- 1Department of Electrical Engineering, University of Azad Jammu and Kashmir, Muzaffarabad, Pakistan
- 2College of Engineering and Information Technology, University of Dubai, Dubai, United Arab Emirates
- 3Department of Electrical and Electronic Engineering Science, University of Johannesburg, Johannesburg, South Africa
- 4Department of Electrical Engineering, Qatar University, Doha, Qatar
Introduction: The integration of electric vehicles (EVs) into the power network challenges the 1) grid capacity, 2) stability, and 3) management. This is due to the 1) increased peak demand, 2) infrastructure strain, and 3) intermittent charging patterns. Previous studies lack comprehensive integration of renewable energy and battery storage with EV charging.
Methods: To address these challenges, this study explores the effectiveness of incorporating renewable energy resources (RERs) and battery energy storage systems (BESS) alongside the traditional grid. The proposed study utilizes the HOMER Grid® and conducted a comprehensive analysis.
Results: The proposed study compares two grid integrated scenarios: 1) Case-1 (grid and photovoltaic (PV) systems), and 2) Case-2 (grid, PV systems, and BESS). Both these scenarios are compared against a Base case relying solely on grid power. The evaluation employed techno-economic analysis while focusing on 1) net present cost (NPC), 2) cost of energy, and 3) annualized savings. Additionally, the proposed study analyzed 4) seasonal variations in EV charging demand, 5) grid interactions, 6) PV production, and 7) the operation of BESS in both summer and winter. The comparative analysis reveals that the Base case incurs a net present cost (NPC) of $546,977 and a cost of energy (COE) of $0.354 per kWh. In contrast, Case-1, which integrates a 100 kW PV system, shows a significantly lower NPC of -$122,962 and a reduced COE of -$0.043 per kWh, with annualized savings of $61,492. Case-2, incorporating both the 100 kW PV system and a BESS with a capacity of 9.8 kWh, has a higher NPC of $309,667 but a COE of $0.112 per kWh and provides annual savings of $51,233 compared to the Base case.
Discussion: Seasonal analysis highlights that Case-2 achieves the lowest carbon emissions in summer, ranging from 2.0 to 2.5 tons, while Case-1 shows the lowest emissions in winter, ranging from 3.2 to 3.4 tons. This model 1) reduces operational costs, 2) minimizes carbon emissions, while 3) making it compelling for future energy systems in increasing EV adoption.
1 Introduction
1.1 The global shift towards electrical vehicles and their impact – motivation of the research
Presently, there is a remarkable global transition towards more ecologically responsible modes of transportation and EVs are leading this transformative movement (Patel et al., 2023; Zhang et al., 2023). This shift is largely driven by increased worldwide consciousness about the adverse effects of climate change (Mishra et al., 2021; Su et al., 2022). Moreover, it is amplified by solid and progressive governmental policies that increasingly favor renewable and clean energy sources (Babaki et al., 2019; Ziyat et al., 2023). The collection of concrete benefits offered by EVs also significantly contributes to their rising appeal. EVs present a notable leap forward in tackling greenhouse gas (GHG) emissions which are a principal cause of global warming (Liu et al., 2018; Chaurasiya and Singh, 2022). They operate on electric power while eliminating the requirement for fossil fuel combustion and subsequently lowering the emission of harmful pollutants into the environment (Mishra et al., 2023; Kumar et al., 2023). Furthermore, the prospect of fuel cost savings is an additional major benefit of EVs (Wang and Dorrell, 2014; Theodoropoulos et al., 2016). With the price of fossil fuels persistently subject to fluctuation and often trending upwards, the steady and generally lower cost of electricity serves as an economic motivator (Vithayasrichareon et al., 2015; Ebrahimi and Rastegar, 2023). The propagation of EVs has produced an upsurge in demand for charging. This imposes a considerable burden on the current power grid infrastructure (Lu et al., 2023; Haddoun et al., 2008). The existing grid which is based on fossil fuel-based generation faces challenges in accommodating the escalated power demands necessitated by the widespread adoption of EVs (Hu et al., 2011; Iqbal et al., 2022). It’s vital to quickly find and adopt alternative energy solutions to reduce grid strain and integrate EV charging effectively (Ullah et al., 2023; Rehman et al., 2023a).
1.2 The potential of RERs for EVs charging and challenges – focus of the research
The RERs comprises of 1) solar, 2) wind, 3) hydro, and 4) other resources. It presents a promising alternative to the longstanding dependency on fossil fuels (Haegel and Kurtz, 2021; Du et al., 2018). Specifically, in the context of EV charging infrastructure, these RERs possess the potential to generate electricity in a 1) sustainable, 2) economically efficient, and 3) environmentally beneficial manner (Huang et al., 2024; Lenka et al., 2024; Mouhy-Ud-Din et al., 2024; Varone et al., 2024; Yin and Ji, 2024; Du et al., 2019; Celik et al., 2018). RERs include various advantages that render them an appealing alternative to conventional power sources. One of the most significant benefits is their infinite nature. This offers an unlimited supply of energy that contrasts with the finite reserves of fossil fuels (Elavarasan et al., 2020; Ogunrinde et al., 2018). Moreover, RERs do not emit harmful pollutants during operation. Economically, while initial setup costs for RERs are high, their long-term operational and maintenance expenses are significantly lower than fossil fuels (Akhtar et al., 2021; Zsiborács et al., 2023). These costs are further offset by their durability and the relative stability of renewable energy prices in comparison to the often-volatile fossil fuel markets (Rehman et al., 2020; Rehman et al., 2022).
