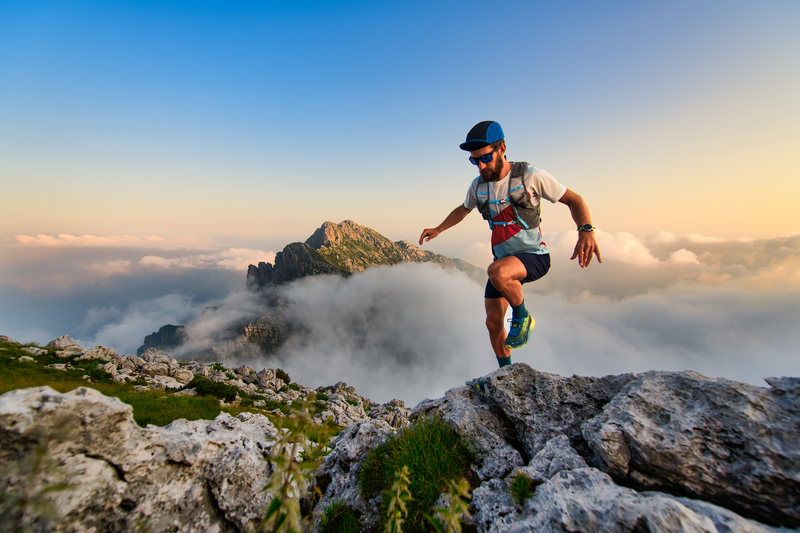
94% of researchers rate our articles as excellent or good
Learn more about the work of our research integrity team to safeguard the quality of each article we publish.
Find out more
ORIGINAL RESEARCH article
Front. Energy Res. , 26 July 2024
Sec. Sustainable Energy Systems
Volume 12 - 2024 | https://doi.org/10.3389/fenrg.2024.1425313
This article is part of the Research Topic Advancing Urban Sustainability: Integrating Renewable Energy for Accelerated Zero-Carbon Community Transitions View all 7 articles
As a novel economic form, the digital economy plays an important role in promoting urban low-carbon sustainable development (LCSD). Based on panel data from 270 cities in China from 2011 to 2021, this study used principal component analysis and the global entropy weight method to calculate the level of digital economy development and LCSD as well as to empirically discuss any correlated effects and mechanisms. The research showed that the digital economy has significantly promoted urban LCSD and has become an important source for promoting green low-carbon development. The digital economy can indirectly promote LCSD by accelerating industrial structure upgrading and promoting technological innovation; Moreover, this promotion can show significant threshold characteristics with these factors. When industrial structure upgrading and technological innovation reach a certain threshold, the digital economy can further enhance urban LCSD. The promotion of the digital economy is positively influenced by marketization level and environmental regulation, and it exhibits distinct regional characteristics. During the critical period of economic development and transformation, the government must continuously promote the development of the digital economy, fully release its dividends on LCSD, and implement a differentiated digital development strategy to promote low-carbon economic development according to local conditions.
Faced with the challenges of global sustainable development and the rapid development of the digital economy, how to achieve a win-win situation between the two has become a key issue that needs to be resolved urgently. Following agricultural and industrial economies, the digital economy is a new economic model that employs data resources as the key factor, modern information technology as the main medium, and its wide application promotes low-carbon sustainable development (LCSD). Throughout the stage of high-quality economic development, the digital economy has shown unprecedented scale and speed. According to the “White Paper on the Development of China’s Digital Economy (2023)” published by the China Information and Communication Academy, the scale of the digital economy reached 50.2 trillion yuan in 2022, accounting for 41.5% of the national gross domestic product (GDP), and has become the primary driving force behind China’s economic growth.
The digital economy has demonstrated an irreplaceable role in stimulating consumption, driving investment, and enhancing innovation capacity using network information technology, providing a core driving force for promoting urban sustainable ecological development (Lee et al., 2022). Among the 47 major countries worldwide, the digital economy contributes nearly 50% of GDP, and digital technology innovation has helped to promote global green development. In 2019, the World Health Organization identified air pollution as the most threatening environmental risk to health, with approximately 7 million people dying prematurely each year from diseases caused or aggravated by air pollution. Additionally, the annual loss of ecosystem service value exceeds 10% of the total global economic value. China’s carbon dioxide emissions increased from 11.35 × 108t in 1980 to 96.20 × 108t in 2018, with coal accounting for the largest proportion, and an average annual growth rate of 5.78% (Jia et al., 2023). It is expected that by 2050, the number of people displaced due to environmental crises will reach 200 million. At the 2015 United Nations Development Summit, the Sustainable Development Goals for 2016–2030 were adopted, and the digital economy was emphasized as a key driver to achieve 17 Sustainable Development Goals by 2030. Cities accommodate more than 50% of the world’s population today and are an important source of greenhouse gas emissions, making them highly sensitive to climate change. Chinese cities generate 75% of GDP while consuming 84% of energy (Dhakal, 2009). The energy intensity effect was the primary driving force behind urban decoupling, highlighting cities’ critical role in reducing carbon emissions and achieving sustainable development (Yang et al., 2020). However, the issue of carbon dioxide emissions in underdeveloped cities in China deserves more attention, urgently requiring energy conservation to improve energy efficiency (Jia et al., 2018b; Jia et al., 2018). To address the challenge of global emissions reduction, developing low-carbon cities has become a key strategic choice. Low-carbon cities can not only mitigate the impact of climate change by reducing carbon emissions but also improve living standards and enhance the sustainable competitiveness of cities (Lou et al., 2019). By building low-carbon cities, a win-win situation of economic growth and environmental protection can be realized. Therefore, pursuing the urban LCSD is considered an important way to achieve a low-carbon future (Qu and Liu, 2017). The 14th Five-Year Plan proposed that China should drive the transformation of production, lifestyle, and governance modes with digital transformation and promote the comprehensive green transformation of economic and social development. The report of the 20th National Congress of the Communist Party of China indicated that promoting the greening and decarbonization of the economy and society is a key link in achieving high-quality development. Promoting urban LCSD is the major means of achieving the “dual carbon” goals.
Under the background that the government has rigid constraints on ecological construction, achieving the coordinated development of the digital economy and low-carbon economy is the key to achieving China’s “green mountains and clear waters” goals in the new era. Therefore, it is necessary to clarify the actual role and impact mechanisms of the digital economy on LCSD, conduct a comprehensive analysis of the intrinsic mechanisms by which the digital economy affects LCSD, and verify the gradual impact effects of the digital economy across different regions. This provides beneficial theoretical support for the realization of the urban low-carbon economic development leap and the implementation of the “digital” strategy, as well as a practical foundation for global economic sustainable development.
Given the increasing importance of achieving environmental sustainability with economic development, this study may potentially make marginal contributions in several ways. First, distinct from previous studies that predominantly focus on the impact of the digital economy on high-quality economic development, green economic development, and green total factor productivity, this study investigates the influence of the digital economy on LCSD for the first time. This has significant guiding implications for achieving China’s “dual carbon” goals and global sustainable development. Second, building upon the connotations of the digital economy and endogenous growth theory, this study proposes two major influencing mechanisms: technological innovation (TI) and industrial structure upgrading (ISU). These mechanisms refine the theoretical framework regarding the impact of the digital economy on green low-carbon development, clarify the transmission pathways through which the digital economy influences LCSD, and unravel the theoretical “black box” between the two. Third, this paper applies the global entropy weight method to calculate the LCSD Index, ISU index, and the digital economy development Index, expanding upon the traditional entropy weight method and offering more precise data for index measurement. Finally, this study integrates traditional econometric regression methods with threshold models, considering the dual linear and nonlinear relationships between the digital economy and LCSD. It addresses endogeneity using the endogenous instrumental variable method. Simultaneously, this study extends heterogeneous analysis by examining the moderating effects of marketization level (ML) and environmental regulation (ER) based on geographical location and resource endowment disparities, thereby providing policymakers with a crucial, context-specific reference.
