- Institute of Chemical, Environmental and Bioscience Engineering, TU Wien, Vienna, Austria
Carbon Capture, Storage and Utilization (CCS/CCU) is critical for achieving net-zero emissions. Although the recent surge in CCS/CCU projects announcement, there is a clear gap between announced capacity (around 400 Mt CO₂ per year) and the Net Zero Emissions (NZE) scenario deployment target (around 1 Gt per year) by 2030. This review examines breakthroughs and advancements across both established and emerging CCS/CCU systems with different Technology Readiness Levels (TRLs) in various industrial sectors, emphasizing the necessity of prospective assessments for their acceleration and scalability. It examines the development and application of prospective Life Cycle Assessment (pLCA) and prospective Techno-Economic Assessment (pTEA), highlighting their limitations and importance of their outcomes in decision-making processes. Differences between the evolving dynamics of the technological systems (foreground) and the evolution of the overall socioeconomic system (background) are discussed. Incorporating scenario data from Integrated Assessment Models (IAMs) into pLCA and pTEA reveals an iterative relationship that significantly influences the outcome of both the environmental assessments and the economics of large-scale production of the CCS/CCU systems under study. This, in turn, could reshape investment strategies towards advanced technologies, necessitating their consideration within the evolving structure of IAMs. It is concluded that the inherent limitations of CCS/CCU technologies at an early stage of development require quantitative uncertainty analysis and demand robustness, interdisciplinary collaboration, policy intervention, and data transparency. The rigorous evaluative frameworks are key for developing economic, environmental and climate policies and enable well-informed decisions across rapidly evolving sectors. A framework is proposed in this review, outlining a multistep process that includes a series of databases and open-source tools to interface pTEA and pLCA with enhanced IAMs for CCS/CCU, demonstrating its potential to improve decision-making and policy development.
1 Introduction
Limiting human-caused global warming requires net zero CO₂ emissions (IPCC, 2023). Carbon Capture, Storage and Utilization (CCS/CCU), or CCUS, plays a significant role to decarbonize hardto-abate industrial sectors and achieve net negative CO₂ emissions (IEA, 2023a). The IPCC Special Report on 1.5°C highlights that substantial application of CCS/CCU is projected in three out of four pathways, potentially capturing between 350 and 1200 Gigatons of CO₂ by 2100 (IPCC, 2022). The United Nations’ Sustainable Development Goals (SDGs) include 17 principles aimed at global sustainable development. In particular, CCS/CCU is primarily contributing to Climate Action (Goal 13), Industry, Innovation, and Infrastructure (Goal 9) and Sustainable Cities and Communities (Goal 11) (United Nations, 2015), since it has high mitigation potential for point source emissions from fossil-based energy and challenging industrial processes where alternative technologies are limited or underdeveloped. When CO₂ is captured directly from the atmosphere (DACCS), or biomass (BECCS), CCS provides the storage infrastructures of these Carbon Dioxide Removal (CDR) methods. CCU is attractive because of its ability to substitute conventional carbon-intensive production routes and offsets high capture costs (Mac Dowell et al., 2017), especially for the production and use of chemicals. However, it is crucial to consider how the deployment of CCS/CCU might impact other SDGs, and any potential adverse effects should be mitigated through life cycle and prospective assessments that respect planetary boundaries (Rocha and Costa, 2021; Ioannou et al., 2023a).
Despite the recent surge in announcements for CO₂ capture, transport, storage, and full-chain projects (Global CCS Insitute, 2023), global rates of CCS/CCU deployment are far below than in modelled pathways limiting global warming to 1.5°C and 2°C (IEA, 2023a). This is critical in hard-to-abate industrial sectors where reducing greenhouse gas emissions is particularly challenging due to the integral role of carbon in their production processes and unavailability of avoidance and alternative options currently. These sectors include steel, cement, and chemicals, which collectively contribute to approximately 70% of total industrial emissions. The term hard-to-abate highlights the importance of reducing emissions and increasing energy efficiency first. This approach aims to ensure efforts to cut emissions are not weakened and that we avoid investing in outdated, fossil fuel-based technologies that might soon become worthless. It also helps prevent getting stuck with old, polluting infrastructure, making the shift to cleaner alternatives easier and more effective. CCS/CCU technologies not only span a range from early development to fully operational stages, but also exhibit considerable variation in their application and maturity across different sectors. The oil and gas sector exhibit a higher level of CCS maturity compared to the power sector, the cement, and chemical industries, where, for example, captured CO₂ is utilized in Enhanced Oil Recovery (EOR), this additional oil production can offset some of the costs associated with CCS/CCU, making it more economically viable for the industry. The large-scale implementation of CCS/CCU is hindered by a range of barriers spanning technological, economic, institutional, environmental, and social dimensions (IPCC, 2023). Technical challenges include enhancing the efficiency of capture technologies to improve capture rates while minimizing energy demands, and devising strategies to reduce the significant capital and operational expenses associated with CCS/CCU projects. Additionally, the identification of suitable storage sites and uncertainty regarding the permanence of ocean storage present significant challenges. The development of extensive transportation infrastructure and the establishment of robust regulatory frameworks for implementating and managing CCS projects that span national borders are also crucial. These frameworks must define long-term responsibilities for storage sites, address potential leakage issues and establish protocols for medicating international treaties, which may not currently be equipped to handle issues specific to CCS as a zero- or low-emission mechanism. Additionally, CCS was incorporated as a Clean Development Mechanism (CDM) under the Kyoto Protocol, enabling projects to generate certified emission reduction (CER) credits, thus promoting global CCS technology adoption to mitigate GHGs (Arlota and Costa, 2021b). However, there still exist legal aspects that need to be considered and regulatory gaps that need to be clarified to achieve the large-scale of CCS/CCU. For example, Frattini et al., 2022 pointed out the lack of actions taken by countries towards the provisional application of the London Protocol and the lack of inclusion of CO2 transport modes other than pipelines in European legislation. From a social perspective, Arlota and Costa, 2021a states that policymakers and stakeholders must address potential injustices associated with low-carbon technologies, such as corporate takeover of community resources and poor labour conditions, by integrating considerations of gender, housing, and socioeconomic structures into energy policies. Integrating climate justice into CCS/CCU legislation promotes sustainable development by addressing environmental, social, and economic dimensions simultaneously, in particular the disproportionate impact of climate change on vulnerable, low-income groups due to inadequate urban infrastructure.
Techno-Economic Assessment (TEA) and Life Cycle Assessment (LCA) are crucial for providing systematic, quantitively, and comprehensive insights to decision-makers regarding CCS/CCU systems (Zimmermann et al., 2020; Roussanaly et al., 2021; Skone et al., 2022). However, given the rapid technological evolution and dynamic development of socioeconomic environment there is a growing need for prospective assessment methods that can incorporate these changes from a mid-to long-term perspective that matches the application horizon of the investigated technological systems (Sacchi et al., 2022). Prospective TEA (pTEA) and prospective LCA (pLCA) have attracted significant attention and development in recent years especially for emerging low-carbon technologies (Cox et al., 2018; Thomassen et al., 2020; Langkau et al., 2023). Projections of technological change play a critical factor in analysis of alternative futures and the impacts of policy interventions to address global climate change (Luderer et al., 2019). With the urgency to reduce greenhouse gas (GHG) emissions, the energy sector and various industries are undergoing swift transformations. This context renders prospective LCA particularly valuable for assessing the environmental performance of rapidly developing technologies, including those that are emerging or yet to be developed (Sacchi et al., 2022). Prospective application of TEA and LCA at low TRLs can enable technology developers to understand the implications of different design choices on future performances (i.e., technical, economic, and environmental) of an emerging technology. This approach is instrumental to reduce costs, avoid environmental consequences, and prevent regrettable investments by supporting technology developers to optimize different parameters without major disruptions.
Several quantitative models have been developed to study systemwide human and natural systems and the effects of various mitigation technologies and public policies on projections of future climate change, which have become well-known as “Integrated Assessment Models (IAMs)” (Weyant, 2017). With the rapid deployment and expansion of renewable energy in energy systems and technological evolution of mitigation technologies, there is a growing call for more open source, transparent and flexible integrated models (Brown et al., 2018; Middleton et al., 2020).
There are various aspects that emphasize the necessity to implement prospective assessments for CCS/CCU systems. The rapid expansion of renewable energy sources introduces volatility and uncertainty into electricity mix projections (Gaugl et al., 2023). The presence of competitive and diverse mitigation options in transportation (Smit et al., 2024), heating (Madeddu et al., 2020), and industrial processes (Georgiades et al., 2023; Lei et al., 2023) adds to the uncertainty and dynamism of integrating relevant components into CCS/CCU systems. Furthermore, the evolving nature of alternative technologies for CO₂ capture, storage, transport, and utilization across different technology readiness levels (Dziejarski et al., 2023) along the value chains complicates this landscape. The growing consumer demand for sustainable and low-carbon footprint products further influences the market projection of CO₂-based products (Gabrielli et al., 2023). The feasibility and scalability of projects highly depend on regional carbon pricing policies (Becattini et al., 2022) and supporting infrastructures (Burger et al., 2024). As technologies that are energy-intensive and intricately connected with industries, the projection of both background and foreground systems directly impacts the prospective assessment from technological, economic, environmental, and social perspectives across the entire value chains of CCS/CCU (Lamers et al., 2023). Capturing the dynamic changes and technological evolution of these systems and considering their interactions poses significant challenges. Additionally, all existing and continuously evolving regulatory barriers or opportunities, as well as social perspectives on justice, must be considered in prospective assessments to provide a more accurate and comprehensive analysis of the potential impacts and benefits of large-scale CCS/CCU technologies. Furthermore, IAMs should encompass wider regions and account for differences in current legal frameworks to more precisely simulate the economic, environmental, and social outcomes of CCS/CCU projects. This approach helps policymakers understand the implications of international agreements on CCS/CCU deployment, facilitating informed decision-making and promoting the adoption of best practices across different regions. Ultimately, this leads to more effective climate mitigation strategies and contributes to achieving SDGs with minimal burden-shifting. This review thoroughly examines the overview and developments of prospective assessment methodologies for established and emerging CCS/CCU technologies (see Figure 1). The introduction provides an insight in prospective methodologies for assessing CCS/CCU technologies deployment. Section 2 explores recent breakthroughs and progress in CCS/CCU technologies and projects across the spectrum of TRLs over time, emphasising on the contribution of quantitative prospective assessment in promoting scalability. In Section 3, a distinction between foreground and background systems underlines the dynamic changes and interconnections within prospective assessments of CCS/CCU systems. Sections 4, 5 analyse the developments of pTEA and pLCA respectively, introducing their importance and limitations in adapting to rapid technological evolution and dynamic socioeconomic environments. Section 6 provides an overview of the pioneering research and framework in integrating scenario data from IAMs with pLCA and pTEA methodologies and applications in CCS/CCU. Furthermore, Section 6 summarises the available open-source tools and databases, addressing the challenges with uncertainties, consistency, transparency, and flexibility. Finally, the review concludes by underscoring the challenges and limitations of prospective assessment in scaling-up of CCS/CCU technologies, identifying areas for further development.
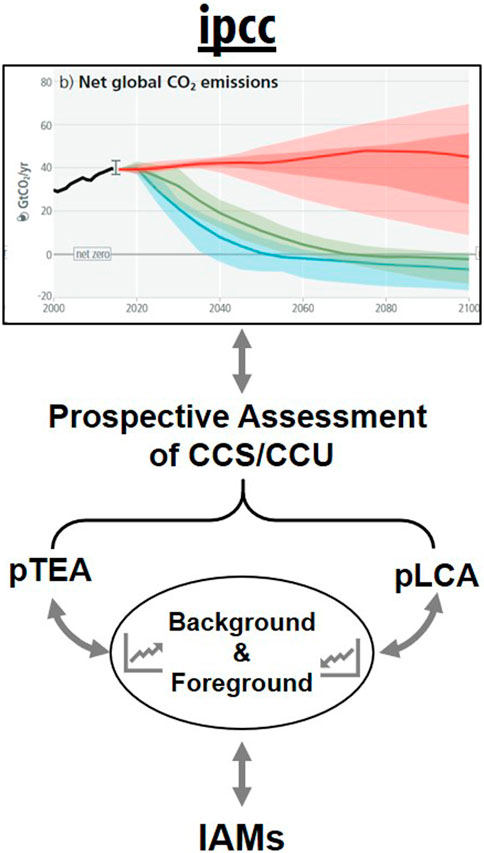
Figure 1. Network showcasing connections and feedback between IPCC and prospective assessment for CCS/CCU. pLCA and pTEA represent interconnectedness of prospective environmental and economic insights. The feedback loops between pLCA/pTEA and IAMs highlight the iterative process of incorporating dynamic changes from background and learning from foreground technology scaling to refine socioeconomic scenarios.
The main objective of this paper is to highlight the significant role and implications of utilizing pLCA and pTEA methodologies, in conjunction with IAMs scenario data, for evaluating the potential of emerging CCS/CCU technologies. This study sheds light on how these assessments should be conducted, suggesting the importance of considering the unique contexts of different CO₂-emitting industries to avoid relying solely on a single solution, henceforth, directing the decision-making process across evolving sectors and advancing towards a comprehensive understanding of how deploying specific CCS/CCU technologies will impact the background system, potentially influencing it in a feedback loop. To solve this complexity, this review provides insights on the importance of interdisciplinary collaboration, system integration, consistency, and transparency of data for emission mitigation technologies in driving the transformation of society-wide systems to deal with climate change.
2 Development in CCS/CCU
Despite CCS/CCU projects beginning in the 1970s and 1980s, their broad adoption has been sluggish, particularly between 2010 and 2020. This period was marked by unexpected costs and delays due to economic challenges and lack of policies, alongside the rising competitiveness of renewable energy technologies (Energy Transitions Commission, 2022). Yet, the landscape has considerably shifted in recent years, thanks to significant policy developments that have boosted the potential for CCS/CCU project investments. As shown in Figure 2, by July 2023, 41 commercial capture facilities and 392 projects in the pipeline around the world are applying CCS/CCU to industrial processes, fuel transformation and power generation. The sector witnessed a 102% increase in projects under development compared to the previous year, reaching a total capture capacity of 361 Mt. The leading countries in CCS/CCU project development include the US, UK, Canada, China, and Norway, primarily focusing on natural gas processing, hydrogen production, refining and coal-to-gas plants (IEA, 2023a; Global CCS Insitute, 2023).
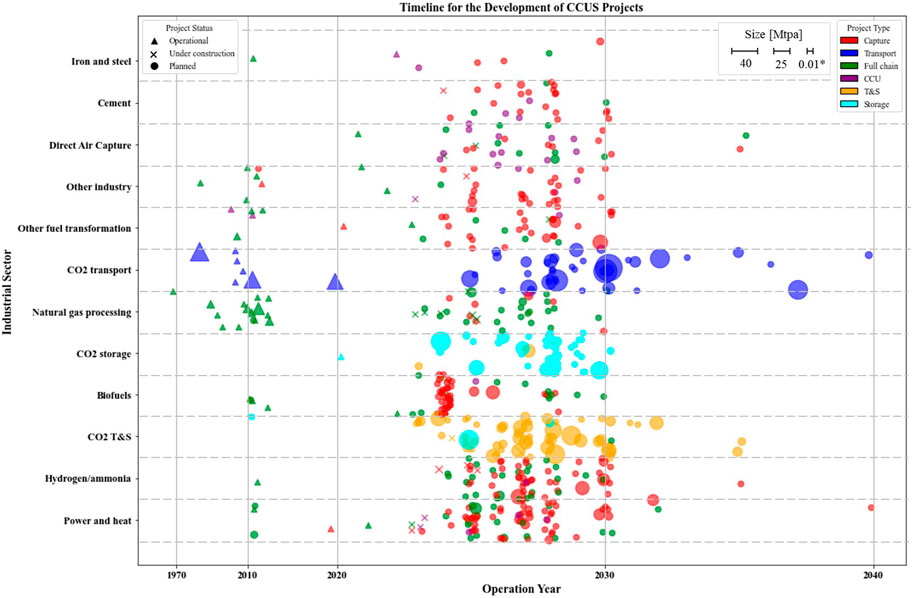
Figure 2. Timeline for the development of CCS/CCU projects (Data from IEA, 2023a). The smallest scale in the plot with the marker represents unknown capacity of announced projects. Industrial Processes include Iron and steel, Cement, Natural gas process, Hydrogen/ammonia, and other industry; Fuel Transformation covers other fuel transformation and Biofuels; Power Generation is represented by Power and heat. CCU/CCS specific processes include Direct Air Capture, CO₂ transport, CO₂ storage, and CO₂ T & S (Transport & Storage).
The evolution of CCS/CCU projects has also seen a shift from vertical integration towards networked deployments, which offer economies of scale and reduced risks. Capture hubs and transport networks are being facilitating to service regional CO₂ source solutions (Global CCS Insitute, 2023). Power and heat generation, hydrogen/ammonia production, biofuels and natural gas processing are leading sectors in CCS adoption, with cement emerging as the fastest-growing industry. Additionally, there are more than 30 DAC plants in development (IEA, 2023a). The hard-to-abate sectors such as cement (Gallego Dávila et al., 2023), oil-refinery (Ioannou et al., 2023b) and aviation (Sacchi et al., 2023a) are positively exploring the potential of CO₂ utilization and/or DAC in various applications, including methanol, synthetic fuels, mineralization and plastics, highlighting the role of CO₂ utilization and recycling in achieving net-zero emissions.
Despite this progress, a significant portion of CCS/CCU projects remains in the early stages of development, revealing a discrepancy between the deployment announcements (around 400 Mt CO₂ per year) and the targets set for Net Zero Emissions scenarios (around 1 Gt CO₂ per year) by 2030. The existing gap underscores the urgent need for enhanced policy support, public engagement, and technological innovation to meet the ambitious goals of limiting global warming to 1.5°C–2°C. Moreover, the vast potential capacity for CO₂ storage exceeding 1,000 Gt, presents a significant opportunity to mitigate climate change impacts. However, achieving this potential directly depends on the rapid and sustained development and deployment of CCS/CCU technologies to match the pace required for substantial emission reductions in line with global climate targets (IEA, 2023a; Calvin et al., 2023).