Despite the numerous advantages, RERs come with their own set of challenges which are 1) their intermittent nature, 2) the need for large-scale storage, and 3) issues related to grid connectivity require strategic planning and management (Shafiq et al., 2022; Shafiq et al., 2023). The numerous advantages play a major role towards 1) effective EV load management, 2) efficient charging and discharging of battery energy storage systems (BESS), and 3) optimal use of RERs. EV load management refers to managing the time and rate at which EVs are charged (Rehman et al., 2023b; Gogoi et al., 2024). This aligns the charging process with the availability of renewable energy, thus ensuring optimal utilization of RERs. Energy storage systems are a crucial component of the RERs-EV ecosystem (Abubakr et al., 2022; Mohamed et al., 2020). Their efficient charging and discharging play a significant role in energy management. This helps to balance supply and demand, especially considering the intermittent nature of many RERs which is the focus of this research.
1.3 Literature review
Numerous research studies have been presented in scholarly literature about EVs and RERs (Table 1). The study (Jaganath et al., 2023) investigates the techno-economic aspects of optimal energy configurations for EV charging station infrastructures. It highlights the environmental benefits of 100% renewable generation and provides insights into component degradation costs, battery state of charge (SoC), and cybersecurity considerations in EVs. The research (Attaianese et al., 2023) focuses on ultra-fast charging stations for EVs. It proposes an online scheduling algorithm that considers power and energy constraints related to the infrastructure and EVs. The study focuses on efficiency, power loss estimation, and the influence of battery SoC on EV charging rates. Sridharan et al. (2023) discusses an efficient hybrid technique to boost energy resilience in buildings against natural disasters. Paper utilizes an innovative combination of chicken search optimization and the spike neural network learning algorithm. It considers buildings with shared EV parking stations and employing a variety of power management strategies. The study seeks to minimize energy costs and increase resilience without necessitating additional components for building energy management. The study (Lamedica et al., 2023) proposes a novel methodology, employing custom-developed MATLAB software, to optimize the power unit architecture of ultra-fast charging stations for EVs. The study focuses on enhancing system efficiency and energy savings. The validation of the chosen configuration, based on efficiency-power curves of available power modules, is conducted through Simulink software.
Liu et al. (2023a) investigates the growing EV industry in China and highlighting a prevalent demand-supply gap in EV charging stations. By employing a grey correlation and long-and-short-term memory-based prediction model, the paper forecasts a significant increase in EV numbers by 2030. The article (Liu Y. et al., 2023) introduces a novel EV classification approach within a smart grid context to effectively manage energy transactions and minimize costs. It proposes charging price schemes and use chance-constrained and probabilistic methods to handle PV generation uncertainty. The research also showcases significant cost savings for green charging stations and vehicle-to-grid EVs. The research study (Rochd et al., 2023) offers an in-depth analysis of EVs supply equipment (EVSE) usage. The study aims to understand real-world urban charging patterns by utilizing a 2-year historical dataset from a public EVSE in Rabat. The extensive evaluation of over 2,800 events not only reveals average energy deliveries and charging durations but also leads to recommendations for various stakeholders. The research (Li et al., 2022) explores the technical and economic feasibility of hybrid solar PV and WT systems for green EV charging stations in five Chinese locations using HOMER Pro software. The findings favor hybrid PV/WT/battery solutions with varying degrees of economy across the locations. Furthermore, the study illuminates the inverse relationship between load or EV number and station reliability. It underscores the need for balancing EV numbers for optimal economic and reliability outcomes.
The study (Beyazıt and Taşcıkaraoğlu, 2023) proposes a novel energy management strategy for mobile charging to alleviate challenges in fixed charging station (FXCS) infrastructure for EVs. The optimization algorithm presented minimizes total operational costs for microgrid control systems (MCSs). It considers battery degradation costs and ensures fairness with FXCS using time-of-use tariff prices. The paper addresses both system operator and EV user perspectives. The research study (Qiao et al., 2023) introduces a two-phase approach to tackle the fast-charging station location problem in urban areas. It combines data processing with model optimization to minimize total social cost. The study utilizes a spatiotemporal mathematical program with equilibrium constraints. It also used a modified genetic algorithm to capture the charging choice behavior of EVs. The article (Huang et al., 2023) explores the potential impact of rapidly increasing EVs on power grids. The study proposes a combined scheduling of pricing and power management for EV charging stations. It applies a bilevel optimization framework to the Markov decision process model. The study illustrates the operational benefits and enhancements of this method through a comparative analysis. The article also highlights its potential to mitigate negative impacts on EV charging stations. The research (Wang et al., 2023) presents an optimization model for planning EV charging facilities in new urban areas. The study explores characteristics of EV charging demand to minimize costs while meeting supplier and driver constraints. The research implements a case study in Yizhuang, Beijing, showcases the model effectiveness in optimizing charging facilities according to traffic conditions. It also highlights the link between charging demand, population density, and regional functionality.
1.4 Main contribution of this work
This research introduces a optimization strategy for integrating EVs, PV systems, and BESS within power distribution networks. Our study offers a detailed techno-economic analysis, balancing power distribution among grid, PV, and BESS to reduce costs. It optimizes BESS operations based on real-time demand and supply, and assesses environmental impacts to minimize emissions from EV charging. Additionally, it presents an innovative EV load management strategy that uses peak PV production to cut energy costs and lessen grid reliance. These contributions set a new standard for achieving both economic efficiency and environmental sustainability in energy systems. Figure 1 shows a graphical abstract illustrating the comprehensive analysis of EV charging infrastructure.