The term “digital economy” was coined by Tapscott (1996) and gradually attracted the interest of institutions and scholars alike. With continuous breakthroughs in new-generation digital information technology, the concept, content, and connotations of the digital economy are becoming increasingly enriched. Kim et al. (2002) indicated that the digital economy could complete the transactions of goods and services through virtual means, thereby penetrating and altering the operational modes of various professions. Wang et al. (2022) pointed out that the combination of the digital and real economies represented the predominant trend of future development. They also suggested that the digital economy would accelerate the rapid increase of new business models, endowing the sharing and platform economies with certain green characteristics. Li et al. (2020) proposed that the digital economy has reshaped business processes and accelerated the green transformation of the real economy through government economic growth policies and digital entrepreneurship.
It has been considered that the effect of the digital economy is multi-dimensional and composite. At the micro level, it can quickly and effectively integrate production factors (Bunje et al., 2022), promote the intelligent transformation of enterprises, enhance information analysis capacity and management decision levels of enterprises, and enable enterprises to improve LCSD via economies of scale, technological innovation, and other channels (Vu, 2013; Zhang et al., 2022). At the macro level, the digital economy can boost economic growth by investing in new elements and resources, and by improving allocation efficiency. Simultaneously, information sharing strengthens knowledge diffusion and technological spillover, driving coordinated innovation among industries in neighboring regions to improve resource allocative efficiency and LCSD (Wan et al., 2022). At the industry level, the element input in the growth framework of the digital economy is far greater than that of traditional scale growth. With the addition of variables, such as data, the fusion of the digital economy and the modern service industry is realized through capital deepening, resource optimization, and technological change, which promotes the rapid development of the platform economy and presents a significant long-tail effect with substantial differences in the impacts on the manufacturing industry (Berkhout and Hertin, 2004; Zhou et al., 2022).
Efforts have been made to identify sustainable development and provide a theoretical basis. Existing research mainly includes energy sustainability (Elavarasan et al., 2022), ecological sustainability (Ghobakhloo, 2020), economic sustainability (Mondejar et al., 2021), and other aspects. Low-carbon development regards the low-carbon economy as a long-term development goal, which can play a pivotal role in guiding project directions, particularly concerning energy use structure and urbanization rate (Jia et al., 2012). However, a common standard for green, low-carbon, and sustainable development indicators for cities is lacking. Most studies have measured carbon emissions (Chien et al., 2021), sustainable development efficiency (Khan et al., 2021), and sustainable development index (Hickel, 2020), which cannot fully reflect the profound connotation of LCSD. To reduce the carbon emission intensity in cities, LCSD proposed the decoupling of economic growth from carbon emissions, optimization of industrial structure, improvement of energy utilization efficiency, and development of clean energy. At the same time, it is necessary to promote the development of a green economy, promote innovation and the application of technology, and explore potential economic growth opportunities for cities (Wen et al., 2023). LCSD is a holistic concept that requires comprehensive consideration and balance in multiple aspects such as economy, society, and environment (Tan et al., 2023). In this study, we constructed an evaluation system for urban LCSD in five aspects, namely, low-carbon economy, low-carbon energy, low-carbon society, low-carbon environment, and urban mobility.
Existing research has formed several viewpoints such as the digital economy can help to promote green and low-carbon urban development (Ulucak et al., 2020; Jayaprakash and Pillai, 2022), Shobande and Ogbeifun (2022) analyzed the role of information and communication technology in promoting environmental sustainability in 24 countries of the Organization for Economic Cooperation and Development from 1980 to 2019 based on a dynamic panel model. The digital economy may have a negative impact on green low-carbon development (Cheng et al., 2019; Avom et al., 2020). Cheng et al. used data from 285 Chinese cities from 2003 to 2016 to analyze whether information technology has exacerbated environmental pollution. Other scholars believe that the digital economy has dual effects on low-carbon urban development, both promoting and hindering (Danish et al., 2019). Khan et al. (2020) argue that information and communication technology will exacerbate carbon dioxide emissions, it could lead to reductions once a threshold level is reached.
The inconsistent results of these studies may be due to the following factors: first, most existing analyses have largely focused on the impacts of the Internet (Ren et al., 2021), and only focus on analyses in the country- (Bunje et al., 2022) or provincial-level (Li et al., 2020), ignoring the differences in internet development between cities. Second, there is a lack of unified low-carbon sustainability assessment standards, and commonly used carbon emission indicators cannot comprehensively measure the complex connotations of sustainable development (Asongu et al., 2018; Chien et al., 2021). Third, there are differences in ISU and TI among different cities, which, to some extent, affects the degree to which the digital economy plays a role in low-carbon development (Li et al., 2021). Digital economy development can promote productivity (Tranos et al., 2020), affect total factor productivity (Pan et al., 2022), as well as bolster high-quality economic development (Ma and Zhu, 2022), energy economy development (Jiang et al., 2023), and regional sustainable development (Luo et al., 2023); however, few studies have put the digital economy and urban LCSD into an analytical framework, representing the research gap focused on by the present study.
In contrast to existing literature, this study makes contributions to the following three aspects: First, with regard to research methods, this study builds a comprehensive LCSD system and a digital economy development indicator system. Second, this study focuses on 270 cities in China and explores the main pathways through which the digital economy affects the LCSD within a unified research framework, presenting an alternative for promoting urban green economic development via the digital economy, and deepening existing research. Third, with regard to research content, this study examined the mechanisms and nonlinear effects of the digital economy on LCSD using the intermediary effect and panel threshold models.
The following is the structure of the remaining sections of this study: In Section 3, the mechanism analyses and research hypothesis explain the contributive path of the digital economy to LCSD. Section 4 provides the econometric model, variable selection, and data description. Section 5 introduces the results and discusses the intermediary effect, panel threshold effect, and heterogeneity analysis results. Lastly, Section 6 summarizes the conclusions and proposes relevant policy recommendations.
Digital economy characteristics, such as immediacy, speed, self-expansion, and high permeability, have interrupted the deep-rooted monopoly in traditional business forms and driven supply-side structural reform. The endogenous economic growth theory posits that TI is a crucial factor driving sustained economic expansion. The digital economy can propel the integration and development of technical progress and industries, thereby bringing about comprehensive transformations in economic and social productivity, as well as modes of production. Therefore, this paper proposes to explore the impact mechanisms of TI and ISU from two aspects: the process and results of the influence of digital economy on LCSD. Sustainable development is a multi-dimensional developmental concept involving the efficient utilization of resources and comprehensive protection of the environment via coordinated development (Guo et al., 2022). With the upcoming new round of technology and industrial revolutions, especially the application of new-generation information technologies, the digital economy can promote TI, adjust industrial structure, and stimulate green low-carbon development. The digital economy can directly affect LCSD through its inherent characteristics as well as indirectly through intermediary variables. Simultaneously, considering the “Metcalfe rule” of the Internet (i.e., the cost of a web is proportional to the square of its number of nodes), which presents an increasing phenomenon of marginal utility under the network economy, the digital economy impacts on LCSD may also maintain nonlinear characteristics.
The rapid development of the digital economy helps to eliminate the information asymmetry problem caused by time and spatial dislocation in traditional economic development models, thus giving rise to new economic phenomena: in the supply-demand relationship, demand gradually replaces supply as the dominant factor, forming a new economic structure. Demand information is effectively collected and fed back to suppliers, becoming a crucial basis for supply decisions, challenging traditional standardized and large-scale production models, prompting enterprises to shift towards precise docking and perfect matching production models, and improving supply efficiency. The demand-centric matching model will further highlight the people’s demand for a better life and a beautiful ecological environment, and the resulting new supply will continue to promote industrial optimization, becoming an important component of the digital economy in promoting urban low-carbon development.
