- 1Department of Industrial Engineering, Gaziantep University (UoG), Gaziantep, Türkiye
- 2Department of Research and Development, ENERGCO, Istanbul, Türkiye
- 3Israa Universit, Gaza Strip, Palestine
Recently, artificial intelligence (AI) and blockchain have become two of the most trending and disruptive technologies. Blockchain technology can automate payment in cryptocurrency and provide access to a shared ledger of data, transactions, and logs in a decentralized, secure, and trusted manner. In addition, with smart contracts, blockchain has the ability to govern interactions among participants with no intermediary or a trusted third party. AI, on the other hand, offers intelligence and decision-making capabilities to machines similar to humans. This review presents a detailed survey on blockchain and AI basics and features. This paper provides a review of the literature and a brief on the integration of blockchain and AI applications in multiple areas. We also identify some sole cases of blockchain–AI integration in the energy sector with current use cases. Eventually, we discuss research advantages and challenges associated with integrating blockchain with AI in the energy domain.
1 Introduction
Both blockchain and artificial intelligence (AI) technologies have been gaining a huge traction as underlying core technologies are being adopted as a combination in various applications, such as healthcare, military, digital world simulation, real-time, decision agonistic, data security (Salah et al., 2019). However, with the novelty and limitation aspect, few research studies have been conducted on how blockchain and AI can be combined to facilitate their use in the energy space.
Blockchain technology was solely deployed in some potential use cases in energy applications, including energy company operations, wholesale energy trading and supply, Internet of Things (IoT) platforms and digitalization, decentralized energy, and peer-to-peer (P2P) trading (Andoni et al., 2019a). Meanwhile, AI has been actively used in different renewable energy (RE) source-based systems including wind, solar, geothermal, and hybrid energy for the design, optimization, estimation, management, and distribution (Jha et al., 2017).
Blockchain and AI are very promising technologies and could potentially shape the future of energy and provide solutions to some of the challenges faced by the energy sector as blockchain provides solutions with decentralization and AI provides intelligent autonomous optimization and can act as a decision-maker for power system operation (Hua et al., 2022).
Interestingly, blockchain and AI integration in the energy sector is gaining attention due to their potential for enhancing efficiency and security. Recent research highlights how this combination addresses critical issues, particularly in energy management. While the pairing of AI and blockchain is relatively rare, it holds unique promise (Kuzior et al., 2022).
Keyword searches such as “Artificial Intelligence” and “Blockchain” on databases such as Scopus and Web of Science reveal frequent usage alongside terms related to energy and energy management (Kuzior et al., 2022). Blockchain’s ability to process millions of transactions per second (TPSs) has interested researchers (see Figure 1) as it enhances system speed and efficiency. AI further supports this by autonomously managing the system, resulting in a secure and efficient solution. These technologies also drive significant shifts in business models, profitability, and income streams (Kuzior et al., 2022).
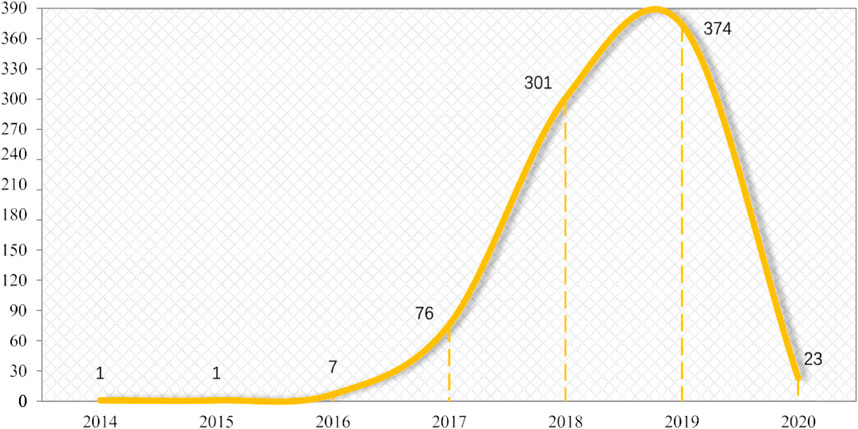
Figure 1. Graph depicting the number of articles about blockchain technology in the energy sector from 2014 to 2020 (Salman et al., 2018).
Blockchain technology is revolutionary in securing and privatizing data. With various consensus mechanisms such as proof-of-work (PoW) and proof-of-stake (PoS), its primary appeal to the energy sector is security. As a distributed ledger, blockchain creates a peer-to-peer network, making it a cost-effective and faster alternative to traditional systems. Initially introduced by Satoshi Nakamoto in 2008 for decentralization, blockchains have since evolved, focusing on faster transactions. Smart contracts (SCs) enable automation (Goli et al., 2024), gaining interest from startups, government agencies, and firms (Andoni et al., 2019b). Blockchain’s broad applicability makes its integration in the energy sector promising, especially for securely storing data, as seen in a case study involving energy tokens and peer-to-peer utilities (Andoni et al., 2019b).
AI, functioning like a supercomputer, enhances systems with learning, prediction, and problem-solving abilities. It improves productivity, reduces machinery breakdown, and lowers costs (Kuzior et al., 2022). Ongoing research on AI offers valuable insights for both professionals and businesses (Bishaw, 2024), fostering innovation and industry growth. Given AI’s focus on automation, its role in the energy sector is inevitable, automating processes and transactions, while blockchain handles the technical aspects of transaction creation and data storage.
However, integrating blockchain and AI in the field of energy trading is still in its infancy, and there are many challenges that need to be addressed and opportunities that need to be reaped. To date, the available studies show that researchers reviewed blockchain and AI separately, along with their applications in various business domains (Salman et al., 2018; Zheng X. et al., 2018; Baltrušaitis et al., 2018; Fioretto et al., 2016; Yeow et al., 2018a; Fernández-Caramés and Fraga-Lamas, 2018; Panarello et al., 2018; Christidis and Devetsikiotis, 2016; Neudecker and Hartenstein, 2018; Cai et al., 2018; Brundo and De Nicola, 2018; Dinh et al., 2018; Li et al., 2017; Zheng et al., 2016). In the AI domain, Safaei et al. (2022a) developed a closed-loop supply chain (CLSC) network model, aiming to minimize total costs and improve service levels by incorporating suppliers, manufacturers, distribution centers, customers, and recycling units. The model utilized mixed-integer linear programming (MILP) and ARIMA for demand estimation, effectively reducing shortages. Problem-solving was enhanced using GAMS and genetic algorithms for different problem sizes. Similarly, Momenitabar et al. (2022a) introduced a sustainable closed-loop supply chain network (SCLSCN) model that integrates backup suppliers and lateral transshipment to address cost, environmental impact, and shortages while optimizing job creation. Tested in the tire industry, this model utilized a fuzzy inference system (FIS) for demand estimation and demonstrated robustness through sensitivity analysis on demand variations, effectively reducing costs and shortages.
AI techniques have been instrumental in forecasting demand and optimizing supply chain networks. Momenitabar et al. (2022b) and Momenitabar et al. (2023) used AI techniques such as random forest, XGBoost, and AdaBoost to forecast bioethanol demand, with random forest providing superior accuracy. These AI-driven projections informed strategic decisions in designing sustainable bioethanol supply chain networks (SBSCNs). Additionally, the studies highlighted the importance of preprocessing and biorefinery center costs and the efficacy of the MOIWO algorithm, which outperformed NSGA-II in optimizing the SBSCN. Goodarzian et al. (2023) addressed sustainability goals in CLSC design using a multi-objective MILP model for a sustainable citrus CLSC. This model integrated social and environmental impacts and used machine learning and meta-heuristic algorithms such as ε-constraint, SPEA-II, and PESA-II to optimize decisions across production, distribution, and recycling, demonstrating its applicability through a real case study in Mazandaran, Iran, with broader implications for improving global citrus supply chain sustainability.
The integration of AI and blockchain is crucial for advancing energy management systems. Khan A. et al. (2023) proposed a B-PDA framework combining blockchain and AI for the dynamic management of smart grid automation, addressing advancements in real-time analysis, security, and privacy management through AI-driven smart contracts and consensus protocols. Similarly, Li et al. (2023a) explored the integration of AI and blockchain in smart energy management, using AI models to predict energy demand and optimize scheduling, supported by big data for enhanced performance. Blockchain ensured secure energy trading through smart contracts, promoting transparency and resilience in energy grid operations. The AEBIS system discussed by Wang et al. (2020) merges electric vehicles with virtual power plants (VPPs) for smart grid management, using AI techniques such as neural networks and federated learning to enable precise EV power consumption forecasts and blockchain for enhanced security and transparency in data transactions. Furthermore, Kumari et al. (2020) emphasized the significance of integrating AI and blockchain in energy cloud management (ECM) systems to address challenges such as increasing energy demand, renewable energy integration, IoT expansion, and cybersecurity risks. Kumari et al. (2021) have proposed a unique study that demonstrates P2P trading by utilizing the effectiveness of big data by combining blockchain technology. 6G and a trading process on the Ethereum blockchain initiated by smart contracts with AI that learns has been emphasized. In addition, (Ma et al., 2022) has proposed a demand and control management framework that utilizes blockchain and AI’s reinforcement learning feature. For example, widely distributed machine learning algorithms in smart grid operations such as proposed in (Mureddu et al., 2020) have become a hot topic in today’s research in energy systems utilizing blockchain and AI. (Said et al., 2022) builds focus on a unique framework that utilizes connected electric vehicles (CEVs) are deployed, and the peer-to-peer trading system powered by blockchain and AI are observed when encountered with cybersecurity attacks. Smart Distributed Energy Resources (DER) and energy-related parameters have been discussed in (Jin et al., 2022), and a model has been proposed to highlight the aspects of the system. Moreover, AI assists with computer algorithms, and deep learning is utilized to enhance decision-making. AI supports accurate demand forecasting and consumer analysis, while blockchain ensures data integrity and trust. The decentralized AI-driven ECM framework was validated through case studies, illustrating its effectiveness in enhancing energy management security and privacy.
