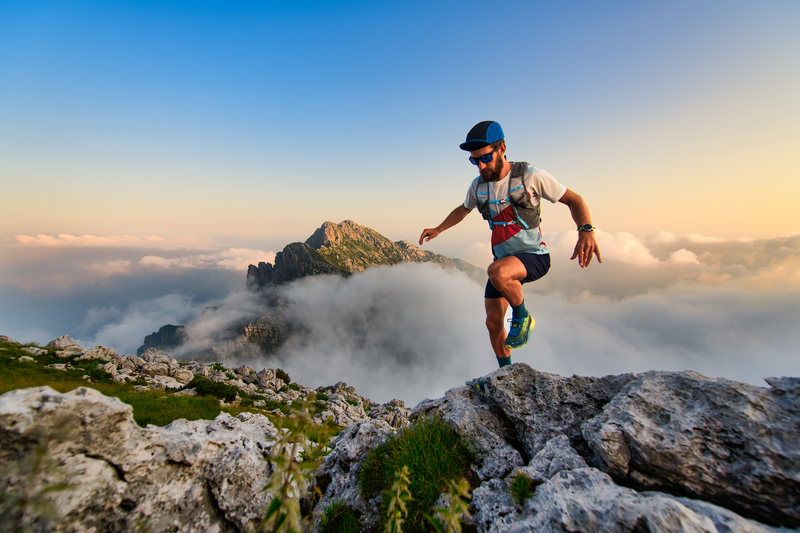
95% of researchers rate our articles as excellent or good
Learn more about the work of our research integrity team to safeguard the quality of each article we publish.
Find out more
ORIGINAL RESEARCH article
Front. Energy Res. , 02 August 2024
Sec. Nuclear Energy
Volume 12 - 2024 | https://doi.org/10.3389/fenrg.2024.1400905
The future electric loads in the Kingdom of Saudi Arabia (KSA) are increasing significantly, particularly in the Eastern Province of the KSA. These high-rise loads are primarily driven by the operational needs of the Saudi Arabian oil company Aramco, including oil refineries, and the infrastructures of the Saudi Basic Industries Corporation (SABIC) factories. This study aims to construct a nuclear power plant in that area to supplement and support the baseload currently covered by conventional generation units powered by fossil fuels within the Saudi Electricity Company (SEC) operations. The objective function is to minimize the operational costs of the power systems to the greatest extent possible. This paper describes a case study conducted using the IBM CPLEX Optimizer software to compare the operational costs of KSA’s power systems for a 24-h period. Two scenarios were considered and addressed: the first scenario without the inclusion of a nuclear power plant (NPP) and the second scenario with the inclusion of the NPP. The unit commitment problem was modeled for both scenarios. The obtained results revealed that the second scenario, which involved the penetration of the NPP, offered an optimal economic solution for operating KSA’s power systems. By employing the CPLEX Optimizer software and analyzing the unit commitment problem, this study provides valuable insights into the economic advantages of integrating the NPP into the power systems of the Kingdom of Saudi Arabia. The NPP shows viability in terms of minimizing the operational costs to 32.10 $/MWh compared to the first scenario where the operational costs were 42.10 $/MWh and resulted in almost 24% reduction in operational costs. In addition, the NPP is deemed as an optimal technology to contribute to the net zero goal by 2060, where it can reduce the reliance on fossil fuel power plants and contribute to the reduction of CO2 emissions.