In terms of the advanced industrial structure, the permeability of the digital economy has promoted the development of traditional industries towards digitalization and intelligence, and has pushed the management, technology, and products of traditional industries to become increasingly advanced. The innovation of the digital economy has promoted the vigorous growth of emerging industries, especially the proportion of the information industry has gradually risen. In terms of the rationalization of industrial structure, the digital economy, with its digital and convenient characteristics, can promote the circulation and sharing of information within the industry, which not only helps to solve the imbalance in resource allocation among departments, but also enables the industry to quickly obtain external information and dynamically adjust the unreasonable industrial structure based on market demand.
With the upgrading and rationalization of industrial structure, digital and other high-tech industries have promoted the innovation of emerging business models, which is conducive to the effectiveness of environmental governance. The continuous emergence of new industries and business models will accelerate the elimination of high-polluting and high-energy-consuming industries, thus promoting the development of strategic emerging industries and modern service industries, and injecting new industrial vitality into the urban LCSD. In the process of ISU, the asynchronism of technological progress directly leads to the differences in production efficiency among different sectors, which promotes the gradual flow of production factors from high-energy-consuming, low-productivity, low-value-added sectors to low-energy-consuming, high-productivity, high-value-added sectors. This shift not only enhances production efficiency but also improves energy utilization efficiency. It can be seen that the “structural dividend” brought by ISU can improve environmental quality and promote economic growth, which is a key measure to promote high-quality economic development and a necessary path to achieve low-carbon and sustainable economic development. Therefore, this paper proposes the second research hypothesis:
Hypothesis 1. The development of the digital economy enhances the level of LCSD by promoting ISU.
TI is the fundamental approach to achieving low-carbon development under the constraint of limited environmental carrying capacity. Compared with the traditional extensive development models, the LCSD model not only considers factors such as labor, capital, and economic output but also takes into account resource elements and unexpected outputs, aiming for a win-win situation between economic and environmental benefits. No factor alone can ensure that economic and social development can be permanently sustained. Within the framework of endogenous economic growth theory, technological progress is the determining factor that ensures sustained economic growth. However, when Aghion et al. (1998) integrated environmental pollution and resource constraints into Schumpeter model, they found that if a continuous flow of innovations can be maintained through TI and the productivity of innovations is greater than the discount rate of time, it can drive the economic equilibrium point outward in the new stage of development, thereby achieving more output.
The digital economy driven by big data, the Internet of Things and artificial intelligence, has its own technological attributes. The scale and diffusion effects manifested by the digital economy have promoted technological advancement and innovation, thereby accelerating the improvement of market conditions. Firstly, the rapid development of information and communication technology provides a cost-effective competitive advantage for data innovation. Enterprises can use big data to analyze consumer information, promote targeted innovation, and reduce costs. Secondly, the rise of the digital economy accelerates the speed of information transmission, enriches knowledge acquisition channels, promotes a more open and transparent market environment, which is conducive to TI. Digital technology investment also contributes to enhancing regional human capital and labor productivity, attracting the gathering of high-quality labor force and promoting TI such as “learning by doing.” Finally, the proliferation of the Internet of Things eliminates the constraints of time and space on innovation activities, allowing different innovation entities to participate simultaneously. The industrial digital transformation brought by the digital economy facilitates better matching among developers, producers, and consumers, further motivating scientific research and innovation entities engaged in research and development activities to more actively convert knowledge into new products, processes, and services. Moreover, the development of the digital economy also helps innovative entities to better utilize and protect intellectual property, promotes the refinement of relevant laws and regulations, and improves the efficiency of intellectual property protection, thus advancing the level of urban TI.
The goal of TI is to promote economic growth by achieving efficient resource utilization, reducing carbon emissions and their impact on the environment, and providing technical support and paths for LCSD. TI promotes the development of low-carbon economy as enterprises reduce carbon emissions in the production process through the research and application of low-carbon technologies, thereby improving resource utilization efficiency, reducing production costs, and increasing competitiveness. This contributes to both economic growth and sustainable development. TI facilitates the promotion and utilization of low-carbon energy. The continuous emergence of new energy technologies such as solar energy, wind energy, geothermal energy provides a more sustainable option to replace traditional high carbon energy. The application of these technologies not only reduces dependence on fossil fuels but also helps to reduce carbon emissions during energy production and consumption processes. TI also plays an important role in building a low-carbon society. Innovative applications such as smart city technology, green building design, and sustainable transportation systems provide people with more convenient and comfortable lifestyles, improving their quality of life; TI has also given rise to a new eco-friendly, resource saving, and economically efficient technological approach, supporting the transformation of eco-friendly production models and effectively reducing harmful emissions in industrial production, such as exhaust gas, wastewater, and dust, providing technical solutions for reducing pollutant emissions levels; TI can provide key support for constructing low-carbon transportation systems. The application of emerging technologies such as intelligent transportation management systems, electric vehicles, and shared mobility services can help alleviate traffic congestion, reduce exhaust emissions, and improve urban mobility efficiency, thereby enhancing people’s travel experience. Therefore, this paper proposes the first research hypothesis:
Hypothesis 2. The development of the digital economy enhances the level of LCSD by promoting TI.
The Kuznets curve was originally used to describe the trend of income inequality in a country or region during the process of economic development. But the theoretical framework has also been applied in the field of environmental economics. In particular, it is used to analyze the relationship between environmental degradation and economic growth, resulting in the so-called “Environmental Kuznets Curve.” In this model, environmental pressure initially increases with economic growth, but after a certain point, decreases with further economic growth. This theory is used to explore how the development of digital technology affects the urban LCSD in the context of the digital economy.
In the early stages of digital economic development, limited by the resource consuming development model, the urban industrial structure was relatively low, the infrastructure level was not high, and problems such as high industrial proportion and serious environmental pollution were often accompanied. The role of digital technology in environmental pollution control was relatively weak, and the development of the digital economy was relatively immature, which had a negative externality effect on the environment. In the process of digital industrialization and industrial digitization, the technological progress brought about by the digital economy has increased the scale of mining rare minerals and consumed a large amount of electricity resources; The high input and cost associated with the digital economy have elevated carbon emissions in production and daily life. While the digital economy forces enterprises to conduct green technology research and development, it also leads to a cumulative impact of energy consumption input. This results not in an increase but rather a decline in the level of green sustainable development in the early stages.
As the digital economy matures and technological progress advances, ER and energy control become more effective. Industrial production and sales gradually optimize, leading to stable enterprise output. Initial capital, human resources, and technology inputs gradually yield positive net effects. A highly green industrial structure can provide a solid foundation for reducing environmental pollution in the production process. Market participants can efficiently match market supply and demand, reduce information transaction costs, and improve information services efficiency, thus reducing resource consumption (Litvinenko, 2020). With the continuous popularization of digital applications, digital technologies allow producers to tap consumer demand preferences more extensively, in addition to scientifically positioning market demand, properly coordinating production plans, and gradually improving resource utilization efficiency. Additionally, enterprises can achieve improved alignment efficiency between upstream and downstream of the supply chain through digital network delivery platforms, promote the rational flow of factors, and improve the efficiency of the supply chain, enterprise transactions, and resource allocation (Acemoglu and Restrepo, 2018). For the digital economy, the incremental cost of linkage among enterprises, governments, universities, scientific research institutions, and other departments has been continuously reduced. In contrast, joint innovation has achieved substantial group breakthroughs, and the benefits obtained by participants have continued to increase, showing geometric growth (Zhao et al., 2020). Moreover, this effect will become more obvious with the advancement of ISU and TI; that is, the “Metcalfe Law” will be established in this study. Therefore, this paper proposes the third research hypothesis:
Hypothesis 3. The digital economy has a nonlinear impact on LCSD.