Despite significant advancements, several research gaps remain. Safaei et al. (2022a) and Momenitabar et al. (2022a) developed models for CLSC networks, focusing on minimizing costs and improving service levels through demand forecasting using methods such as MILP, ARIMA, and FIS. However, these studies do not address the potential integration of AI with blockchain to enhance the accuracy and security of demand forecasting and supply chain optimization. Momenitabar et al. (2022b) and Momenitabar et al. (2023) showed the efficacy of AI in demand forecasting but did not explore the integration of blockchain to secure and validate the large volumes of data used in AI models. Goodarzian et al. (2023) addressed sustainable CLSC design using MILP and machine learning algorithms but did not explore blockchain’s role in enhancing data security and transparency. Research is needed to examine how blockchain can be integrated with AI and MILP models to ensure data integrity and enhance the sustainability of supply chains.
In energy management, frameworks combining AI and blockchain for smart grid automation and energy management, such as those proposed by Kumari et al. (2020) and Samuel et al. (2022), need further refinement to handle the increasing complexity and scale of modern energy grids. Blockchain-based energy trading systems highlighted by Hua et al. (2022) and Samuel et al. (2022) present innovative solutions but face challenges in scalability and efficiency. The integration of electric vehicles with VPPs, as explored by Chenli et al. (2021), requires efficient data transaction protocols to manage memory and latency issues. AI-driven ECM systems, as presented by Nakamoto (2008), need to ensure data privacy and integrity while maintaining trust and transparency. The proof-of-deep learning (PoDL) mechanism discussed by Chenli et al. (2021) addresses energy waste issues in blockchain but requires extensive real-world testing to validate its effectiveness and security.
General research gaps in the integration of AI and blockchain technologies in energy management highlight the need for novel and comprehensive coverage in literature reviews as the first attempt to cover both technologies in the energy domain. Previous reviews may have overlooked relevant studies and emerging topics, thereby not fully capturing the latest advancements and interdisciplinary approaches. Future reviews should include a broader range of studies and the latest research developments to ensure all relevant innovations are considered. There is also a noted lack of methodological rigor in some review papers, which undermines the reliability and validity of their findings. Adopting systematic review methodologies with clear inclusion and exclusion criteria and using both quantitative and qualitative analysis techniques are essential to enhance the quality of future reviews. Additionally, many reviews focus on theoretical aspects without providing insights into real-world challenges and applications. Future research should emphasize practical case studies, pilot projects, and applications to bridge the gap between theory and practice, highlighting the feasibility, challenges, and benefits of integrating AI and blockchain in energy management.
The main contribution of this paper can be summed up as follows.
We briefly introduce the basics of blockchain and AI, including their structures, taxonomies, and their fundamental features. We present our review of the major applications of the integration of blockchain and AI in multiple areas with some use cases. We discuss the applications of blockchain and AI in the energy sector and how this can help in forming a new ecosystem in the energy domains. In addition, we discuss the major benefits incurred in each. We report and discuss many novel use cases and systematically review works of blockchain–AI applications in the energy sector. Finally, we identify the outcomes of the study and the advantages and challenges associated in adopting AI–blockchain in the energy sector. The rest of the paper is organized as follows: Section 2 discusses the background of blockchain and AI technologies and their features; Section 3 presents our review of the integration of AI and blockchain and how this integration can help in shifting toward new ecosystems in different areas; Section 4 describes the individual applications of AI and blockchain in the energy sector; Section 5 reviews the novel research work on blockchain–AI integration and its applications in the energy sector; Section 6 analyzes the research outcomes; and finally, Section 7 discusses the conclusion.
2 Background
This section briefly introduces the basics of blockchain and AI and their fundamental features, structures, and taxonomies.
2.1 Blockchain
Blockchain was first introduced in late 2008 by Satoshi Nakamoto (pseudonym) (Goodarzian et al., 2023), who helped develop the first bitcoin software application and introduced the concept of cryptocurrency to the world. Unlike conventional centralized transaction systems (e.g., the central bank), blockchain is a technology that eliminates the middleman from digital transactions. It is a decentralized, open-source, public digital ledger technology (DLT) distributed across network peers (Nakamoto, 2008). Since then, blockchain has gained attention due to its anonymity, security, and data integrity features. Each block stores encrypted data, creating a chain of cryptographic hashes, ensuring that no third party can alter the information (Pilkington et al., 2016). Blockchain’s popularity has grown across various industries due to its advantages, with studies focusing on its applications. The technology operates through cryptographic hashes, digital signatures, and consensus mechanisms (Zheng Z. et al., 2018). Smart contracts play a crucial role in securing transactions, deciding whether to permit or trigger activities on the blockchain (Pilkington et al., 2016). These contracts, initiated by users, not only secure transactions but also automate processes on the blockchain (Pilkington et al., 2016). Visual representations can help better understand the architecture.
Essentially, blockchain comprises a continuous chain of locked blocks. Each block holds a permanent list of transactions from the ledger among participants involved in the blockchain network. Common consensus algorithms include PoW, PoS, and practical Byzantine Fault Tolerance (PBFT), which is a consensus strategy used in Bitcoin (Brundo and De Nicola, 2018), which calculate the hash value through the miner nodes (mining procedure). The calculated value must be equal or smaller than a certain value until one node reaches the target value, and the other nodes must mutually confirm the correctness of the hash value. Once the block is validated, other miners would add this new block to their own blockchain.
Blockchains are mainly characterized as permissioned and permissionless blockchains (Golosova, 2018). Permissioned blockchains restrict access to the blockchain of some nodes unless granted permission, and their identities are known to other users of that permissioned blockchain. Conversely, permissionless blockchains do not restrict any user from joining the blockchain and becoming a node in that network.
According to Wegrzyn and Wang (2021), there are four types of blockchains, namely, public, private, consortium, and hybrid blockchains (see Figure 2). Public blockchains are completely decentralized and permissionless. Unlike public blockchains, private blockchains are permissioned blockchains governed by a single organization. Similar to private blockchains, consortium blockchains are permissioned, yet they are governed by a group of organizations instead of a single entity. Finally, hybrid blockchains are a mix of private and public blockchains. They are controlled by a single organization but under certain levels of supervision executed by the public blockchains. Figure 1 shows the relations between the four types of blockchains.
Several studies have reviewed the key characteristics of the blockchain system. Wegrzyn and Wang (2021), Viriyasitavat and Hoonsopon (2010), and Xinyi et al. (2018) identified the technical and business characteristics of blockchain technology.
Decentralization: Contrary to the conventional centralized transaction systems that require validation through central trusted intermediaries (e.g., the central bank), blockchain is a promising solution that eliminates any third party, saving a lot of intermediary costs and enhancing the transaction performance and speed.
Persistency and immutability: Each node maintains and validates its records with nearly no room to rollback or delete transactions. In addition, it is not prone to any major attacks (Chatterjee and Chatterjee, 2017).
Auditability and traceability: Derived properties such as transparency and temper-proof features indicate that blockchains are auditable. They enable users to easily track and verify previous records through nodes in the network.
Validity: In blockchains, only valid transactions would be executed by other nodes. So, any invalid transactions could be detected and deleted instantly.
Anonymity: Blockchain enables users to interact with its network without revealing their actual identity. A user can have more than one identity to avoid identity exposure (Yeow et al., 2018b).
Programmability: The blockchain system enables users to create sophisticated SCs for decentralization applications through a flexible script code system.
2.1.1 Transaction on the blockchain
Integrating blockchain technology in the energy sector requires us to define the process of initiating and processing transactions in the blockchain, which involves explaining the technical basis behind it. To perform a transaction on the blockchain, a user typically should start initiating the process, which then will be redirected to signing a transaction. Each user on the blockchain has a private and a public key, and private keys are used to sign transactions (Zheng Z. et al., 2018). After the confirmation (the signature) of the user, the transaction details will be created and added to the block. We can divide the transaction process into four steps: creation, propagation, validation, and confirmation (see Figure 3) (Belotti et al., 2019). The creation step is the first step in creating the transaction and the first step to initiate the process. In this step, the sender must define the transaction details of the “object” that is going to be sent on the blockchain, such as the origin and the destination of the asset (Belotti et al., 2019). Examples for blockchain assets (objects) can be digital assets, such as BTC or non-fungible tokens (NFTs). For the sake of our research, we instead focus on an energy-utility token, which will be used in the industry. The propagation step involves transaction details being transmitted to validating nodes. By the time the creation step is completed, transaction details should be fully visible to the validating nodes. Validator nodes are similar to participants in the network who are responsible for validating transactions and their details. After validator nodes approve of the transaction details, the process continues to the validation step. This step is important, and it is reflective of the true nature (consensus mechanism) of the blockchain. A consensus mechanism is similar to the working nature of the blockchain. The validation step evaluates whether the transactions are true to the character of the blockchain, valid, and executable (Belotti et al., 2019). Upon approval, the block consisting of transaction details can be added to the blockchain, which will then update itself (Belotti et al., 2019). Another transmission step is made before the final step of adding the block to the blockchain, so that validator nodes can update their own copy (Belotti et al., 2019). The confirmation step is the final step in the transaction process. In this step, once validated and published on the blockchain, the block can be fully integrated to the heart of the blockchain, and the consensus mechanism is fully executed. It is important to emphasize that transactions are not always visible to the public.