The Kingdom of Saudi Arabia (KSA) relies heavily on oil for electricity production in its current state. However, the country is undergoing significant transformation, with a focus on diversifying its energy sources and increasing the proportion of renewable energy in its energy mix. According to the KSA 2030 vision, the goal is striving to shift to environmentally friendly energy resources to mitigate its reliance and dependence on fossil fuels. Therefore, the KSA is shifting its focus toward acquiring electric power of more than 60 GW from sources other than conventional energy resources only. Hence, this research paper aims to introduce nuclear energy into the energy mix of the KSA to cover and satisfy such expected increasing electric loads and population growth by 2030 and the necessity for energy by then. In The Kingdom needs for Nuclear Energy (2020), the Saudi Government announced that it was considering a nuclear power program on its own, and in April 2010, a royal decree stated: “The development of atomic energy is essential to meet the Kingdom’s growing requirements for energy to generate electricity, producing desalinated water and reducing reliance on depleting oil and gas resources.” The King Abdullah City for Atomic and Renewable Energy (KA-CARE) was set up in Riyadh to advance this agenda as an alternative to fossil fuels and to be the competent agency for treaties on nuclear energy signed by the kingdom. The KA-CARE also supervises works related to nuclear energy and radioactive waste projects. Nuclear power plants offer many advantages such as providing emission-free electricity, which is irreplaceable in protecting the environment. In addition, it can respond to the growing energy demand and helps the KSA meet sustainable development goals and plans. Furthermore, nuclear power might be used to power the shortly coming electric vehicles, which will ensure reduction in carbon emissions when powered by carbon-free nuclear energy.
There is an ever-increasing electric demand in the Eastern Operation Area (EOA) of the Saudi Electric Company (SEC), particularly due to the huge oil infrastructures and refineries of the Saudi Arabian oil company Aramco, in addition to energy requirements of the Saudi Basic Industries Corporation (SABIC) factories’ infrastructures, all being located and operated in that area. Therefore, it is imperative to build more power generation facilities to cope with these rapidly increasing electric loads. In addition, with the increase in CO2 emissions worldwide and the commitment of the KSA among all countries in taking measures to reduce greenhouse gas emissions in accordance with the 2015 Paris Climate Agreement along with net zero goal by 2060, introducing clean energy is vital and necessary to achieve such goals, and NPP is one of the cleanest energy sources that can contribute to reducing CO2 emissions.
Nuclear power technology is a crucial focus of this work; therefore, relevant references in this field will be cited and documented accordingly. In Establishment of Saudi Nuclear Energy Holding CompanySaudi National Atomic Energy Project SNAEP and King Abdullah City for Atomic and Renewable Energy KA-CARE (2019), the KA-CARE confirmed that the KSA has been increasing its efforts in meeting the growing demands by incorporating alternative sources of energy in its energy mix to reduce the dependence on fossil fuels. Nuclear energy is one of the alternative energy sources that can assist in electricity power generation and water desalination. In Nuclear Power in Saudi Arabia (2022), during the 67th International Atomic Energy Agency General Conference, the Minister of Energy reaffirmed the KSA’s commitment in developing peaceful utilization methods for nuclear energy across various sectors. The Saudi National Atomic Energy Project includes the construction of a nuclear power plant to support the sustainable development objectives outlined in the Saudi Vision 2030 roadmap. The country is actively working with the International Atomic Energy Agency (IAEA) to leverage its expertise and advisory services in implementing safeguards. This collaboration aims to develop the necessary national infrastructure and enhance human capabilities to support the transition toward nuclear energy. To facilitate the development of nuclear energy, Saudi Arabia has established the Nuclear Energy Holding Company, which is the country’s nuclear developer. According to the World Nuclear Association’s Nuclear Fuel Report in 2023, the initial plans involve the construction of two 1.4-GWe nuclear reactors, with a long-term goal of reaching a nuclear capacity of 17 GWe by 2040. Saudi announces establishing Saudi Nuclear Energy Holding Company (2022) reveals that the KSA has announced its plan to establish a holding company for nuclear energy to participate in local and international commercial nuclear programs. In addition, it confirmed that this holding company would participate in nuclear economic projects locally and internationally and will cooperate with international institutes for atomic energy research and work toward creating a national digital platform to build and attract human capabilities in the field of nuclear energy. The report in Electric Grid Reliability and