To accelerate the deployment of CCS/CCU, policy responses must be tailored to national and sector-specific circumstances. Countries that produce a considerable number of hydrocarbons (Algeria, Brazil, Canada, Japan, Norway, Saudi Arabia, United Arab Emirates, United Kingdom, and United States) or countries that refine it in considerable quantities (China, Brazil, Canada, Japan, Saudi Arabia, and United States) present a trend in the installation and operation of CCS facilities and can serve as a state-of-the art in regulations and deployment for the rest of the countries (Nunes and Costa, 2021). These countries developed roadmaps that converge on several critical aspects: 1) improve legal situations, 2) support rapid ramp-up with flagship projects by 2030, 3) promote research and development, 4) strength international and regional cooperation, 5) incentivize CO₂ capture in hard-to-abate sectors, 6) support infrastructure development by 2040, 7) strengthen public awareness and acceptance, etc. The IEA projects that globally, over $160 billion in cumulative investment is needed in CCS/CCU by 2030 to support its role in achieving climate targets (IEA, 2023a). These efforts must be complemented by robust policies and legal frameworks. Rapidly changing environments and adjusting policies pose challenges for prospective assessments of CCS/CCU technologies, which require a higher regional and temporal resolution background system, as well as a wider range of foreground technology systems to support comprehensive analysis. Technology development typically advances through various TRLs, from initial concept and discovery in the lab (TRL 1–3), through larger lab-scale development (TRL 4–5), to pilot-scale testing (TRL 6), demonstration (TRL 7), the need for commercial refinement requirements (TRL 8) and finally, commercial scale (TRL 9). CCS/CCU systems involve multiple components at varying TRLs as shown in Figure 3, especially for CO₂ capture and utilization technologies, which underscores the complex nature of CCS/CCU systems (Bui et al., 2018).
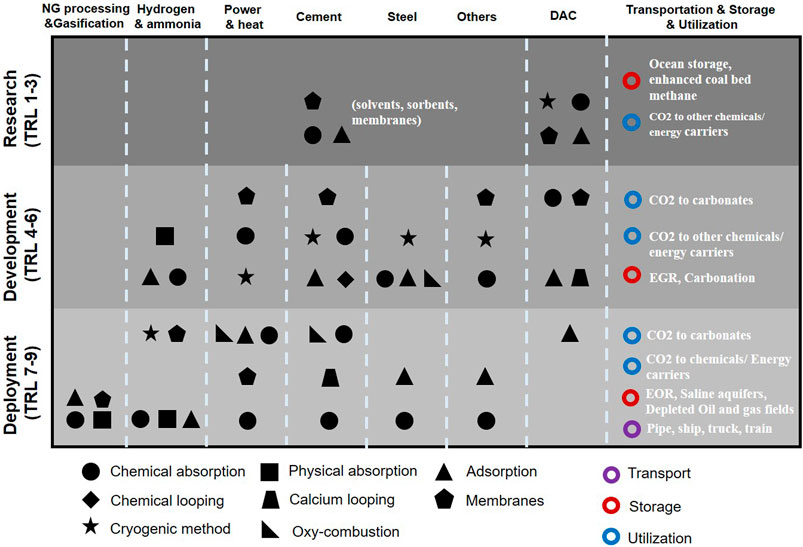
Figure 3. TRLs of CO₂ Capture, Transport, Storage and Utilization technologies (Sources: Global CCS Insitute, 2021; Air Liquide, 2022; Dziejarski et al., 2023; Bouaboula et al., 2024).
For CO₂ capture, amine-based chemical absorption has been a cornerstone, widely utilized in natural gas processing to separate CO₂ from methane since the 1930s. In the 1940s, physical absorbents like Selexol and Rectisol began their application in coal or biomass gasification plants. The development of adsorption technologies, leveraging solid sorbents for hydrogen production and ammonia synthesis, emerged in the 1950s and 1960s. The 1980s saw the introduction of membrane technologies in the natural gas industry, aimed at enhancing CO₂ capture efficiency (Global CCS Insitute, 2021).
As the focus on decarbonization increased, traditional chemical absorption techniques have been applied in power generation and other industrial sectors. Concurrently, a series of innovative technologies is under research and development, aiming to boost capture performance and reduce associated costs. Among these, the cryogenic approach, noted for its superior CO₂ recovery and purity, has garnered interest, often complemented by advanced processes like polymeric membrane techniques, currently at TRL 6 (Air Liquide, 2022). Active research areas such as Chemical Looping Combustion (CLC) and calcium looping, particularly relevant for the cement industry, are making strides with CLC evaluated at TRL 5-6 and calcium looping slightly further along at TRL 6-8 (Global CCS Insitute, 2021). A diverse spectrum of solvents for chemical or physical absorption is under development across various TRLs, including ionic liquids (TRL 2-3), phase-change solvents (TRL 5-6), water-lean solvents (TRL 4-7), and the chilled ammonia process (TRL 6-7). Similarly, an array of membrane materials is under investigation, with notable advancements like the commercial-scale membrane system (TRL 4-7) developed by Membrane Technology and Research for power plants (DOE-EPRI, 2020). The past decade has also seen considerable progress in adsorption technologies, attracted by their potential for high capacity at low temperatures and the promise of purer products through fewer processing steps. Materials such as activated carbon, silica, alumina, Metal-Organic Frameworks (MOFs), and zeolites are being applied in industries including cement, ammonia, methanol, and steel, with TRLs ranging from 5 to 8 (Dziejarski et al., 2023). Notably, Svante’s VeloxoTherm system, based on a rapid-cycle temperature swing adsorption process, demonstrates the potential of emerging adsorption technologies (TRL 5-7). Breakthrough technologies that enable inherent CO₂ capture, requiring no additional energy for CO₂ separation, such as the Allam-Fetvedt power generation cycle and the Calix Advanced Calciner for lime and cement production, offer cost reductions, facing however considerable challenges for retrofitting existing plants (Global CCS Insitute, 2021).
For achieving net negative CO₂ emissions, Direct Air Capture (DAC) technologies have attracted considerable attention. DAC’s versatility allows for deployment without geographical constraints, with methods based on solid or liquid absorbents reaching pilot or demonstration scales, such as hydroxide-carbonate and temperature-vacuum-swing adsorption (TRL 6-7). Research continues into other DAC technologies, including amine-based absorption (TRL 3-4), electrolysis (TRL 1-4), bipolar-membrane electrodialysis (BPMED) (TRL 2-4), and cryogenic methods (TRL 1-3) (Bouaboula et al., 2024).
CO₂ transport, encompassing offshore and onshore methods such as highways, railroads, pipelines, and ships, has universally achieved TRL 9, facilitating commercial-scale operations (Global CCS Insitute, 2021). In CO₂ storage, technologies like CO₂-EOR and saline formations are commercially implemented (TRL 9), while depleted oil and gas fields, and enhanced gas recovery (EGR) methods are undergoing demonstration (TRL 6-8). Less conventional storage options, such as the carbonation of CO₂ in basalts demonstrated by the CarbFix project in Iceland (Carbfix, 2024) and the Wallula project in the United States (McGrail et al., 2017), are under development.
Lastly, CO₂ utilization aims to recycle captured CO₂ into various products, such as solvents, fuels, carbonates, polymers, and other products. Mature technologies are employed across the fertilizer, food, beverage, and chemical industries, producing items like methanol and CO₂-based polycarbonates, polyurethanes, and urea, achieving TRL 9 (Hepburn et al., 2019). Technologies under development span conversion processes for energy carriers like methane (TRL 8), ethanol (TRL 6), formic acid (TRL 6), syngas (TRL 6), Fischer-Tropsch products (TRL 5), and raw materials such as dimethyl carbonate (TRL 8), dimethyl ether (TRL 3), carbamates (TRL 2), fine chemicals (TRL 1-3) and others at varying TRL from 1 to 8 (Dziejarski et al., 2023). Mineral carbonation processes for products like magnesium carbonates and sodium bicarbonates, including concrete curing, show progress from TRL 4 to TRL 8 (Chauvy and De Weireld, 2020).
3 System description of CCS/CCU
The CCS/CCU framework is characterized by its multifaceted operational segments including CO₂ sources, capture mechanisms, compression stages, transportation logistics, utilization pathways, and ultimate sequestration (IEA, 2020). However, a comprehensive description of the framework is not complete without considering energy supply, materials, infrastructures, policy support and social acceptance (Jin et al., 2017; Dalla Longa et al., 2020). In Figure 4, we introduce a schematic diagram to illustrate the foreground and background systems within CCS/CCU frameworks.
A foreground process includes technology developments, technological learning, and the technology diffusion, which are directly impacted by changes in the production volume of the system under investigation. For CCS/CCU systems, it encompasses the direct operational elements for the capture, conditioning, temporary storage, transportation, and final storage or utilization of CO₂. These components are directly influenced by production volume changes resulting from the adoption or enhancement of CCS/CCU technologies (Hasan et al., 2022). The precision in defining these elements enhances the clarity and applicability of LCA and TEA. The functional unit is a quantitative reference to describe the service or function that a system provides, and usually it is linked to the foreground system through the production volume, to which all data are normalized. It is an essential concept in the LCA study that ensures comparability across different systems or products (Tillman, 1998). It is key to keep it consistent across TEA to provide a common basis for measuring and assessing environmental and economic impacts.
On the other hand, a background process is defined as one where production volumes remain unchanged, or are only indirectly influenced (e.g., via market dynamics), following a shift in demand due to the change being analysed. Thus, the background system includes the broader context necessary for CCS/CCU deployment, such as energy supply, materials, infrastructures, policy support, and social acceptance (Turgut et al., 2021). A notable debate arises regarding the classification of CO₂ emissions from specific emission sources. Differentiating foreground from background systems is crucial to select an appropriate functional unit for analysis. When considering DACCS process, the CO₂ sourced from the atmosphere, which arises from various dispersed sources, human activities included, is considered part of the background system. For DACCS process, a fitting functional unit could be “1 kg of net CO₂ removed and stored”, which reflects the contribution of DACCS to lowering atmospheric CO₂ level. This way of defining the functional unit aligns with the background nature of the CO₂ sources for DACCS, and implies that scaling up the operation, proportionally increases the environmental impact assessment, while maintaining the nature of the CO₂ source (Liu et al., 2020). Conversely, CO₂ captured and storage from point sources, such as power plants or industrial facilities, is considered part of the foreground system, since emission of CO₂ is a direct result of the foreground activities (e.g., electricity generation, cement production). Integrating CO₂ capture technologies at these sites directly impacts the volume of emissions avoided, linking the functional unit closely with specific capacity of foreground operations. As further discussed in Section 5, a more suitable functional unit could be “1 kg of net CO₂ emissions avoided directly from the point sources and stored”. In both cases, if CO2 is utilized instead of stored, the functional unit of the whole CCU system is preferably “1 kg of CO2 treated”. In this case, the main net CO2 savings arise from the avoidance of production from fossil resources for the product to which CO2 was converted. Depending on the end-of-life of this product (e.g., incineration or storage), the 1 kg treated may or may not be considered as CO2 saving.
The distinction between foreground and background systems aids in structuring these assessments, allowing for a focused analysis of the operational units while considering the broader environmental and socio-economic impacts (Luderer et al., 2019). It is essential to define the system boundaries clearly to perform a sounding LCA and TEA, typically encompassing the full life cycle from cradle-to-grave (Tanzer and Ramírez, 2019; Zimmermann et al., 2020).
The interaction between foreground and background systems is crucial for the overall performance and impact of CCS/CCU technologies. The background system dictates the availability and sustainability of resources and feedstock (e.g., CO2 for CCS/CCU) required for the foreground operations (Slameršak et al., 2022). Policy support and regulatory frameworks within the background system (as described in Section 1 and Section 2) can significantly influence the deployment and scalability of CCS/CCU technologies. Additionally, the socioeconomic context, including market dynamic and societal acceptance, affects the viability and success of these technologies (IEA, 2023b). The energy and materials required for CCS/CCU technologies have their own environmental footprints (Volkart et al., 2013; Marchese et al., 2021). Assessing these impacts is essential for ensuring that the foreground system contributes positively to environmental goals. Especially in the context of the rapidly evolving energy systems, the scenarios of background system will directly affect the demand and performance of foreground system (Qiu et al., 2022). Therefore, a thorough assessment of the background system is indispensable for enhancing the performance of foreground system in CCS/CCU technologies. Understanding this interplay is essential for optimizing CCS/CCU technologies and ensuring their contribution to environmental goals.
CCU technologies exhibit significant variability in their LCA results, which hinders their utility in informing decision-making processes. This variability is attributed to the dual nature of CO₂, which serves both as an emission to be mitigated and a feedstock for creating value-added products (Heijungs and Frischknecht, 1998; Von Der Assen et al., 2013). Despite the standardization of LCA (ISO 14040, 2006a; ISO 14044, 2006b) the guidelines offer flexibility in methodological choices. While allowing for adaptability to specific study contexts, this flexibility often leads to inconsistencies across LCA studies on CCU technologies, making them challenging to compare. Thonemann and Pizzol (2019) and Müller et al. (2020) introduced a decision-making framework for defining functional units, system boundaries, background processes, and environmental impact assessment methods. Such guidelines should clarify ambiguities and identify common pitfalls in assessing CCU enhancing the transparency, comparability, and reliability of LCA studies for CCU technologies. They pointed out that a cradle-to-gate method is sufficient when performing an LCA of CO₂-based products, to be compared to their conventional counterparts (i.e., fossil based products), when these products and fuels present the same chemical structure and composition. This approach ensures that the assessment focuses on the production processes differences and inputs up to the factory gate, making it a suitable method for such comparative analyses. However, they also caution that in cases where the end-use of CO₂-based products, especially in their early developmental stages, remains uncertain, excluding combustion from the analysis might still be necessary.
The prospective assessment for CCS/CCU aligns with the overarching goal of pLCA: to project the future potential impacts of technologies by considering changes over time in both foreground and background systems and scenarios. This concept of system division is not only applicable to environmental impact assessment, but also to economic and social impacts assessment. The division of CCS/CCU systems into foreground and background components, coupled with the delineation of system boundaries, has significant implications for assessing the prospective environmental, economic, and social impacts of these technologies. A thorough understanding of these systems facilitates a holistic evaluation, ensuring that CCS/CCU technologies contribute effectively to mitigating climate change while considering the broader socio-economic context.
4 Prospective techno-economic assessment
4.1 Hybrid costing method
To evaluate the competitiveness and economic viability of technologies, a common way is based on detailed engineering-economic analysis using established costing methods for a proposed plant or process installation. Typically, this “bottom-up” costing approach is utilized for mature technologies (Perry et al., 2008). However, there is a growing need to evaluate the feasibility of emerging technologies that are in the early stages of TRLs. The technologies from low to high TRL will experience cost escalation during scaling-up from lab-scale to the First-of-a-kind (FOAK) plant and then starting to fall because of technological learning, as shown in Figure 5A. The experience gained during the FOAK to Nth-of-a-kind (NOAK) often leads to a series of process improvements and cost savings. Learning curves have been widely used to characterize this “learning-by-doing” effect in the deployment of energy and low-carbon technologies (Ferioli et al., 2009; Bolinger et al., 2022). However, projecting cost reductions through learning curve for emerging technologies is challenging due to the limited historical data available for generate learning curves (Rubin et al., 2007).
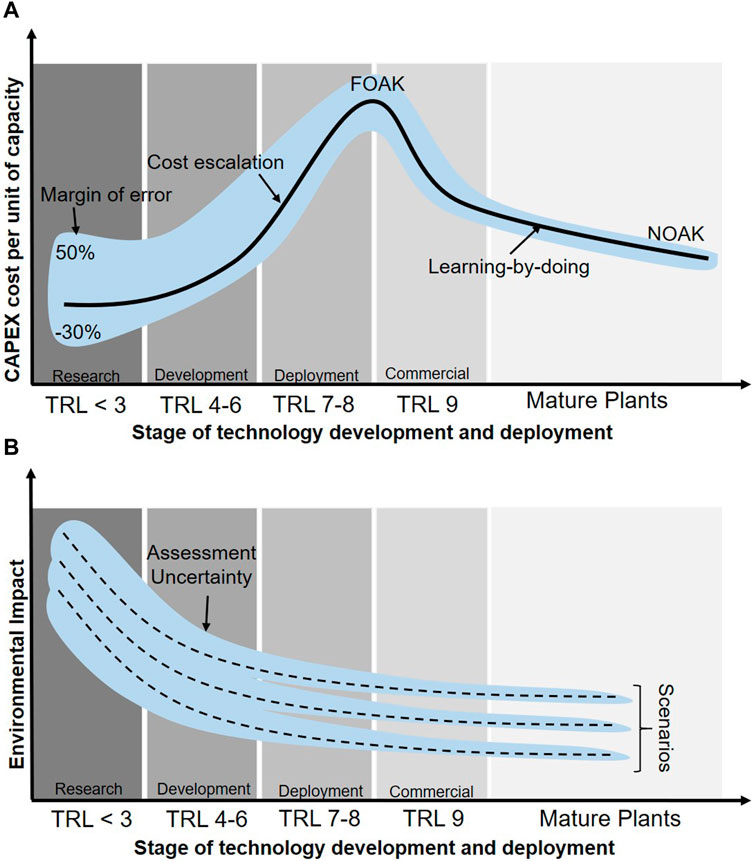
Figure 5. (A) Conceptual representation of cost evolution patterns in process technologies, adapted from Van Der Spek et al. (2017); (B) Conceptual diagram of prospective environmental assessment during scaling-up of process technologies at different scenarios, adapted from Thonemann et al. (2020).
To address the limitations using “bottom-up” costing and learning curves during the early stages of technology development, Rubin (2019) developed a framework for estimating the NOAK cost of advanced low-carbon technologies that are currently at early pre-commercial stages of development. This framework addresses the two types of questions that commonly motivate a cost analysis called “what if” and “what will” questions. They introduced a hybrid costing method that combines a bottom-up analysis of the FOAK commercial cost and technological learning-curve projections (a top-down method) to estimate how costs may develop in the future.
As shown in Figure 5A, a sound FOAK cost estimation is critical for applying the hybrid method. Firstly, the selection of the FOAK capacity is significant because it gives the initial points for applying learning curves. Then, the capital expenditures (CAPEX) are calculated using a series of “bottom-up” calculation to reach total plant costs.