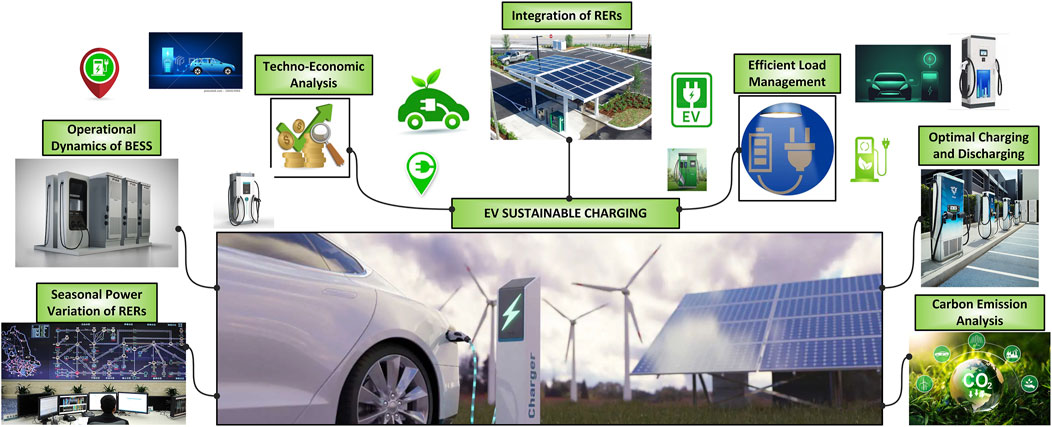
Figure 1. Grid-integrated solutions for sustainable EV charging with RERs and BESS. RER and BESS are the acronyms of renewable energy resources and battery energy storage system, respectively.
2 Problem formulation and methodology
This section presents a comprehensive problem formulation and methodology aimed to address multiple critical aspects: 1) techno-economic factors, 2) environmental impact, 3) optimal power-sharing, 4) effective use of BESS, and 4) optimize EV load on charging station.
2.1 Techno-economic analysis
The techno-economic analysis involves determining the financial feasibility of the proposed system by considering various costs over its lifetime. Initially, the system needs to calculate the capital cost, which represents the initial expenditure for purchasing and installing the system’s components. The capital cost is given by Equation 1.
where
Once the system is in operation, the system has annual O&M costs which include expenditures on system operation, maintenance, and repairs. The O&M cost in year t is represented with Equation 2. Where
Components of the system may need to be replaced over the project’s lifetime. The costs associated with this are considered replacement costs, denoted as
The proposed study then computes the NPC which represents the present value of all the costs (capital cost, O&M costs, and replacement costs) incurred over the project’s lifetime. The NPC is given by Equation 3.
where, r is the discount rate, and n is the project life.
Finally, it calculates the COE which represents the cost per unit of electricity produced by the system. The COE is defined through Equation 4 as.
2.2 Optimal power sharing between grid, PV and BESS
The optimal power-sharing strategy seeks to distribute the power demand among the grid, PV, and BESS in a way that minimizes the overall cost. This strategy must adhere to a power balance constraint ensuring that the total power supplied equals the power demand. Moreover, the power supplied by each source should not exceed its available capacity. The power balance constraint for each EV is represented by Equation 5.
The power limit constraints for the grid, PV, and BESS are represented through Equation 6.
2.3 Optimal charging/discharging of BESS
The optimization of BESS charging and discharging seeks to control the amount of power flowing into and out of the BESS. This is based on the system demand and supply. This needs consideration of the BESS’s energy capacity and efficiency. The energy balance constraint of the BESS is represented by Equation 7.
Here,
2.4 Environmental analysis
The main focus of environmental analysis is to evaluate and minimize the emission of pollutant gases from the electricity used to charge EVs. For each unit of power drawn from the grid, a specific amount of gas emissions is produced. These emissions have detrimental effects on the environment. This contributes to problems like 1) air pollution, and 2) climate change. Therefore, it is important to consider these emissions in the optimization model. The environmental analysis involves quantifying the pollutant gas emissions from the system and working towards minimizing them. The total emissions are given by Equation 9.
Here,
2.5 Efficient load management of EVs
Optimal scheduling of EVs is an essential part of proposed research. It aims to reduce energy costs and increase the consumption of cleaner energy from PV systems. The objective is to determine the best charging schedule for each EV to minimize the overall cost while ensuring that all EVs’ energy demands are met. In an ideal scenario, EVs should be charged when the PV system is producing maximum power. This not only reduces the dependency on the grid but also decreases the cost associated with charging the EVs.
The
Objective Function: Minimize the total cost of charging all EVs as given by Equation 10.
Here,
Constraints: The total power used to charge each EV must equal the EV’s power demand (See Equation 11):
The power drawn from the PV system for charging EVs should not exceed the PV system’s available power (See Equation 12):
These optimization problems can be solved using appropriate optimization software, like HOMER Grid®, to find the optimal charging schedule for EVs. The schedule should prioritize the use of power from the PV system to minimize energy cost.
2.6 Proposed model for the integration of RERs and BESS for EVs charging
The research presents a comprehensive model designed to address the integration of EV charging stations. The model evaluates two main scenarios:
• Case 1: Integrates a 100 kW PV system with the grid.
• Case 2: Combines a 100 kW PV system, a BESS with a capacity of 9.8 kWh (LG Chem), and the grid.
• Base Case: Grid only.
Both cases are compared with a base case (grid only) to demonstrate potential improvements. The network topology includes the PV system connected to the grid and, in Case 2, the addition of BESS to enhance energy management. The topology of the network involves the following parameters:
• PV System: 100 kW capacity
• BESS: LG Chem 9.8 kWh
• Grid: Standard power grid integration
The model is flexible and adaptive, using HOMER Grid® software for simulations and optimization. Figure 2 illustrates the network topology for both scenarios: Case 1 shows the PV system connected directly to the grid, while Case 2 includes the additional BESS. The integration of the BESS in Case 2 supports improved energy management and optimized EV charging practices.