Interface with Nuclear Power Plants (2012) describes the characteristics of the electrical grid system that are required for the connection and successful operation of an NPP, as well as the characteristics of an NPP that are significant for the design and operation of the electrical grid system. It addresses the issues to be considered for planning of an NPP and describes the information exchange necessary between the developer of a nuclear power plant and the organization responsible for the electrical grid. Collier (1984) proposed that it is essential that nuclear power is developed completely to meet increasing energy demands. However, the future of nuclear power does not depend much on the developments of the technology but on public installation acceptance. Overly (2022) author stated that the energy market shifts the potential of nuclear energy to meet the need for clean and affordable energy solutions in the future. In fact, nuclear energy remains the greatest contributor to clean power in many countries. Nuclear energy can fill the intermittent gaps of clean energy sources like solar and wind and create new jobs. Over the past 50 years, research and development have made huge strides toward a future for nuclear energy that is both safe and cost-effective, while maintaining a low environmental impact. Beck et al. (2002) showed that for a nuclear power plant, it is important that an expert system supplies useful information to the operator to meet the increasing demand for high-level plant operation. It is difficult to build a user-friendly expert system that supplies useful information in real time using existing general-purpose expert system shells. Therefore, we selected a domain-specific expert system shell with a useful knowledge representation for problem-solving in NPP operation. An expert system for a nuclear power plant operation and guidance system utilizing the plant table P/T was developed to assist automatic plant operation and surveillance test operation. In these systems, automatic plant operation signals to the plant equipment and operation guidance messages to the operators are both outputs based on the processing and assessment of plant operation conditions by the P/T. Robertson and Lamont (2015) mentioned that nuclear power is more subject to debate than ever: with the push to reduce carbon emissions and the increasing demand in energy, nuclear energy may seem an ideal way to meet both concerns. However, the environmental and safety hazards associated with nuclear energy are not negligible and are crucial factors to be considered in decisions to proceed with nuclear power as a source of energy. The discussion provides a broad, informed overview of the nuclear sector, identifying key players, current developments, and current and future technology. The report in Use of Expert Systems in Nuclear Safety (1988) reveals that the one dominant aspect of improvement in safe nuclear power plant operation is the extremely high speed in the development and introduction of computer technologies. This development commenced recently with incorporation of advanced control technology into the nuclear industry. This led to an increasing implementation of information displays, annunciator windows, and other devices inside the control room, eventually overburdening the control room operator with detailed information. The logical next step is therefore to concentrate the collected data in a well-structured display manner with prioritization capabilities. Advancement in software development subsequently helped transform the computerized operator support system from a simple status-quo presentation into a veritable decision aid with capacities for diagnosis, trend analysis, and checking of recovery actions. Expert systems are a further step in this direction, being designed to apply large knowledge bases to solve practical problems. These “intelligent” systems must incorporate enough knowledge to reach expert levels of importance and represent a very advanced man–machine interface. Schlissel and Biewald (2008) indicated that the construction cost estimates for new NPPs are very uncertain and have increased significantly in recent years. Companies that are planning new nuclear units are currently indicating that the total costs (including escalation and financing costs) will be in the range of $5,500/kW–$8,100/kW or between $6 billion and $9 billion for each 1,100 MW plant. These added cost estimates are far higher than the industry had previously predicted. For example, as recently as the years 2000–2002, the industry and the Department of Energy were talking about overnight costs of $1,200/kW–$1,500/kW for new nuclear units. This range of estimated overnight costs suggested the total plant costs of between $2 and $4 billion per new nuclear unit. The MIT Future of Nuclear Study in 2003 increased the estimated prices of new nuclear plants to $2,000/kW, not including financing costs. However, the estimated costs for new NPPs began to increase significantly starting in 2006–2007. Other recently announced NPP cost estimates are in the same range as Florida Power & Light. For example, Progress Energy has projected a cost of approximately $10.5 billion for two new nuclear units with financing