Besides, the estimation for FOAK cost also include the operating expenditure (OPEX) that is expected to be higher than for a mature technology. Proper and transparent assumptions are significant for OPEX estimations. The fixed OPEX are highly linked with CAPEX, while the variable O&M and CAPEX are roughly independent. The learning curves are often applied to CAPEX. However, some research pointed out it is also important to apply separate learning rates for variable OPEX accounting for improvements in energy efficiency, heat integration, and process improvements.
Then, from FOAK to NOAK cost estimation, the guidelines from NETL (2013), Rubin (2019), Roussanaly et al. (2021) all recommended to decompose each plant design into major technology sub-sections, select an appropriate learning rate (LR) for each component and set the period of learning and ending period. The learning rate can be defined as shown in Eq. 1. The total cost is calculated using the formula provided in Eq. 2.
in which the index i represents a given cost component. Each component is in principle characterized by a different learning parameter bi, a different initial and cumulative capacity
The direct choice of LR for a sub-section is the value for a technology that is same or like one under analysis. For some cases, expert judgments or general heuristics could be required for some novel technological components. Recently, Malhotra and Schmidt, 2020 proposed a technology typology that distinguishes three types of novel technologies based on the combination of inherent characteristics of design complexity and customization need. Each technology type is coupled with different average learning rates and standard deviations (type 1: LR = 0.22,
4.2 Applications of pTEA of CCS/CCU
This section will introduce the application of pTEA across different fields of the CCS/CCU chain: CCS in power and industrial sectors, DACCS, and CCU.
4.2.1 CCS in power and industrial sectors
In the power generation and industrial sectors, CO₂ capture represents a significant portion of the total costs associated with the entire CCS chain. Early studies, such as those by Rubin et al. (2007, 2012) pioneered the use of learning curves to project the future costs of CO₂ capture in power plants. With the increasing commercial scale of CO₂ capture in power plants and natural gas processing, there are several established cost estimations guidelines for CO₂ capture technologies (IEAGHG, 2013; NETL, 2014; Rubin, 2019). In the recent years, with the increasing attention for hard-to-abate sectors, there is surge research about CO₂ capture. Leeson et al. (2017) conducted a comprehensive TEA of CCS technologies applied to the iron and steel, cement, oil refinery, and pulp and paper industries. Their modelling, based on gathered data, projected CO₂ avoidance costs up to 2050, highlighting that the cost for the cement industry could potentially reach $28 per tonne of CO₂ avoided through calcium looping. The application of amine absorption in steel plants and refineries was projected to cost $55 and $59 respectively by 2050. However, the projections are marred by significant uncertainty due to the limited and inconsistent data on alternative technologies. The CEMCAP project (Gardarsdottir et al., 2019; Voldsund et al., 2019, p. 1), through quantitative assessment of five different CO₂ capture technologies, underscored the importance of comparing various technologies to identify the most cost-effective options for cement plants specifically. It is critical to note that, as IEAGHG (2018a) pointed out, the cost-effectiveness of CCS solutions is highly location dependent, influenced by factors such as waste heat availability and transport distances (Garcia and Berghout, 2019).
4.2.2 DACCS
The emergence of DACCS technologies has been significantly supported by policies such as the US Inflation Reduction Act (IEA, 2023b) and CRETE Act (CREATE, 2023), the EU Innovation Fund in Europe (European Commission, 2022), and various demonstration projects, e.g., by Climeworks (2024) and Carbon Engineering (2024). Table 1 summarizes these recent developments, highlighting the variance in cost projections rooted in differing assumptions, regarding learning rates for CAPEX and variable OPEX as well as initial capacity and cost. Thus, costs have been projected to range from below $100 to over $1000 per ton of net CO₂ captured and stored as shown in Table 1. Young et al. (2023) applied a hybrid costing method to explore the potential for cost reduction in DACCS across seven countries, considering the impact of different low-carbon electricity sources. Their findings suggest that DAC could achieve substantial cost reductions at the gigaton scale, especially when leveraging nuclear electricity and heat pumps for low-grade heat. More recently, Sievert et al. (2024) proposed a novel framework for estimating LRs of DAC technologies using multi-component learning curves combing probabilistic cost estimations. This approach, which assesses both the design complexity and customization needs of DAC components and systems, offers a more nuanced and potentially less risky method of projecting cost reductions compared to single-component learning curves. Recent projected results from Pett-Ridge et al. (2023) and Sievert et al. (2024) also doubt on the $100/t CO₂ target established by the US Department of Energy, which also reflects the significance of further accelerating the development and large-scale development of diversified DAC technologies.
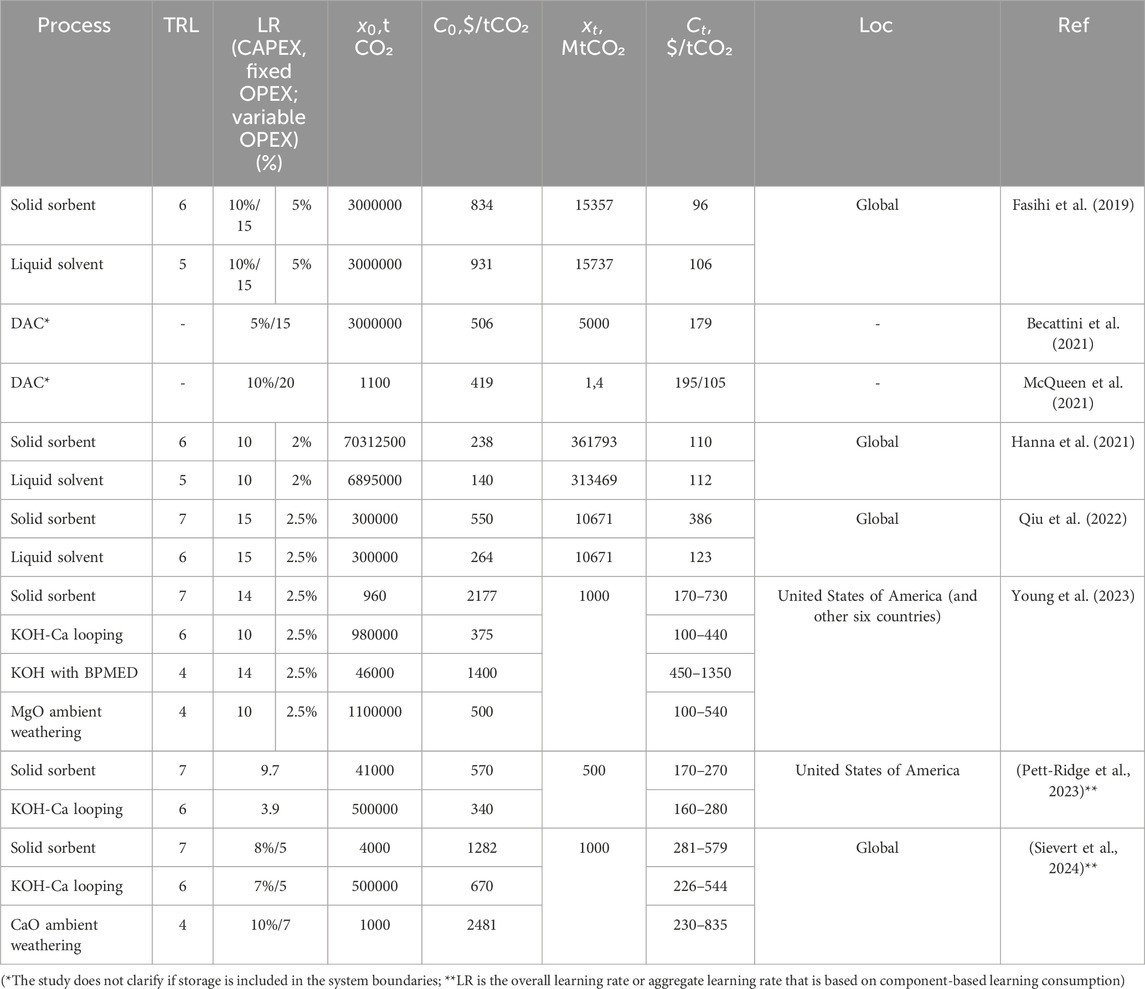
Table 1. Projected costs of pTEA for DACCS (Adapted from Sievert et al., 2024).
4.2.3 CCU
Compared to the research on CCS, the exploration of CO₂ utilization within the framework of pTEA is notably limited, particularly for emerging CCU technologies. Faber et al. (2022) adopted the hybrid costing method to disassemble a direct aqueous mineralization system into its core components: CO₂ capture and compression, mineral pre-treatment, the reactor, and post-treatment processes. This approach enables the identification of the scale of deployment needed to achieve targeted capital costs and greenhouse gas emissions per unit of the mineral product. However, it is crucial to note that their projections only account for CAPEX and not consider the variability in OPEX due to changes in raw materials and energy prices.
Detz et al. (2018) utilized advanced learning curves for individual system components to assess the potential competitiveness of CO₂-based fuels against fossil fuels. Their analysis suggests that, even under conservative scenarios, H2 production and CO₂-based Fischer-Tropsch diesel could reach cost parity, driven by the swift cost reductions and efficiency gains of solid oxide electrolysis technology. Beyond the technological advancements, the widespread deployment of renewable energy sources plays a critical role in influencing both the affordability and demand for CO₂ utilization technologies.
Contrasting with the deployment scale of CCS, the commercial viability of CO₂-based products encompasses more intricate challenges (Desport and Selosse, 2022). These include competition with existing fossil fuel-based products, market demand, the carbon footprint of the products, and CO₂ pricing—all of which are intricately linked to policy support and socio-economic trends.
4.3 Challenges and development
4.3.1 Uncertainties of hybrid costing method
All cost estimates inherently come with uncertainties; for instance, the accuracy of the FOAK capital cost can vary between −30% and +50% of the calculation value as shown in the Figure 5A, which the Association of the Advancement of Cost Engineering (AACE) expects for a class 4 estimate (AACE, 2020). In general, FOAK cost estimates are most appropriate for technologies that have advanced to at least large pilot plant or full-scale testing of the new technology (e.g., TRL 6-8). Technologies at earlier stages of development are inherently much riskier (Rubin et al., 2021). The maturity of technologies across different TRLs necessitates a varied approach to process contingency, as recommended by existing guidelines (AACE, 2020). The selection of an appropriate process contingency value for technologies at lower TRLs, which exhibit a broader range and greater uncertainties, demands careful technical and empirical judgment based on the specific technology’s current state. Moreover, system contingency, which accounts for additional capital costs associated with system integration and complexity following the first commercial installation, also relies on professional judgment for accurate estimation.
Projecting the FOAK costs to NOAK further amplifies these uncertainties. The adoption of the hybrid costing method, which integrates uncertainties linked to learning curve analyses, adds another layer of complexity. The challenge of calculating LRs is exacerbated by the scarcity of high-quality data. Thomassen et al. (2020) emphasized the importance of understanding the drivers of learning at different developmental stages and advocated for a hybrid approach that combines expert projections with extrapolated performance metrics derived from learning curves. Similarly, recent research by Sievert et al. (2024) on the cost estimation of DAC systems highlighted the critical role of expert elicitation, particularly in the absence of empirical data for the analysis of novel technological components.
Despite the pervasive uncertainties in cost estimation for emerging technologies, acknowledging, and meticulously analysing these uncertainties through methods such as sensitivity analysis and Monte Carlo simulations enables decision-makers to make more informed decisions. For more comprehensive uncertainty discussion of methods for applications of low-carbon technologies, one may refer to relevant publications (Van Der Spek et al., 2020; Way et al., 2022) in the field.
4.3.2 Assessment for technologies at low TRLs
Evaluating early-stage technologies in hybrid methods presents significant challenges, particularly for those still under development (TRL<6). Early technology assessments carry higher uncertainties due to insufficient operational and performance data (Zimmermann et al., 2022). This often results in overlooking innovative technologies and underestimating the importance of process intensification and optimization in scale-up phases (Van Der Spek et al., 2017), a critical challenge in rapidly evolving fields seeking promising yet immature technologies.
In CO₂ capture technology, the broad range of solvents and sorbents available in labs poses a challenge in accurately predicting their CO₂ capture capacities. Traditional metrics like selectivity and working capacity do not always correlate with ultimate process efficiency, underscoring the need for integrating process modeling and optimization early in material selection. For instance, the study by Burns et al. (2020) on over 5000 MOFs highlights the necessity of integrating detailed process modelling and optimization into sorbent screening processes. Innovations like the PrISMa (Charalambous et al., 2023) platform demonstrate the benefits of combining materials science, process design, TEA and LCA to enable the efficient screening of materials and effectively rank their performance against critical performance indicators, such as Henry selectivity, Purity, Productivity, Net Carbon Avoidance Cost, Climate Change, and Materials Resources: Metals/Minerals. This holistic approach underlines the significance of integrating various dimensions to identify the most suitable structures for cost-effective and environmentally friendly carbon capture processes.
Advancements in adsorption technologies offer low-cost, energy-efficient alternatives to traditional absorption systems. Yet, scaling up solid adsorbents for commercial use remains challenging. Collaborations, such as between BASF and Svante, have successfully scaled up of the CALF-20 (Zinc-based Calgary Framework) MOF sorbents, highlighting potential for industrial applications (Lin et al., 2021).
For the evaluation of emerging CO₂ utilization technologies, a systematic evaluation methodology sensitive to the technology’s developmental stage is crucial. Roh et al. (2020) proposed a systematic methodology designed for this purpose, unfolding in three strategic steps: the gathering of primary data, the generation of secondary data, and the calculation of performance indicators. This structured approach highlights how the accuracy of performance indicators at TRLs 2 and 3 hinges significantly on the type of energy powering the CCU system and the realistic recovery rates of CO₂-based products. Through four illustrative case studies including electrochemical CO₂ reduction, ethylene production, synthetic fuels and microalgal co-firing power plant, which demonstrated the applicability of their methodology in assessing specific CO₂ utilization technologies.
In the domain of CO₂ conversion to chemicals, the development and optimization of catalysts are crucial for the transformation of CO₂ (Artz et al., 2018). The effectiveness of new catalysts is typically measured by their conversion efficiency, selectivity, or space-time yield within laboratory reactors. However, the ultimate validation of a catalyst’s performance lies in its application at the plant level. Spiekermann et al., (2023) contributed to this area by assessing catalysts for CO₂ hydrogenation through a process optimization approach. By employing consistent assumptions to mediate the trade-offs between reaction kinetics and process design, and by fine-tuning parameters, their work bridges the link of lab-scale data on novel catalysts to their process-level performance.
4.3.3 Influence of background system
The uncertainties linked with technological progress and renewable energy proliferation make it challenging to project background systems, particularly due to the energy-intensive nature of CO₂ capture from industrial and atmospheric sources. Therefore, it is critical to incorporate dynamic background scenarios into the quantitative analysis of CCS/CCU technologies. Roussanaly et al. (2021) introduced comprehensive guidelines for evaluating the costs of CCS technologies, underscoring the need for transparent scenarios that realistically portray future heat and power supply strategies. These evaluations must account for the variability in costs and emissions intensity of heat and power, which are highly location and situation-dependent, thereby affecting CO₂ avoidance costs and the comparative assessment of CO₂ capture technologies.
In the long term, post-2050, the grid is expected to accommodate all power demand with a reliable supply of net-zero-emissions electricity for all users. However, in the short term, CO₂ utilization processes may require the deployment of clean power generation and energy storage to enable the 24/7 operation likely needed for economic viability. Many CO₂ utilization processes require significant amounts of clean electricity, water, and green hydrogen. Given the complexities of transporting and storing hydrogen, it is crucial to integrate the design of hydrogen production with CO₂ utilization to ensure efficiency and feasibility (National Academies of Sciences, Engineering, and Medicine, 2023).
Biermann et al. (2022) proposed a novel approach for integrating local heat sources in post-combustion CO₂ capture processes, enhancing abatement cost effectiveness. Their methodology, based on multi-period linear optimization, aims to identify an optimal mix of heat sources that minimizes costs or reduces external energy imports, considering the existing site’s energy system capacity. This provides more realistic values compared to annually averaging, which can vary from 7% to 26% and 9%–66% for higher costs and emissions respectively.
Regarding CO₂-based products, Christensen and Petrenko (2017) analysed four policy scenarios to assess synthetic fuel production potentials under varying electricity source restrictions. They concluded that substantial production volumes are achievable only with subsidies ranging from 1.00 to 1.50 euros per litre, indicating that significant policy support and energy cost are essential for the widespread adoption of power-to-liquids technologies (Zhou et al., 2022).
4.3.4 Evaluation of overall supply chain
The holistic integration of CCS/CCU systems reveals significant opportunities to refine transport and storage cost estimations. In the United States, the existing infrastructure boasts 9,000 miles of pipelines dedicated primarily to CO₂ transportation, with 90% utilized for EOR processes. This contrasts starkly with Europe, where with lack of specialized CO₂ pipeline infrastructure in operation, however, around 40 dedicated transport projects have been planned recently in the North Sea region (IEA, 2020). To effectively scale up CCS/CCU technologies, developing a versatile infrastructure capable of accommodating diverse CO₂ transport methods is essential to manage large CO₂ volumes efficiently.
Roussanaly et al. (2021) criticized the oversimplification of transport and storage costs into a fixed per-tonne charge. They proposed a more detailed approach considering factors such as CO₂ flow rate, mode of transport, distance, and storage type, to derive more accurate cost estimates. The National Energy Technology Laboratory (NETL, 2022) introduced a CO₂ transport cost model to estimate the financial aspects of pipeline transportation, enabling adjustments for various project-specific variables such as CO₂ mass flow rate and pipeline length. The UK’s Department for Business, Energy, and Industrial Strategy (BEIS, 2018) provided cost estimates for shipping CO₂ to geological storage sites, factoring in the complete infrastructure requirements of CO₂ shipping chains, including liquefaction and temporary storage processes. Recently, Oeuvray et al. (2024) published a comprehensive multi-criteria assessment considering realistic CO₂ transport options including tank containers that can be transported by trucks, trains, barges, or ships, dedicated tanks permanently integrated with different transport modes or pipelines. Their analysis revealed that short-term transport costs could soar above 200 EUR per ton of CO₂, exceeding the costs associated with pipeline transport noted in prior studies, which also reflects the urgency to build corresponding transport infrastructures.