Based on the above research hypothesis, the impact path of the digital economy on LCSD is shown in Figure 1.
Based on the above theoretical analyses, to test the direct transmission mechanism of the digital economy on LCSD (Liu et al., 2022), the benchmark regression model was set according to Eq. 1:
where
ISU and TI may be important reasons why the digital economy promotes LCSD; therefore, these two variables were introduced, and the intermediary effect model was used as a reference to test the mechanisms by which the digital economy promoted LCSD (Eqs 2, 3):
where
In addition, the empirical test of the indirect transmission mechanism must also consider the “network effect” and “Metcalfe rule” of the Internet; namely, the value of the Internet and the number of users should show a square proportional relationship. Based on the Hansen panel threshold model (Hansen, 1999), ISU and TI may have a non-linear impact on promoting LCSD by the digital economy. Therefore, the panel threshold model was set as a single model here (Eq. 4):
Considering the potential for multiple problems with threshold values, the multi-threshold panel regression model can be generalized into Eq. 5:
In Eqs 4, 5,
Urban LCSD is a multi-objective issue, and although it has received attention, there has been no official publication of a unified measurement standard. We draw on existing studies (Tan et al., 2017; Shen et al., 2021) and construct an evaluation framework for urban LCSD from 18 quantitative indicators in 5 categories, including low-carbon economy, energy, society, environment, and urban mobile. Low-carbon economy emphasizes economic development from the perspectives of industry and technology. Low-carbon energy is not blindly reducing carbon emissions, but rather adjusting the energy structure while developing the economy. Low-carbon society places greater emphasis on the status of consumers and highlights the development of eco-friendly transportation. Low-carbon environment uses urban space as a vehicle to achieve low-carbon infrastructure and minimize pollutant emissions to the greatest extent possible. Urban mobility may bring about issues such as housing and transportation, affecting urban energy consumption. The indicator system is illustrated in Figure 2 and is calculated using the global entropy weight method; the larger the indicator value, the better the level of urban LCSD. The global entropy method improves upon the traditional entropy method by retaining its objective weighting advantages. Additionally, it introduces a global perspective for analyzing evaluation indicators both vertically and horizontally. Compared to the traditional entropy weight method, the global entropy weight method provides more accurate calculation results.
As a core explanatory variable, no unified consensus has been formed regarding the concept and scope of the digital economy, and the measurement standards are also not integrated. Considering data availability, the digital economy evaluation system used here was constructed from the dual aspects of digital financial inclusion and Internet evolution, per Zhao et al. (2020). Digital financial inclusion was expressed by the Digital Inclusive Financial Index, and Internet development was measured via three aspects: digital infrastructure, digital innovation and development, and digital economy demand (Figure 3).
Figure 3. Low-carbon sustainable development evaluation indicators. Notes: (+) represents a positive indicator, i.e., the larger the value of the indicator, the better it is; (−) represents a negative indicator, i.e., the smaller the indicator value, the better it is.
Principal component analysis primarily examines the correlations among multiple variables, exploring how to reveal the internal structure of these variables through several principal components. Its goal is to preserve as much information from the original data as possible while ensuring the independence among components. Therefore, the digital economy index was calculated using principal component analysis. To facilitate the comparison of the level gap among cities in different years, the results were standardized according to the methods of Pan et al. (2022) (Eq. 6), and the processed results ranged between 0 and 1:
where
Figure 4 shows that since 2012, the level of digital economy in municipalities, which are directly under the central government, and that of provincial capital cities, has undergone a leapfrog development, but the differences among cities are obvious, leading to a digital divide. In the eastern region represented by cities such as Shanghai, Nanjing, Hangzhou, and Guangzhou, the digital economy is in a leading position, higher than the other regions, especially in the western region represented by Nanning, Yinchuan, and Chongqing. The digital economy is developing at a slower pace. As a globally influential inland economic belt, the Yangtze River Economic Belt (YREB) occupies half of China’s population and total economic output. Its digital economy started earlier and has more effectively gathered resources such as capital, talent, and information; therefore, its level of digital economy development is ahead of non-YREB cities.
Based on previous theoretical analysis, this study constructed mediation and threshold variables from the dual dimensions of ISU and TI, in addition to exploring the transmission path of the digital economy to the LCSD. Industrial structure rationalization (ISR) and industrial structure advancement (ISA) were selected to measure ISU (Zhu et al., 2019). Among them, Industrial structure rationalization is a measurement of the coupling degree between factor input and output structures (Shao et al., 2023); however, these might offset the deviation among industries, resulting in a “false” rationalization of industrial structure. Accordingly, the present study drew inspiration from the improved practices of Zhu et al. (2019) and measured the industrial structure rationalization by calculating the Thiel index, as shown in Eq. 7:
where Y is the value of production, the unit is hundred million yuan. L is the total employment, and the unit is ten thousand people,
Industrial structure advancement is reflected by calculating the angle value of the industrial structure, which includes two definitions: the evolution of proportional relationships among the three industries and the improvement of labor productivity. Referencing the idea of Sun et al. (2022), this study constructed the industrial structure advancement index according to Eq. 8:
where
TI plays a critical role in achieving environmentally sustainable development and carbon neutrality. Referring to Wang et al. (2021), the natural logarithm of green patent applications adding 1 was taken as the measure of TI. Here, the TI level increases with the value. The green patent applications were sorted through the International Patent Classification number of the “Green List,” along with the patent application information supplied by the China National Intellectual Property Administration. The present study selected patent applications instead of authorizations, as there is a time difference between the application and authorization of a patent, impacting overall scheduling and efficiency. Further, patent authorizations are susceptible to the preferences of patent agencies, policy fluctuations, and other factors.
To more comprehensively and accurately analyze how China’s digital economy affects the urban LCSD, it is crucial to control the important variables that affect the LCSD. This study selected the economic development level (EDL), openness to the outside world (OP), level of urbanization (URB), human capital (HC), and government intervention (GI) as the control variables. Improving regional economic development requires the enlargement of economic scope, the increment of resource utilization efficiency, and the enhancement of technological innovation ability, thereby promoting LCSD advancement. Simultaneously, the only theory of GDP growth brought about via the enlargement of economic scope may inhibit China’s LCSD (Li et al., 2021). Under the relatively new situation of openness, free trade, and investment will increase the speed of economic growth, thus improving the output level of resources and environmental inputs. Following the influence of scale economies, there is a gradual improvement in environmental quality, which has an important impact on green low-carbon development. The academic community maintains two different views on this matter, namely, “pollution refuge” and “pollution halo” (Li et al., 2020). Improving level of urbanization can improve urban public service facilities, promote the transformation and advancement of economic structures, as well as accelerate the construction of compact and intensive urban agglomerations. Human capital is the source for promoting the environmental protection of technological development. Optimizing and allocating human resources will have a positive impact on LCSD. Simultaneously, the improvement of human capital displays a lag, which can make the impact insignificant. The government can facilitate the green transformation of enterprises by formulating green market trading rules, controlling green market entry conditions, and strengthening market moral constraints. Moreover, local governments may strive for high GDP at the cost of damaging the environment while pursuing the “promotion championship” of maximizing economic benefits, with negative implications for sustainable economic development (Liu et al., 2022). The specific original indicators were the logarithm of per capita GDP, the proportion of utilized foreign investment in GDP, the logarithm of population density, the proportion of college students in universities to the total population at the end of each year, and the proportion of general public budget expenditure in GDP.