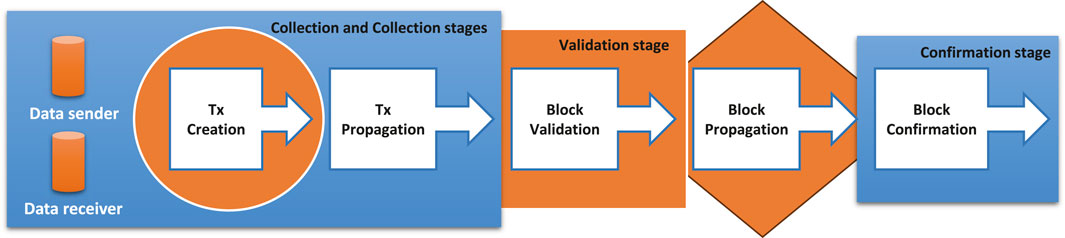
Figure 3. Journey of a transaction through different steps in the blockchain. Nodes are responsible for validating transaction details (Belotti et al., 2019).
2.1.2 Energy transaction process
Using blockchain technology for energy payments has the potential to transform the energy sector. Efficiency and security are crucial for facilitating energy transactions—efficiency ensures productivity and speed, while security offers privacy and decentralization. Traditional energy transactions, although straightforward, have limitations such as time consumption, low security, and high costs due to centralized services (Zhao et al., 2018). Implementing blockchain for energy transactions is technically complex, but smart contracts play a key role. Smart contracts bring functionality to transactions, allowing users to initiate the process securely. They store data such as energy type (e.g., heat and electricity) and transaction details on the blockchain, enabling secure execution. The combination of blockchain and smart contracts facilitates complex energy exchanges (Zhao et al., 2018).
A user initiates a blockchain transaction, deploying smart contracts to manage the process. System availability and technical requirements should be verified, with AI assisting in this assessment. Smart contracts, which are agreements between the buyer and seller without intermediaries, confirm the success of the transaction. In energy transactions, a native blockchain token may be used for payments. If the buyer meets technical requirements and has sufficient token credit, the contract is signed, and the transaction is completed. For example, as shown in Figure 4 and as detailed by Zheng et al. (2020), the process can be divided into three contracts: contract 1 involves the restaurant offering its menu and the customer making a selection; contract 2 covers the preparation and serving of the meal; and contract 3 handles the payment. Contract 3 is triggered automatically once contract 2 is completed. If the customer has sufficient funds, the smart contract is valid; otherwise, it is revoked.
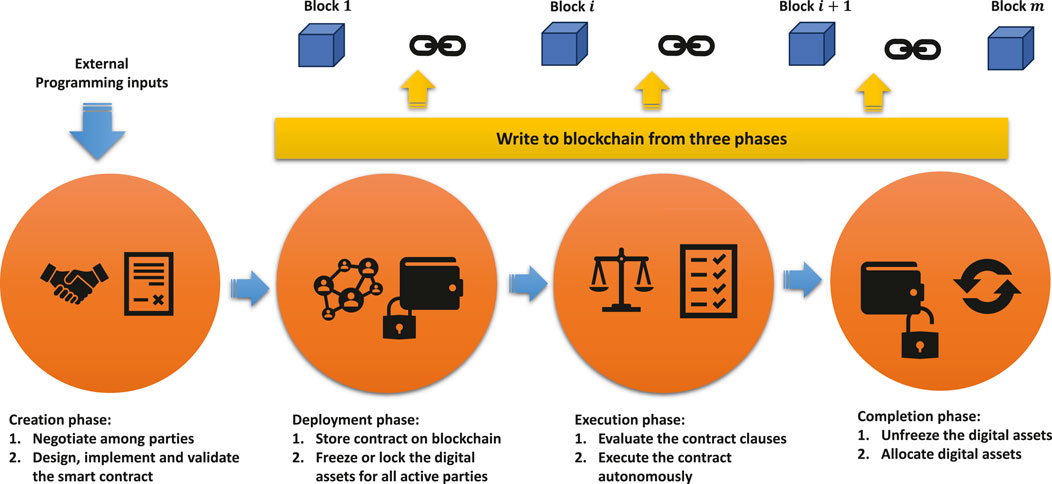
Figure 4. Cycle of smart contracts consisting of four essential phases (Zheng et al., 2020).
In our energy transaction example, if the buyer has sufficient credit, the energy transfer process begins (Di Silvestre et al., 2018). The system efficiency relies on the input and output power. The transaction can be broken down into three contracts: contract 1 involves offering energy types and taking the customer’s order; contract 2 triggers the grid to deliver the specified amount of energy, with AI expected to enhance automation; and contract 3 handles payment approval. If the credit is sufficient, the smart contract is validated and energy is transferred. If not, the purchase is canceled, and no energy is transmitted.
Blocks in the blockchain, as shown in Figure 5, store transaction details such as total power, input, output, and efficiency, providing immutable and secure data storage (Hu and Li, 2021). In a private blockchain, this information remains inaccessible and unchangeable. Nodes in the blockchain, which play roles from buyers and sellers to passive participants, uphold the network’s consensus mechanism and ensure its stability. They are crucial for network growth and efficiency. Implementing tracing systems to monitor microgrids (MGs) and power grids for power loss enhances system efficiency. Blockchain-recorded values offer reliability and trust for both customers and providers. Smart contracts that detail and manage essential information open new research opportunities and benefit corporations and government agencies in improving power systems. However, analyzing complex systems, particularly the generator–load relationship, poses challenges. Physical checks may be necessary to verify the system’s ability to handle transactions (Sanseverino et al., 2017).
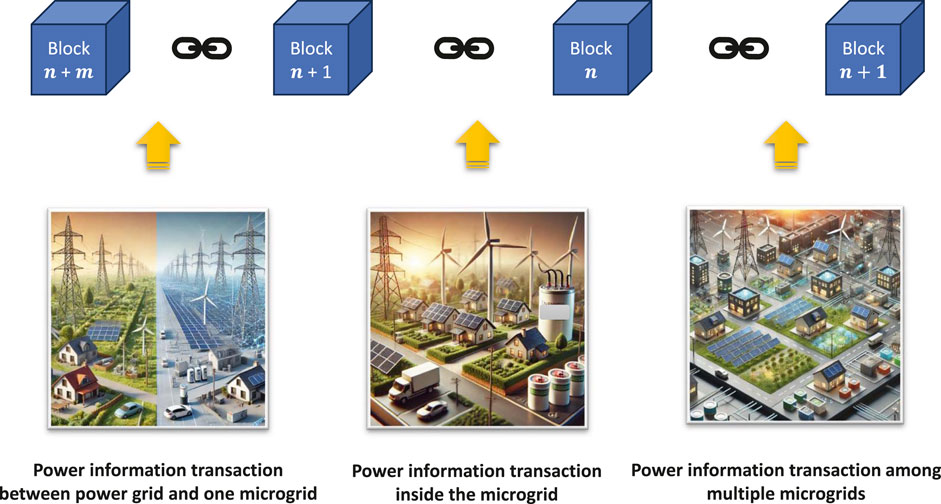
Figure 5. Model regarding information stored in blocks (Xu et al., 2019).
2.1.3 Latest development
Blockchain technology has revolutionized the energy sector since its inception in 2008, becoming a pivotal tool for industries. Especially since 2018, significant research has focused on integrating blockchain with energy management and transactions, particularly in renewable energy rather than fossil fuels (Wang and Su, 2020). The concept of “energy blockchain” highlights the advantages of blockchain, including security, privacy, decentralization, and faster transactions (Boumaiza and Sanfilipp, 2022). Smart contracts, initially proposed by Nick Szabo in the 1990s, now play a vital role in decentralizing energy systems. Encryption algorithms, such as SHA256, remain central to energy transactions, although alternatives such as SM3 or MD5 are also viable. The inclusion of AI offers further opportunities for the energy sector.
According to Wang and Su (2020), key research topics include “blockchain technology,” “smart grid,” “Internet of Things (IoT),” “energy system,” “intelligent connection,” and “energy commodity trading.” Recent advancements in blockchain technology impact its integration in the energy sector, with a focus on renewable energy applications. Smart grids and connectivity are prominent research areas, reflecting a shift toward reducing carbon emissions and enhancing sustainability. Notable developments include P2P power trading microgrids, solar power surplus trading systems, oil and gas trading platforms, and blockchain e-wallets. The rise of IoT, AI, and smart devices has added complexity to power grid management, with P2P networks becoming increasingly central to energy transactions (Teng et al., 2021). Figure 6 shows different application scenarios of blockchain technology (Wang and Su, 2020).