2.7 Flowchart of proposed study
In the methodology of the research, the process begins with the input parameters stage. Essential data like EV numbers, charging needs, power sources (grid, PV), BESS capacity, costs, and emission factors are collected. The scenario definition stage then outlines two cases: one combining PV and grid power, and the other adding BESS to this mix. Next, in the techno-economic analysis stage, various cost factors like capital, O&M, and replacement costs are calculated for each scenario, along with the NPC and COE. This is followed by the optimal power sharing stage. In that power distribution among the grid, PV, and BESS is optimized for efficiency and cost-effectiveness. The optimal BESS management stage then adjusts the charging and discharging schedules of the BESS to support the grid and PV system in meeting EV power demands. In the environmental analysis stage, total system emissions are calculated, aiming to minimize the environmental impact.
The optimal EV load management stage devises charging schedules for EVs, focusing on maximizing renewable energy use. Finally, the result analysis and comparison stage evaluate the outcomes against a base case to determine the most cost-effective and environmentally friendly strategy. This methodology is visually summarized in Figure 3 of the study.
3 Results and discussion
In the results and discussion section, two test cases have been utilized for the analysis. Case – 1 comprises of combining grid and PV systems whereas Case – 2 has the integrating grid, PV systems, and BESS.
3.1 Techno-economic analysis
In this techno-economic study, two alternative scenarios, Case-1 (combining grid and PV systems) and Case-2 (integrating grid, PV systems, and BESS) are evaluated against a traditional Base case that relies solely on grid power for EV charging. Financial analyses focus on NPC, COE, and annualized savings. Findings reveal that the Base case incurs an NPC of $546,977. In contrast, Case-1 exhibits a significant economic advantage with an NPC at -$122,962, indicating a net financial gain. Case-2, while showing an increased NPC of $309,667 compared to Case-1, still represents a substantial improvement over the Base case. The COE also follows a similar pattern: the Base case stands at $0.354/kWh, Case-1 dramatically lowers this to -$0.043/kWh, and Case-2 maintains a competitive rate at $0.112/kWh. Additionally, annualized savings are notable in both cases, with Case-1 achieving $61,492 and Case-2 securing $51,233 in savings per year. These results highlight the financial efficacy of incorporating RERs and BESS into existing grid structures for EV charging, with Case-1 offering the most significant economic benefits. For a detailed visual representation of these financial comparisons, refer to Figures 4, 5.
3.2 Seasonal analysis of EVs charging, grid interactions and PV production
The winter and summer details in Figures 6, 7 reveal distinct seasonal patterns in EV charging, grid transactions, and PV production in Case-1. In a day of January, the EV load peaks at 24.17 kW around 11 a.m., primarily met by grid purchases (21.95 kW). PV production contributes modestly, peaking at 12.15 kW at 10 a.m., enabling a small grid sale of 0.71 kW. Notably, during evening hours (7–9 p.m.), the EV load again increases, reaching 17.5%, with no PV support. In contrast, summer exhibits robust PV production, significantly influencing grid transactions. PV output peaks at 69.22 kW at 2 p.m., while the highest EV load, 22.5%, occurs at 4 p.m. and 6 p.m. Notably, during morning hours (6–9 a.m.), grid sales are substantial due to high PV output and low EV load, reaching 24.59 kW at 9 a.m. In the evening (6–7 p.m.), grid purchase is required (3.5 kW at 6 p.m.) as PV production drops and the EV load remains significant (16.67% at 6 p.m.). These observations underscore the variability in energy dynamics across seasons, with PV production playing a critical role in managing grid interactions and EV charging demands.
As depicted in Figures 8, 9, the battery’s SoC remains steady at 10.2% during the early hours, reaching 100% by noon, maintaining this level until evening in Case-2. Notably, the peak PV production occurs at noon with 81.66 kW. During this period, grid sales are evident, and there’s a grid purchase at 11 p.m., indicating active management. In contrast, the summer data displays a dynamic pattern in Figure 9. The battery charge starts at 10.2%, increases steadily, and experiences a grid purchase at 6 a.m., coinciding with the onset of significant PV production. A notable drop in battery state to 11.7% at noon aligns with high grid sales and substantial PV output. The battery’s state fluctuates in the afternoon, reflecting dynamic interactions between PV generation, grid transactions, and battery usage. Evening grid sales are prominent, and the battery stabilizes at 11.7% overnight. These figures illustrate the intricate relationship between PV production, grid interactions, and battery management in both winter and summer scenarios.
Figures 10, 11 provide insights into the operation of a BESS across different seasons, specifically summer and winter. During summer, the BESS primarily discharges power in the morning and evening hours (7 a.m., and between 12 p.m. and 9 p.m.), with the maximum discharge occurring at 3 p.m. (14.11 kW) and 4 p.m. (11.35 kW). The battery’s SoC is mostly maintained at or near 100% during the early hours (1 a.m.–6 a.m.) and starts varying from 10 a.m. onwards, dropping to a minimum of 72.13% by midnight. This suggests efficient energy management, balancing energy storage and usage throughout the day.
In contrast, the winter data show a different pattern. The battery starts at a lower SoC (42.85% at 1 a.m.), indicating higher energy usage or lower energy storage capacity due to seasonal changes. Notable charging activities occur from 8 a.m. to 2 p.m., peaking at 1 p.m. (61.81 kW) and 2 p.m. (56.90 kW). The battery discharges in the evening, particularly from 6 p.m. to 10 p.m., with the highest discharge at 7 p.m. (32.39 kW). The SoC decreases throughout the evening, reaching 16.88% by midnight. Overall, these Fig. highlight the dynamic operation of BESS in response to seasonal variations, with different charging and discharging patterns observed in summer and winter. This reflects the system’s adaptation to changing energy demands and availability of renewable energy sources like solar power, which is likely more abundant in summer.
3.3 Alignment of EVs load distribution with PV production
The presented Figures 12, 13 illustrate a strategic alignment between EV load distribution and PV production, particularly in summer. During this season, EV load commences at 1 a.m. and peaks at 11 a.m. and 6 p.m., closely paralleling the onset and zenith of PV production, which starts at 6 a.m. and peaks at 11 a.m. This synchronization suggests a harmonious integration, potentially minimizing costs by leveraging renewable energy during peak EV demand periods. In contrast, the winter data exhibits a less synchronized pattern, emphasizing the need for further exploration of storage or load management strategies to optimize the cost-effectiveness of the integrated system.