Eligible CO₂ storage zones must meet several criteria to ensure safety and efficiency. These include adequate capacity and injection rates, the containment ability of sealing rocks to prevent CO₂ migration, geochemical and geomechanical stability, appropriate stratigraphy and reservoir depth, favourable geothermal and hydrodynamic conditions, and low seismic activity (Nunes and Costa, 2021). Continuous monitoring of the post-operational phase of CO₂ injection is also crucial. Based on the EU experience, most regulatory challenges are attributed to the geologic CO₂ storage component of the CCS chain, particularly in managing CO₂ geological storage risks and predicting and quantifying the costs of potential CO₂ leakage in long-term liability scenarios (Rocha and Costa, 2021).
Enabling and expanding CO₂ utilization requires consideration of policies, regulations, equity, and environmental justice. The most effective and efficient policies for enabling CO₂ utilization would ensure that externalities from GHGs from all sources are considered in the full cost of using the technology. Additionally, these policies should incentivize knowledge creation and reduce costs and risks for early adopters rather than subsidize specific technologies (National Academies of Sciences, Engineering, and Medicine, 2023).
5 Prospective life cycle assessment
5.1 LCA frameworks: Introduction and definitions
Heijungs and Frischknecht (1998) define Life Cycle Assessment as a “systematic method for evaluating the environmental impacts of a product or system through all stages of its life cycle, from raw material extraction through production, use, and disposal.” This comprehensive approach ensures that environmental impacts are not simply shifted from one stage or category to another. Traditionally, LCA studies have focused on analyzing well-established systems after they have been defined, requiring extensive data on both background and foreground processes, and typically aligning the assessment time in the present (Cucurachi et al., 2018). Notwithstanding the importance of the traditional LCA approach, a prospective element seems to be missing. Anticipating an improvement in system performance may be difficult, at early stages of system design when applying changes is easy; however implementing potential improvements becomes difficult and costly if the need for such change is apparent at later stages of system design (Collingridge, 1982). This dilemma highlights the importance of adopting a strategic approach to capture the environmental implications of technologies during their early development phases by shifting the focus of the LCA study to a more distant future. Prospective LCA addresses this dilemma by intervening in the early growth phases when the technology’s environmental blueprint can still be meaningfully shaped and modelling background system scenarios, for instance, future energy systems (Arvidsson et al., 2018).
In Table 2, side-by-side comparison of various LCA methodologies is presented.
- Traditional LCA models the effects determined by changes in the technology landscape as outlined in standards like ISO 14040, 2006; ISO 14044, 2006; European Commission - Joint Research Centre, 2010;
- pLCA goes a step further by evaluating emerging technologies and incorporating scenario modelling. This prospective approach involves working closely with technology developers and considering future market and technological changes (Hetherington et al., 2014; Villares et al., 2017; Cucurachi et al., 2018; Mendoza Beltran et al., 2020; Van Der Giesen et al., 2020; Sacchi et al., 2022; Kleinekorte et al., 2023; Langkau et al., 2023);
- Dynamic LCA is separately introduced as a method that stresses the importance of improving the accuracy of LCA by addressing the temporal component of technological developments, especially emissions and related impacts over relatively shorter time periods than pLCA (Ferrari et al., 2021; Briones-Hidrovo et al., 2022).
5.2 Foreground and background influence in pLCA
While emerging technologies often seek to enhance existing functions performed by established technologies, the comparison may not always be straightforward, particularly when emerging technologies possess unique properties. The scarcity of primary data is a notable issue, often representing only lab-scale or pilot-scale conditions, impacting the reliability and relevance of results (Cucurachi et al., 2018). This scarcity extends to both foreground and background processes.
To conduct a pLCA, it is necessary to model the system at a future time, when the production scale of the emerging technology under study has increased compared to its current level. Additionally, established technologies should also be included in these evaluations as they may still improve over time, including possible changing market shares (Buyle et al., 2019) and background systems.
The evolution from qualitative to fully quantitative models in LCA is parallel to the increase in technological maturity. Figure 3, representing TEA of various technologies across TRLs, can be also extended for LCA. At the nascent stages (TRL 1-2), where basic principles and concepts are the focus, LCA plays a crucial role in major screening processes such as the selection of raw materials and the energy mix, with assessments grounded in thermodynamic principles. This early-stage LCA aims to identify environmental hotspots that could be critical in later stages of development. As the technology progresses to proof of concept (TRL 3), LCA facilitates the environmental impacts assessment and selection of technology components, providing more detailed insights that guide technology refinement. At the lab scale (TRL 4), LCA is used to compare process alternatives based on mass and energy balance, which steers the direction of technological advancement. Moving to pilot scale (TRL 5-6), LCA becomes an essential tool for the selection of promising alternatives and comparison with existing technologies (Hellweg and Milà I Canals, 2014). This comparative analysis is crucial to highlight potential improvements and optimize the technology before scaling up. At the demonstration to full-scale phase (TRL 7-9), LCA evolves to provide a full-scale environmental assessment, where it measures the technology’s maturity as it increases and ensures that process parameters are optimized for minimal environmental impact. This stage of LCA is fully quantitative and include analyses of contributions, scenarios, uncertainties, and sensitivities to conduct comparative LCA (Roh et al., 2020).
PLCA requires the construction and analysis of scenarios that provide quantified data for the technologies under study which can directly be controlled, the foreground system. Since material and energy inputs are a function of time and production scale. These scenarios must contemplate how new technologies will evolve, including aspects like technological advances, scaling up processes, and market development. Different strategies have been proposed for modelling the future foreground production system and scale in pLCA such as the use of learning curves to calculate the environmental impacts of a technology development over time (Bergesen and Suh, 2016), scenario ranges including best and worst case environmental impacts (Arvidsson et al., 2014), engineering-based scaling laws; additionally, scientific articles, patents, expert interviews, unpublished lab results, process simulations, and realistic assumptions.
As for the background system, which cannot be directly affected, scenarios should contemplate the broader technological and economic context in which they operate, such as shifts in the energy sector and overall transitions towards sustainability. If current background systems are expected to remain constant over a more extended period, there might be a case for omitting the modelling of the background system entirely (Arvidsson and Molander, 2017). In some instances, focusing solely on assessing heat and electricity inputs, excluding other background aspects, might be sufficient (Pini et al., 2017). However, as the expected saturation phase moves further into the future, the importance of accounting for changes in background systems grows. This consideration is essential to avoid discrepancies in time between the studied foreground system and the background systems, ensuring the study’s findings remain relevant. Consequently, future scenarios of the background system are modelled to reflect these potential changes (Gibon et al., 2015). Taking it one step further, Mendoza-Beltran et al., 2020 introduced an approach to systematically modify the ecoinvent database (Frischknecht et al., 2005) parameters by integrating the scenario information of the technology-rich IMAGE yielding a database that is both time-sensitive and scenario-specific. Moreover, Sacchi et al., 2022 generalizes a background modelling technique applicable across various sectors with the premise approach to generate more consistent and transparent databases (See Section 5.4).
5.3 Application of prospective LCA for CCS/CCU
The varying and evolving TRLs of CCS and CCU technologies present significant challenges in the execution of pLCA for CCS and CCU systems due to the intricate scenario analysis and the diverse technological and methodological perspectives involved. Table 3 presents the methods and findings of traditional and prospective LCAs from various articles discussed in this subsection. It displays the connections between the functional unit, the foreground and background systems, and the environmental trade-offs associated with prospective and traditional LCA across CCU/CCS technologies and supply chain. Due to the uncertainties in the LCI data, no quantitative conclusion can be outlined regarding the clear convenience of a certain technology. The climate benefits of one technology over another depend strongly on the energy source, the location considered, the assumptions made, and the boundaries of the system, among other considerations. Trade-offs are due to the energy penalty of infrastructure development from the capture process, air separation units, and other processes in the value chain. CCU examples of Table 3 are not pLCA studies, since there is a lack of studies on this regard in literature compared to the prospective status of CCS.
Initial LCA research primarily focused on post-combustion capture technologies, especially those using Monoethanolamine (MEA) at power plants (Spath and Mann, 2004; Koornneef et al., 2008), with lesser attention given to pre-combustion and oxyfuel combustion technologies (Singh et al., 2011; Gładysz and Ziębik, 2015). In contrast, a limited number of studies have assessed the environmental impact of Calcium looping technology (Kursun et al., 2014; Petrescu et al., 2017; Tang and You, 2018). With growing focus on hard-to-abate sectors and the pursuit of negative emissions, recent studies have broadened to include a variety of CO₂ capture technologies for cement plants (García-Gusano et al., 2015) and DAC (Deutz and Bardow, 2021; Terlouw et al., 2021). These studies underscore the significant influence of energy sources on LCA results for CCS systems, highlighting the trade-offs between reducing GHG emissions and increasing other life cycle burdens (Volkart et al., 2013; Negri et al., 2021). Research has also revealed that the environmental performance of certain technologies, like tail-end calcium looping, significantly depends on fuel selection, indicating that alternatives to coal, such as natural gas or biomass, can reduce GWP (Schakel et al., 2018). There are a few LCA studies conducting the overall supply chains including the transport and storage networks for CCS systems (Singh et al., 2011), where a lack of detailed information regarding critical parameters such as transportation distances and the CO₂ purity levels required for injection. Recently, research from Burger et al. (2024) showed that the high global warming impact contrasts the previous studies because they considered ready-to-use transport technologies such as truck, train, barge, and ship rather than pipelines that are still not available in Europe.
Compared to CCS, developing LCA frameworks for CCU systems encounters additional hurdles at both technological and methodological levels. Most these technologies are in early development stages and are designed to minimize environmental impacts (Garcia-Garcia et al., 2021). As such, numerous LCA studies on CCU focus on quantifying the potential reductions in environmental impact that CCU processes or products may offer when compared to conventional processes. Despite the provision of methodological guidance for LCAs of CCU technologies (von der Assen et al., 2014; von der Assen et al., 2013), debates persist over key issues such as the system boundaries and duration of CO₂ storage in products (Tanzer and Ramírez, 2019). Moreover, the need for harmonized LCA methodologies for CCU technologies has been emphasized (Sick et al., 2020), revealing that methodological variations across all stages of LCA for CCU significantly impact the comparability of results (Thonemann and Pizzol, 2019; Thonemann, 2020). These challenges are compounded by the reliance on literature and simulation data for LCI analysis and the often-unspecified TRLs of the CCU systems under investigation.
CCU technologies, especially in their emerging stages, are not competing directly with current technologies but rather with those that will become prevalent in the future. Selecting a suitable benchmark process is essential for accurately assessing the reduction capabilities of CCU technologies. To this end, projecting future technological advancements is essential, and techniques such as learning curves can be employed for both CCU and reference processes to reflect their evolutionary nature. Most of the research results and framework proposed are dependent on specific type of processes. Thonemann and Pizzol, 2019 developed four-step approach for deploying pLCA to produce electrochemical formic acid production. They pointed out that validation of alternative production routes is essential to ensure comparability and liability. Voelker et al. (2022) performed the well-to-wheel LCA for blending electricity-based polyoxymethylene ethers (OMEs) with fossil diesel, including four alternative production routes and different scenarios for hydrogen, heat, electricity, and sources of captured CO₂. From their results, they addressed the importance to further expand the foreground systems and uncertainties about market share because of development of other competitive technologies. Bachmann et al., 2023 conducted a consistent environmental comparison between alternatives to produce syngas switching to biomass, mill-gas, or CO₂ from fossil resources. The results showed that CCU-based syngas is viable alternative if CO₂ is the only available carbon source and low-carbon electricity is abundantly available. Besides, research conducted by Fernández-Dacosta et al. (2018), Hoppe et al. (2018), and Sternberg and Bardow (2016) identified the demand for hydrogen as a hotspot. However, some mitigation technologies like water electrolysis and DAC can lead to trade-offs in other impacts, such as eutrophication and ozone depletion (Thonemann and Pizzol, 2019).
5.4 Prospective LCA to CCS/CCU: challenges and insights
Synthesizing the recommendations in the works of Arvidsson et al. (2018), Langkau et al. (2023), Mendoza Beltran et al. (2020), Müller et al. (2020), Sacchi et al. (2022), Thonemann et al. (2020) and Van Der Giesen et al. (2020) a systematic approach for conducting structured, transparent, and consistent pLCAs of CCS/CCU technology systems is presented in Figure 6. The study’s Goal and Scope Definition focuses on the future commercial state of the technology, considering performance, market situation, and expected maturity and market penetration at a specific future time.
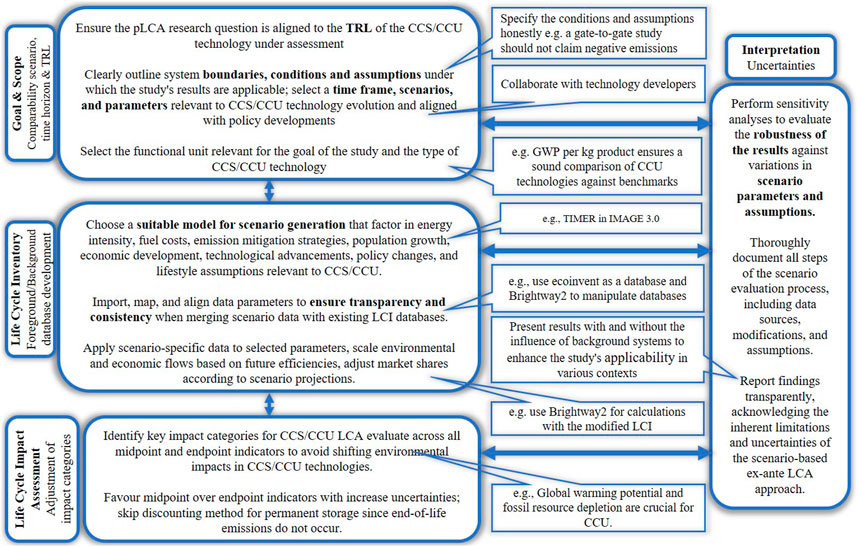
Figure 6. Framework for conducting structured, transparent, and consistent prospective Life Cycle Assessments of CCS/CCU technology systems.
Current databases like ecoinvent (Frischknecht et al., 2005), designed to reflect present conditions, may need to be complemented with technological advancements or anticipate shifts in technology and policy. To compile the Life Cycle Inventory, new technology data are sourced from scientific research, patents, expert interviews, lab results, or process simulations (Arvidsson and Molander, 2017). While this data initially proves the concept of an innovative technology, it must be extrapolated using assumptions (e.g., based on learning curves) to depict future commercial operations. IAMs play a key role in shaping CCS/CCU deployment scenarios influenced by policy changes (Langkau et al., 2023). The process of how to add necessary data not found in existing databases, integration of these scenarios into the LCI database and how to perform the Life Cycle Impact Assessment with LCA software is further elaborated in Section 6.3.
The final stage of a pLCA, Interpretation, requires conducting uncertainty and sensitivity analyses to assess the robustness of its findings, which involves examining how variations in data and underlying assumptions could influence the outcomes. While stochastic uncertainties, those related to data variability, can be addressed with techniques like Monte-Carlo simulations, epistemic uncertainties remain as a limitation due to the inherent unpredictability of future developments (Van Der Giesen et al., 2020). Scenario analysis is thus employed to bring transparency to the assumptions made and their resultant uncertainties. But this approach also has its challenges since creating future scenarios requires consistency and clarity, ensuring that assumptions align across the projected background (global economic and technological context) and foreground (specific technology under study) (Gibon et al., 2015). The variability in assumptions regarding technological efficiency and the choice of background systems or allocation methods further complicates LCA outcomes, even for identical products. For example, cradle-to-gate carbon footprints for CO₂-based methanol in LCA studies vary between −1.7 and +9.7 kg of CO₂-eq per kg of methanol (Müller et al., 2020). Such variability can be explained because of the differences in assumptions taken by the practitioner and highlighted in initial stages of development as depicted in Figure 5B. The results, considering uncertainty and sensitivity analyses, are interpreted to provide comprehensive documentation and transparent reporting of assumptions and limitations, facilitating informed decision-making.
6 Prospective assessment with Integrated Assessment Models
6.1 Overview
IAMs are computer simulations that represent complex interactions and feedback on a long-time scale between the socioeconomic system (including climate policies) and the natural system, which are explicitly designed to inform climate policymaking (Parson and Fisher-Vanden, 1997; Van Vuuren et al., 2011). The models vary in their structure, detail, and type of policy questions they are designed to address (Weyant et al., 1996). IAMs are the backbone of scenario analysis of Working Group III (WGIII) of the IPCC. IAMs enable the examination of various technologies and their contributions to mitigating climate change. Since the Fifth Assessment Report, they became a crucial tool in the IPCC’s efforts to evaluate the pathways for limiting global warming to below 2°C within this century (Cointe et al., 2019; Calvin et al., 2023; IPCC, 2023).
Most IAMs now incorporate prospective TEA for various technologies. These models account for the ongoing evolution of energy systems and technological advancements in energy technologies. This is achieved either through exogenous cost reductions based on detailed bottom-up analyses, as seen in the GCAM (Calvin et al., 2019) and MESSAGE (Kishimoto et al., 2024) models, or by modelling learning-by-doing endogenously, as in the REMIND (Baumstark et al., 2021), IMAGE (Elke et al., 2014), and POLES models (Luderer et al., 2019). Regional variations in the deployment of renewable energy are influenced by factors such as the potential for renewable resources, the costs and availability of alternative technologies, and the alignment of renewable energy supply with demand. Specifically, the REMIND model details each technology with characteristic parameters, including specific overnight investment costs that remain fixed for most technologies but decrease through learning-by-doing. It models all technologies as capacity stocks, meticulously tracking each one’s vintage. Since the model imposes no strict limits on the pace of investment shifts, it allows for significant flexibility in technological development, enabling different capital stocks to be invested in and adapted over time. This approach offers a dynamic framework for exploring the pathways of technological evolution and its impact on energy systems (Baumstark et al., 2021).
IAMs of the energy-economy-climate system are commonly used to analyse alternative climate change mitigation strategies and their implications, with a primary emphasis on reducing GHG emission. Only recently have IAMs begun to consider other specific environmental impacts such as air pollution, land-use for bioenergy or water demand (Calvin et al., 2019; Gaugl et al., 2023) However, these models have yet to address the broader range of impacts fully. As a result, a comprehensive and integrated evaluation of the additional benefits of various mitigation approaches is still lacking.