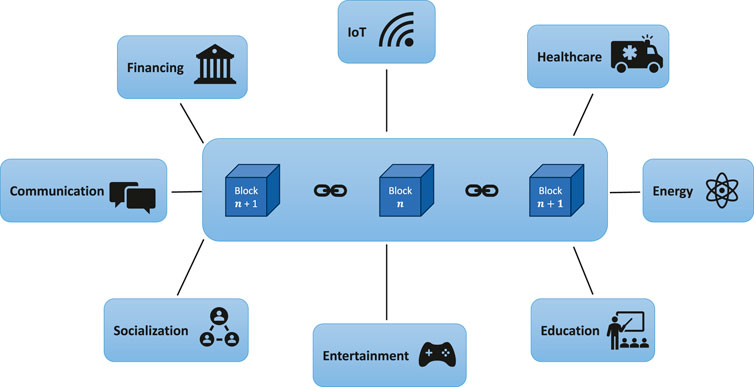
Figure 6. Diagram depicting application scenarios of blockchain technology (Wang and Su, 2020).
Over the years, publications have explored distributed grid operations, decentralized energy trading, carbon trading, green certificates, and information security (Figure 7). Energy management, including technologies such as microgrids and load aggregators, is a growing research field (Teng et al., 2021). PoW blockchains, notably Bitcoin, are power-intensive compared to PoS blockchains. However, electricity consumption is expected to decrease with each Bitcoin-halving event (Sedlmeir et al., 2021). The energy sector aims to adopt more efficient, ecofriendly blockchains for applications such as renewable energy sources, BEV batteries, solar energy, microgrids, and P2P trading, which require secure data storage and transactions (Yap et al., 2023).
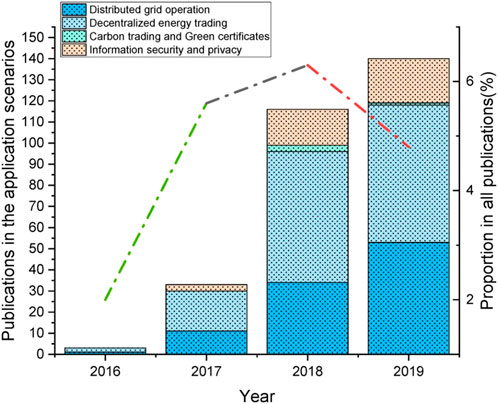
Figure 7. Annual number of related blockchain-based publications and their proportion among all publications (Teng et al., 2021).
2.2 Artificial intelligence
The term “artificial intelligence” (AI) was first proposed in 1956 by John McCarthy in an attempt to academically describe modern AI. In a systematically analyzed study (Monett et al., 2019), researchers proposed a modern definition of AI according to a set of criteria: “adaptation with insufficient knowledge and resources.” As the definition implies, AI is a system that will react not worse than a human in an arbitrary world (Dobrev, 2012); AI systems can comprehend, learn from experience, reason, and solve problems exactly like the human intelligence displays. However, AI has some advantages over humans, which are as follows: (1) it can perform multiple tasks faster and simultaneously and (2) can easily perform complicated tasks with fewer errors in less space (Khanzode and Sarode, 2020).
The historical evolution of AI shows a trend toward full-system automation, enhancing efficiency for corporations, government agencies, or businesses. Today, large-scale AI language models continuously improve as they interact with users. In the energy sector, integrating AI with blockchain technology is essential for fully autonomous energy management. AI’s ability to learn and adapt through machine learning—an imitation of human learning using vast data and algorithms—underscores its growing capabilities. AI is mainly categorized into three main areas: problem-solving, which involves calculations and finding solutions; pattern recognition, which classifies objects and applies logical reasoning; and machine learning, which uses large datasets and algorithms to expand AI applications (Hunt, 2014).
AI continually learns and automates systems through machine learning, a key branch of AI that uses data to train models. By integrating data and modeling responses, AI can act like humans, managing smart homes and power grids effectively. This involves handling transmission details, power loss, and other related parameters to improve AI’s actions. Blockchain data—such as transaction details—support accurate energy management, allowing AI to optimize transactions and create fully autonomous systems. Demand-side management (DSM), as proposed by Rocha et al. (2021), uses daily energy price curves to reflect demand and supply, with AI bridging these aspects to streamline operations and automate processes.
An IoT platform is crucial for accessing and managing smart devices and technologies, connecting seamlessly with AI. It controls processes such as connection requests, device activation, and energy management while efficiently handling and transmitting data for valuable insights (Li et al., 2023b) (see Figure 8).
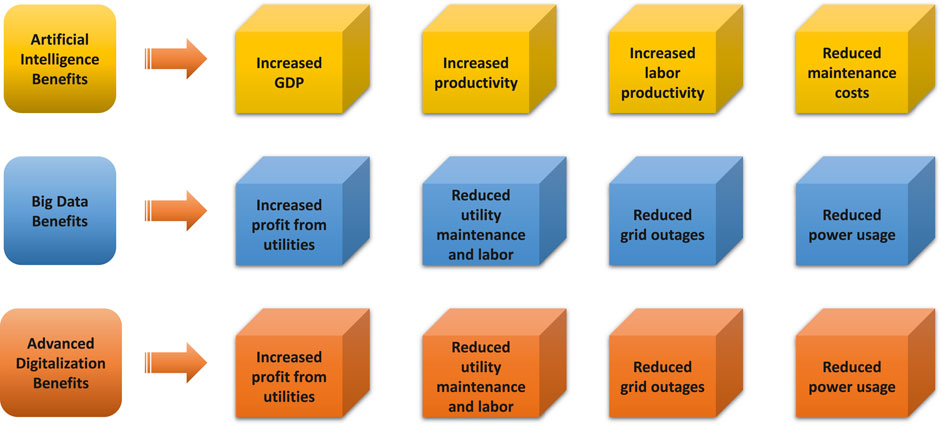
Figure 8. Image explaining the benefits of AI, big data, and advanced digitalization, which are the largest contributors to achieving energy management (Li et al., 2023b).
Understanding energy management with AI involves studying its application areas. The home energy management system (HEMS) proposed by Ma et al. (2021) highlights AI’s role in household systems, emphasizing the need for decentralization and automation. This model integrates distributed power technologies such as energy storage and photovoltaic systems to manage electricity usage intelligently. AI can then control household devices to optimize energy use. The IoT connects smart gadgets, allowing AI to capture and evaluate energy consumption details. The HEMS regulates household energy, reducing electricity costs and improving efficiency. It is crucial that all energy-consuming devices are compatible within this smart ecosystem.
2.2.1 Types of AI
Majorly, AI is classified into six sub-fields:
Machine learning (ML) is a primal subset of AI and has emerged as a result of combining computational learning theory and pattern recognition, which is applied in data analytics (Angra and Ahuja, 2017). As ML executes more assigned tasks, it learns from its past experiences and gradually improves its forecasting performance based on a set of data (Ray, 2019). ML uses different programmed algorithms that receive and analyze input data to make predictions or decisions in the form of output values within a certain data range. Some ML algorithms are linear classifier, logistic regression, naïve Bayes (NB), Bayesian network, support vector machines (SVMs), decision tree, random forest, k-nearest neighbor (k-NN), and artificial neural network (ANN) (Shinde and Shah, 2018). ML approaches are classified into supervised learning, un-supervised learning, semi-supervised learning, and reinforcement learning. Table 1 explains the difference between each approach (Kang and Jameson, 2018). Some ML applications are prediction, natural language processing, semantic analysis, computer vision, and information retrieval (Rincy and Gupta, 2020).
1. ANNs are computing systems inspired by biological neural networks that exist in the brain of living beings (Jain et al., 1996). The ANN is a subset of ML consisting of multiple layers (input, hidden, and output) grouped by artificial neurons, similar to biological neurons. Artificial neurons are elementary units in the ANN, representing a mathematical function that is made up of three parts: input, activation function (in the hidden layers), and output. Figure 9 shows the multilayer perceptron (MLP) diagram, which consists of the three parts. The ANN is a data-driven algorithm that learns from a dataset and tries to explore hidden functional relations between input data and output data. These relations could be complex and nonlinear in nature. The ANN is a powerful tool used for pattern recognition, forecasting, signal processing, classification, regression, and data analysis (ArulRaj et al., 2021).
2. Deep learning (DL) is a subset of machine learning. However, it distinguishes itself from ML in terms of the learning approaches and type of data it deals with based on the ANN. DL follows hierarchical category structure of data, making it ideal for discovering complex structures in high-dimensional data such as medical imaging (Djavanshir et al., 2021). DL is applicable in a wide spectrum of AI tasks, especially in supervised learning for image processing, computer vision, pattern recognition, neural language processing, imitation learning, and multimedia applications (Cao, 2022).
3. Natural language processing (NLP) is an important sub-field of AI concerned with achieving reliable and effective communication between human and machine natural language (Jiang and Lu, 2021), enabling computers to comprehend the natural way of human communication. The use of ML and DL in the learning process of NLP has significantly enhanced the accuracy and efficiency of the tasks handled in applications such as speech recognition, business analytics, auto prediction and correction, translation, and social media (Nagarhalli et al., 2022).