3.4 Monthly carbon emission analysis
Figure 14 depict the monthly carbon emissions (in tons) for three cases: Base Case (solely grid-powered), Case-1 (utilizing PV and grid), and Case-2 (employing BESS, PV, and grid). In the summer months (June, July, and August), Case-2 consistently exhibits the lowest carbon emissions, ranging from 2.0 to 2.5 tons, indicating the effectiveness of incorporating Battery Energy Storage Systems (BESS) alongside PV and grid sources in reducing carbon output during peak demand periods. Conversely, in the winter months (December, January, and February), Case-1 consistently demonstrates the lowest emissions, ranging from 3.2 to 3.4 tons, showcasing the efficacy of the PV and grid combination in minimizing carbon impact during low-sunlight periods. The Base Case consistently shows higher emissions across all months, emphasizing the environmental benefits of integrating renewable energy sources and BESS for overall carbon reduction throughout the year.
3.5 Comparative analysis of studies on EV charging and renewable energy integration
Table 2 presents a comprehensive overview of six research studies of EV charging and renewable energy integration. Each study has distinct objectives ranging from optimizing EV charging loss prediction to managing energy fluxes in solar-powered parking lots. These studies utilize a variety of methods, such as advanced algorithms, software platforms, and control systems. This leads to significant findings and innovations like improved prediction precision for EV charging loads, balanced power distribution among various energy sources, and enhanced grid power quality. Notably, the innovations include self-adaptive hyper-parameter adjustment, multi-step charging methods, and smart energy management techniques. However, the proposed study stands out as the most comprehensive and potentially impactful. It aims to explore the integration of EVs with renewable energy and battery storage for grid management, employing tools like HOMER Grid® and techno-economic analysis. This study promises not only to demonstrate the economical superiority of integrating RERs and BESS but also to reduce operational costs significantly. Its comprehensive seasonal analysis and alignment of EV load with PV production represent a pioneering approach. It offers a cost-efficient and environmentally sustainable solution for the future of EV charging and renewable energy utilization.
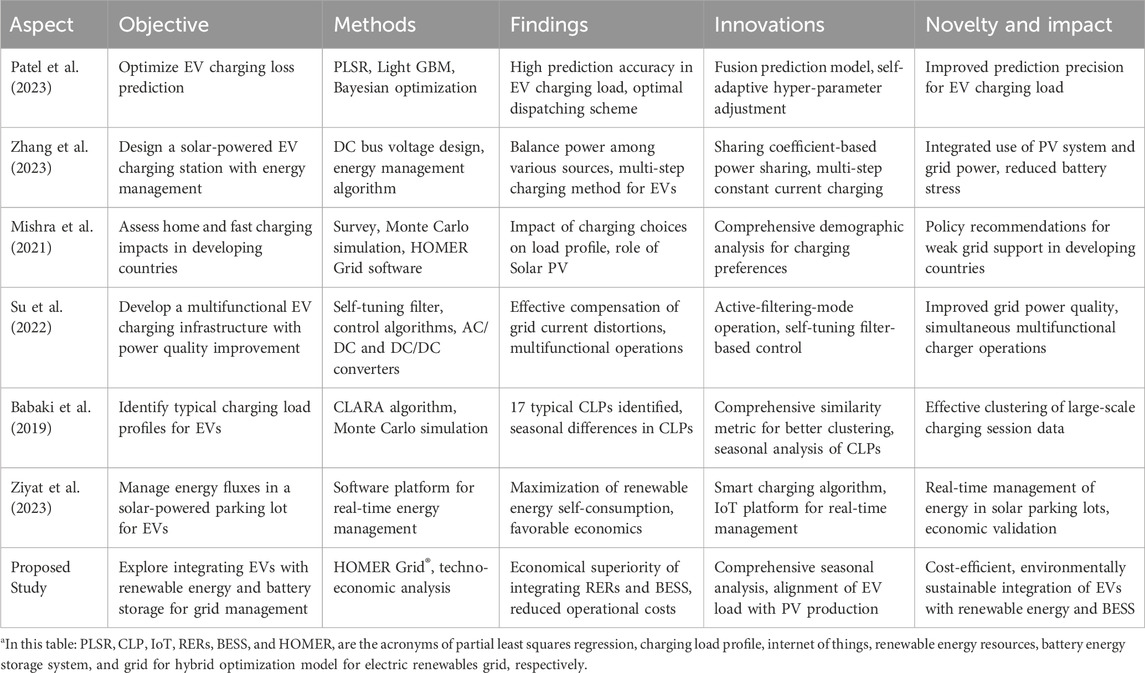
Table 2. Comparative overview of EV charging innovations: from predictive models and solar-powered stations to grid management and renewable energy integration.a
4 Conclusion
This study analyzed the integration of renewable energy and battery storage in EV charging infrastructure across three scenarios: a grid-only base case, a grid plus PV system (Case 1), and a grid, PV, and BESS combination (Case 2). The techno-economic analysis revealed that Case 1 was the most cost-effective, with a net present cost (NPC) of -$122,962, a cost of energy (COE) of -$0.043/kWh, and annual savings of $61,492. Case 2 also outperformed the base case, showing an NPC of $309,667, a COE of $0.112/kWh, and annual savings of $51,233. Seasonal analyses indicated that Case 2 had the lowest carbon emissions in summer (2.0–2.5 tons), while Case 1 was more efficient in winter (3.2–3.4 tons). The study underscores the economic and environmental benefits of integrating renewable energy, especially PV systems, with or without BESS, into EV charging infrastructure, offering a sustainable solution for EV charging needs and reducing carbon emissions.