costs bringing the total up to approximately $13–14 billion. However, Progress Energy has not yet released any of the details underlying this cost estimate. Mazzoni (2019) indicated in the record of the past 50 years that nuclear power has an edge over other forms of energy sources, both in terms of limiting adverse health and environmental effects, including greenhouse gas emissions and in terms of the frequency and toll of major accidents. This study provides a review of nuclear power generation, which is necessary to study the electrical systems and their importance in safety. The burning of fossil fuels creates grim environmental effects. Nuclear plant accidents are discussed, with particular emphasis on the accident contribution from electrical and instrumentation safety system operation. This study also discusses major nuclear plants, advanced reactors, and past major nuclear accidents, such as those at Three Mile Island, Chernobyl, and Fukushima. Allan (2013); Shaalan (2019) displayed a methodology for optimal dispatching of generating units based on a loading order considering the least cost operating units. Dernayka et al. (2022) states that two potential candidate sites for accommodating the first NPP in the KSA are Umm Huwayd and Khor Duwehin located on the east coast near to the UAE and Qatar borders. In addition, site selection parameters are very significant in terms of assessing the suitability of the potential sites such as geology and seismology, meteorology, population consideration, and hydrology in Dernayka et al. (2022), and the location of the NPP considered a major milestone to be identified and selected based on the aforementioned criteria, and in this research study, the proposed potential location would be the Eastern Province region, specifically the Khor Duwehin site, which is situated near to the UAE and Qatar Borders and the most nearest load center which is approximately 200 km.
The key objectives and contributions of this study include the following:
⁃ Developing a strategic plan for integrating NPPs into the existing energy infrastructure.
⁃ Fostering the adoption of nuclear energy for electricity generation.
⁃ Promoting the sustainable use of nuclear technology for peaceful applications.
⁃ Aligning with global standards in the nuclear industry to ensure safety and efficiency.
⁃ Enhancing the reliability and quality of electricity supply, while reducing costs for the Saudi Electricity Company.
⁃ Providing actionable design recommendations for maximizing safety and reliability in NPPs.
Electricity providers are facing the problem of deciding which power units to run, in which periods, and at what level to satisfy the electricity demand. This problem is known as the unit commitment problem (UCP).
The optimization inputs of the UCP are as follows:
- Some known data such as the characteristics of the generators (minimum and maximum capacity, ramp-up, and down capacity (how much more or less the generator can produce from one period to the next), availability, fixed costs, and variable costs.
Decision optimization problem-solving requires the following:
- A model: some mathematical formulation of the UCP where UCP’s constraints and objectives are expressed as mathematical statements.
- Some input data for UCP and unit loading priority to solve.
- An optimization engine: that will compute a solution for these input data and this optimization model formulation [in this case, IBM CPLEX Optimizer will be utilized]. Mixed-integer quadratic programming (MIQP) will be used in this modeling, and MIQP is used in optimization of power system operations besides other methods such as mixed-integer linear programming.
The unit commitment model will be developed based on KSA’s power grid. The current installed capacity of KSA’s power grid is approximately 90 GW (Statistical Booklet Wera, 2022). The energy mix nowadays within the KSA’s power grid is shown in Figure 1 below which consists of diesel generators (3.7%), gas turbines (59.7%), steam turbines (21.7%), renewable energy (0.9%), and combined cycle (14%) (Statistical Booklet Wera, 2022).
The 24-h load variation curve during August 2022 in the Eastern Region in KSA is shown in Figure 2. It shows the load duration curve during August 2022, which considers the peak load of KSA’s power grid. In Figure 2, the load duration curve is plotted in ascending order, and the reason behind the ascending order from the power system planning perspective is to help the power system planning process in terms of choosing the right power plants for accommodating needed power for baseload, intermediate load, and peak load cases.
In the study by Hamieh et al. (2022), CO2 emissions in million tons per year (mty) per region are shown in Figure 3, which depicts that the Eastern Region (called in Figure 3 “Ash Sharqiyah”) is the second largest region that emits CO2 due to the number of power plants accomodated in the Eastern Region.
The case study load profile will be based on August, specifically for 24 h only, as illustrated in Table 1. Peak hours will be from 12:00 p.m. to 5:00 p.m. The baseload of the Eastern Province during 24 h in August is 16 GW according to the monthly demand variation of Eastern Region, and the peak load is 19.9 GW.