4. Computer vision can resemble NLP since it deals with deriving, processing, and analyzing data. However, computer vision enables computers to identify and process data in the form of objects seen in images and videos by imitating the natural way. Computer vision has been used in many modern applications in different areas including healthcare (X-ray analysis and cancer detection), transportation (pedestrian detection), manufacturing (defect inspection), and agriculture (insect detecting).
5. Cognitive computing is the simulation of the human thought process in a sophisticated way where answers may be uncertain or complicated (Megha et al., 2017). Cognitive computing can not only support human–machine interaction but also introduce a kind of emotional interaction between humans and robots. Moreover, cognitive computing can assist in diagnosing and making decisions to perform suitable operations for the patient based on given data.
2.2.2 Latest development
The latest developments in the field show that intelligent energy management systems are gaining popularity. With intelligent management systems, efficiency is greatly achieved, which results in low costs and optimal energy usage in commercial, industrial, and residential sectors (Ali et al., 2021). Machine learning and energy management in hybrid electric vehicles (EVs) were researched, which indicates that scientific effort is put in applications of management systems in different areas of renewable energy.
Moreover, some interesting research areas in the field are IoT-based blockchain-powered platforms for distributed energy management, machine learning for enormous energy data, P2P energy trading and demand-side energy management systems, future computing technologies, Internet of Energy (IoE)-based energy management systems, and intelligent forecasting models (Ali et al., 2021). There are applications involving AI with hydrogen energy, with topics ranging from production to demand and control (Ahmad et al., 2021). Smart grids and conventional grids differ in use cases and technologies. Conventional grids typically transmit energy in a one-way direction without any extra technology involved. These grids are the standard way of delivering energy to industrial, commercial, and residential zones. However, smart grids are one of the main topics of research regarding AI in energy management systems. Smart grids combine IoT and AI to distribute energy loads; they consist of a different infrastructure from conventional grids. Different types of energy sources can be transmitted through smart grids with the integration of AI, and large datasets can greatly contribute to the development of intelligent management systems (Ahmad et al., 2021). Controlling energy fluctuations has become one of the primary purposes of AI integration in management systems, and IoT is the father ecosystem making this possible. Figure 10 shows the challenges of implementing intelligent energy management systems (Ali et al., 2021). The latest research indicates that power demand is increasing rapidly, and developing the most efficient energy management system can be challenging (Shafiullah et al., 2022). The basic view of a generalized microgrid is shown in Figure 11.
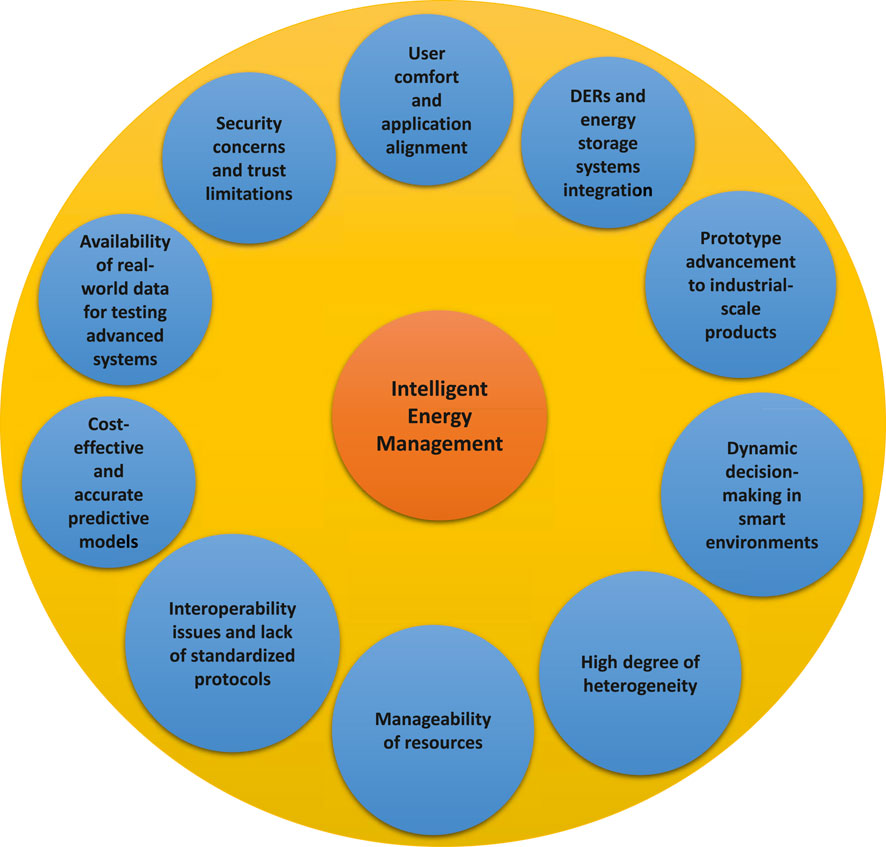
Figure 10. Challenges of implementing intelligent energy management systems (IEMSs) (Ali et al., 2021).
An innovative architecture proposed for microgrids will increase efficiency, further promoting innovation and development. Renewable energy such as solar energy has become a hot topic in the latest research. For example, Hamidi et al. (2023) focused on integrating solar energy in UAE buildings based on AI, and Ahmad et al. (2022) proposed many applications of AI in the energy sector, discussing different perspectives of its integration in the industry. Mischos et al. (2023) discussed the advantages and disadvantages of intelligent management systems that regulate our energy consumption, moreover elaborating on how these systems can reduce the carbon footprint and diminish climate change effects. Many research papers focus on implementing green energy with AI management systems, indicating a future with renewables. Altin and Eyimaya (2023) explained the implementation of AI combined with microgrids and renewable energies, with an introduction of the advantages of microgrids backed by energy management systems and further developing the narrative on AI.
3 AI–blockchain applications
In this section, we present the major frameworks reported in the literature on integrating AI and blockchain in different application areas.
3.1 Healthcare
Recently, the healthcare sector has seen a rapid growth in technology advancement as blockchain and AI together provided progressive solutions to many challenges associated with the smart healthcare systems (E-health systems). AI, with its outstanding ability to recognize patterns, analyzes data and makes optimal decisions, and blockchain ensures the security and legitimacy of the data. Cognitive computing, as a subset of AI, transforms interactions between humans and machines within the healthcare sector. It achieves this by providing personalized treatment plans through in-depth analysis of patient data, precise medical diagnostics using AI-driven systems, and improved patient engagement via virtual assistants (Lee and Yoon, 2021). These advancements are complemented by blockchain technology, which ensures the secure and transparent handling of healthcare information. Blockchain technology safeguards patient records, supports confidential telemedicine sessions, and facilitates the transparent management of clinical research data. Additionally, blockchain technology enhances the integrity of pharmaceutical supply chains by verifying the authenticity of medications and preventing the circulation of counterfeit drugs (Daniel et al., 2017). As discussed by Jabarulla and Lee (2021), and as the COVID-19 outbreak unfolded, a conceptual framework based on blockchain–AI integration was presented in response to the pandemic. The system can contribute in four ways: (1) the data exchange between stakeholders, e.g., government, researchers, pharmacies, and payers; (2) patients can securely store and retrieve their health records on the patient-centric platform; (3) the blockchain eliminates centralized organizations to reduce soiled patient datasets, and AI provides predictive diagnosis and analysis; and (4) a global model is created, and its parameters are shared with the hospital management, while model training is performed locally there. Thus, the global models are trained in the distributed network while maintaining hospital and patient data privacy.
3.2 Agriculture
Integrating AI and blockchain technology offers innovative solutions to key agricultural challenges such as land scarcity and excessive pesticide use. AI-driven precision farming leverages data from satellite images, weather patterns, and soil sensors to provide specific farming recommendations, enabling better land use and addressing land scarcity (Torky and Hassanein, 2020). Additionally, AI systems can monitor crops in real-time, detect early signs of pest infestations or diseases, and apply pesticides only where necessary, significantly reducing their usage and minimizing environmental impact (Chen et al., 2023). Blockchain technology enhances transparency in the agricultural supply chain by recording every transaction in an immutable ledger, making it possible to trace the origin, quality, and handling of products. This transparency allows consumers to verify sustainable sourcing and ensures regulatory compliance. Blockchain-enabled smart contracts automate agreements between farmers, suppliers, and buyers, ensuring that commitments are met and promoting fair compensation for farmers. Moreover, the blockchain can create transparent and secure land registries, which are crucial in regions prone to land disputes, and help farmers access credit by using their land as collateral. Combining AI and blockchain also optimizes resource management: AI predicts the best times for irrigation and fertilization, while blockchain records and verifies these actions, leading to more efficient resource use and sustained land productivity (Chen et al., 2023). Land scarcity, excessive use of pesticides, and global food demand are the main challenges in the agriculture sector. However, the emerging concept of the smart agriculture/smart farming, which refers to the use of key enablers technologies (e.g., IoT, AI, and blockchain) in cultivation, has increased the quality and quantity of crops and optimized the productivity of the labor working in the farm. Jadav et al. (2023) proposed a blockchain and AI-based smart agriculture framework that efficiently predicts human life expectancy based on pesticide usage in food. They built different AI models based on data obtained from crop pesticide datasets. AI models were adopted to classify human life prediction based on pesticide consumption with the best model predication performance. Ultimately, they stored correctly predicted agricultural data inside the IPFS-based public blockchain to enhance the privacy and security of the proposed framework.