Data availability statement
The raw data supporting the conclusions of this article will be made available by the authors, without undue reservation.
Author contributions
AR: Conceptualization, Data curation, Methodology, Resources, Software, Validation, Writing–review and editing. HK: Conceptualization, Investigation, Project administration, Software, Supervision, Validation, Writing–review and editing. SM: Data curation, Funding acquisition, Investigation, Methodology, Resources, Supervision, Writing–review and editing.
Funding
The authors declare that no financial support was received for the research, authorship, and/or publication of this article.
Conflict of interest
The authors declare that the research was conducted in the absence of any commercial or financial relationships that could be construed as a potential conflict of interest.
The authors declared that they were an editorial board member of Frontiers, at the time of submission. This had no impact on the peer review process and the final decision.
Publisher’s note
All claims expressed in this article are solely those of the authors and do not necessarily represent those of their affiliated organizations, or those of the publisher, the editors and the reviewers. Any product that may be evaluated in this article, or claim that may be made by its manufacturer, is not guaranteed or endorsed by the publisher.
References
Abubakr, H., Vasquez, J. C., Mahmoud, K., Darwish, M. M. F., and Guerrero, J. M. (2022). Comprehensive review on renewable energy sources in Egypt—current status, grid codes and future vision. IEEE Access 10, 4081–4101. doi:10.1109/ACCESS.2022.3140385
Akhtar, I., Kirmani, S., Jameel, M., and Alam, F. (2021). Feasibility analysis of solar technology implementation in restructured power sector with reduced carbon footprints. IEEE Access 9, 30306–30320. doi:10.1109/ACCESS.2021.3059297
Attaianese, C., Di Pasquale, A., Franzese, P., Iannuzzi, D., Pagano, M., and Ribera, M. (2023). A model based EVs charging scheduling for a multi-slot ultra-fast charging station. Electr. Power Syst. Res. 216, 109009. doi:10.1016/j.epsr.2022.109009
Babaki, A., Vaez-Zadeh, S., and Zakerian, A. (2019). Performance optimization of dynamic wireless EV charger under varying driving conditions without resonant information. IEEE Trans. Veh. Technol. 68 (11), 10429–10438. doi:10.1109/TVT.2019.2944153
Beyazıt, M. A., and Taşcıkaraoğlu, A. (2023). Electric vehicle charging through mobile charging station deployment in coupled distribution and transportation networks. Sustain. Energy Grids Netw. 35, 101102. doi:10.1016/j.segan.2023.101102
Celik, I., Philips, A. B., Song, Z., Yan, Y., Ellingson, R. J., Heben, M. J., et al. (2018). Energy payback time (EPBT) and energy return on energy invested (EROI) of perovskite tandem photovoltaic solar cells. IEEE J. Photovoltaics 8 (1), 305–309. doi:10.1109/JPHOTOV.2017.2768961
Chaurasiya, S., and Singh, B. (2022). “An adaptive EV charger based reconfigurable bidirectional isolated DC-DC converter with wide voltage range of operation,” in 2022 IEEE Transportation Electrification Conference and Expo (ITEC), China, June 2022, 1301–1306. doi:10.1109/ITEC53557.2022.9813778
Du, E., Zhang, N., Hodge, B. M., Wang, Q., Kang, C., Kroposki, B., et al. (2018). The role of concentrating solar power toward high renewable energy penetrated power systems. IEEE Trans. Power Syst. 33 (6), 6630–6641. doi:10.1109/TPWRS.2018.2834461
Du, E., Zhang, N., Hodge, B. M., Wang, Q., Lu, Z., Kang, C., et al. (2019). Operation of a high renewable penetrated power system with CSP plants: a look-ahead stochastic unit commitment model. IEEE Trans. Power Syst. 34 (1), 140–151. doi:10.1109/TPWRS.2018.2866486
Ebrahimi, M., and Rastegar, M. (2023). Data-driven charging load estimation of behind-the-meter V2G-capable EVs. IEEE Trans. Industry Appl. 59 (6), 7949–7956. doi:10.1109/TIA.2020.3012111
Elavarasan, R. M., Shafiullah, G. M., Padmanaban, S., Kumar, N. M., Annam, A., Vetrichelvan, A. M., et al. (2020). A comprehensive review on renewable energy development, challenges, and policies of leading Indian states with an international perspective. IEEE Access 8, 74432–74457. doi:10.1109/ACCESS.2020.2988011
Gogoi, D., Bharatee, A., and Ray, P. K. (2024). Implementation of battery storage system in a solar PV-based EV charging station. Electr. Power Syst. Res. 229, 110113. doi:10.1016/j.epsr.2024.110113
Haddoun, A., Benbouzid, M. E. H., Diallo, D., Abdessemed, R., Ghouili, J., and Srairi, K. (2008). Modeling, analysis, and neural network control of an EV electrical differential. IEEE Trans. Industrial Electron. 55 (6), 2286–2294. doi:10.1109/TIE.2008.918392
Haegel, N. M., and Kurtz, S. R. (2021). Global progress toward renewable electricity: tracking the role of solar. IEEE J. Photovoltaics 11 (6), 1335–1342. doi:10.1109/JPHOTOV.2021.3104149
Hu, X., Lin, S., Stanton, S., and Lian, W. (2011). A foster network thermal model for HEV/EV battery modeling. IEEE Trans. Industry Appl. 47 (4), 1692–1699. doi:10.1109/TIA.2011.2155012