This study focused on 270 Chinese cities from 2011 to 2021 and obtained 2970 sets of panel data. All price-related variables were normalized involving prices, considering 2011 as the base period. The data of the LCSD were retrieved from the China Urban Statistical Yearbook (2012–2022) and China Environmental Statistical Yearbook (2012–2022), whereas the measurement data of the digital economy were retrieved from the Digital Inclusive Financial Index (2012–2022), Statistical Yearbook of China’s Industrial Economy (2012–2022), and the Statistical Yearbook of China’s Third Industry (2012–2022). Partial missing data are processed by referring to the annual statistical reports of prefecture-level cities and fitting them via linear interpolation. Descriptive statistics are displayed in Table 1.
Excluding the issue of endogeneity, this study derived the fixed effects model through the Hausman test; the results are displayed in Table 2.
In Column (3), the estimated coefficient of the digital economy was significantly positive(p < 0.01), indicating that the digital economy development has a positive impact on LCSD. In the booming stage of the digital economy, with the rapid progress of high-tech, the joint construction and sharing of network infrastructure have been promoted, leading to the transformation of the traditional industrial economy into an intelligent industrial economy, reducing social transaction and information search costs, and improving resource allocation efficiency (Luo et al., 2022). The continuous innovation of communication technologies such as big data, cloud computing, and cloud storage, has improved the balance between supply and demand, while forming economies of scale effects, thus promoting LCSD improvement.
After adding two-way fixed effects and control variables, the influence coefficient of the economic development level was significantly positive(p < 0.01), indicating that cities with more developed economies focus more on green innovation, and possess stronger green innovation ability as well as low-carbon development awareness. The influence coefficient of OP was negative and insignificant, possibly due to the absorption of high energy consumption and high pollution industrial transfers by openness. Meanwhile, the introduction of foreign capital can readily lead to technological dependence, which restricts the enhancement of the level of urban independent innovation; thus, its impact on LCSD is insignificant. The influence coefficient of urbanization level was significantly positive (p < 0.05), showing that its rise was conducive to the integration of urban resources, as well as the advancement of urban operational efficiency. The coefficient of human capital was also significantly positive (p < 0.05), supporting that its input enhanced the ability of urban scientific and technological innovation and optimized resource allocation, injecting new vitality into the urban green low-carbon development. Although the influence coefficient of government intervention was negative, it was not significant following the addition of the two-way fixed effect, indicating that the government may be restricted by budget and investment use during the process of intervention in environmental protection and governance, in addition to the presence of certain limitations. Accordingly, it appears that government intervention has not become the primary support of urban LCSD.
Endogeneity issues are commonly encountered in economics. Here, endogenous factors may have arisen from the following sources: First, when analyzing the factors that affect the urban LCSD, the control variables considered here may not encompass all influencing factors, leading to missing variables. Second, the improvement of low-carbon economy level benefits from the digital economy development, and the digital economy development itself may be influenced by the technological strength represented by low-carbon development, leading to a potential two-way causal relationship between them. Accordingly, this study adopted the instrumental variable approach to mitigate potential endogenous issues and more accurately analyze the net effect of the digital economy on LCSD.
Regarding the construction of the instrumental variable and drawing on the methods of Sun et al. (2023), telephone ownership per 10,000 people in each city in 1984 was taken as the instrumental variable for the digital economy. The reasons are as follows: First, the development of traditional Internet technology originated from the telephone line dialing intervention service, and then ushered in the rapid development of integrated services digital networks and fiber broadband access technology. Accordingly, the birth of the digital economy depends on traditional communication and post-telecommunications industries, and the area with earlier and more telephones may correspond to that with the highest penetration rate of the Internet, as well as the area with the most rapid digital economy development; however, with the advancement of information technology, the fixed-line telephone has been replaced as the traditional telecommunication tool, and it is unlikely to directly affect the current LCSD. To this end, the relevance and exogenous requirements of the instrumental variables have been met. Since the instrumental variable is sectional data, the practice proposed by Nunn and Qian (2014) was referred to for specific applications. Here, the interaction item was constructed based on the number of Internet users nationwide in the previous year and telephone ownership per 10,000 people in 1984 to obtain the instrumental variable in panel form.
Table 3 presents the regression results of instrumental variables. After accounting for endogeneity, the influence coefficient of the digital economy remained significantly positive (p < 0.01). For the insufficient identification test, the p-value of the Kleibergen-Paap rk LM statistic is 0, rejecting the under-identification test. For the weak identification test, the Kleibergen-Paap rk Wald F statistic is greater than 16.38 at the 10% level. Therefore, the selection of instrumental variables is reasonable.
The digital economy may impact LCSD through ISU and TI. To verify this mechanism, it was analyzed using the mediation effect model (Table 4). Column (2) shows that the digital economy has a significantly positive promoting effect on ISU at the 1% level. Column (3) indicates that both the digital economy and ISU have significantly positive effects on LCSD at the 5% levels, respectively. The estimated coefficient of the digital economy decreased from 0.2616 to 0.1846 after considering the impact of ISU. Specifically, ISU plays a partial mediating role in the effect of the digital economy on LCSD, which was 0.077 and accounted for 29.43% of the total effect. Column (4) displays that the digital economy can significantly improve the TI at the 1% level, whereas Column (5) suggests that both the digital economy and TI have significantly positive impacts on LCSD at the 5% level. The intermediary effect was calculated (0.061) by comparing the influence coefficient and accounted for 23.32% of the total effect; therefore, ISU and TI were both effective intermediary variables for the digital economy to promote urban LCSD. Thus, the digital economy can improve LCSD through these variables, hence supporting Hypothesis 1, 2.
With improvements in ISU and TI, the impact of the digital economy on urban LCSD may not be linear in the traditional sense. To verify this nonlinear feature, ISU and TI were used as variables to test for a threshold effect during the promotion of the digital economy on LCSD. First, the Hansen (1999) method was used as a reference to determine the existence of a panel threshold by repeatedly sampling 1,000 times using a bootstrap method. Subsequently, a regression model with the corresponding threshold number was set.
Table 5 shows that both ISU and TI have a single threshold effect, with threshold values of 0.2795 and 6.5525, respectively (Table 6). In Table 6, when the threshold value of ISU was <0.2795, the influence coefficient was negative and had no significant impact, whereas when it was >0.2795, the influence coefficient was significantly 0.2179 at the 1% level. This indicated that with the “structural dividend” brought by the ISU, the promotion effect of the digital economy on LCSD was continuously increasing, presenting a nonlinear change feature.
When the TI was <6.5525, the influence coefficient was significantly 0.1659 at the 5% level. When the TI was >6.5525, the influence coefficient was significantly 0.2931, indicating that with the increase of the TI level, the promotion effect of the digital economy on LCSD increased marginally, and nonlinear characteristics still existed, thereby supporting Hypothesis 3.
Owing to various geographical locations and resource endowments, LCSD and the digital economy displayed marked regional characteristics. To explore the differences in the impact of the digital economy on urban LCSD, this study divided the sample cities into eastern, central, and western cities based on their geographical locations. The results are listed in Table 7. The urban digital economy in the eastern and central regions significantly promoted LCSD, while there was no significant impact in the western region (Li et al., 2021). The reason may be that compared with western regions, the central and eastern regions maintain higher economic levels, more complete digital infrastructure, higher technological innovation levels, greater digital economy development levels, and an improved development dividend of the digital economy, the promotion effect on LCSD is more apparent. However, the digital industry in western regions is rare, where the digital economy development is relatively lagging; therefore, its effect on improving the level of LCSD has not yet been shown.
The YREB is China’s economic center of gravity and vitality and has an important strategic position in promoting sustainable economic and social development. In this study, China’s cities were divided into YREB and non-YREB cities to test for heterogeneity. The results suggest that the digital economy significantly contributes to LCSD in two types of cities. However, as a globally influential inland economic zone and a pioneer demonstration zone for ecological civilization construction, the YREB not only expands the positive externalities of the digital economy but also promotes urban low-carbon development (Luo et al., 2022), resulting in a higher impact than that of non-YREB cities.