3.3 Manufacturing and supply chain
With the rapid evolution of the industrial sector toward cyber–physical systems, disruptive technologies such as blockchain and AI have introduced radical shifts in the fourth industrial revolution (IR 4.0). The amalgamation of AI and blockchain carries enormous potential to create digitalized business models. Moreover, supply chains integrated in the business are vulnerable to various types of security and privacy threats. For instance, in vaccine supply chains, Yong et al. (2020) proposed a blockchain-based intelligent system in which blockchain detects expired vaccines and AI provides vaccine recommendation information to different users. Furthermore, AI and blockchain technologies are revolutionizing manufacturing and supply chains, particularly in vaccine distribution and in the context of the fourth industrial revolution. AI’s predictive maintenance capabilities forecast equipment needs, reducing downtime and optimizing production schedules crucial for vaccine manufacturing. Quality control benefits from AI-driven computer vision and machine learning, ensuring that vaccines meet stringent standards. AI also enhances supply chains by analyzing real-time data to manage inventory efficiently and optimize transportation routes, improving vaccine distribution effectiveness (Safaei et al., 2022b). Blockchain technology ensures transparency and traceability across the vaccine supply chain, preventing counterfeit products and maintaining conditions such as temperature-sensitive storage through smart contracts. It facilitates secure data sharing among stakeholders—manufacturers, distributors, healthcare providers, and regulators—enhancing collaboration and decision-making during vaccine distribution (Musamih et al., 2021). Integrating IoT, automation, and digital twins further optimizes manufacturing processes, while machine learning in predictive maintenance supports IR 4.0 by monitoring the equipment health to minimize downtime and extend lifespan through advanced analytics and automation (Cinar et al., 2020).
3.4 Financial transactions
Blockchain technology operates on a decentralized network (Patel et al., 2018), unlike traditional systems that rely on a central authority. This decentralization means that there is no single point of failure, making the system more robust and less vulnerable to attacks or fraud. Each transaction is transparently recorded on the blockchain and becomes immutable once added to a block and linked in the chain. This ensures that records remain accurate and trustworthy, which is vital for verifying signatures. Cryptographic security (Li et al., 2017) is a core feature of blockchain, with each user possessing a pair of cryptographic keys: a public key (serving as an address on the blockchain) and a private key (used to sign transactions). This setup ensures that only the rightful owner can authorize transactions. The validation of transactions relies on consensus mechanisms such as PoW or PoS, requiring multiple network participants to agree on the validity of transactions, thus preventing fraud. The blockchain also enhances efficiency and speed through smart contracts, which are self-executing contracts with the terms written directly into code. These contracts automate the verification of signatures and execution of transactions, making processes faster and more efficient (Hazari and Mahmoud, 2020). Additionally, the blockchain provides a comprehensive, real-time audit trail of all transactions, simplifying the verification of their history and authenticity, which is important for compliance and regulatory purposes. Either through the conventional way or cryptocurrency, intentional illicit transactions remain the main challenge in online transactions as there is a high level of anonymity (Yin et al., 2019). Thus, it is hard to trace the identity of illegal users and their transactions as well. To tackle this issue, different solutions were proposed by authors. For instance, a system founded on the amalgamation of AI, blockchain, IoT, and online signature verification was proposed (Jain et al., 2019). The transactions in the system are executed by dynamic handwritten signatures using a specialized pen implanted with an accelerometer and a gyroscope. Ultimately, the identity of users is verified, and handwritten signatures are authenticated through an algorithm named “dynamic time wrapping” algorithm.
3.5 Transportation
Luckily, the convergence of AI and blockchain has effectively addressed current transportation issues. For instance, Reebadiya and Gupta (2022) proposed a novel solution to deal with issues related to peak cruising time, security, and illegal parking of the vehicles. They introduced intelligent architecture based on AI and blockchain to predict parking availability and parking pricing rate, which is decided based on vehicle type and peak time (nonpeak time). Thus, this will contribute to decreasing traffic congestion and increasing profit of the parking service providers.
3.6 Smart city and buildings
The integration of AI and blockchain technologies in smart cities (See Figure 12) and buildings presents substantial benefits across data accuracy, security, privacy, efficiency, and transparency. AI utilizes advanced machine learning algorithms to analyze real-time data from IoT devices, offering precise insights into energy consumption and resource utilization. Simultaneously, the blockchain ensures data integrity by maintaining immutable transaction records across distributed networks, safeguarding sensitive information such as energy usage data from unauthorized alterations (Ahmed et al., 2022a). In terms of security, AI enhances surveillance and access control systems by detecting anomalies and potential threats, complemented by the blockchain’s decentralized and encrypted architecture that provides robust protection against data tampering and unauthorized access. Furthermore, AI anonymizes and aggregates data to protect individual privacy while extracting valuable insights, such as analyzing traffic patterns without compromising personal identities. The blockchain reinforces privacy by securely managing data transactions through encrypted keys, ensuring transparency and user control over data access. Additionally, AI optimizes operational efficiency by predicting equipment failures via predictive maintenance, while the blockchain enhances transparency by securely recording transactions, thereby fostering trust through transparent audit trails (Badidi, 2022). In addition, the proliferation of new information and communication technologies, e.g., IoT, big data, sensors, smart meters, and controllers, as shown in Figure 3, has set the concept of smart city (SC) and smart buildings (SBs) as a new era of a revolutionized way of living. Despite all the deficiencies pertaining designing big data analysis in the SC networking, it has become a challenge in terms of presenting accurate data in real-time applications. In addition, data security and privacy and the limits of centralization remain major challenges in data exchange. To overcome such issues, Sharma et al. (2021) proposed AI and blockchain-based decentralized architecture with a 6G-enabled IoT framework to achieve secure and scalable transactions in IoT at device, cloud, and edge intelligence. The experiment showed that the proposed architecture could efficiently obtain high performance in terms of accuracy, security and privacy, and inversely less latency.
4 Sole applications of AI and Blockchain in the energy sector
In this section, we discuss challenges associated with the adoption of renewable energy sources (RESs) in the distribution power system and how the integration of AI with blockchain can help overcome these challenges and transform the energy sector into a new era.
4.1 Applications of AI in the energy sector
AI has been increasingly playing a pivotal role in the energy sector, particularly in the renewable energy (RE) industry, as it deals with a complex and large dataset. According to many research studies, AI can be leveraged to optimize the operation and control of the RE systems. AI is also a great tool for better monitoring and maintenance of the RE systems when integrated in national power systems.
Demand response (DR): AI can be used to manage the energy supply and demand by predicting the energy demand of the consumers and adjusting their needs (peak loads) according to the energy production peaks. At the time of low-energy production (demand is more than supply), neutral (decentralized) RE-based suppliers would request energy from the national grid and return extra energy to the grid when they produce more than they consume in a continuous and reciprocal manner between the prosumers and the consumers. DR is one of the techniques that enables the consumer to reduce their energy demand in response to supply constraints under some incentives. AI analyzes historical energy consumption data and real-time smart grid information to accurately predict the demand and reduce peak loads. It automates responses by adjusting smart appliances, dynamically managing settings to stabilize the grid during peak usage. In managing energy storage, AI-driven predictive analytics monitor system performance, predicting maintenance needs and optimizing operations for greater efficiency (Al-Fatlawi et al., 2024). AI also enhances energy storage efficiency by forecasting renewable energy generation patterns and optimizing charging and discharging schedules to maintain grid stability. Blockchain technology complements these efforts by maintaining immutable transaction records that prevent data tampering and ensure the accuracy of energy transactions. Its smart contracts automate energy transactions based on predefined conditions, promoting transparency and eliminating intermediaries (Junaidi et al., 2024).
DR originated from the historical concepts of DSM or energy service management (ESM). Khan M. et al. (2023) thoroughly investigated more than 200 articles, businesses, and projects, and they concluded that AI presents a potential tool for DR, and its usage is a guarantee for a successful future in the energy industry. In addition, they showed that ANNs are widely used for short-term load (STLF) and price forecasting.
Smart storage: energy storage technology (EST) became an integral key feature for RE systems as they connect RESs with the smart grids and provide a reliable solution for the issue of volatility and demand fluctuations originating from the solar and wind energy production behavior. Ferrag and Maglaras (2020) studied various EST applications in a smart grid in detail. They discussed the main challenges that occurred in the integration of RESs in the power systems, such as intermittency, reliability, safety, and reverse power flow issues, and how EST can primarily contribute to tackle these issues. Many works have showed that AI can successfully tackle these issues and maximize profit. AI is reshaping investment in energy projects as it would maximize the return on investment, and both energy producers and consumers can enjoy very competitive prices and energy resource availability in the energy market.