Huang, Q., Yang, L., Zhou, C., Luo, L., and Wang, P. (2023). Pricing and energy management of EV charging station with distributed renewable energy and storage. Energy Rep. 9, 289–295. doi:10.1016/j.egyr.2022.10.418
Huang, W., Wang, J., Wang, J., Zeng, H., Zhou, M., and Cao, J. (2024). EV charging load profile identification and seasonal difference analysis via charging sessions data of charging stations. Energy 288, 129771. doi:10.1016/j.energy.2023.129771
Iqbal, S., Jan, M. U., Rehman, A. U., Shafiq, A., Rehman, H. U., Aurangzeb, M., et al. (2022). Feasibility study and deployment of solar photovoltaic system to enhance energy economics of King Abdullah campus, University of Azad Jammu and Kashmir Muzaffarabad, AJK Pakistan. IEEE Access 10, 5440–5455. doi:10.1109/ACCESS.2022.3140723
Jaganath, M. M., Ray, S., and Choudhury, N. B. D. (2023). Eco-friendly microgrid carport charging station for electric vehicles (EVs). e-Prime - Adv. Electr. Eng. Electron. Energy 5, 100196. doi:10.1016/j.prime.2023.100196
Kumar, N., Singh, B., and Panigrahi, B. K. (2023). Voltage sensorless based model predictive control with battery management system: for solar PV powered on-board EV charging. IEEE Trans. Transp. Electrification 9 (2), 2583–2592. doi:10.1109/TTE.2022.3213253
Lamedica, R., Ruvio, A., Falessi, P., Maccioni, M., Sammartino, E., and Palumbo, M. (2023). Power unit architecture in a high-power charging system for EVs. Int. J. Electr. Power and Energy Syst. 149, 109052. doi:10.1016/j.ijepes.2023.109052
Lenka, R. K., Panda, A. K., and Senapati, L. (2024). Grid integrated multifunctional EV charging infrastructure with improved power quality. J. Energy Storage 76, 109637. doi:10.1016/j.est.2023.109637
Li, C., Shan, Y., Zhang, L., Zhang, L., and Fu, R. (2022). Techno-economic evaluation of electric vehicle charging stations based on hybrid renewable energy in China. Energy Strategy Rev. 41, 100850. doi:10.1016/j.esr.2022.100850
Liu, B., Song, J., Wang, Q., Xu, Y., and Liu, Y. (2023a). Charging station forecasting and scenario analysis in China. Transp. Policy 139, 87–98. doi:10.1016/j.tranpol.2023.05.012
Liu, H., Qi, J., Wang, J., Li, P., Li, C., and Wei, H. (2018). EV dispatch control for supplementary frequency regulation considering the expectation of EV owners. IEEE Trans. Smart Grid 9 (4), 3763–3772. doi:10.1109/TSG.2016.2641481
Liu, Y., Jian, L., and Jia, Y. (2023b). Energy management of green charging station integrated with photovoltaics and energy storage system based on electric vehicles classification. Energy Rep. 9, 1961–1973. doi:10.1016/j.egyr.2023.04.099
Lu, S., Han, B., Xue, F., Jiang, L., and Qian, K. (2023). Multi-objective optimization of EV charging and discharging for different stakeholders. CSEE J. Power Energy Syst. 9 (6), 2301–2308. doi:10.17775/CSEEJPES.2020.02300
Mishra, D., Singh, B., and Panigrahi, B. K. (2023). Bi-directional EV charging with robust power controlled adaptive phase-shift algorithm. IEEE Trans. Veh. Technol. 72 (12), 15491–15501. doi:10.1109/TVT.2023.3290116
Mishra, D., Singh, B., and Panigrahi, B. K. (2021). Adaptive current control for a bidirectional interleaved EV charger with disturbance rejection. IEEE Trans. Industry Appl. 57 (4), 4080–4090. doi:10.1109/TIA.2021.3074612
Mohamed, S. H., Halim, M. B. A., Elgorashi, T. E. H., and Elmirghani, J. M. H. (2020). Fog-assisted caching employing solar renewable energy and energy storage devices for video on demand services. IEEE Access 8, 115754–115766. doi:10.1109/ACCESS.2020.3004314
Mouhy-Ud-Din, M. A., Azeem, F., Memon, Z., and Arshad, J. (2024). Comparative techno-economic assessment of electric vehicle charging preferences: a quantitative study for sustainable EV policy in Pakistan. Energy Sustain. Dev. 78, 101355. doi:10.1016/j.esd.2023.101355
Ogunrinde, O., Shittu, E., and Dhanda, K. K. (2018). Investing in renewable energy: reconciling regional policy with renewable energy growth. IEEE Eng. Manag. Rev. 46 (4), 103–111. doi:10.1109/EMR.2018.2880445
Patel, N., Lopes, L. A. C., Rathore, A., and Khadkikar, V. (2023). A soft-switched single-stage single-phase PFC converter for bidirectional plug-in EV charger. IEEE Trans. Industry Appl. 59 (4), 1–13. doi:10.1109/TIA.2023.3270387
Qiao, D., Wang, G., and Xu, M. (2023). Fast-charging station location problem: a two-phase approach with mathematical program with equilibrium constraints considering charging choice behaviour. Sustain. Cities Soc. 96, 104678. doi:10.1016/j.scs.2023.104678
Rehman, A. U., Ali, M., Iqbal, S., Habib, S., Shafiq, A., Elbarbary, Z. M. S., et al. (2023b). Transition towards a sustainable power system: MA-DA&DC framework based voltage control in high PV penetration networks. Energy Rep. 9, 5922–5936. doi:10.1016/j.egyr.2023.05.035
Rehman, A. U., Ali, M., Iqbal, S., Shafiq, A., Ullah, N., and Otaibi, S. A. (2022). Artificial intelligence-based control and coordination of multiple PV inverters for reactive power/voltage control of power distribution networks. Energies 15 (17), 6297. Art. no. 17. doi:10.3390/en15176297
Rehman, A. U., Ullah, Z., Shafiq, A., Hasanien, H. M., Luo, P., and Badshah, F. (2023a). Load management, energy economics, and environmental protection nexus considering PV-based EV charging stations. Energy 281, 128332. doi:10.1016/j.energy.2023.128332