The model consists of the ranges where the time range will be based on 24-h in Table 1, number of units for each unit type (steam, combined cycle, gas, and nuclear) in Figure 4, defining variables within the model, unit type information in terms of the minimum and maximum power each unit can generate, defining fuel cost coefficients for each unit type, start-up and shutdown costs for each unit type, CO2 emission costs for each unit type, and objective function with relevant equations along with constraints.
The objective function of KSA’s power system aims to minimize the operating cost and consists of the fuel cost of steam, gas, and nuclear units; start-up costs; shutdown costs; and CO2 emissions costs over the scheduling period (24 h). The objective function is given in Eq. 1 (Matar, 2017; Hayati and Sati, 2019; Hamieh et al., 2022; Statistical Booklet Wera, 2022; Olkiluoto 3 1600MW nuclear power plant, 2024).
where
T: denotes the case study time, which is equal to 24 h.
G: denotes the number of units
Fuel cost extracting from the consumed fuel in thermal and nuclear units. Thus, the aggregate fuel cost is depicted in Eqs 2–6 below.
where
Table 2 outlines cost parameters and fuel cost values for each unit type. Each unit type has its own cost coefficient parameter and values which have been extracted by proposed values in Islam et al. (2020).
The typical refueling cycle (Güvenç et al., 2021) for nuclear units is in the range of 12–18 months (Nuclear Fuel Cycle Overview, 2021). In addition, reactor core control measures within nuclear units in terms of reactivity, chemical compensation, and fuel burn-ups are very complex and necessitate careful fuel assembly design (Wang et al., 2021; Zou et al., 2023). Hence, the start-up costs of the NPP will be negligible. In addition, the start-up costs for steam, combined cycle, and gas units are depicted in Eqs 7–10 below.
where
Figure 5 illustrates the start-up costs for each unit type, the steam power plant start-up costs are 130$ per MW, combined cycle power plant start-up costs are 140$ per MW, gas power plant start-up costs are 150$ per MW, and nuclear power plant start-up cost will be negligible and assumed as the baseload power plant (Xu et al., 2017).
The typical refueling cycle for nuclear units is in the range of 12–18 months (Nuclear Fuel Cycle Overview, 2021). Hence, the shutdown costs will be negligible. In addition, the shutdown costs for steam, combined cycle, and gas units are given in Eqs 11–14 below.
where
Figure 6 outlines the shutdown costs for each unit type, with steam power plants incurring shutdown costs of $12 per megawatt (MW), combined cycle power plants incurring $14 per MW, gas power plants incurring $15 per MW, and nuclear power plants assumed to have negligible shutdown costs, as they are considered baseload power plants (Xu et al., 2017).
The electricity sector in the KSA is considered a major CO2 emitter (26.72% of the total CO2 emission) (Hamieh et al., 2022). The following Eqs 15–19 represent the total cost of CO2 from steam, combined cycle, gas, and nuclear units.
where
Figure 7 presents the CO2 emission costs and factors for each unit type, outlining the contribution of different power plants to CO2 emissions. Steam power plants are noted for generating and emitting 670 kg of CO2 emissions per megawatt-hour (MWh), combined cycle power plants emit 370 kg per MWh, gas power plants emit 540 kg per MWh, while nuclear power plants emerge as the least CO2 emitters, emitting only 50 kg per MWh (Hamieh et al., 2022; Yildirim and Özcan, 2022).
The generated power shall meet the needed loads to balance any power system. The following Eq. 20 illustrates the mathematical relationship between load and generation in the power systems.
Each unit type has its own minimum and maximum capacities. The following Eqs 21–24 illustrate the minimum and maximum capacities for steam, combined cycle, gas, and nuclear units.
where
Figure 8 shows the minimum and maximum capacities for each unit type, steam power plants can produce power in the range from 22 MW to 757 MW, combined cycle power plants can generate power in the range from 58 MW to 360 MW, gas power plant can produce 5 MW to 239 MW, and nuclear power plant is deemed to be baseload with full power capacity, which is 1600 MW (Statistical Booklet Wera, 2022; Olkiluoto 3 1600MW nuclear power plant, 2024).