The digital economy in different regions may have varying impacts on LCSD due to differences in ML, ER, and other factors. Therefore, this study will conduct an extended heterogeneity analysis from two dimensions: ML and ER. ML is represented by the marketization index of various provinces and municipalities in China (Guo et al., 2022), and ER is measured by the comprehensive utilization rate of industrial solid waste in each city (Li et al., 2021). Regression results show that the interaction terms of the digital economy with ML and ER are significantly positive at the 1% level (Table 7). The improvement of urban green and low-carbon development by the digital economy is positively regulated by ML and ER. Cities with elevated ML and robust ER tend to prioritize intellectual property rights, formulate and implement targeted innovation support and environmental protection policies, enforce rigorous market supervision, and foster a conducive environment for cultivating dynamic new formats in the digital economy; Conversely, cities with lower ML and weaker ER may be dominated by administrative power and face more restrictions on factor mobility, hindering the green and low-carbon transformation of enterprises and diminishing the promotion effect of the digital economy on urban LCSD.
To avoid the impact of outliers and extreme values, this study conducted bilateral reduction and truncation analyses on the LCSD at the 1% level. Table 8 suggests that the influence coefficient of the digital economy on urban LCSD remained significantly positive, indicating that the benchmark regression results in this study were robust when considering outliers and extreme values.
In this study, the principal component analysis was used to calculate the digital economy index. To further verify the robustness of the regression results, the global entropy weight method was used here to recalculate the values (Table 8). Column (3) indicates that the size and symbol of the influence coefficient for the new digital economy index on LCSD did not change evidently; thus, the regression result is robust.
We have used the 1984 telephone ownership data per 10,000 people as the instrumental variable to resolve the endogenous issue; however, the control variable may also maintain the endogenous issue because of “reverse causality.” To address this, the digital economy lagging by one period was selected as the new instrumental variable, while all other control variables were also treated with a one-stage lag. The results showed that the digital economy had a significant positive at the 1% level (Table 8, Column (4)).
Based on the major strategic opportunities for a rapid digital economy and the strategic orientation of low-carbon development advocated in the “14th Five-Year Plan,” this study included 270 cities in China as the perspective and calculated the urban LCSD and the digital economy index by the annual urban-level panel data from 2011 to 2021. Further, the impact and mechanism of the digital economy on LCSD were systematically tested using the panel fixed effect, intermediary effect, panel threshold, and grouping regression models producing the following conclusions: first, overall, the digital economy has significantly promoted urban LCSD and has become a key driving factor for promoting urban green and sustainable development in the modern era. This conclusion remained valid after considering the outliers, changing the core explanatory variables, and adjusting the instrumental variables. Second, for the impact mechanism analysis, the digital economy can indirectly promote LCSD by accelerating the ISU and promoting TI. Third, for the nonlinear analysis, the promotion of the digital economy on LCSD will present threshold characteristics for the ISU and TI. Fourth, for the heterogeneity analysis, the impact of the digital economy in the central and eastern regions is relatively high. The urban LCSD in the YREB can enjoy more digital economy development dividends. In an environment with higher levels of ML and ER, the digital economy plays a more significant role in promoting urban LCSD. Furthermore, applying these conclusions globally can provide valuable insights for the sustainable development of cities worldwide.
Based on the above research conclusions, we propose the following recommendations:
First, the construction of the urban digital infrastructure was improved. It is necessary to leverage the green empowerment role of the digital economy, government should constantly improve the new digital infrastructure, such as Internet Protocol Version 6 and 5G, as well as increase investment in industries related to the big data platforms (Nuccio and Bertacchini, 2022). Further, digital dividends should be more extensively exploited, and high-quality development of the urban green economy should be vigorously promoted. Simultaneously, the relevant government should consistently optimize the data supervision system while preventing data leakage, data monopolies, or other impacts and providing a new impetus for the digital economy to promote LCSD.
Second, the role of ISU and TI in promoting LCSD during digital economy development should be strengthened. Notably, it is necessary to optimize the positive impacts of the digital economy on ISU; thus, the government should take measures to guide the flow of production factors to resource-saving and environment-optimizing emerging industries, take ISU as the main line of transforming the economic growth mode, and help to promote the urban green sustainable development. Enterprises should actively play the core role of digital technology advancement in TI, improve the digital content of TI, shape new advantages of green development and improve urban LCSD. Furthermore, governments and enterprises must incorporate the nonlinear impact characteristics of the digital economy on LCSD while striving to eliminate the threshold effect during the digital economy development, promote the linkages of the digital economy with ISU and TI, implement differentiated market strategies, and efficiently adjust and optimize to release the driving advantages of the digital economy on LCSD.
Third, a diversified development strategy was implemented here. Considering the heterogeneity of the digital economy’s impact on urban LCSD, when formulating digital economy development strategies, the government should fully consider the characteristics and advantages of local resources, integrate with local industrial development methods, and create a good digital education environment. At the same time, local governments should comprehensively promote market-oriented reforms, fully leverage the leading role of the market in resource allocation, ensure fair market competition through impartial market supervision, and promote the green and low-carbon transformation of industries. The eastern and central regions can rely on their economic development, technological innovation, and other resource advantages to steadily improve the digital economy, which can have a radiating effect on exporting talent, technologies, and achievements to the western regions, and thus drive the development of its digital economy. The western regions can also learn from the development experience, further strengthening the construction of the digital infrastructure, cultivating digital economy pilot projects that integrate with local resources and digital technology, guiding a new model of industrial development with regional characteristics, and promoting the low-carbon advantages of the digital economy to be highlighted as soon as possible. For cities in the YREB, the government should continue to support innovation and development of digital technology, provide more efficient and secure digital technology platforms, and help China’s industries upgrade to higher levels of the value chain.
The following limitations and future recommendations are acknowledged:
1. Due to data lag, updated data cannot be used for modeling and analysis.
2. The construction of a digital economy indicator system was based on existing literature. In future studies, a more reasonable indicator system to describe the digital economy from multiple perspectives should be constructed.
The datasets presented in this study can be found in online repositories. The names of the repository/repositories and accession number(s) can be found in the article/Supplementary Material.
YS: Methodology, Resources, Writing–original draft, Writing–review and editing. XS: Conceptualization, Data curation, Funding acquisition, Writing–original draft. XC: Formal Analysis, Investigation, Project administration, Writing–review and editing. XL: Supervision, Validation, Visualization, Writing–review and editing.
The author(s) declare that financial support was received for the research, authorship, and/or publication of this article. This work was supported by the National Social Science Fund of China (Grant No. 22BTJ048), Planning Project of China Business Statistics Society (Grant No. 2023STY21), Hefei Xingtai Financial Holding Co., Ltd. Cooperation Special Project (Grant No. 2023XTQTZD02), Campus level scientific research project of Hefei Normal University (Grant No. 2023KYJX07). The funder was not involved in the study design, collection, analysis, interpretation of data, the writing of this article, or the decision to submit it for publication.
The authors declare that the research was conducted in the absence of any commercial or financial relationships that could be construed as a potential conflict of interest.
All claims expressed in this article are solely those of the authors and do not necessarily represent those of their affiliated organizations, or those of the publisher, the editors and the reviewers. Any product that may be evaluated in this article, or claim that may be made by its manufacturer, is not guaranteed or endorsed by the publisher.
The Supplementary Material for this article can be found online at: https://www.frontiersin.org/articles/10.3389/fenrg.2024.1425313/full#supplementary-material
LCSD, low-carbon sustainable development; ISU, industrial structure upgrading; TI, technological innovation; GDP, gross domestic product; ML, marketization level; ER, environmental regulation; YREB, Yangtze River Economic Belt.