Centralized smart control systems and microgrids refer to the ability of the managing platform to deal with the exponential growing data in MGs using AI. MGs are small-scale power systems that connect a group of components such as local distributed generators (DGs), local loads, and ESTs that work independently. DGs differ from traditional power systems in that they (1) use RESs effectively, (2) are energy efficient as they are near the transmission lines, (3) have higher power quality as they use inverters, and finally, (4) better utilize conventional energy (Sabri et al., 2019). Integrating AI in the centralized control system will help prevent any sudden decrease in energy production by identifying errors in a timely manner and reducing their repair time spans. In general, the applications of AI techniques in MGs are (1) energy management systems (EMSs), (2) consumer LF on the grid, (3) forecasting generation curves for PV and wind power, (4) online fault diagnostics, protection schemes, and fault-tolerant control, (5) cyberattack detection, (6) sensor-less robust estimation of feedback signals, (7) noise and delay-less filtering of signals, (8) neural network modeling of static and dynamical systems, (9) intelligent scheduling of generation and storage, (10) high-performance intelligent control of system elements, and (11) real-time pricing predictions of electricity with demand-side management (Mohammadi et al., 2022).
4.2 Applications of blockchain technology in the energy sector
With the rapid development of energy systems characterized by the introduction of microgrids and smart grids (SGs), serious concerns are being raised over the security, reliability, and privacy of modern energy systems (Yan et al., 2017). Blockchain technology has proven to effectively deal with such issues. Benefiting from its prominent decentralization feature, the blockchain can be deployed to provide a decentralized energy system that prioritizes customer satisfaction, secure energy markets, and transparent transactions (Al-Abri et al., 2022). Decentralization strengthens system resilience by reducing the dependence on centralized authorities, thereby enhancing reliability against cyber threats and disruptions (Patel et al., 2018). Blockchain also secures energy data integrity through cryptographic verification across distributed nodes, safeguarding consumption and production records from unauthorized access. As renewable energy systems expand and interconnect, the blockchain’s robust consensus mechanisms and encryption protocols play a critical role in enhancing cybersecurity, addressing vulnerabilities in an increasingly interconnected energy landscape. Table 2 shows the major applications of the blockchain in the energy domain.
EMS: The blockchain is a perfect tool that can detect fraud and provide a transparent environment for all users in the energy systems (Chen et al., 2022). Dargan et al. (2021) proposed a comprehensive model for a blockchain-based energy management system that includes all energy sources and stakeholders. The model executes transactions and incentivizes the use of clean energy by generating certificates/tokens.
Energy trading—P2P: In energy trading, the concept of P2P (interchangeably known as a shared economy) has evolved to encompass a group of participants (e.g., consumers, prosumers, and generators). The P2P scheme allows energy exchange operation between peers, prosumer and consumer, directly (without a third party) at prices lower than the retail prices to encourage the prosumers to sell their surplus energy in order to balance the distributed energy generation (Soto et al., 2020). Trejo (2020) presented a blockchain-based P2P energy trading model that aims to reduce loads on the substations at peak hours under certain constrains while enabling P2P energy trading through a radial low-voltage-based smart contract.
SG refers to the electricity supply network that includes a variety of advanced information and control infrastructure (e.g., computers, control, and automation). According to Bouckaert et al. (2021), the blockchain can be used to enhance the cybersecurity against potential cyberattacks. Thus, the blockchain can provide sufficient protection for data when they are stored in its network, validated, and verified for further data usage in the smart grid, such as load forecasting. Once the data have been stored in the blockchain network, it would be almost impossible to alter or delete them.
EVs: According to the International Energy Agency (IEA), it is estimated that the global EV volume will increase by more than 30% of the global market share by 2030. The large volume implies that EVs will have an adversely high impact on power systems. Interestingly, Al-Saif et al. (2021) presented several exemplary blockchain-based use cases of the EVs in energy trading. They identified multiple key features of the blockchain in EV energy trading, such as immutability, security, and accountability. The integration of AI and blockchain technology enhances the efficiency and security of energy trading by combining their respective strengths. AI models are capable of forecasting energy consumption and load profiles, which helps in optimizing energy resource utilization and ensuring consistent performance. The IoT platform, consisting of edge, fog, and cloud layers, connects AI with various hardware and software systems, improving data transmission and storage. Blockchain technology, when embedded in the cloud layer of the IoT platform, guarantees data security and integrity, enabling transparent and tamper-proof energy transactions. Moreover, the use of the blockchain in cryptocurrency can facilitate secure transactions within the energy sector (Li et al., 2023b).
The integration of blockchain and AI in P2P systems is essential for improving security, transparency, and efficiency in decentralized transactions. Blockchain technology offers a secure, unchangeable ledger for transactions, while AI optimizes decisions through real-time data analysis. In the energy trading sector, AI predicts energy demand and supply, and blockchain technology ensures the integrity of transactions. This combination reduces costs and enhances system responsiveness and reliability. Nguyen (2020) investigated the bidirectional energy exchange between EVs and road lanes embedded with wireless power transfer technologies, known as wireless charging–discharging lanes (WCDLs). This innovative system enhances grid services by balancing energy supply and demand while providing convenience for EV users as it eliminates the need for cables and stops. The study introduces a decentralized P2P trading mechanism where EVs and WCDLs directly negotiate energy prices and amounts. This negotiation is guided by an optimization problem that aims to minimize private costs for both parties and is secured by a privacy-preserving consensus protocol. Furthermore, the paper proposes an analytical method for selecting cost function parameters to ensure successful trading. The effectiveness and scalability of the proposed algorithm are validated through simulations, confirming the viability of this approach.
Energy storage: A blockchain-based energy storage sharing platform between individuals or corporations is perhaps the right solution to help stabilize the electricity grid by creating flexible and a real-time “on-demand” provision of energy. Lu et al. (2020) designed an energy storage sharing platform based on blockchain technology to cope with the limitations that exist in the energy storage demand and resources. A bidding mechanism was introduced in the energy joint market through the P2P energy storage sharing mechanism. The simulation of the results proved the efficiency of the proposed mechanism.
Carbon emission: The Environmental Protection Agency (EPA) estimates that more than 80% of greenhouse gases released are carbon emissions, primarily in the form of CO2 (Khezami et al., 2022). Effah et al. (2021) proposed a blockchain and IoT-based framework on the FISCO BCOS platform to monitor and calculate carbon emissions produced by business corporations. The volume of carbon emissions is paired off with carbon credit trading using a smart contract, while the framework showed excellent performance in terms of response time.
5 Review methodology of the novel research work of blockchain–AI integration and its applications in the energy sector
In this section, we present a systematic review to analyze the current research works performed in the area of the integration of blockchain and AI in the energy sector in the time interval between 2018 and 2024. Figure 13 shows the three stages of the conducted literature review. The planning stage identifies the objective of the research, the developed review protocol, and its validation. In the conducting phase, we identified relevant research studies and selected the primary set of studies before we extracted the required data for evaluation and ultimately synthesized these data. Finally, during the documentation stage, we disseminated the report based on the outcomes derived from the previous stage.
5.1 Planning stage
Identifying research objective and questions: Being disruptive technologies, blockchain and AI have been deployed in different areas, allowing for enhanced performance in terms of security, privacy, and provision of decentralization for the system while optimizing the operations and providing better monitoring and maintenance. Moreover, the existing challenges associated with the energy systems such as security, decentralization, and optimization triggered the need for the integration of blockchain and AI in the energy sector, although it is still in its infancy. Thus, we realized the need to review the novel use cases in this sector and extract opportunities and challenges while studying this novel topic as our research objective. Therefore, we identified the following as our research questions:
1. What are the general applications of blockchain technology?
2. What are the applications of integrating AI with blockchain?
3. How can blockchain technology be deployed in the energy sector?
4. How can AI be deployed in the energy sector?
5. What is the scope of integrating blockchain and AI in the energy sector?
Setting the protocol for the reviewing approach: Blockchain and AI are popular and continuously rising topics. They have been drawing the attention of different researchers for the last few years. However, very few have explored the novel approach of integrating blockchain with AI in the energy sector. As a result, this literature review examines current sources published between 2018 and 2023 (January), unveiling research in the last 5 years on AI–blockchain integration and deployment in the energy domain. Our search engines comprise the main major oriented databases, which are (1) IEEE Xplore digital library, (2) SpringerLink, (3) ScienceDirect, (4) ACM Digital Library, and other digital libraries such as MDPI. We considered quality research works such as published academic journals, peer-reviewed research articles, conference papers, book chapters, and magazines.
Selecting searching terms and keywords: Initially, we considered reviewing all the search terms and keywords such as “Blockchain” or “Artificial Intelligence” before we distinctly define the exact keywords and search queries in order to narrow down thousands of initial outcomes set from the available databases.
5.2 Conducting stage
Research protocol: Based on the inclusion and exclusion criteria (as of Table 3), multiple screening search queries have been executed in the initial search in each electronic database.
At first, 113,547 outcomes were obtained from the IEEE Xplore digital library as a preliminary result. Following with first screening, we extracted all papers with publication date <2018, with 40,862 remaining papers. Likewise, initial results were obtained from SpringerLink, ScienceDirect, and ACM Digital Library with outcomes of 16,916, 194,103, and 144,636, respectively. After the first screening, papers were reduced to 16,852, 100,130, and 46,633, respectively. As summarized in Table 4, 236,300 papers remained after the first screening (extracting all papers before 2018). In the next screening step, we applied all inclusion and exclusion criteria (as referred in Table 3) to reduce the number of papers to 1,236 only, as indicated in Table 5.