Nuclear units will operate as baseload units because of the characteristics of operational aspects of NPPs and stringent regulatory requirements from nuclear regulators to ensure the safe operation of NPPs.
The flowchart shown in Figure 9 consists of the two scenarios covered in this study. The first scenario which does not include the NPP is to build the unit commitment model by using a CPLEX Optimizer that encompasses the objective function and power system constraints. The second scenario which includes the NPP is to build the unit commitment model by using a CPLEX Optimizer that comprises the objective function and power system constraints.
The case study is based on the KSA’s power systems and consists of two scenarios. Scenario 1, which does not encompass the NPP, involves building a unit commitment model by using CPLEX Optimizer to evaluate the operational costs of the KSA’s power system, as is without the inclusion of the NPP. On the other hand, Scenario 2, which comprises the NPP, involves building the unit commitment model by using CPLEX Optimizer to assess the operational costs of KSA’s power system after the inclusion of the NPP. Thus, the operational costs of the proposed scenarios are depicted in Table 3 below. The presented results in Table 3 indicate that the operational cost of running the KSA power system, excluding the NPP, is $42.10/MWh, which is higher compared to the scenario where the NPP is included, resulting in an operational cost of $32.10/MWh. This reflects a significant reduction in operational costs by almost 24%. The economic viability of the NPP is underscored by its low fuel costs in comparison to steam power plants utilizing crude oil, known for their prohibitive costs. By substituting 76,800 MWh of energy generated by NPPs for steam power plants, the combined total costs of both steam and NPPs amount to $11,479,861, contrasting with the scenario without an NPP where total costs were $15,723,667. This highlights that the incorporation of an NPP in the baseload has reduced the overall costs of generated energy from baseload power plants by approximately 27%. The equation to acquire the operational cost per MWh is to divide the total cost incurred from all units by total generation produced from all units.
Figure 10 below illustrates two scenarios regarding CO2 emission costs, revealing an approximate 20% reduction in CO2 emission costs upon the introduction of the NPP. This reduction can be attributed to the stark differences in emission levels between steam power plants and NPPs. Specifically, steam power plants emit 670 kg per MWh, while NPPs emit only 50 kg per MWh. This significant disparity has led to a considerable reduction in the total costs of CO2 emissions, with NPP CO2 costing $38,400 over a 24-h period, compared to steam power plants costing $1,831,271 over the same duration.
Figure 11 below depicts two scenarios concerning fuel costs for each unit type, revealing a notable reduction of approximately 33% in steam units’ operational costs upon the introduction of the NPP. Furthermore, Figure 6 demonstrates a significant decrease in steam units’ fuel costs, decreasing from $13,339,968 to $8,957,719, resulting in a reduction of almost $3 million with the inclusion of the NPP, which incurs a cost of approximately $1 million over a 24-h period.
The study aims to integrate an NPP into the existing energy infrastructure to support baseload requirements currently met by fossil fuel-based generation units within the SEC. The objective is to minimize the operational costs of the power systems. A case study was conducted using IBM CPLEX Optimizer software to compare the operational costs of KSA’s power systems over a 24-h period. Two scenarios were examined: one without the inclusion of the NPP and the other with the NPP included, with the unit commitment problem modeled for both scenarios. The main objective was to compare and evaluate the economic aspects of operating these scenarios. The modeling analysis using the mixed-integer quadratic programming method through CPLEX software revealed that the KSA power system without the NPP incurred higher costs where operational costs equal to 42.10 $/MWh compared to the scenario with the NPP where operational costs equal to 32.10 $/MWh. Thus, decreases in operational costs by almost 24% indicate that an NPP is one of the cleanest and most reliable technologies.