In contrast, LCA examines a wide set of environmental impacts and monitors numerous substance flows. Yet, most conventional LCAs focus on current technologies and individual systems, failing to account for changes in environmental performance or the effects of large-scale and structural changes to systems (Arvesen et al., 2018). As discussed in Section 5 regarding the development of pLCA, there has been some progress in adapting LCA to include future technological changes. This development marks a step towards incorporating more dynamic and forward-looking assessments in environmental evaluations.
The fields of LCA and IAMs share a common interest in evaluating future and large-scale transformation pathways, focusing on environmental, economic, and social aspects. Efforts to integrate these two fields have been pursued in two primary directions to leverage their strengths. One approach involves incorporating LCA results into IAMs to enhance the environmental precision and breadth of IAM projections. For instance, Pehl et al. (2017) integrated LCA energy coefficients into the REMIND model to assess GHG associated with future global electricity systems and examine how including life cycle emissions influences optimal technology selection. Arvesen et al. (2018) developed a method to derive energy and impact indicators from detailed LCA for use in IAMs, applying this method to future global electricity supply scenarios. This approach attributes construction, operation, and end-of-life coefficients to specific years, aligning them with IAM data on technology performance and deployment. Luderer et al. (2019) combined five different IAMs and LCA methods to evaluate the environmental co-benefits and adverse effects of various power sector decarbonization pathways. The approach of integrating IAM scenario results into LCA analyses is more common in the LCA community. Cox et al. (2020, 2018) used scenario outcomes from the IMAGE model to compare the life cycle environmental impacts and costs of current and future passenger cars. Sacchi et al. (2022) developed an open-source tool named Premise, which streamlines the integration of IAM prospective scenarios into the LCI database ecoinvent (Frischknecht et al., 2005), enabling pLCA. Premise is not limited to power generation but also extends to other energy-intensive sectors, including cement and steel production, and transportation. Its open-source nature has led to widespread use in pLCA research across various low-carbon technologies, constantly updating to include more sectors. To offer comprehensive insights for national GHG reduction strategies, there is a growing trend towards developing localized integrated models coupling with LCA. Volkart et al. (2017) combined a cross-sectoral energy system model with LCA to explore three scenarios for Switzerland’s energy transition, highlighting the importance of cross-sectorial technologies, and high temporal and spatial resolution in evaluating environmental impacts comprehensively. Baumstark et al. (2021) proposed SecMOD, a sector-coupled energy system model with LCA designed to optimize Germany energy system transitions, covering electricity, heat, and private transportation, and addressing the importance of multi-indicators of environmental assessment over the full life cycle for a holistic planning of decarbonization strategies.
As we transition to net-zero energy and industrial processes, there’s a rising interest in combining IAMs with LCA and TEA, especially for the power sector and low-carbon technologies. In this chapter, we first review how CCS/CCU technologies are assessed within IAMs. Then, we build on the initial concepts introduced in Section 4, Section 5 to outline a framework for effectively incorporating IAMs in future assessments for CCS/CCU systems. We conclude by discussing the importance of these assessments for environmental and climate policies, while acknowledging the challenges in this rapidly evolving area.
6.2 Prospective assessment of CCS/CCU in IAMs
The integration of CCS/CCU within IAM scenarios has emphasized in studies highlighting its essential role in meeting the Paris Agreement objectives, but it is also necessary to trade-off between large-scaled deployment and socioeconomic and environmental impacts.
With the TIAM-ECN IAM, Longa et al. (2020) projected how large CCS’s contribution could be in Europe in the power sector and industry by 2050. They found that around 25% power generation and 35% energy use in industrial sectors could be coupled with CCS, in competition with emissions mitigation alternative technologies. They also emphasized stringent climate policy is a greater driver than cost reduction for wider CCS diffusion. At the country level, Yu et al. (2019) compared the mitigation role of CCS/CCU across different IAMs and varying socioeconomic pathways (SSPs) for China. To reduce the uncertainty in the near-term deployment of technologies, they developed GCAM-China model providing provincial-level analysis based on the open-sourced framework of GCAM. The results show the early deployment in China occurs within industrial and synthetic fuel production sectors following by increasing deployment in power sector.
The shifts toward long-term CO₂ removal by DACCS addresses both mitigation cost reduction and land-use competition, despite its substantial energy and material demands. By century’s end, DACCS could consume about a quarter of global energy demand (Marcucci et al., 2017). Qiu et al. (2022) analyzed DACCS through pLCA using IMAGE 3.2, predicting the evolving requirements of DACCS technologies. Their study indicates potential enhancements in electricity sector decarbonization but raises concerns about terrestrial ecotoxicity and metal depletion, which emphasizes trade-offs among different impact categories.
Integrating LCA indicators into Swiss TIMES energy model (STEM), Vandepaer et al. (2020) quantified the long-term life cycle environmental impacts of the technologies used in the future energy system. They also explored the environmental and economic trade-offs of DAC and CCS processes, highlighting how striving for zero direct GHG emissions via DAC and BECCS elevates costs. They emphasize the crucial role of indirect GHG emissions and other environmental impacts such as metal depletion and human toxicity from infrastructure and supply chains in achieving climate goals. Shu et al. (2023) applied the open-source SecMOD framework and explored CCS in Germany’s transition to net-zero emissions. From their results, CCS is selected as the cost optimal transition pathways, where it can reduce the need for renewable energy expansion, grid storage and even electric vehicles. However, large-scale CCS implementation may impact resource use, land, and ozone layer.
Furthermore, research is expanding into the mitigation potential of CCS in challenging sectors like cement (Kermeli et al., 2019) and steel production (Harpprecht et al., 2022). These studies suggest improvements for IAMs. The uncertainties surrounding electrification, alternative materials, and competition from low-carbon technologies such as hydrogen are profound, necessitating multi-objective optimization and technology superstructure considerations for sector specific mitigation measures in IAMs. These results also highlight the importance of capturing cross-sectoral relationships between industries.
For the prospective assessment of CCU technologies, there is limited research deployed with IAMs. Blanco et al. (2018b) used JRC-EU-TIMES model to explore the potential of Power-to-Methane (PtM) in providing flexibility to the power grid and aiding decarbonization for other sectors. They also further assessed the potential of hydrogen and Power-to-Liquids (PtL) in decarbonizing of transportation and contributing to energy security and independence (Blanco et al., 2018a). From their study, the developing level of PtL will be defined by policy adoption on CO₂ storage and biomass availability. Compared with the coupling of CCS with IAMs, the modeling of CCU into IAMs faces more barriers. Recently, Desport and Selosse (2022) gave a review about the CCU in energy models, where they pointed out the CO₂ utilization is still poorly represented and rarely fully integrated into IAMs. They highlighted four major obstacles for modeling CCU into IAMs, a lack of integration for capture processes, a lack of modeling of various utilization routes for industrial sectors, a lack modeling at the global level and top-down models. Besides, they also addressed if CCU were modeled in the context of a carbon circular economy (Olah et al., 2009), the potential for CCU is likely to be much larger than it would be in the context of emissions mitigation.
6.3 Framework and guidance
Figure 7 shows a multistep process that include a series of databases and open-source tools that outlines a framework for pTEA and pLCA interfacing with IAMs for CCS/CCU.
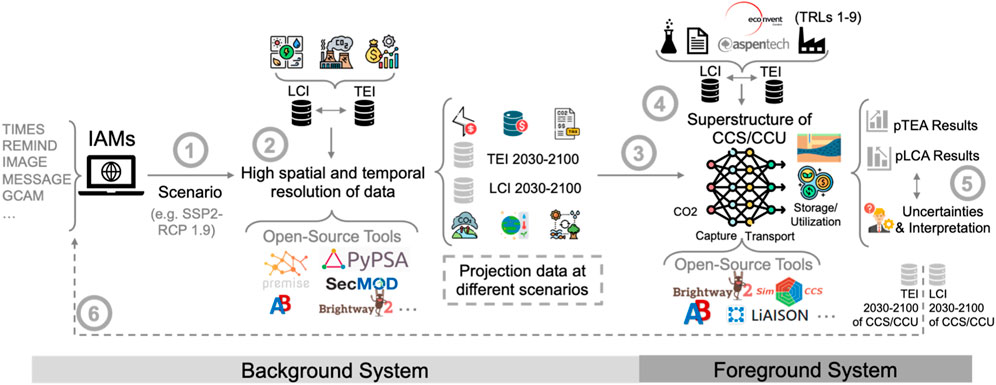
Figure 7. Schematic ramework of IAMs with prospective LCA and TEA for CCS/CCU. (Open-source tools: Premise (Sacchi et al., 2023b), PyPSA (Brown et al., 2024), SecMOD (Reinert et al., 2022), Brightway2 (Chris, 2024), AB-Activity-Browser (Steubing et al., 2020), SimCCS (Middleton et al., 2020), LiAISON (Lamers et al., 2023)).
The initial stages (step 1 and 2) involve refining Life Cycle Inventory (LCI) and Techno-Economic Inventory (TEI) databases for the background systems through the integration of scenario data from IAMs. These databases are adjusted to reflect scenario-driven transformations in technology, emissions, cost projections, and supply networks, forming a comprehensive background for prospective analysis. Based on the framework of open-source tools, such as Premise (Sacchi et al., 2023b) and PyPSA (Brown et al., 2024), IAMs scenarios are processed to update high spatial and temporal resolution of prospective data for background system. The next stage (step 3 and 4) is characterized by a thorough aggregation and construction of superstructure of CCS/CCU databases for the foreground system. Collecting of TEI and LCI data of different technologies along the overall supply chains at varying TRLs, meanwhile, keeping consistency of their prospective projections is the major work and challenge. This is followed by the application of specialized LCA software or open-source platforms, such as Brightway2 (Chris, 2024), to compute the modified LCI, which is informed by scenario-specific databases. Python could serve as the connective thread, intertwining disparate data sources, computations, uncertainty analyses, and the visualization of pTEA and pLCA outcomes (step 5). The backgrounds crafted by IAMs, and the enhanced database cohesively integrate future dynamics of socio-economic systems. The foreground addresses multiple scenarios concerning the superstructure and technological evolution within the CCS/CCU system. Results from pTEA and pLCA are then mapped onto the evolving landscapes of IAMs (step 6). This cyclic enhancement of the database and the iterative methodologies employed are instrumental in providing IPCC with nuanced insights, thereby refining their guidance on mitigation and adaptation policies.
The integration with IAMs will be useful for using consistent and comprehensive data source and scenarios for the modelling of background systems. Nonetheless, these models often suffer from outdated datasets and the absence of emerging mitigation technologies. The demand is rising for models that offer high temporal and geographical resolution, and which are also highly adaptable to updated, local data – demands that open-source tools as displayed in Figure 7 are beginning to meet. Some countries such as Sweden (Krook-Riekkola et al., 2017), Denmark (Andersen et al., 2019), Switzerland (Gjorgiev et al., 2022) and Austria (Kettner et al., 2024) have also initiated the construction of localized, high-resolution national integrated models.
Employing a superstructure-based modelling approach for the foreground system is advantageous for encapsulating all alternatives. A CCS/CCU superstructure allows one to represent feasible pathways of capture and storage or utilization from multiple CO₂ sources to products and identify optimal ones for given objectives, constraints, and parameters. For instance, Chung et al. (2022) developed a computer-aided tool named ArKaTAC3, which includes a flexible, expandable TEI and LCI database for CCS/CCU and a superstructure framework that identifies optimal CCU pathways under various scenarios. Yet, assessing the foreground system is often hindered by the diverse maturity levels (TRLs) of technologies and the difficulty in quantitatively gauging uncertainties related to the scalability potential and efficiency.
6.4 Challenges and opportunities
Projection for the future is always a challenging topic, especially for integrated models processing complex and interconnected systems. The integration of pLCA and pTEA into IAMs for CCS/CCU such rapid transformation fields reveal a complex landscape of challenges and opportunities. These challenges can be categorized into four primary areas: 1) quantitative uncertainties assessment, 2) matching between different models, 3) transparency of models and projections, 4) the comprehensiveness and flexibility of models. Challenges also mean opportunities for advancement, which are also active areas of research.
6.4.1 Quantitative uncertainties assessment
The combination of a heterogeneous set of data sets and their integration to existing databases introduce several inherent uncertainties. The quantitative assessment of these uncertainties remains a pivotal concern within IAMs (Weyant, 2017), TEA (Van Der Spek et al., 2020), and LCA (Hellweg and Milà I Canals, 2014) communities. Despite the availability of numerous methods for quantifying uncertainties, their practical application remains limited. Critical questions arise regarding the acceptable levels of uncertainty and which uncertainties are most significant for decision-making processes.
Uncertainties in CCS/CCU systems are broadly classified as either endogenous, originating within the system, or exogenous, stemming from external factors (Chung et al., 2022). This distinction is crucial, especially when considering the separate impacts on background and foreground systems. The background system, encompassing a wide array of technological systems, is rife with endogenous uncertainties such as energy efficiency, demands, and technology lifespan—factors intrinsic to the energy system. It also faces uncertainties from the development of other decarbonization technologies like electrification, alternative production routes, and hydrogen technology. Furthermore, exogenous uncertainties, including energy policy, fuel costs, technology costs, and climate change, represent external challenges beyond technological control (Li and Maréchal, 2023).
Foreground systems of CCS/CCU confront endogenous uncertainties inherent to their operations (Chung et al., 2022), such as CO₂ recovery rates, kinetic rates of conversion reactions, mass transfer coefficients, and leakage rates. These uncertainties, typically epistemic, suggest areas for potential research and experimental validation to reduce margins of uncertainty. In contrast, exogenous uncertainties, arising from economic, regulatory, environmental, and societal factors, such as cost of utilities, carbon pricing, societal acceptance, atmospheric changes, pose aleatory challenges due to their unpredictability and impact on the feasibility and adoption of CCS/CCU technologies.
The interplay between internal systems of CCS/CCU and external factors generates a dynamic feedback loop where technological advancements draw increased investor and stakeholder attention, spurring policy reforms and further technical research and optimization (Mo et al., 2018). This complex interaction highlights the necessity for an integrated approach to uncertainty management in CCS/CCU systems, combining technological innovation with policy and economic strategies to enhance technology adoption and scalability.
6.4.2 Matching between different models
Drawing from the insights of Sacchi et al. (2022) a significant challenge in IAMs and LCA emerges from the potential misalignment between the technologies modelled in IAMs and the corresponding LCIs (e.g., the lack of a distinction based on grades for steel products in the IAMs, while such distinction is especially important for calculating material and energy inventory in the LCI database). This misalignment often results in discrepancies in data granularity, leading to semantic ambiguities where a single IAM process may correspond to multiple activities within the LCI database without clear delineation of their respective contributions.
For instance, notable models such as REMIND (Baumstark et al., 2021) and IMAGE (Elke et al., 2014) do not account for the utilization of CCS technologies across sectors for achieving climate change mitigation targets. Despite this, CCS is expected to play a pivotal role in mitigating emissions in sectors that are difficult to decarbonize, regardless of the level of modelling, be it national or sectoral. This highlights existing inconsistencies between the realistic deployment of CCS technologies, nationally announced scenarios, and the net-zero emissions scenarios projected by IAMs. The IEA has developed scenarios such as Stated Policies, Announced Pledges, and Net Zero Emissions to illustrate the variance between realistic and idealistic projections (IEA, 2023a).
6.4.3 Transparency of models and projections
The divergence in objectives and structures across different modelling approaches and communities exacerbates the challenge of achieving coherence and expanding integration. Understanding and correctly interpreting data from both IAMs and LCAs can mitigate these discrepancies. Nonetheless, for CCS/CCU technologies and other emerging technologies undergoing rapid transformation, the integration of external, non-standard data sources remains a widespread practice. This integration is crucial for identifying and quantitatively analysing inconsistencies between model projections and external data, underscoring the importance of bridging these gaps to enhance model reliability and relevance.
The literature reveals a broad spectrum of mitigation cost projections, highlighting the inherent uncertainty in these forecasts. These projections, often rooted in model-based analyses, frequently rely on parameters set within narrowly defined ranges, leading to a prevalence of mean projections from diverse models without comprehensive uncertainty analyses (Weyant, 2017). For instance, the projections concerning electricity mix and the levelized cost of electricity generation serves as foundational elements across numerous IAMs and TEA studies. Yet, divergent outcomes from different models bring debates, underscoring the imperative for transparency regarding the original data sources and the structural framework of models. Recently, some research work is attempting to bridge such divides, for example, Gaugl et al. (2023) linked a bottom-up electricity system model and a top-down macroeconomic model to analyse the increasing the share of renewable electricity on wholesale electricity prices and demand. This study illustrates the significance of socio-economic impacts of changes in the electricity and carbon price, advocating for the employment of transparent data in the development of higher spatial resolution models. This approach not only facilitates a deeper understanding of the underlying causes of projection inconsistencies but also enhances the capacity for critical evaluation and refinement of models. By prioritizing transparency, the research community can work collaboratively to identify and mitigate the sources of discrepancies, thereby improving the reliability and consistency of future projections.
6.4.4 Comprehensiveness and flexibility of models
The dual principles of comprehensiveness and flexibility are essential in the development of IAMs for CCS/CCU, particularly under the stringent emission targets necessitated by climate change mitigation efforts. Flexibility, in this context, refers to the capability of energy systems to adapt to variabilities in electricity supply and demand across various timescales (Bardow et al., 2023). This encompasses adjustments in energy supply, conversion, demand, storage, and the interaction with coupling systems, ensuring resilience and efficiency in both planning and operational phases (Gabrielli et al., 2018).
The evolving energy landscape, marked by the increased penetration of renewable sources and the introduction of new low-carbon technologies, places a premium on flexibility not just in the background energy systems but also within the process designs of the foreground systems, especially in chemical processing (Luo et al., 2022). Operational flexibility within these systems is essential for managing uncertainties and maintaining system efficiency amidst changing energy dynamics.
