., Chen, S., et al. (2021). Surge analysis for lightning strike on overhead lines of wind farm. Electr. Power Syst. Res. 194, 107066. doi:10.1016/j.epsr.2021.107066
Sutikno, H., Prasojo, R. A., and Abu-Siada, A. (2024). Machine learning based multi-method interpretation to enhance dissolved gas analysis for power transformer fault diagnosis. Heliyon 10 (4), e25975. doi:10.1016/j.heliyon.2024.e25975
Talib, M. A., Muhamad, N., Malek, Z. A., and Jamail, N. M. (2013). Application of PDC analysis to identify effect of overheating on dielectric response and conductivity of mineral insulating oil of in-services transformers. IEEE, 583–586. doi:10.1109/CEIDP.2013.6748298
Taneja, M. S., Pandey, K., and Sehrawat, S. (2016). A review on prognosis and diagnosis of transformer oil quality using intelligent techniques based on dissolved gas analysis. IEEE, 1–6. doi:10.1109/IICPE.2016.8079419
Vatsa, A., and Hati, A. S. (2024). Insulation aging condition assessment of transformer in the visual domain based on SE-CNN. Eng. Appl. Artif. Intell. 128, 107409. doi:10.1016/j.engappai.2023.107409
Vatsa, A., Hati, A. S., Kumar, P., Margala, M., and Chakrabarti, P. (2024). Residual LSTM-based short duration forecasting of polarization current for effective assessment of transformers insulation. Sci. Rep. 14 (1), 1369. doi:10.1038/s41598-023-50641-z
Velásquez, R. M. A., and Lara, J. V. M. (2020). Root cause analysis improved with machine learning for failure analysis in power transformers. Eng. Fail. Anal. 115, 104684. doi:10.1016/j.engfailanal.2020.104684
Wang, L.-Z., Chi, J.-F., Ding, Y.-Q., Yao, H.-Y., Guo, Q., and Yang, H.-Q. (2024). Transformer fault diagnosis method based on SMOTE and NGO-GBDT. Sci. Rep. 14 (1), 7179. doi:10.1038/s41598-024-57509-w
Wang, M., Vandermaar, A. J., and Srivastava, K. D. (2002). Review of condition assessment of power transformers in service. IEEE Electr. Insul. Mag. 18 (6), 12–25. doi:10.1109/MEI.2002.1161455
Wang, X., Wu, K., and Xu, Y. (2014). Research on Transformer Fault Diagnosis based on multi-source information fusion. Int. J. Control Autom. 7 (2), 197–208. doi:10.14257/ijca.2014.7.2.19
Wani, S. A., Rana, A. S., Sohail, S., Rahman, O., Parveen, S., and Khan, S. A. (2021). Advances in DGA based condition monitoring of transformers: a review. Renew. Sust. Energy Rev. 149, 111347. doi:10.1016/j.rser.2021.111347
Wattakapaiboon, W., and Pattanadech, N. (2016). The state of the art for dissolved gas analysis based on interpretation techniques. IEEE, 60–63. doi:10.1109/CMD.2016.7757763
Xiao, Y., Pan, W., Guo, X., Bi, S., Feng, D., and Lin, S. (2020). Fault diagnosis of traction transformer based on Bayesian network. Energies 13 (18), 4966. doi:10.3390/en13184966
Xing, Z., and He, Y. (2023). Multimodal mutual neural network for health assessment of power transformer. IEEE Syst. J. 17 (2), 2664–2673. doi:10.1109/JSYST.2023.3237225
Xing, Z., He, Y., Wang, X., Chen, J., Du, B., He, L., et al. (2023). Vibration signal-based deep noisy filtering model for online transformer diagnosis. IEEE Trans. Ind. Inf. 19 (11), 11239–11251. doi:10.1109/TII.2023.3245193
Xu, J., Jin, Z., Fu, J., Shao, Y., Wang, F., and Jaing, Y. (2010). Detection of transformer winding deformation under short-circuit impulse based on improved wavelet packet algorithm. East China Electr. Power 38, 376–380.