Rehman, W. U., Bhatti, A. R., Awan, A. B., Sajjad, I. A., Khan, A. A., Bo, R., et al. (2020). The penetration of renewable and sustainable energy in Asia: a state-of-the-art review on net-metering. IEEE Access 8, 170364–170388. doi:10.1109/ACCESS.2020.3022738
Rochd, A., Laamim, M., Benazzouz, A., Kissaoui, M., Raihani, A., and Sun, H. (2023). Public charging infrastructure for EVs: a comprehensive analysis of charging patterns and real-world insights—case study of Rabat City, Morocco. Energy Rep. 9, 216–234. doi:10.1016/j.egyr.2023.05.259
Shafiq, A., Iqbal, S., Habib, S., Rehman, A. U., Selim, A., et al. (2022). Solar PV-based electric vehicle charging station for security bikes: a techno-economic and environmental analysis. Sustainability 14, 13767. doi:10.3390/su142113767
Shafiq, A., Iqbal, S., Rehman, A. U., S Elbarbary, Z. M., Kotb, H., Selim, A., et al. (2023). Integration of solar based charging station in power distribution network and charging scheduling of EVs. Front. Energy Res. 11. doi:10.3389/fenrg.2023.1086793
Sridharan, S., Sivakumar, S., Shanmugasundaram, N., Swapna, S., and Vasan Prabhu, V. (2023). A hybrid approach-based energy management for building resilience against power outage by shared parking station for EVs. Renew. Energy 216, 119002. doi:10.1016/j.renene.2023.119002
Su, Y.-C., Li, H.-M., Chen, P.-L., and Cheng, P.-T. (2022). Integration of PV panels and EV chargers on the modular multilevel converter-based SST. IEEE Trans. Industry Appl. 58 (5), 6428–6437. doi:10.1109/TIA.2022.3189969
Theodoropoulos, T. V., Damousis, I. G., and Amditis, A. J. (2016). Demand-side management ICT for dynamic wireless EV charging. IEEE Trans. Industrial Electron. 63 (10), 6623–6630. doi:10.1109/TIE.2016.2570198
Ullah, Z., Wang, S., Wu, G., Hasanien, H. M., Rehman, A. U., Turky, R. A., et al. (2023). Optimal scheduling and techno-economic analysis of electric vehicles by implementing solar-based grid-tied charging station. Energy 267, 126560. doi:10.1016/j.energy.2022.126560
Varone, A., Heilmann, Z., Porruvecchio, G., and Romanino, A. (2024). Solar parking lot management: an IoT platform for smart charging EV fleets, using real-time data and production forecasts. Renew. Sustain. Energy Rev. 189, 113845. doi:10.1016/j.rser.2023.113845
Vithayasrichareon, P., Mills, G., and MacGill, I. F. (2015). Impact of electric vehicles and solar PV on future generation portfolio investment. IEEE Trans. Sustain. Energy 6 (3), 899–908. doi:10.1109/TSTE.2015.2418338
Wang, S., and Dorrell, D. G. (2014). Loss analysis of circular wireless EV charging coupler. IEEE Trans. Magnetics 50 (11), 1–4. doi:10.1109/TMAG.2014.2334895
Wang, Y., Zhao, Y., Gan, S., Li, K., Chen, Y., and Lai, J. (2023). Optimization of charging stations integrated with dynamic transportation systems in metropolises. Transp. Res. Part D Transp. Environ. 119, 103726. doi:10.1016/j.trd.2023.103726
Yin, W., and Ji, J. (2024). Research on EV charging load forecasting and orderly charging scheduling based on model fusion. Energy 290, 130126. doi:10.1016/j.energy.2023.130126
Zhang, J., Che, L., and Shahidehpour, M. (2023). Distributed training and distributed execution-based stackelberg multi-agent reinforcement learning for EV charging scheduling. IEEE Trans. Smart Grid 14 (6), 4976–4979. doi:10.1109/TSG.2023.3309528
Ziyat, I., Gola, A., Palmer, P. R., Makonin, S., and Popowich, F. (2023). EV charging profiles and waveforms dataset (EV-CPW) and associated power quality analysis. IEEE Access 11, 138445–138456. doi:10.1109/ACCESS.2023.3340131
Zsiborács, H., Vincze, A., Pintér, G., and Baranyai, N. H. (2023). The accuracy of PV power plant scheduling in Europe: an overview of ENTSO-E Countries. IEEE Access 11, 74953–74979. doi:10.1109/ACCESS.2023.3297494
Glossary
Keywords: ancillary services, charging station, electrical vehicles, energy management, environmental impact, renewable energy integration, renewable energy resources, smart grid
Citation: Rehman Au, Khalid HM and Muyeen SM (2024) Grid-integrated solutions for sustainable EV charging: a comparative study of renewable energy and battery storage systems. Front. Energy Res. 12:1403883. doi: 10.3389/fenrg.2024.1403883
Received: 20 March 2024; Accepted: 30 August 2024;
Published: 17 September 2024.
Edited by:
Lei Chen, Wuhan University, ChinaReviewed by:
Tong Ding, Anhui University, ChinaPan Hu, State Grid Hubei Electrical Power Research Institute, China
Copyright © 2024 Rehman, Khalid and Muyeen. This is an open-access article distributed under the terms of the Creative Commons Attribution License (CC BY). The use, distribution or reproduction in other forums is permitted, provided the original author(s) and the copyright owner(s) are credited and that the original publication in this journal is cited, in accordance with accepted academic practice. No use, distribution or reproduction is permitted which does not comply with these terms.
*Correspondence: Haris M. Khalid, aGFyaXNtLmtoYWxpZEB5YWhvby5jb20=, bWtoYWxpZEB1ZC5hYy5hZQ==