For IAMs, capturing this flexibility means accurately reflecting the dynamic interplay between technological advancements, operational strategies, and market conditions. Models must be comprehensive, detailing the myriad components of energy systems and their interactions, while also remaining adaptable to integrate new technologies and insights. This balance ensures IAMs can provide relevant and actionable guidance for achieving net-zero emissions through the effective implementation of CCS/CCU technologies and other decarbonization strategies.
7 Conclusion
Why, then, should pLCA and pTEA be utilized despite the uncertainties it carries, particularly in the context of CCS/CCU systems? The energy and process industry stands at the threshold of a significant transformation with the introduction of these technologies. Meanwhile, these technologies are still continuously evolving and improving. Yet, this shift presents the challenge of harmonizing technological progress with environmental stewardship. In this regard, pLCA and pTEA can identify technological and environmental hotspots and also warn for lock-in effects early in the development of these technologies, enabling sustainable design improvements and early intervention. These methodologies provide insights into the potential economic and environmental impacts and the efficacy of new technologies, enabling a comparison of the efficiencies of various CCS/CCU systems in development.
The comprehensive analysis performed in this review, highlights the complexity and necessity of incorporating CCS/CCU technologies within IAMs to achieve global climate targets underlining several key insights.
• Deployment Gap and Policy Interventions: A significant gap exists between the current CCS/CCU deployment (400 Mt CO₂/year) and the Net Zero targets (1 Gt CO₂/year) by 2030. Recent projected costs of DACCS cast doubt on achieving the $100/ton CO2 target. Effective and robust policy interventions, particularly market-based policies, are crucial for reducing costs, creating market demand, and accelerating large-scale deployment.
• Comprehensive System and Life Cycle Evaluation: Prospective assessments must consider changes over time in both background and foreground systems. A comprehensive life cycle analysis framework is needed to evaluate cost reductions and environmental impacts during the scaling-up of technologies.
• Trade-offs within planetary boundaries: Integrating IAMs with prospective assessments helps navigate trade-offs between renewable energy sources, socioeconomic factors, and resource availability, expanding the assessment to include broader impact categories.
• Technology Superstructure and High-Resolution Assessments: Expanding the technology superstructure in modeling to include various TRLs and conducting high-resolution, regionalized assessments are vital for optimizing resource use and planning effective mitigation pathways.
• Carbon Circular Economy: Current IAMs inadequately represent and integrate CO₂ utilization within a carbon circular economy framework, highlighting the need for better incorporation of CCU.
• Open-Sourced and Flexible Tools: Adopting integrated tools like “Premise” and “PyPSA” that combine prospective assessments and enhanced IAMs enhances the transparency and applicability of assessments.
• Data Uncertainties: Addressing data uncertainties and refining the integration of prospective assessment results with IAMs can improve the credibility and impact of these models on policy and IPCC reports, accelerating CCS/CCU deployment.
This review indicates that there are still numerous challenges and limitations associated with applying prospective approaches to CCS/CCU technologies. Building a variety of consistent future scenarios of various likelihood is a complex, highly interdisciplinary task. The different communities including IAMs, TEA, LCA (industry ecology), process system engineering (PSE), experimental groups, stakeholders in both research and industry, which should interact more closely to integrate multiple scales and develop the technologies needed to achieve sustainable development. The effectiveness of these scenarios is often constrained by the creativity of those who devise them and the willingness of the audience to accept their plausibility. Capturing disruptive changes, such as technological breakthroughs, wars, climate tipping points, and unforeseeable events, ranges from difficult to impossible. However, the objective in question does not revolve around predicting future outcomes but rather shaping them through a collaborative approach that involves engaging stakeholders and informing policy decisions, thereby actively influencing the course of technological advancement through strategic investment and innovation. As the field evolves, continuous improvement in modelling approaches and technology assessments will be crucial for informing policy decisions and guiding the global response to climate change.
Author contributions
PW: Conceptualization, Data Curation, Formal Analysis, Methodology, Visualization, Writing–original draft. AJR: Conceptualization, Data Curation, Formal Analysis, Methodology, Visualization, Writing–original draft. SP: Conceptualization, Funding Acquisition, Resources, Supervision, Writing–review and editing.
Funding
The author(s) declare that financial support was received for the research, authorship, and/or publication of this article. PW: TU Wien global budget; AJR: Funded by the European Union. This project has received funding from the European Union’s Horizon Europe research and innovation programme under grant agreement No. 101075727.
Acknowledgments
The authors would like to thank the participants of the GRC Gordon Research Conference Carbon Capture, Utilization, and Storage (2023), of the Autumn School of Open Approaches to Time in LCA (2023).
Conflict of interest
The authors declare that the research was conducted in the absence of any commercial or financial relationships that could be construed as a potential conflict of interest.
Publisher’s note
All claims expressed in this article are solely those of the authors and do not necessarily represent those of their affiliated organizations, or those of the publisher, the editors and the reviewers. Any product that may be evaluated in this article, or claim that may be made by its manufacturer, is not guaranteed or endorsed by the publisher.
Author disclaimer
Views and opinions expressed are however those of the author(s) only and do not necessarily reflect those of the European Union or European Climate, Infrastructure and Environment Executive Agency (CINEA). Neither the European Union nor the granting authority can be held responsible for them.
References
AACE (2020). Cost estimation classification system - as applied in engineering, procurement, and construction for the process industries. Morgantown: AACE.
Air Liquide (2022). Cryocap-Carbon Capture Technologies. Available at: www.engineering-airliquide.com.
Andersen, K. S., Termansen, L. B., Gargiulo, M., and Ó Gallachóirc, B. P. (2019). Bridging the Gap Using Energy Services: Demonstrating a Novel Framework for Soft Linking Top-Down and Bottom-Up Models. Energy 169, 277–293. doi:10.1016/j.energy.2018.11.153
Arlota, C., and Costa, H. K. D. M. (2021a). “Climate change, Carbon Capture and Storage (CCS), energy transition, and justice: where we are now, and where are (should be) we headed?,” in Carbon Capture and Storage in International Energy Policy and Law (Amsterdam: Elsevier), 385–393. doi:10.1016/B978-0-323-85250-0.00019-0
Arlota, C., and Costa, H. K. D. M. (2021b). “Who is taking climate change seriously? Evidence based on a comparative analysis of the carbon capture and storage national legal framework in Brazil, Canada, the European Union, and the United States,” in Carbon Capture and Storage in International Energy Policy and Law (Amsterdam: Elsevier), 235–246. doi:10.1016/B978-0-323-85250-0.00018-9
Artz, J., Müller, T. E., Thenert, K., Kleinekorte, J., Meys, R., Sternberg, A., et al. (2018). Sustainable Conversion of Carbon Dioxide: An Integrated Review of Catalysis and Life Cycle Assessment. Chem. Rev. 118, 434–504. doi:10.1021/acs.chemrev.7b00435
Arvesen, A., Luderer, G., Pehl, M., Bodirsky, B. L., and Hertwich, E. G. (2018). Deriving life cycle assessment coefficients for application in integrated assessment modelling. Environ. Model. Softw. 99, 111–125. doi:10.1016/j.envsoft.2017.09.010
Arvidsson, R., Kushnir, D., Sandén, B. A., and Molander, S. (2014). Prospective Life Cycle Assessment of Graphene Production by Ultrasonication and Chemical Reduction. Environ. Sci. Technol. 48, 4529–4536. doi:10.1021/es405338k
Arvidsson, R., and Molander, S. (2017). Prospective Life Cycle Assessment of Epitaxial Graphene Production at Different Manufacturing Scales and Maturity. J Industrial Ecol. 21, 1153–1164. doi:10.1111/jiec.12526
Arvidsson, R., Tillman, A., Sandén, B. A., Janssen, M., Nordelöf, A., Kushnir, D., et al. (2018). Environmental Assessment of Emerging Technologies: Recommendations for Prospective LCA. J Industrial Ecol. 22, 1286–1294. doi:10.1111/jiec.12690
Bachmann, M., Völker, S., Kleinekorte, J., and Bardow, A. (2023). Syngas from What? Comparative Life-Cycle Assessment for Syngas Production from Biomass, CO2, and Steel Mill Off-Gases. ACS Sustain. Chem. Eng. 11, 5356–5366. doi:10.1021/acssuschemeng.2c05390
Bardow, A., Fiorentini, M., Heer, P., Kämper, A., Koirala, B., Knoeri, C., et al. (2023). Flexibility and sector coupling in energy systems: definitions and metrics: Synthesis report. doi:10.3929/ETHZ-B-000641177
Baumstark, L., Bauer, N., Benke, F., Bertram, C., Bi, S., Gong, C. C., et al. (2021). REMIND2.1: transformation and innovation dynamics of the energy-economic system within climate and sustainability limits. Geosci. Model Dev. 14, 6571–6603. doi:10.5194/gmd-14-6571-2021
Becattini, V., Gabrielli, P., Frattini, L., Weisbach, D., and Mazzotti, M. (2022). A two-step carbon pricing scheme enabling a net-zero and net-negative CO$$_2$$-emissions world. Clim. Change 171, 18. doi:10.1007/s10584-022-03340-z
Becattini, V., Gabrielli, P., and Mazzotti, M. (2021). Role of Carbon Capture, Storage, and Utilization to Enable a Net-Zero-CO2-Emissions Aviation Sector. Ind. Eng. Chem. Res. 60, 6848–6862. doi:10.1021/acs.iecr.0c05392
Bergesen, J. D., and Suh, S. (2016). A Framework for Technological Learning in the Supply Chain: A Case Study on CdTe Photovoltaics. Appl. Energy 169, 721–728. doi:10.1016/j.apenergy.2016.02.013
Biermann, M., Langner, C., Roussanaly, S., Normann, F., and Harvey, S. (2022). The Role of Energy Supply in Abatement Cost Curves for CO2 Capture from Process Industry – A Case Study of a Swedish Refinery. Appl. Energy 319, 119273. doi:10.1016/j.apenergy.2022.119273
Blanco, H., Nijs, W., Ruf, J., and Faaij, A. (2018a). Potential for Hydrogen and Power-to-Liquid in a low-carbon EU Energy System Using Cost Optimization. Appl. Energy 232, 617–639. doi:10.1016/j.apenergy.2018.09.216
Blanco, H., Nijs, W., Ruf, J., and Faaij, A. (2018b). Potential of Power-to-Methane in the EU Energy Transition to a Low Carbon System Using Cost Optimization. Appl. Energy 232, 323–340. doi:10.1016/j.apenergy.2018.08.027
Bolinger, M., Wiser, R., and O’Shaughnessy, E. (2022). Levelized Cost-Based Learning Analysis of Utility-Scale Wind and Solar in the United States. iScience 25, 104378. doi:10.1016/j.isci.2022.104378
Bouaboula, H., Chaouki, J., Belmabkhout, Y., and Zaabout, A. (2024). Comparative review of Direct air capture technologies: From technical, commercial, economic, and environmental aspects. Chem. Eng. J. 484, 149411. doi:10.1016/j.cej.2024.149411
Briones-Hidrovo, A., Copa Rey, J. R., Cláudia Dias, A., Tarelho, L. A. C., and Beauchet, S. (2022). Assessing a bio-energy system with carbon capture and storage (BECCS) through dynamic life cycle assessment and land-water-energy nexus. Energy Convers. Manag. 268, 116014. doi:10.1016/j.enconman.2022.116014
Brown, T., Hörsch, J., Hofmann, F., Neumann, F., Zeyen, L., Syranidis, C., et al. (2024). PyPSA: Python for Power System Analysis. J. open Res. Softw. 6 (1), 4. doi:10.5334/jors.188
Brown, T., Schlachtberger, D., Kies, A., Schramm, S., and Greiner, M. (2018). Synergies of sector coupling and transmission reinforcement in a cost-optimised, highly renewable European energy system. Energy 160, 720–739. doi:10.1016/j.energy.2018.06.222
Bui, M., Adjiman, C. S., Bardow, A., Anthony, E. J., Boston, A., Brown, S., et al. (2018). Carbon capture and storage (CCS): the way forward. Energy Environ. Sci. 11, 1062–1176. doi:10.1039/C7EE02342A
Burger, J., Nöhl, J., Seiler, J., Gabrielli, P., Oeuvray, P., Becattini, V., et al. (2024). Environmental impacts of carbon capture, transport, and storage supply chains: Status and the way forward. Int. J. Greenh. Gas Control 132, 104039. doi:10.1016/j.ijggc.2023.104039
Burns, T. D., Pai, K. N., Subraveti, S. G., Collins, S. P., Krykunov, M., Rajendran, A., et al. (2020). Prediction of MOF Performance in Vacuum Swing Adsorption Systems for Postcombustion CO 2 Capture Based on Integrated Molecular Simulations, Process Optimizations, and Machine Learning Models. Environ. Sci. Technol. 54, 4536–4544. doi:10.1021/acs.est.9b07407
Buyle, A., Billen, B., Van, P., Boonen, K., and Van Passel, S. (2019). The Future of Ex-Ante LCA? Lessons Learned and Practical Recommendations. Sustainability 11, 5456. doi:10.3390/su11195456
Calvin, K., Dasgupta, D., Krinner, G., Denton, F., Diongue-Niang, A., Dodman, D., et al. (2023). “IPCC, 2023: Climate Change 2023: Synthesis Report,” in Contribution of Working Groups I, II and III to the Sixth Assessment Report of the Intergovernmental Panel on Climate Change Core Writing Team. Editors H. Lee, and J. Romero (Geneva, Switzerland: IPCC). doi:10.59327/IPCC/AR6-9789291691647
Calvin, K., Patel, P., Clarke, L., Asrar, G., Bond-Lamberty, B., Cui, R. Y., et al. (2019). GCAM v5.1: representing the linkages between energy, water, land, climate, and economic systems. Geosci. Model Dev. 12, 677–698. doi:10.5194/gmd-12-677-2019
Carbfix (2024). Carbfix-We turn CO2 into stone. Available at: https://www.carbfix.com.
Carbon Engineering (2024). 1 PointFive-the first DAC facility of Carbon Engineering. Available at: https://www.1pointfive.com/ector-county-tx.
Charalambous, C., Moubarak, E., Schilling, J., Sanchez Fernandez, E., Wang, J.-Y., Herraiz, L., et al. (2023). Shedding Light on the Stakeholders’ Perspectives for Carbon Capture. Chemistry. doi:10.26434/chemrxiv-2023-sn90q
Chauvy, R., and De Weireld, G. (2020). CO 2 Utilization Technologies in Europe: A Short Review. Energy Tech. 8, 2000627. doi:10.1002/ente.202000627
Chris, M. (2024). Brightway-lca/Brightway2. Available at: https://github.com/brightway-lca/brightway2 (Accessed March 19, 2024).
Christensen, A., and Petrenko, C. (2017). CO2-Based Synthetic Fuel: Assessment of Potential European Capacity and Environmental Performance. Available at: https://theicct.org/publication/co2-based-synthetic-fuel-assessment-of-potential-european-capacity-and-environmental-performance/.
Chung, W., Lim, H., Lee, J. S., Al-Hunaidy, A. S., Imran, H., Jamal, A., et al. (2022). Computer-aided identification and evaluation of technologies for sustainable carbon capture and utilization using a superstructure approach. J. CO2 Util. 61, 102032. doi:10.1016/j.jcou.2022.102032
Climeworks (2024). Mammoth: our newest facility. Available at: https://climeworks.com/plant-mammoth.
Cointe, B., Cassen, C., and Nadaï, A. (2019). Organising Policy-Relevant Knowledge for Climate Action: Integrated Assessment Modelling, the IPCC, and the Emergence of a Collective Expertise on Socioeconomic Emission Scenarios. Sci. Technol. Stud. 32 (4), 36–57. doi:10.23987/sts.65031
Collingridge, D. (1982). The Social Control of Technology. New York: St.Martin’s Press. Available at: https://repository.library.georgetown.edu/handle/10822/792071 (Accessed March 19, 2024).
Cox, B., Bauer, C., Mendoza Beltran, A., Van Vuuren, D. P., and Mutel, C. L. (2020). Life cycle environmental and cost comparison of current and future passenger cars under different energy scenarios. Appl. Energy 269, 115021. doi:10.1016/j.apenergy.2020.115021
Cox, B., Jemiolo, W., and Mutel, C. (2018). Life cycle assessment of air transportation and the Swiss commercial air transport fleet. Transp. Res. Part D Transp. Environ. 58, 1–13. doi:10.1016/j.trd.2017.10.017
CREATE (2023). Capito, Sinema, Murkowski, Whitehouse Introduce Bipartisan Carbon Capture Legislation. Available at: https://www.epw.senate.gov/public/index.cfm/2023/6/capito-sinema-murkowski-whitehouse-introduce-bipartisan-carbon-capture-legislation.
Cucurachi, S., Van Der Giesen, C., and Guinée, J. (2018). Ex-ante LCA of Emerging Technologies. Procedia CIRP 69, 463–468. doi:10.1016/j.procir.2017.11.005
Dalla Longa, F., Detz, R., and Van Der Zwaan, B. (2020). Integrated assessment projections for the impact of innovation on CCS deployment in Europe. Int. J. Greenh. Gas Control 103, 103133. doi:10.1016/j.ijggc.2020.103133
Desport, L., and Selosse, S. (2022). An overview of CO2 capture and utilization in energy models. Resour. Conservation Recycl. 180, 106150. doi:10.1016/j.resconrec.2021.106150
Detz, R. J., Reek, J. N. H., and Van Der Zwaan, B. C. C. (2018). The future of solar fuels: when could they become competitive? Energy Environ. Sci. 11, 1653–1669. doi:10.1039/C8EE00111A
Deutz, S., and Bardow, A. (2021). Life-cycle assessment of an industrial direct air capture process based on temperature–vacuum swing adsorption. Nat. Energy 6, 203–213. doi:10.1038/s41560-020-00771-9
DOE-EPRI (2020). Initial Engineering Design of a Post-Combustion CO2 Capture System for Duke Energy’s East Bend Station Using Membrane-based technology. Washington, D.C: DOE-EPRI.
Dziejarski, B., Krzyżyńska, R., and Andersson, K. (2023). Current status of carbon capture, utilization, and storage technologies in the global economy: A survey of technical assessment. Fuel 342, 127776. doi:10.1016/j.fuel.2023.127776
Elke, S., Detlef, von V., Tom, K., and Lex, B. (2014). Integrated Assessment of Global Environmental Change with IMAGE 3.0. The Hague: PBL Netherlands Environmental Assessment Agency.