The main recommendations are that the necessity of introducing an NPP into the KSA power systems is very crucial and needs to be considered viable, reliable, and clean energy technology to produce and generate power to accommodate expected loads. In addition, decreasing the reliance on fossil fuel unit types for the sake of diminishing the emissions of CO2 is achieved by introducing clean energy, and one of the cleanest energy producers is an NPP, which will contribute to achieving the net zero goal by 2060 and fulfill the 2015 Paris agreement.
The original contributions presented in the study are included in the article/Supplementary Materials; further inquiries can be directed to the corresponding authors.
AA-Q: conceptualization, data curation, formal analysis, funding acquisition, investigation, software, writing–original draft, and writing–review and editing. AA-S: conceptualization, data curation, investigation, methodology, project administration, supervision, and writing–review and editing. WA-M: methodology, supervision, and writing–review and editing. HH: data curation, investigation, methodology, supervision, validation, and writing–review and editing.
The author(s) declare that financial support was received for the research, authorship, and/or publication of this article. This work was supported by the Researchers Supporting Project number (RSP2024R337), King Saud University, Riyadh, Saudi Arabia.
The authors declare that the research was conducted in the absence of any commercial or financial relationships that could be construed as a potential conflict of interest.
All claims expressed in this article are solely those of the authors and do not necessarily represent those of their affiliated organizations, or those of the publisher, the editors, and the reviewers. Any product that may be evaluated in this article, or claim that may be made by its manufacturer, is not guaranteed or endorsed by the publisher.
Allan, R. N. (2013). Reliability evaluation of power systems. Berlin, Germany: Springer Science and Business Media.
Beck, C. E., Behera, A. K., and Reed, M. L. (2002). “The applicability of expert systems to the control and operation of nuclear power generating plants,” in Department of electrical and computer Engineering, Illinois institute of technology, Chicago, IL, USA, August 6, 2002 (Published by the IEEE Xplore).
Collier, J. G. (1984). Generation Development and construction division, central electricity generating board, Barnwood, UK. Nuclear energy 30 (8), 609–615.
Dernayka, S., Wuhayb, F. A., and Zohbi, G. A. (2022). Assessment of potential nuclear in selected sites in Saudi Arabia. J. Nucl. Ene Sci. Power Gener. Technol. 11 (8), 2.
Electric Grid Reliability and Interface with Nuclear Power Plants (2012). IAEA nuclear energy Series, A report issued by the international atomic energy agency (IAEA), IAEA nuclear. energy, series No. NG-T-3.8.
Establishment of Saudi Nuclear Energy Holding CompanySaudi National Atomic Energy Project (SNAEP), King Abdullah City for Atomic and Renewable Energy (KA-CARE) (2019). Second meeting of the technical working group for small and medium-sized or modular reactor (TWG-SMR).
Güvenç, U., Bakir, H., and Duman, S. (2021). Investigation the success of semidefinite programming for the estimating of fuel cost curves in thermal power plants. Politeknik Derg. 24, 247–254. doi:10.2339/politeknik.645827
Hamieh, A., Rowaihy, F., Al-Juaied, M., Abo-Khatwa, A. N., Afifi, A. M., and Hoteit, H. (2022). Quantification and analysis of CO2 footprint from industrial facilities in Saudi Arabia. Energy Convers. Manag. X 16, 100299. doi:10.1016/j.ecmx.2022.100299
Hayati, M., and Sati, S. E. (2019). “Operating cost comparison of Sudan’s power system grid with and without NPP integration,” in 2019 international Conference on computer, control, electrical, and Electronics Engineering (ICCCEEE), September 2019 (IEEE), 1–5.
Islam, M. A., Hasan, N., and Mohammad, N. (2020). “Power system optimization model using economic load dispatch,” in 2020 IEEE region 10 Symposium (TENSYMP), June 2020 (IEEE), 469–472.
Matar, W. (2017). Reforming industrial fuel and residential electricity prices in Saudi Arabia. No. ks-2017--dp018.