Acemoglu, D., and Restrepo, P. (2018). The race between man and machine: implications of technology for growth, factor shares, and employment. Am. Econ. Rev. 108, 1488–1542. doi:10.1257/aer.20160696
Aghion, P., Howitt, P., Brant-Collett, M., and García-Peñalosa, C. (1998). Endogenous growth theory. USA: MIT press.
Asongu, S. A., Le Roux, S., and Biekpe, N. (2018). Enhancing ICT for environmental sustainability in sub-Saharan Africa. Technol. Forecast. Soc. 127, 209–216. doi:10.1016/j.techfore.2017.09.022
Avom, D., Nkengfack, H., Fotio, H. K., and Totouom, A. (2020). ICT and environmental quality in Sub-Saharan Africa: effects and transmission channels. Technol. Forecast. Soc. 155, 120028. doi:10.1016/j.techfore.2020.120028
Berkhout, F., and Hertin, J. (2004). De-materialising and re-materialising: digital technologies and the environment. Futures 36, 903–920. doi:10.1016/j.futures.2004.01.003
Bunje, M. Y., Abendin, S., and Wang, Y. (2022). The multidimensional effect of financial development on trade in Africa: the role of the digital economy. Telecommun. Policy 46 (10), 102444. doi:10.1016/j.telpol.2022.102444
Cheng, Z., Li, L., and Liu, J. (2019). The effect of information technology on environmental pollution in China. Environ. Sci. Pollut. Res. 26, 33109–33124. doi:10.1007/s11356-019-06454-7
Chien, F., Anwar, A., Hsu, C. C., Sharif, A., Razzaq, A., and Sinha, A. (2021). The role of information and communication technology in encountering environmental degradation: proposing an SDG framework for the BRICS countries. Technol. Soc. 65, 101587. doi:10.1016/j.techsoc.2021.101587
Danish, H., Zhang, J., Wang, B., and Latif, Z. (2019). Towards cross-regional sustainable development: the nexus between information and communication technology, energy consumption, and CO2 emissions. Sustain. Dev. 27, 990–1000. doi:10.1002/sd.2000
Dhakal, S. (2009). Urban energy use and carbon emissions from cities in China and policy implications. Energ. Policy 37, 4208–4219. doi:10.1016/j.enpol.2009.05.020
Elavarasan, R. M., Pugazhendhi, R., Irfan, M., Mihet-Popa, L., Campana, P. E., and Khan, I. A. (2022). A novel Sustainable Development Goal 7 composite index as the paradigm for energy sustainability assessment: a case study from Europe. Appl. Energ. 307, 118173. doi:10.1016/j.apenergy.2021.118173
Ghobakhloo, M. (2020). Industry 4.0, digitization, and opportunities for sustainability. J. Clean. Prod. 252, 119869. doi:10.1016/j.jclepro.2019.119869
Guo, L., Tan, W., and Xu, Y. (2022). Impact of green credit on green economy efficiency in China. Environ. Sci. Pollut. Res. 29, 35124–35137. doi:10.1007/s11356-021-18444-9
Hansen, B. (1999). Threshold effects in non-dynamic panels: estimation, testing, and inference. J. Econ. 93, 345–368. doi:10.1016/S0304-4076(99)00025-1
Hickel, J. (2020). The sustainable development index: measuring the ecological efficiency of human development in the anthropocene. Ecol. Econ. 167, 106331. doi:10.1016/j.ecolecon.2019.05.011
Jayaprakash, P., and Pillai, R. R. (2022). The role of ICT for sustainable development: a cross-country analysis. Eur. J. Dev. Res. 34, 225–247. doi:10.1057/s41287-021-00369-1
Jia, J., Fan, Y., and Guo, X. (2012). The low carbon development (LCD) levels’ evaluation of the world’s 47 countries (areas) by combining the FAHP with the TOPSIS method. Expert Syst. Appl. 39 (7), 6628–6640. doi:10.1016/j.eswa.2011.12.039
Jia, J., Gong, Z., Chen, C., Jian, H., and Xie, D. (2018). Urban carbon dioxide equivalent (CO2e) accounting based on the GPC framework. Int. J. Clim. Chang. Str. 10 (5), 812–832. doi:10.1108/IJCCSM-03-2017-0074
Jia, J., Gong, Z., Xie, D., Jian, H., and Chen, C. (2018). Study of urban carbon dioxide equivalent (CO2e) accounting based on the comparable GPC framework: a case of the underdeveloped city, Nanning, China. Environ. Sci. 15 (1), 59–81. doi:10.1080/1943815X.2018.1447970
Jia, J., Xin, L., Lu, C., Wu, B., and Zhong, Y. (2023). China's CO2 emissions: a systematical decomposition concurrently from multi-sectors and multi-stages since 1980 by an extended logarithmic mean divisia index. Energy Strategy Rev. 49, 101141. doi:10.1016/j.esr.2023.101141
Jiang, L., Niu, H., Ru, Y., Tong, A., and Wang, Y. (2023). Can the digital economy promote the development of the energy economy? Evidence from China. Front. Energy Res. 11, 1257794. doi:10.3389/fenrg.2023.1257794
Khan, F. N., Sana, A., and Arif, U. (2020). Information and communication technology (ICT) and environmental sustainability: a panel data analysis. Environ. Sci. Pollut. Res. 27, 36718–36731. doi:10.1007/s11356-020-09704-1
Khan, S. U., Cui, Y., Khan, A. A., Ali, M. A. S., Khan, A., Xia, X., et al. (2021). Tracking sustainable development efficiency with human-environmental system relationship: an application of DPSIR and super efficiency SBM model. Sci. Total Environ. 783, 146959. doi:10.1016/j.scitotenv.2021.146959
Kim, B., Barua, A., and Whinston, A. B. (2002). Virtual field experiments for a digital economy: a new research methodology for exploring an information economy. Decis. Support Syst. 32, 215–231. doi:10.1016/S0167-9236(01)00094-X
Lee, C., Yuan, Z., and Wang, Q. (2022). How does information and communication technology affect energy security? International evidence. Energ. Econ. 109, 105969. doi:10.1016/j.eneco.2022.105969
Li, J., Chen, L., Chen, Y., and He, J. (2021). Digital economy, technological innovation, and green economic efficiency—empirical evidence from 277 cities in China. Manag. Decis. Econ. 43, 616–629. doi:10.1002/mde.3406
Li, T., Han, D., Ding, Y., and Shi, Z. (2020). How does the development of the internet affect green total factor productivity? Evidence from China. IEEE Access 8, 216477–216490. doi:10.1109/ACCESS.2020.3041511
Litvinenko, V. S. (2020). Digital economy as a factor in the technological development of the mineral sector. Nat. Resour. Res. 29, 1521–1541. doi:10.1007/s11053-019-09568-4
Liu, Y., Yang, Y., Li, H., and Zhong, K. (2022). Digital economy development, industrial structure upgrading and green total factor productivity: empirical evidence from China’s cities. Int. J. Env. Res. Pub. He. 19, 2414. doi:10.3390/ijerph19042414
Lou, Y., Jayantha, W. M., Shen, L., Liu, Z., and Shu, T. (2019). The application of low-carbon city (LCC) indicators—a comparison between academia and practice. Sustain. Cities Soc. 51, 101677. doi:10.1016/j.scs.2019.101677
Luo, K., Liu, Y., Chen, P., and Zeng, M. (2022). Assessing the impact of digital economy on green development efficiency in the Yangtze River Economic Belt. Energ. Econ. 112, 106127. doi:10.1016/j.eneco.2022.106127
Luo, S., Yimamu, N., Li, Y., Wu, H., Irfan, M., and Hao, Y. (2023). Digitalization and sustainable development: how could digital economy development improve green innovation in China? Bus. Strateg. Environ. 32, 1847–1871. doi:10.1002/bse.3223
Ma, D., and Zhu, Q. (2022). Innovation in emerging economies: research on the digital economy driving high-quality green development. J. Bus. Res. 145, 801–813. doi:10.1016/j.jbusres.2022.03.041