Yang, S., Jiao, W., and Wu, Z. (2004). Independent component analysis based networks for fault features extraction and classification of rotating machines. Chin. J. Mech. Eng. En. 40 (3), 45–49. doi:10.3901/jme.2004.03.045
Yang, X., Chen, W., Li, A., Yang, C., Xie, Z., and Dong, H. (2019). BA-PNN-based methods for power transformer fault diagnosis. Adv. Eng. Inf. 39, 178–185. doi:10.1016/j.aei.2019.01.001
Yang, Z., Tang, W. H., Shintemirov, A., and Wu, Q. H. (2009). Association rule mining-based dissolved gas analysis for Fault Diagnosis of power transformers. IEEE Throughput Syst. Man. Cy. C 39 (6), 597–610. doi:10.1109/TSMCC.2009.2021989
Yi, J. H., Wang, J., and Wang, G. G. (2016). Improved probabilistic neural networks with self-adaptive strategies for transformer fault diagnosis problem. Adv. Mech. Eng. 8 (1), 168781401562483. doi:10.1177/1687814015624832
Yu, S., Zhao, D., Chen, W., and Hou, H. (2016). Oil-immersed power transformer internal fault diagnosis research based on probabilistic neural network. Procedia Comput. Sci. 83, 1327–1331. doi:10.1016/j.procs.2016.04.276
Zhang, C., He, Y., Du, B., Yuan, L., Li, B., and Jiang, S. (2020a). Transformer fault diagnosis method using IoT based monitoring system and ensemble machine learning. Future. Gener. Comp. sy. 108, 533–545. doi:10.1016/j.future.2020.03.008
Zhang, L., Sheng, G., Hou, H., and Jiang, X. (2020b). A fault diagnosis method of power transformer based on cost sensitive one-dimensional convolution neural network. IEEE, 1824–1828. doi:10.1109/ACPEE48638.2020.9136223
Zhang, X., and Li, H. (2021). A probabilistic calculation method of transformer temperature fault based on photoelectric infrared sensor. IEEE Sens. J. 21 (18), 20129–20135. doi:10.1109/JSEN.2021.3099213
Zhang, X., Li, H., Lu, L., and Sun, X. (2020c). Transformer Fault diagnosis model and method based on DBNI in photoelectric sensors diagnosis system. Prog. Electromagn. Res. M. 91, 197–211. doi:10.2528/PIERM20010701
Zhang, Y., Tang, Y., Liu, Y., and Liang, Z. (2022). Fault diagnosis of transformer using artificial intelligence: a review. Front. Energy Res. 10, 1006474. doi:10.3389/fenrg.2022.1006474
Zhang, Y., Wang, Y., Fan, X., Zhang, W., Zhuo, R., Hao, J., et al. (2020d). An integrated model for transformer fault diagnosis to improve sample classification near decision boundary of support vector machine. Energies 13 (24), 6678. doi:10.3390/en13246678
Zhang, Z., Wu, Y., Zhang, R., Jiang, P., Liu, G., Ahmed, S., et al. (2019). Novel transformer fault identification optimization method based on mathematical statistics. Mathematics 7 (3), 288. doi:10.3390/math7030288
Zhao, X., Yao, C., Abu-Siada, A., and Liao, R. (2019). High frequency electric circuit modeling for transformer frequency response analysis studies. Int. J. Electr. Power Energy Syst. 111, 351–368. doi:10.1016/j.ijepes.2019.04.010
Zhao, Z., Tang, C., Zhou, Q., Xu, L., Gui, Y., and Yao, C. (2017). Identification of power transformer winding mechanical fault types based on online IFRA by support vector machine. Energies 10 (12), 2022. doi:10.3390/en10122022
Zheng, J., Huang, H., and Pan, J. (2018). Detection of winding faults based on a characterization of the nonlinear dynamics of transformers. IEEE Trans. Instrum. Meas. 68 (1), 206–214. doi:10.1109/TIM.2018.2838178
Zheng, J., Pan, J., and Huang, H. (2015). An experimental study of winding vibration of a single-phase power transformer using a laser Doppler vibrometer. Appl. Acoust. 87, 30–37. doi:10.1016/j.apacoust.2014.06.012
Zhou, X., Luo, Y., Tian, T., Bai, H., Wu, P., and Liu, W. (2023). Transformer fault diagnosis based on probabilistic neural networks combined with vibration and noise characteristics. Front. Energy Res. 11, 1169508. doi:10.3389/fenrg.2023.1169508
Zhu, J., Li, S., Liu, Y., and Dong, H. (2022). A hybrid method for the fault diagnosis of onboard traction transformers. Electron. 11 (5), 762. doi:10.3390/electronics11050762
Zhu, W., Wei, Y., and Xiao, H. (2019). Fault diagnosis of neural network classified signal fractal feature based on SVM. Clust. Comput. 22, 4249–4254. doi:10.1007/s10586-018-1795-x
Keywords: transformer, fault diagnosis, dissolved gas analysis, artificial intelligence, multi-source information fusion
Citation: Cao H, Zhou C, Meng Y, Shen J and Xie X (2024) Advancement in transformer fault diagnosis technology. Front. Energy Res. 12:1437614. doi: 10.3389/fenrg.2024.1437614
Received: 24 May 2024; Accepted: 08 July 2024;
Published: 22 July 2024.
Edited by:
Feng Liu, Nanjing Tech University, ChinaReviewed by:
Haiya Qian, Nanjing Normal University, ChinaCopyright © 2024 Cao, Zhou, Meng, Shen and Xie. This is an open-access article distributed under the terms of the Creative Commons Attribution License (CC BY). The use, distribution or reproduction in other forums is permitted, provided the original author(s) and the copyright owner(s) are credited and that the original publication in this journal is cited, in accordance with accepted academic practice. No use, distribution or reproduction is permitted which does not comply with these terms.
*Correspondence: Haiou Cao, Y2FvaGFpb3UxMjNAcHJvdG9ubWFpbC5jb20=
Disclaimer: All claims expressed in this article are solely those of the authors and do not necessarily represent those of their affiliated organizations, or those of the publisher, the editors and the reviewers. Any product that may be evaluated in this article or claim that may be made by its manufacturer is not guaranteed or endorsed by the publisher.
Research integrity at Frontiers
Learn more about the work of our research integrity team to safeguard the quality of each article we publish.