Nuclear Power in Saudi Arabia (2022). World nuclear association, information library, tower House, 10 Southampton Street, London, WC2E 7HA, United Kingdom.
Olkiluoto 3 1600MW nuclear power plant (2024). Olkiluoto 3 1600MW nuclear power plant. Power technology.
Overly, S. (2022). Nuclear energy primer. Conserv. Energy Netw. Cen. 101 N Washington Sq, Ste 400A Lansing, MI 48933, January 19, 2022.
Robertson, L. K., and Lamont, L. A. (2015). “An overview of nuclear power,” in 2015 5th international Youth Conference on energy (IYCE), May 2015 (IEEE), 1–6.
Saudi announces establishing Saudi Nuclear Energy Holding Company (2022). Memo (Middle East Monitor), published by the IAEA international organizations, Middle East, News, Saudi Arabia, March 12, 2022.
Schlissel, D., and Biewald, B. (2008). Nuclear power plant construction costs. Energy economics incorporation (Synapse), 22 Pearle Street. Cambridge, MA 02139.
The Kingdom needs for Nuclear Energy (2020). King Abdullah city for atomic and renewable energy (KACARE). Report.
Use of Expert Systems in Nuclear Safety (1988). Report of A Technical committee meeting organized by the international atomic energy agency (IAEA), Vienna, October 17–21, 1988.
Wang, J., Liu, S., Li, M., Xiao, P., Wang, Z., Wang, L., et al. (2021). Multiobjective genetic algorithm strategies for burnable poison design of pressurized water reactor. International J. Energy Res. 45 (8), 11930–11942. doi:10.1002/er.5926
Xu, T., Birchfield, A. B., Gegner, K. M., Shetye, K. S., and Overbye, T. J. (2017). Application of large-scale synthetic power system models for energy economic studies.
Yildirim, M., and Özcan, M. (2022). Unit commitment problem with emission cost constraints by using genetic algorithm. Gazi Univ. J. Sci. 35 (3), 957–967. doi:10.35378/gujs.942680
Keywords: nuclear power plant, operational costs, CO2 emissions, unit commitment, baseload, CPLEX Optimizer
Citation: Al-Qahtani AM, Al-Shaalan AM, Al-Masry WA and Hussein Farh HM (2024) Mixing nuclear and conventional fossil fuel units within the baseload of PP using the CPLEX Optimizer. Front. Energy Res. 12:1400905. doi: 10.3389/fenrg.2024.1400905
Received: 14 March 2024; Accepted: 16 July 2024;
Published: 02 August 2024.
Edited by:
Muhammad Saeed, University of Electronic Science and Technology of China, ChinaReviewed by:
Shichang Liu, North China Electric Power University, ChinaCopyright © 2024 Al-Qahtani, Al-Shaalan, Al-Masry and Hussein Farh. This is an open-access article distributed under the terms of the Creative Commons Attribution License (CC BY). The use, distribution or reproduction in other forums is permitted, provided the original author(s) and the copyright owner(s) are credited and that the original publication in this journal is cited, in accordance with accepted academic practice. No use, distribution or reproduction is permitted which does not comply with these terms.
*Correspondence: Abdullah M. Al-Qahtani, YWJkdWxsYWhtYWxxYWh0QGdtYWlsLmNvbQ==; Abdullah M. Al-Shaalan, c2hhYWxhbkBrc3UuZWR1LnNh; Waheed A. Al-Masry, d2FsbWFzcnlAa3N1LmVkdS5zYQ==; Hassan M. Hussein Farh, aGhodXNzZWluQGltYW11LmVkdS5zYQ==
Disclaimer: All claims expressed in this article are solely those of the authors and do not necessarily represent those of their affiliated organizations, or those of the publisher, the editors and the reviewers. Any product that may be evaluated in this article or claim that may be made by its manufacturer is not guaranteed or endorsed by the publisher.
Research integrity at Frontiers
Learn more about the work of our research integrity team to safeguard the quality of each article we publish.