Energy Transitions Commission (2022). Carbon Capture, Utilisation and Storage in the Energy Transition: Vital but Limited. London, UK: Energy Transitions Commission. Available at: www.energy-transitions.org.
European Commission (2022). Commission invests €3 billion in innovative clean tech projects to deliver on REPowerEU and accelerate Europe’s energy independence from Russian fossil fuels. Available at: https://ec.europa.eu/commission/presscorner/detail/en/ip_22_6489.
European Commission - Joint Research Centre (2010). International reference life cycle data system (ILCD) Handbook - general guide for life cycle assessment - detailed guidance. Luxembourg: Publications office of the European Union.
Faber, G., Ruttinger, A., Strunge, T., Langhorst, T., Zimmermann, A., Van Der Hulst, M., et al. (2022). Adapting technology learning curves for prospective techno-economic and life cycle assessments of emerging carbon capture and utilization pathways. Front. Clim. 4, 820261. doi:10.3389/fclim.2022.820261
Fasihi, M., Efimova, O., and Breyer, C. (2019). Techno-economic assessment of CO2 direct air capture plants. J. Clean. Prod. 224, 957–980. doi:10.1016/j.jclepro.2019.03.086
Ferioli, F., Schoots, K., and Van Der Zwaan, B. C. C. (2009). Use and limitations of learning curves for energy technology policy: a component-learning hypothesis. Energy Policy 37, 2525–2535. doi:10.1016/j.enpol.2008.10.043
Fernández-Dacosta, C., Stojcheva, V., and Ramirez, A. (2018). Closing carbon cycles: evaluating the performance of multi-product CO2 utilisation and storage configurations in a refinery. J. CO2 Util. 23, 128–142. doi:10.1016/j.jcou.2017.11.008
Ferrari, A. M., Volpi, L., Settembre-Blundo, D., and García-Muiña, F. E. (2021). Dynamic life cycle assessment (LCA) integrating life cycle inventory (LCI) and Enterprise resource planning (ERP) in an industry 4.0 environment. J. Clean. Prod. 286, 125314. doi:10.1016/j.jclepro.2020.125314
Frattini, L., Becattini, V., Krüger, M., Akeret, O., and Mazzotti, M. (2022). “Legal and regulatory gaps for carbon dioxide capture, transport, and storage deployment,” in Proceedings of the 16th Greenhouse Gas Control Technologies Confernece, 23-24 Oct 2022, 431–448. doi:10.2139/ssrn.4285233
Frischknecht, R., Jungbluth, N., Althaus, H.-J., Doka, G., Dones, R., Heck, T., et al. (2005). The ecoinvent database: overview and methodological framework (7 pp). Int. J. Life Cycle Assess. 10, 3–9. doi:10.1065/lca2004.10.181.1
Gabrielli, P., Gazzani, M., Martelli, E., and Mazzotti, M. (2018). Optimal design of multi-energy systems with seasonal storage. Appl. Energy 219, 408–424. doi:10.1016/j.apenergy.2017.07.142
Gabrielli, P., Rosa, L., Gazzani, M., Meys, R., Bardow, A., Mazzotti, M., et al. (2023). Net-zero emissions chemical industry in a world of limited resources. One Earth 6, 682–704. doi:10.1016/j.oneear.2023.05.006
Gallego Dávila, J., Sacchi, R., and Pizzol, M. (2023). Preconditions for achieving carbon neutrality in cement production through CCUS. J. Clean. Prod. 425, 138935. doi:10.1016/j.jclepro.2023.138935
Garcia, M., and Berghout, N. (2019). Toward a common method of cost-review for carbon capture technologies in the industrial sector: cement and iron and steel plants. Int. J. Greenh. Gas Control 87, 142–158. doi:10.1016/j.ijggc.2019.05.005
Garcia-Garcia, G., Fernandez, M. C., Armstrong, K., Woolass, S., and Styring, P. (2021). Analytical review of life-cycle environmental impacts of carbon capture and utilization technologies. ChemSusChem 14, 995–1015. doi:10.1002/cssc.202002126
García-Gusano, D., Garraín, D., Herrera, I., Cabal, H., and Lechón, Y. (2015). Life Cycle Assessment of applying CO 2 post-combustion capture to the Spanish cement production. J. Clean. Prod. 104, 328–338. doi:10.1016/j.jclepro.2013.11.056
Gardarsdottir, S., De Lena, E., Romano, M., Roussanaly, S., Voldsund, M., Pérez-Calvo, J.-F., et al. (2019). Comparison of technologies for CO2 capture from cement production—Part 2: cost analysis. Energies 12, 542. doi:10.3390/en12030542
Gaugl, R., Sommer, M., Kettner, C., Bachhiesl, U., Klatzer, T., Gruber, L., et al. (2023). Integrated power and economic analysis of Austria’s renewable electricity transformation. Energies 16, 2229. doi:10.3390/en16052229
Georgiades, M., Shah, I. H., Steubing, B., Cheeseman, C., and Myers, R. J. (2023). Prospective life cycle assessment of European cement production. Resour. Conservation Recycl. 194, 106998. doi:10.1016/j.resconrec.2023.106998
Gibon, T., Wood, R., Arvesen, A., Bergesen, J. D., Suh, S., and Hertwich, E. G. (2015). A methodology for integrated, Multiregional life cycle assessment scenarios under large-scale technological change. Environ. Sci. Technol. 49, 11218–11226. doi:10.1021/acs.est.5b01558
Gjorgiev, B., Garrison, J. B., Han, X., Landis, F., Van Nieuwkoop, R., Raycheva, E., et al. (2022). Nexus-e: a platform of interfaced high-resolution models for energy-economic assessments of future electricity systems. Appl. Energy 307, 118193. doi:10.1016/j.apenergy.2021.118193
Gładysz, P., and Ziębik, A. (2015). Life cycle assessment of an integrated oxy-fuel combustion power plant with CO2 capture, transport and storage ‒ Poland case study. Energy 92, 328–340. doi:10.1016/j.energy.2015.07.052
Global CCS Insitute (2021). Technology readiness and costs of ccs. Available at: https://www.globalccsinstitute.com/resources/publications-reports-research/technology-readiness-and-costs-of-ccs/.
Global CCS Insitute (2023). The global status of CCS: 2023. Australia: Global CCS Insitute. Available at: https://status23.globalccsinstitute.com/(Accessed January 10, 2024).
Hanna, R., Abdulla, A., Xu, Y., and Victor, D. G. (2021). Emergency deployment of direct air capture as a response to the climate crisis. Nat. Commun. 12, 368. doi:10.1038/s41467-020-20437-0
Harpprecht, C., Naegler, T., Steubing, B., Tukker, A., and Simon, S. (2022). Decarbonization scenarios for the iron and steel industry in context of a sectoral carbon budget: Germany as a case study. J. Clean. Prod. 380, 134846. doi:10.1016/j.jclepro.2022.134846
Hasan, M. M. F., Zantye, M. S., and Kazi, M.-K. (2022). Challenges and opportunities in carbon capture, utilization and storage: a process systems engineering perspective. Comput. Chem. Eng. 166, 107925. doi:10.1016/j.compchemeng.2022.107925
Heijungs, R., and Frischknecht, R. (1998). A special view on the nature of the allocation problem. Int. J. LCA 3, 321–332. doi:10.1007/BF02979343
Hellweg, S., and Milà I Canals, L. (2014). Emerging approaches, challenges and opportunities in life cycle assessment. Science 344, 1109–1113. doi:10.1126/science.1248361
Hepburn, C., Adlen, E., Beddington, J., Carter, E. A., Fuss, S., Mac Dowell, N., et al. (2019). The technological and economic prospects for CO2 utilization and removal. Nature 575, 87–97. doi:10.1038/s41586-019-1681-6
Hetherington, A. C., Borrion, A. L., Griffiths, O. G., and McManus, M. C. (2014). Use of LCA as a development tool within early research: challenges and issues across different sectors. Int. J. Life Cycle Assess. 19, 130–143. doi:10.1007/s11367-013-0627-8
Hoppe, W., Thonemann, N., and Bringezu, S. (2018). Life cycle assessment of carbon dioxide–based production of methane and methanol and derived polymers. J Industrial Ecol. 22, 327–340. doi:10.1111/jiec.12583
IEA (2020). CCUS in clean energy transitions. Paris: IEA. Available at: https://www.iea.org/reports/ccus-in-clean-energy-transitions.
IEA (2023a). CCUS policies and Business models: Building a commercial market. Paris: OECD. doi:10.1787/6ef05538-en
IEA (2023b). Section 45 Q Credit for carbon oxide sequestration. Available at: https://www.iea.org/policies/4986-section-45q-credit-for-carbon-oxide-sequestration.
IEAGHGIEAGHG (2013). Towards a common method of cost estimation for CO2 capture and storage at fossil fuel power plants. Cheltenham, UK: IEAGHGIEAGHG.
Ioannou, I., Galán-Martín, Á., Pérez-Ramírez, J., and Guillén-Gosálbez, G. (2023a). Trade-offs between sustainable development goals in carbon capture and utilisation. Energy Environ. Sci. 16, 113–124. doi:10.1039/D2EE01153K
Ioannou, I., Javaloyes-Antón, J., Caballero, J. A., and Guillén-Gosálbez, G. (2023b). Economic and environmental performance of an integrated CO2 refinery. ACS Sustain. Chem. Eng. 11, 1949–1961. doi:10.1021/acssuschemeng.2c06724
Ipcc (2022). Global warming of 1.5°C: IPCC special report on impacts of global warming of 1.5°C above pre-industrial levels in context of strengthening response to climate change, sustainable development, and efforts to Eradicate Poverty. 1st Edn. Cambridge, UK: Cambridge University Press. doi:10.1017/9781009157940
IPCC ed (2023). Climate change 2022 - mitigation of climate change: working group III contribution to the Sixth assessment report of the Intergovernmental Panel on climate change. 1st Edn. Cambridge, UK: Cambridge University Press. doi:10.1017/9781009157926
ISO 14040 (2006a). Environmental management-Life cycle assessment: Priciples and framework. Available at: https://www.iso.org/standard/37456.html (Accessed March 19, 2024).
ISO 14044 (2006b). Environmental management — life cycle assessment — requirements and guidelines. Available at: https://www.iso.org/standard/38498.html (Accessed March 19, 2024).
Jin, S. W., Li, Y. P., Nie, S., and Sun, J. (2017). The potential role of carbon capture and storage technology in sustainable electric-power systems under multiple uncertainties. Renew. Sustain. Energy Rev. 80, 467–480. doi:10.1016/j.rser.2017.05.230
Kermeli, K., Edelenbosch, O. Y., Crijns-Graus, W., van Ruijven, B. J., Mima, S., van Vuuren, D. P., et al. (2019). The scope for better industry representation in long-term energy models: modeling the cement industry. Appl. Energy 240, 964–985. doi:10.1016/j.apenergy.2019.01.252
Kettner, C., Böheim, M., Sommer, M., Gaugl, R., Bachhiesl, U., Gruber, L., et al. (2024). Transformation to a renewable electricity system in Austria: insights from an integrated model analysis. Renew. Energy 223, 119957. doi:10.1016/j.renene.2024.119957
Kishimoto, P. N., Wienpahl, L., Zakeri, B., Lovat, F., Fricko, O., Gidden, M. J., et al. (2024). MESSAGEix. Available at: https://zenodo.org/badge/DOI/10.5281/zenodo.4005684.svg.
Kleinekorte, J., Kleppich, J., Fleitmann, L., Beckert, V., Blodau, L., and Bardow, A. (2023). APPROPRIATE life cycle assessment: a PRO cess-specific, PR edictive I mpact A ssessmen T method for emerging chemical processes. ACS Sustain. Chem. Eng. 11, 9303–9319. doi:10.1021/acssuschemeng.2c07682
Koornneef, J., Van Keulen, T., Faaij, A., and Turkenburg, W. (2008). Life cycle assessment of a pulverized coal power plant with post-combustion capture, transport and storage of CO2. Int. J. Greenh. Gas Control 2, 448–467. doi:10.1016/j.ijggc.2008.06.008
Krook-Riekkola, A., Berg, C., Ahlgren, E. O., and Söderholm, P. (2017). Challenges in top-down and bottom-up soft-linking: Lessons from linking a Swedish energy system model with a CGE model. Energy 141, 803–817. doi:10.1016/j.energy.2017.09.107
Kursun, B., Ramkumar, S., Bakshi, B. R., and Fan, L.-S. (2014). Life cycle comparison of coal gasification by conventional versus calcium looping processes. Ind. Eng. Chem. Res. 53, 18910–18919. doi:10.1021/ie404436a
Lamers, P., Ghosh, T., Upasani, S., Sacchi, R., and Daioglou, V. (2023). Linking life cycle and integrated assessment modeling to evaluate technologies in an evolving system context: a power-to-hydrogen case study for the United States. Environ. Sci. Technol. 57, 2464–2473. doi:10.1021/acs.est.2c04246
Langkau, S., Steubing, B., Mutel, C., Ajie, M. P., Erdmann, L., Voglhuber-Slavinsky, A., et al. (2023). A stepwise approach for scenario-based inventory modelling for prospective LCA (SIMPL). Int. J. Life Cycle Assess. 28, 1169–1193. doi:10.1007/s11367-023-02175-9
Leeson, D., Mac Dowell, N., Shah, N., Petit, C., and Fennell, P. S. (2017). A Techno-economic analysis and systematic review of carbon capture and storage (CCS) applied to the iron and steel, cement, oil refining and pulp and paper industries, as well as other high purity sources. Int. J. Greenh. Gas Control 61, 71–84. doi:10.1016/j.ijggc.2017.03.020
Lei, T., Wang, D., Yu, X., Ma, S., Zhao, W., Cui, C., et al. (2023). Global iron and steel plant CO2 emissions and carbon-neutrality pathways. Nature 622, 514–520. doi:10.1038/s41586-023-06486-7
Li, X., and Maréchal, F. (2023). Deep excavation of the impact from endogenous and exogenous uncertainties on long-term energy planning. Energy AI 11, 100219. doi:10.1016/j.egyai.2022.100219
Lin, J.-B., Nguyen, T. T. T., Vaidhyanathan, R., Burner, J., Taylor, J. M., Durekova, H., et al. (2021). A scalable metal-organic framework as a durable physisorbent for carbon dioxide capture. Science 374, 1464–1469. doi:10.1126/science.abi7281
Liu, C. M., Sandhu, N. K., McCoy, S. T., and Bergerson, J. A. (2020). A life cycle assessment of greenhouse gas emissions from direct air capture and Fischer–Tropsch fuel production. Sustain. Energy Fuels 4, 3129–3142. doi:10.1039/C9SE00479C
Luderer, G., Pehl, M., Arvesen, A., Gibon, T., Bodirsky, B. L., De Boer, H. S., et al. (2019). Environmental co-benefits and adverse side-effects of alternative power sector decarbonization strategies. Nat. Commun. 10, 5229. doi:10.1038/s41467-019-13067-8
Luo, Y., Wu, X., and Ding, X. (2022). Carbon and water footprints assessment of cotton jeans using the method based on modularity: a full life cycle perspective. J. Clean. Prod. 332, 130042. doi:10.1016/j.jclepro.2021.130042
Mac Dowell, N., Fennell, P. S., Shah, N., and Maitland, G. C. (2017). The role of CO2 capture and utilization in mitigating climate change. Nat. Clim. Change 7, 243–249. doi:10.1038/nclimate3231
Madeddu, S., Ueckerdt, F., Pehl, M., Peterseim, J., Lord, M., Kumar, K. A., et al. (2020). The CO 2 reduction potential for the European industry via direct electrification of heat supply (power-to-heat). Environ. Res. Lett. 15, 124004. doi:10.1088/1748-9326/abbd02
Malhotra, A., and Schmidt, T. S. (2020). Accelerating low-carbon innovation. Joule 4, 2259–2267. doi:10.1016/j.joule.2020.09.004
Marchese, M., Buffo, G., Santarelli, M., and Lanzini, A. (2021). CO2 from direct air capture as carbon feedstock for Fischer-Tropsch chemicals and fuels: energy and economic analysis. J. CO2 Util. 46, 101487. doi:10.1016/j.jcou.2021.101487
Marcucci, A., Kypreos, S., and Panos, E. (2017). The road to achieving the long-term Paris targets: energy transition and the role of direct air capture. Clim. Change 144, 181–193. doi:10.1007/s10584-017-2051-8
McGrail, B. P., Schaef, H. T., Spane, F. A., Horner, J. A., Owen, A. T., Cliff, J. B., et al. (2017). Wallula basalt pilot demonstration project: post-injection results and conclusions. Energy Procedia 114, 5783–5790. doi:10.1016/j.egypro.2017.03.1716
McQueen, N., Gomes, K. V., McCormick, C., Blumanthal, K., Pisciotta, M., and Wilcox, J. (2021). A review of direct air capture (DAC): scaling up commercial technologies and innovating for the future. Prog. Energy 3, 032001. doi:10.1088/2516-1083/abf1ce
Mendoza Beltran, A., Cox, B., Mutel, C., Van Vuuren, D. P., Font Vivanco, D., Deetman, S., et al. (2020). When the background Matters: using scenarios from integrated assessment models in prospective life cycle assessment. J Industrial Ecol. 24, 64–79. doi:10.1111/jiec.12825
Middleton, R. S., Yaw, S. P., Hoover, B. A., and Ellett, K. M. (2020). SimCCS: an open-source tool for optimizing CO2 capture, transport, and storage infrastructure. Environ. Model. Softw. 124, 104560. doi:10.1016/j.envsoft.2019.104560
Mo, J., Schleich, J., and Fan, Y. (2018). Getting ready for future carbon abatement under uncertainty – key factors driving investment with policy implications. Energy Econ. 70, 453–464. doi:10.1016/j.eneco.2018.01.026
Müller, L. J., Kätelhön, A., Bachmann, M., Zimmermann, A., Sternberg, A., and Bardow, A. (2020). A guideline for life cycle assessment of carbon capture and utilization. Front. Energy Res. 8, 15. doi:10.3389/fenrg.2020.00015
National Academies of Sciences, Engineering, and Medicine (2023). Carbon Dioxide utilization markets and infrastructure: status and opportunities: a first report. Washington, D.C.: National Academies Press. doi:10.17226/26703
Negri, V., Galán-Martín, Á., Pozo, C., Fajardy, M., Reiner, D. M., Mac Dowell, N., et al. (2021). Life cycle optimization of BECCS supply chains in the European Union. Appl. Energy 298, 117252. doi:10.1016/j.apenergy.2021.117252
NETL (2013). Technology learning curve (FOAK to NOAK). Houston, TX: National Energy Technology Laboratory.