Mondejar, M. E., Avtar, R., Diaz, H. L. B., Dubey, R. K., Esteban, J., Gómez-Morales, A., et al. (2021). Digitalization to achieve sustainable development goals: steps towards a smart green planet. Sci. Total Environ. 794, 148539. doi:10.1016/j.scitotenv.2021.148539
Nuccio, M., and Bertacchini, E. (2022). Data-driven arts and cultural organizations: opportunity or chimera? Eur. Plan. Stud. 30, 1638–1655. doi:10.1080/09654313.2021.1916443
Nunn, N., and Qian, N. (2014). U S food aid and civil conflict. Am. Econ. Rev. 104, 1630–1666. doi:10.1257/aer.104.6.1630
Pan, W., Xie, T., Wang, Z., and Ma, L. (2022). Digital economy: an innovation driver for total factor productivity. J. Bus. Res. 139, 303–311. doi:10.1016/j.jbusres.2021.09.061
Qu, Y., and Liu, Y. (2017). Evaluating the low-carbon development of urban China. Environ. Dev. Sustain. 19, 939–953. doi:10.1007/s10668-016-9777-8
Ren, S., Hao, Y., Xu, L., Wu, H., and Ba, N. (2021). Digitalization and energy: how does internet development affect China's energy consumption? Energ. Econ. 98, 105220. doi:10.1016/j.eneco.2021.105220
Shao, W., Yang, K., and Jin, Z. (2023). How the carbon emissions trading system affects green total factor productivity? A quasi-natural experiment from 281 Chinese cities. Front. Energy Res. 10, 895539. doi:10.3389/fenrg.2022.895539
Shen, L., Du, X., Cheng, G., and Wei, X. (2021). Capability maturity model (CMM) method for assessing the performance of low-carbon city practice. Environ. Impact Asses 87, 106549. doi:10.1016/j.eiar.2020.106549
Shobande, O. A., and Ogbeifun, L. (2022). Has information and communication technology improved environmental quality in the OECD? —a dynamic panel analysis. Int. J. Sust. Dev. World 29, 39–49. doi:10.1080/13504509.2021.1909172
Sun, J., Tang, D., Kong, H., and Boamah, V. (2022). Impact of industrial structure upgrading on green total factor productivity in the Yangtze River Economic Belt. Int. J. Env. Res. Pub. He. 19, 3718. doi:10.3390/ijerph19063718
Sun, X., Jiang, K., Cui, Z., Xu, J., and Zhao, X. (2023). Exploring the impact of the digital economy on green total factor productivity in China: a spatial econometric perspective. Front. Environ. Sci. 10, 1097944. doi:10.3389/fenvs.2022.1097944
Tan, L., Yang, Z., Irfan, M., Ding, C. J., Hu, M., and Hu, J. (2023). Toward low-carbon sustainable development: exploring the impact of digital economy development and industrial restructuring. Bus. Strateg. Environ. 33, 2159–2172. doi:10.1002/bse.3584
Tan, S., Yang, J., Yan, J., Lee, C., Hashim, H., and Chen, B. (2017). A holistic low carbon city indicator framework for sustainable development. Appl. Energ. 185, 1919–1930. doi:10.1016/j.apenergy.2016.03.041
Tapscott, D. (1996). The digital economy: promise and peril in the age of networked intelligence. New York, NY: Mc Graw-Hill.
Tranos, E., Kitsos, T., and Ortega-Argiles, R. (2020). Digital economy in the UK: regional productivity effects of early adoption. Reg. Stud. 55, 1924–1938. doi:10.1080/00343404.2020.1826420
Ulucak, R., Danish, L., and Khan, S. U. D. (2020). Does information and communication technology affect CO2 mitigation under the pathway of sustainable development during the mode of globalization? Sustain. Dev. 28, 857–867. doi:10.1002/sd.2041
Vu, K. M. (2013). Information and communication technology (ICT) and Singapore’s economic growth. Inf. Econ. Policy 25, 284–300. doi:10.1016/j.infoecopol.2013.08.002
Wan, Q., Chen, J., Yao, Z., and Yuan, L. (2022). Preferential tax policy and R&D personnel flow for technological innovation efficiency of China's high-tech industry in an emerging economy. Technol. Forecast. Soc. 174, 121228. doi:10.1016/j.techfore.2021.121228
Wang, H., Cui, H., and Zhao, Q. (2021). Effect of green technology innovation on green total factor productivity in China: evidence from spatial durbin model analysis. J. Clean. Prod. 288, 125624. doi:10.1016/j.jclepro.2020.125624
Wang, J., Dong, K., Dong, X., and Taghizadeh-Hesary, F. (2022). Assessing the digital economy and its carbon-mitigation effects: the case of China. Energ. Econ. 113, 106198. doi:10.1016/j.eneco.2022.106198
Wen, H., Liang, W., and Lee, C. C. (2023). China's progress toward sustainable development in pursuit of carbon neutrality: regional differences and dynamic evolution. Environ. Impact Asses. Rev. 98, 106959. doi:10.1016/j.eiar.2022.106959
Yang, Y., Jia, J., Devlin, A. T., Zhou, Y., Xie, D., and Ju, M. (2020). Decoupling and decomposition analysis of residential energy consumption from economic growth during 2000-2017: a comparative study of urban and rural Guangdong, China. Energies 13 (17), 4461. doi:10.3390/en13174461
Zhang, J., Lyu, Y., Li, Y., and Geng, Y. (2022). Digital economy: an innovation driving factor for low-carbon development. Environ. Impact Asses. 96, 106821. doi:10.1016/j.eiar.2022.106821
Zhao, P., Zeng, L., Lu, H., Zhou, Y., Hu, H., and Wei, X. (2020). Green economic efficiency and its influencing factors in China from 2008 to 2017: based on the super-SBM model with undesirable outputs and spatial Dubin model. Sci. Total Environ. 741, 140026. doi:10.1016/j.scitotenv.2020.140026
Zhou, R., Tang, D., Da, D., Chen, W., Kong, L., and Boamah, V. (2022). Research on China’s manufacturing industry moving towards the middle and high-end of the GVC driven by digital economy. Sustainability 14, 7717. doi:10.3390/su14137717
Keywords: digital economy, low-carbon sustainable development, industrial structure, technological innovation, threshold effect
Citation: Song Y, Sun X, Cai X and Liu X (2024) Impacts of the digital economy on urban low-carbon sustainable development. Front. Energy Res. 12:1425313. doi: 10.3389/fenrg.2024.1425313
Received: 29 April 2024; Accepted: 03 July 2024;
Published: 26 July 2024.
Edited by:
Ying Sun, Qingdao University of Technology, ChinaReviewed by:
Aravind C. K., Vellore Institute of Technology (VIT), IndiaCopyright © 2024 Song, Sun, Cai and Liu. This is an open-access article distributed under the terms of the Creative Commons Attribution License (CC BY). The use, distribution or reproduction in other forums is permitted, provided the original author(s) and the copyright owner(s) are credited and that the original publication in this journal is cited, in accordance with accepted academic practice. No use, distribution or reproduction is permitted which does not comply with these terms.
*Correspondence: Xin Sun, eGluc3VuXzhAMTYzLmNvbQ==
Disclaimer: All claims expressed in this article are solely those of the authors and do not necessarily represent those of their affiliated organizations, or those of the publisher, the editors and the reviewers. Any product that may be evaluated in this article or claim that may be made by its manufacturer is not guaranteed or endorsed by the publisher.
Research integrity at Frontiers
Learn more about the work of our research integrity team to safeguard the quality of each article we publish.