NETL (2014). Cost of capturing CO2 from industrical sources. Houston, TX: National Energy Technology Laboratory.
NETL (2022). FECM/NETL CO2 transport cost model (2022): description and User’s manual. Houston, TX: National Energy Technology Laboratory.
Nunes, R. de C., and Costa, H. K. D. M. (2021). How ccs projects are developed in selected countries? Int. J. Dev. Res. 11, 48829–48834. doi:10.37118/ijdr.22314.07.2021
Oeuvray, P., Burger, J., Roussanaly, S., Mazzotti, M., and Becattini, V. (2024). Multi-criteria assessment of inland and offshore carbon dioxide transport options. J. Clean. Prod. 443, 140781. doi:10.1016/j.jclepro.2024.140781
Olah, G. A., Goeppert, A., and Prakash, G. K. S. (2009). Chemical recycling of carbon dioxide to methanol and dimethyl ether: from greenhouse gas to renewable, environmentally carbon neutral fuels and synthetic hydrocarbons. J. Org. Chem. 74, 487–498. doi:10.1021/jo801260f
Parson, E. A., and Fisher-Vanden, A. K. (1997). Integrated assessment models of global climate change. Annu. Rev. Energy. Environ. 22, 589–628. doi:10.1146/annurev.energy.22.1.589
Pehl, M., Arvesen, A., Humpenöder, F., Popp, A., Hertwich, E. G., and Luderer, G. (2017). Understanding future emissions from low-carbon power systems by integration of life-cycle assessment and integrated energy modelling. Nat. Energy 2, 939–945. doi:10.1038/s41560-017-0032-9
Perry, R. H., and Don, W. G. (2008). Perry’s chemical engineers’ Handbook. 8th ed. New York: McGraw-Hill.
Petrescu, L., Bonalumi, D., Valenti, G., Cormos, A.-M., and Cormos, C.-C. (2017). Life Cycle Assessment for supercritical pulverized coal power plants with post-combustion carbon capture and storage. J. Clean. Prod. 157, 10–21. doi:10.1016/j.jclepro.2017.03.225
Pett-Ridge, J., Kuebbing, S., Mayer, A., Hovorka, S., Pilorge, H., Baker, S., et al. (2023). Roads to removal: options for carbon dioxide removal in the United States. Technical Report: Roads to Removal: Options for Carbon Dioxide Removal in the United States. doi:10.2172/2301853
Pini, M., Cedillo González, E., Neri, P., Siligardi, C., and Ferrari, A. (2017). Assessment of environmental performance of TiO2 nanoparticles coated self-cleaning float glass. Coatings 7, 8. doi:10.3390/coatings7010008
Qiu, Y., Lamers, P., Daioglou, V., McQueen, N., De Boer, H.-S., Harmsen, M., et al. (2022). Environmental trade-offs of direct air capture technologies in climate change mitigation toward 2100. Nat. Commun. 13, 3635. doi:10.1038/s41467-022-31146-1
Reinert, C., Schellhas, L., Mannhardt, J., Shu, D. Y., Kämper, A., Baumgärtner, N., et al. (2022). SecMOD: an open-source Modular framework combining multi-sector system optimization and life-cycle assessment. Front. Energy Res. 10. doi:10.3389/fenrg.2022.884525
Rocha, H., and Costa, H. K. D. M. (2021). “Legal and regulatory barriers to CO2 geological storage in Brazil: Lessons from the European Union,” in Carbon capture and storage in international energy policy and law (Amsterdam: Elsevier), 263–283. doi:10.1016/B978-0-323-85250-0.00008-6
Roh, K., Bardow, A., Bongartz, D., Burre, J., Chung, W., Deutz, S., et al. (2020). Early-stage evaluation of emerging CO 2 utilization technologies at low technology readiness levels. Green Chem. 22, 3842–3859. doi:10.1039/C9GC04440J
Roussanaly, S., Berghout, N., Fout, T., Garcia, M., Gardarsdottir, S., Nazir, S. M., et al. (2021). Towards improved cost evaluation of Carbon Capture and Storage from industry. Int. J. Greenh. Gas Control 106, 103263. doi:10.1016/j.ijggc.2021.103263
Rubin, E. S. (2019). Improving cost estimates for advanced low-carbon power plants. Int. J. Greenh. Gas Control 88, 1–9. doi:10.1016/j.ijggc.2019.05.019
Rubin, E. S., Berghout, N., Booras, G., Fout, T., Garcia, M., Nazir, S. M., et al. (2021). Toward improved cost guidelines for advanced low-carbon technologies. SSRN J. doi:10.2139/ssrn.3818896
Rubin, E. S., Mantripragada, H., Marks, A., Versteeg, P., and Kitchin, J. (2012). The outlook for improved carbon capture technology. Prog. Energy Combust. Sci. 38, 630–671. doi:10.1016/j.pecs.2012.03.003
Rubin, E. S., Yeh, S., Antes, M., Berkenpas, M., and Davison, J. (2007). Use of experience curves to estimate the future cost of power plants with CO2 capture. Int. J. Greenh. Gas Control 1, 188–197. doi:10.1016/S1750-5836(07)00016-3
Sacchi, R., Becattini, V., Gabrielli, P., Cox, B., Dirnaichner, A., Bauer, C., et al. (2023a). How to make climate-neutral aviation fly. Nat. Commun. 14, 3989. doi:10.1038/s41467-023-39749-y
Sacchi, R., Terlouw, T., Siala, K., Dirnaichner, A., Bauer, C., Cox, B., et al. (2022). PRospective EnvironMental Impact asSEment (premise): a streamlined approach to producing databases for prospective life cycle assessment using integrated assessment models. Renew. Sustain. Energy Rev. 160, 112311. doi:10.1016/j.rser.2022.112311
Sacchi, R., Terlouw, T., Siala, K., Dirnaichner, A., Bauer, C., Cox, B., et al. (2023b). Premise. Available at: https://github.com/polca/premise (Accessed March 19, 2024).
Schakel, W., Hung, C. R., Tokheim, L.-A., Strømman, A. H., Worrell, E., and Ramírez, A. (2018). Impact of fuel selection on the environmental performance of post-combustion calcium looping applied to a cement plant. Appl. Energy 210, 75–87. doi:10.1016/j.apenergy.2017.10.123
Shu, D. Y., Deutz, S., Winter, B. A., Baumgärtner, N., Leenders, L., and Bardow, A. (2023). The role of carbon capture and storage to achieve net-zero energy systems: trade-offs between economics and the environment. Renew. Sustain. Energy Rev. 178, 113246. doi:10.1016/j.rser.2023.113246
Sick, V., Armstrong, K., Cooney, G., Cremonese, L., Eggleston, A., Faber, G., et al. (2020). The need for and Path to harmonized life cycle assessment and techno-economic assessment for carbon dioxide capture and utilization. Energy Technol. 8, 1901034. doi:10.1002/ente.201901034
Sievert, K., Schmidt, T. S., and Steffen, B. (2024). Considering technology characteristics to project future costs of direct air capture. Joule 8, 979–999. doi:10.1016/j.joule.2024.02.005
Singh, B., Strømman, A. H., and Hertwich, E. G. (2011). Comparative life cycle environmental assessment of CCS technologies. Int. J. Greenh. Gas Control 5, 911–921. doi:10.1016/j.ijggc.2011.03.012
Skone, M., Mutchek, M., and Krynock, M. (2022). Carbon Dioxide utilization life cycle analysis guidance for the U.S. DOE Office of fossil energy and carbon management version 2.0. Houston, TX: National Energy Technology Laboratory.
Slameršak, A., Kallis, G., and O’Neill, D. W. (2022). Energy requirements and carbon emissions for a low-carbon energy transition. Nat. Commun. 13, 6932. doi:10.1038/s41467-022-33976-5
Smit, R., Helmers, E., Schwingshackl, M., Opetnik, M., and Kennedy, D. (2024). Greenhouse gas emissions performance of electric, hydrogen and fossil-Fuelled Freight trucks with uncertainty estimates using a probabilistic life-cycle assessment (pLCA). Sustainability 16, 762. doi:10.3390/su16020762
Spath, P. L., and Mann, M. K. (2004). Biomass power and conventional fossil systems with and without CO2 sequestration – comparing the energy balance. Greenh. Gas Emiss. Econ.
Spiekermann, L. A., Araújo, T. P., Seiler, J. M., Pérez-Ramírez, J., and Bardow, A. (2023). Bridging the scales from catalyst synthesis to sustainable processes: Co2 hydrogenation to methanol. San Antonio, TX, USA.
Sternberg, A., and Bardow, A. (2016). Life cycle assessment of power-to-gas: syngas vs methane. ACS Sustain. Chem. Eng. 4, 4156–4165. doi:10.1021/acssuschemeng.6b00644
Steubing, B., de Koning, D., Haas, A., and Mutel, C. L. (2020). The Activity Browser — an open source LCA software building on top of the brightway framework. Softw. Impacts 3, 100012. doi:10.1016/j.simpa.2019.100012
Tang, Y., and You, F. (2018). Life cycle environmental and economic analysis of pulverized coal oxy-fuel combustion combining with calcium looping process or chemical looping air separation. J. Clean. Prod. 181, 271–292. doi:10.1016/j.jclepro.2018.01.265
Tanzer, S. E., and Ramírez, A. (2019). When are negative emissions negative emissions? Energy Environ. Sci. 12, 1210–1218. doi:10.1039/C8EE03338B
Terlouw, T., Bauer, C., Rosa, L., and Mazzotti, M. (2021). Life cycle assessment of carbon dioxide removal technologies: a critical review. Energy Environ. Sci. 14, 1701–1721. doi:10.1039/D0EE03757E
Thomassen, G., Van Passel, S., and Dewulf, J. (2020). A review on learning effects in prospective technology assessment. Renew. Sustain. Energy Rev. 130, 109937. doi:10.1016/j.rser.2020.109937
Thonemann, N. (2020). Environmental impacts of CO2-based chemical production: a systematic literature review and meta-analysis. Appl. Energy 263, 114599. doi:10.1016/j.apenergy.2020.114599
Thonemann, N., and Pizzol, M. (2019). Consequential life cycle assessment of carbon capture and utilization technologies within the chemical industry. Energy Environ. Sci. 12, 2253–2263. doi:10.1039/C9EE00914K
Thonemann, N., Schulte, A., and Maga, D. (2020). How to conduct prospective life cycle assessment for emerging technologies? A systematic review and methodological guidance. Sustainability 12, 1192. doi:10.3390/su12031192
Turgut, O., Bjerketvedt, V. S., Tomasgard, A., and Roussanaly, S. (2021). An integrated analysis of carbon capture and storage strategies for power and industry in Europe. J. Clean. Prod. 329, 129427. doi:10.1016/j.jclepro.2021.129427
Vandepaer, L., Panos, E., Bauer, C., and Amor, B. (2020). Energy system pathways with low environmental impacts and limited costs: minimizing climate change impacts produces environmental cobenefits and challenges in toxicity and metal depletion categories. Environ. Sci. Technol. 54, 5081–5092. doi:10.1021/acs.est.9b06484
Van Der Giesen, C., Cucurachi, S., Guinée, J., Kramer, G. J., and Tukker, A. (2020). A critical view on the current application of LCA for new technologies and recommendations for improved practice. J. Clean. Prod. 259, 120904. doi:10.1016/j.jclepro.2020.120904
Van Der Spek, M., Fout, T., Garcia, M., Kuncheekanna, V. N., Matuszewski, M., McCoy, S., et al. (2020). Uncertainty analysis in the techno-economic assessment of CO2 capture and storage technologies. Critical review and guidelines for use. Int. J. Greenh. Gas Control 100, 103113. doi:10.1016/j.ijggc.2020.103113
Van Der Spek, M., Ramirez, A., and Faaij, A. (2017). Challenges and uncertainties of ex ante techno-economic analysis of low TRL CO2 capture technology: lessons from a case study of an NGCC with exhaust gas recycle and electric swing adsorption. Appl. Energy 208, 920–934. doi:10.1016/j.apenergy.2017.09.058
Van Vuuren, D. P., Stehfest, E., Den Elzen, M. G. J., Kram, T., Van Vliet, J., Deetman, S., et al. (2011). RCP2.6: exploring the possibility to keep global mean temperature increase below 2°C. Clim. Change 109, 95–116. doi:10.1007/s10584-011-0152-3
Villares, M., Işıldar, A., Van Der Giesen, C., and Guinée, J. (2017). Does ex ante application enhance the usefulness of LCA? A case study on an emerging technology for metal recovery from e-waste. Int. J. Life Cycle Assess. 22, 1618–1633. doi:10.1007/s11367-017-1270-6
Voelker, S., Deutz, S., Burre, J., Bongartz, D., Omari, A., Lehrheuer, B., et al. (2022). Blend for all or pure for few? Well-to-wheel life cycle assessment of blending electricity-based OME 3–5 with fossil diesel. Sustain. Energy Fuels 6, 1959–1973. doi:10.1039/D1SE01758F
Voldsund, M., Gardarsdottir, S., De Lena, E., Pérez-Calvo, J.-F., Jamali, A., Berstad, D., et al. (2019). Comparison of technologies for CO2 capture from cement production—Part 1: technical evaluation. Energies 12, 559. doi:10.3390/en12030559
Volkart, K., Bauer, C., and Boulet, C. (2013). Life cycle assessment of carbon capture and storage in power generation and industry in Europe. Int. J. Greenh. Gas Control 16, 91–106. doi:10.1016/j.ijggc.2013.03.003
Volkart, K., Mutel, C. L., and Panos, E. (2018). Integrating life cycle assessment and energy system modelling: methodology and application to the world energy scenarios. Sustain. Prod. Consum. 16, 121–133. doi:10.1016/j.spc.2018.07.001
Volkart, K., Weidmann, N., Bauer, C., and Hirschberg, S. (2017). Multi-criteria decision analysis of energy system transformation pathways: a case study for Switzerland. Energy Policy 106, 155–168. doi:10.1016/j.enpol.2017.03.026
Von Der Assen, N., Jung, J., and Bardow, A. (2013). Life-cycle assessment of carbon dioxide capture and utilization: avoiding the pitfalls. Energy Environ. Sci. 6, 2721. doi:10.1039/c3ee41151f
von der Assen, N., Voll, P., Peters, M., and Bardow, A. (2014). Life cycle assessment of CO2 capture and utilization: a tutorial review. Chem. Soc. Rev. 43, 7982–7994. doi:10.1039/C3CS60373C
Way, R., Ives, M. C., Mealy, P., and Farmer, J. D. (2022). Empirically grounded technology forecasts and the energy transition. Joule 6, 2057–2082. doi:10.1016/j.joule.2022.08.009
Weyant, J. (2017). Some contributions of integrated assessment models of global climate change. Rev. Environ. Econ. Policy 11, 115–137. doi:10.1093/reep/rew018
Weyant, J., Davidson, O., Dowlatabadi, H., Edmonds, J., Grubb, M., Parson, E., et al. (1996). Integrated assessment of climate change: an overview and comparison of approaches and results. Cambridge, UK: Cambridge University Press, 367–396.
Young, J., McQueen, N., Charalambous, C., Foteinis, S., Hawrot, O., Ojeda, M., et al. (2023). The cost of direct air capture and storage can be reduced via strategic deployment but is unlikely to fall below stated cost targets. One Earth 6, 899–917. doi:10.1016/j.oneear.2023.06.004
Yu, S., Horing, J., Liu, Q., Dahowski, R., Davidson, C., Edmonds, J., et al. (2019). CCUS in China’s mitigation strategy: insights from integrated assessment modeling. Int. J. Greenh. Gas Control 84, 204–218. doi:10.1016/j.ijggc.2019.03.004
Zhou, Y., Searle, S., and Pavlenko, N. (2022). Current and future cost of e-kerosene in the United States and Europe. Available at: https://theicct.org/publication/fuels-us-eu-cost-ekerosene-mar22/.
Zimmermann, A. W., Langhorst, T., Moni, S., Schaidle, J. A., Bensebaa, F., and Bardow, A. (2022). Life-cycle and techno-economic assessment of early-stage carbon capture and utilization technologies—a discussion of current challenges and best practices. Front. Clim. 4, 841907. doi:10.3389/fclim.2022.841907
Zimmermann, A. W., Wunderlich, J., Müller, L., Buchner, G. A., Marxen, A., Michailos, S., et al. (2020). Techno-economic assessment guidelines for CO2 utilization. Front. Energy Res. 8, 5. doi:10.3389/fenrg.2020.00005
Glossary
Keywords: prospective life cycle assessment, integrated assessment models, prospective techno-economic assessment, carbon capture and utilisation, carbon capture and storage
Citation: Wang P, Robinson AJ and Papadokonstantakis S (2024) Prospective techno-economic and life cycle assessment: a review across established and emerging carbon capture, storage and utilization (CCS/CCU) technologies. Front. Energy Res. 12:1412770. doi: 10.3389/fenrg.2024.1412770
Received: 05 April 2024; Accepted: 03 July 2024;
Published: 26 July 2024.
Edited by:
Federica Raganati, National Research Council (CNR), ItalyReviewed by:
Hirdan Costa, University of São Paulo, BrazilHenrique A. Matos, University of Lisbon, Portugal
Copyright © 2024 Wang, Robinson and Papadokonstantakis. This is an open-access article distributed under the terms of the Creative Commons Attribution License (CC BY). The use, distribution or reproduction in other forums is permitted, provided the original author(s) and the copyright owner(s) are credited and that the original publication in this journal is cited, in accordance with accepted academic practice. No use, distribution or reproduction is permitted which does not comply with these terms.
*Correspondence: Pingping Wang, cGluZ3Bpbmcud2FuZ0B0dXdpZW4uYWMuYXQ=; Ada Josefina Robinson, YWRhLnJvYmluc29uQHR1d2llbi5hYy5hdA==