For confirming the compliancy of the studies to our research topic, we executed the final screening step that falls within the energy scope. During this step, the number of papers decreased to 40 papers only. Apparently, there are few research works conducted in the field of energy utilizing both blockchain and AI technologies together as they are still in their infant stage. As shown in Table 6, most of the works were published in the electronic database IEEE Xplore (29 papers), followed by ScienceDirect with 7 research works. Finally, we added four more works from the digital library MDPI as another source of publication, making up to 40 papers in total.
5.3 Documentation stage
A technique was chosen for searching the novel works for blockchain and AI amalgamation in the energy domain. Furthermore, a deeper delve into the analysis of each study was conducted along with a quantitative approach, which is discussed in Section 6.
6 Study analysis and research outcomes
This section evaluates the normative literature and summarizes the major findings of each research work. Our final 40 research works are summarized with their references (Hua et al., 2022; Li et al., 2023b; Bouckaert et al., 2021; Al-Saif et al., 2021; Wang et al., 2020; Hamid and Ganne, 2023), and the works are discussed accordingly.
6.1 Analyzing framework similarities
Starting with identifying similarities, all of the research works [(Hua et al., 2022; Li et al., 2023b; Bouckaert et al., 2021; Li et al., 2021)] discussed the need for a secure framework or platform for power management inside the microgrids as there is common privacy and security issues in data sharing and processing within the microgrids. Wang et al. (2020), Li et al. (2021), and Wang (2022) shared another commonality in that they attempted to integrate EVs with different power systems by proposing a private power transaction scheme based on a blockchain platform. Similarly, Li et al. (2021), Jamil et al. (2021), Gupta et al. (2021), Xiao et al. (2021), Chenli et al. (2021), and Al-Saif et al. (2021) proposed energy demand and load schedules based on their predictive models using ML and deep learning methods, thus providing real-time monitoring, decision-making, control optimization, and maximization of profits at the same time.
Aided by IoT technology, Shahinzadeh et al. (2022), Li et al. (2023b), Lin Y. et al. (2022), Yang and Wang (2021), Lin X. et al. (2022), Sherule and Dudhe (2021), Xiao et al. (2021), and Bouckaert et al. (2021) connected different computing devices (e.g., sensors) to collect and have complete control over energy data in order to optimize energy trading processes. The principle is to collect data from different sources including consumption sources, such as sensors and smart meters, and generation sources, like DERs. Scheduling of energy use was the main output of AI in the energy systems. For instance, Lin Y. et al. (2022) and Wang (2022) proposed an optimal charging and discharging scheduling scheme for EVs based on demand-side forecasting.
Kumari et al. (2020) proposed an energy cloud management system, aiming for intelligent energy consumption and meeting consumer demands. Efficient consumption can be achieved using AI, which satisfies customer requirements and goals. To build this ECM system, demand-side predictability and securely transferring data are proposed as significant reasons. Without AI integration, technically, creating this system would not be possible. As for the blockchain, it provides the necessary data protection and management. Kumari et al. (2020) focused on the advantages of SGs, which are emphasized as necessary energy management structures that can provide more efficiency. Focusing on SGs, AI and blockchain are the primary technologies that would carry the system (Jin et al., 2020). Secure digital transactions on the blockchain provide relief to a business or organization; it is reassuring and transparent. Jin et al. (2020) aimed to raise smart grid integration with aspects of blockchain and AI. Figure 14 shows the timeline of blockchain energy evolution in the energy sector Samuel et al., 2022).

Figure 14. Timeline of blockchain energy evolution in the energy sector (Samuel et al., 2022).
The application of AI and blockchain far extends in terms of use cases. Clean energy products such as EVs have also been pushed into this revolution. Wang et al. (2020) proposed an EV integration system based on blockchain and AI, which is a system proposed to foster energy management in a smart grid platform. Furthermore, Wang et al. (2020) explained about VPPs, which are introduced as a vehicle to control energy loads, power grids, and EVs. Wang et al. (2020) introduced this system because efficiently handling EV charging predictions is one of the underlying functionalities. The system’s supply side consists of the VPPs, and the EV fleet is considered the consumer side. Moreover, Salama et al. (2023) reviewed the applications of blockchain technology and smart grid platforms while maintaining an adequate stance on the benefits of AI in the industry. Applications of smart grids are researched to encourage prosumers to participate in the sector, and Hua et al. (2022) researched what applications can be created using AI and blockchain to encourage those individuals. Prosumers represent individuals in residents, businesses, and industries. Those individuals are similar to consumers, but they tend to design and customize the products they need. However, according to Hua et al. (2022), there are challenges involved in adapting consumers from the energy market and system point of view. Plenty of information is provided about blockchain and AI by Hua et al. (2022), discussing both the advantages and disadvantages.
Another significant reason for the integration of AI and blockchain technology in the energy sector is cybersecurity. Businesses can be prone to hacking massive amount of data, and stolen data might not be retrieved easily later on. Comprehensive research such as that by Mengidis et al. (2019) about cybersecurity in energy grids is essential to understand the effects of such cyberattacks in the industry; however, the provided solutions must be further implemented before any damage is incurred. Furthermore, we emphasize the importance of cybersecurity, but we must say that with blockchain technology’s security and transparency, coupled with AI’s management, protecting power systems and smart grids would be greatly probable. It is the core of blockchain technology to keep data safe. Mengidis et al. (2019) provided great insights about how cyberattacks can be diminished with AI and blockchain technology. Yang et al. (2024) captured the importance of AI and blockchain technology integration in the clean energy sector. The amount of research papers regarding AI and blockchain technology in the renewable energy sector has been growing greatly over the years, and the primary topics are EVs, sustainability, and photovoltaic systems. According to Shahinzadeh et al. (2022), the significance of blockchain technology in the IoT, big data analytics, and AI sectors indicates that it has been a satisfactory solution to protect user data without third-party service or intermediaries. Moreover, technical difficulties come in short often. Yildizbasi (2021) discussed the limitations of the energy grid management process and how problems can be overcome. Conversely, negotiations with government agencies, organizations, and policymakers should be handled due to regulation or policy requirements. Furthermore, Yildizbasi (2021) enhanced the discussion using modeling and numerical methods in order to detail the challenges encountered more.
When adopting various energy types in the sector, integrating blockchain technology for seamless transactions with AI, and thus, IoT, will deliver faster transactions. For energy systems, enabling demand-side satisfaction is extremely crucial. Miao et al. (2020) proposed a natural gas management system powered by AI and blockchain technology around IoT. Integrated with AI, the inclusion of IoT will create an ecosystem that enhances user experience. The diverse inclusion of different energy types in the industry will not only foster more opportunities but will also solve more problems, which will greatly improve the industry as solutions to these problems are found. New research will emerge, and theoretical information will pave the way for the foundation of technical solutions. The paper mainly investigates aspects of blockchain and AI by explaining algorithms and introducing experimental models. Blockchain technology adoption in the renewable energy sector has been a matter of research interest in recent years, and Gawusu et al. (2022) aimed to establish an argument of blockchain integration in the renewable energy sector by discussing essential knowledge about blockchain technology, such as consensus mechanisms, smart contracts, and blockchain types. Furthermore, Gawusu et al. (2022) elaborated on the prospects of energy trading markets and touched the matter of P2P energy trading, which is achievable by using blockchain technology in a decentralized manner. Gawusu et al. (2022) instilled technical knowledge of core blockchain technologies and energy markets to develop a course to finally emphasize challenges. Gusc et al. (2022) aimed to explain the TCA model to estimate energy costs. Gusc et al. (2022) identified a unique approach to detect energy costs using big data, AI, and blockchain technology in Europe. True cost accounting supports decision-making; a decision should be made when the primary impacts of the cost of a product or overall costs are evaluated. When it comes to blockchain and AI integration, Zhang et al. (2021) approached the matter with satisfying research. The success of this integration depends on approaching it from every angle. Industry standards, challenges, regulation and compliance, technical challenges, and management are some of the main aspects for the aforementioned success.
Smart grid applications and their management has become one of the prominent reasons of integrating AI and blockchain technology. SG-based distribution control management, automation, and power distribution are some of the challenges seen in the renewable energy-enabled devices (Khan A. et al., 2023). Smart grid solutions and automations processes come with technical challenges; however, most research papers include challenges related to the subject. A realistic approach to evaluate whether AI and blockchain are really becoming a significant part in the energy industry is to research their applications. For instance, the concept of smart cities can turn into a reality with blockchain and AI. Ahmed et al. (2022b) discussed about smart city applications using IoT and blockchain, and AI has been a research topic as well. Blockchain’s fast adoption puts it in an advantageous position, and building an ecosystem has never been easier. ElHusseini et al. (2020) elaborated on the integration of AI, blockchain technology, and smart grids for developing an EV smart charging infrastructure. Charging schedules, security, and privacy were discussed by ElHusseini et al. (2020).
Blockchain-related subjects such as energy trading, power grids and smart grids, transition to renewable energy, and AI are included by Teufel et al. (2019) as subjects of blockchain technology in the energy sector. Overall, the RE sector has been gaining much traction in recent years. Bodemer (2024) proposed different scenarios on blockchain and AI integration for advanced photovoltaic and battery systems, which indicate that challenges might arise. Current research on blockchain and AI should be investigated as well, for they reflect new trends and might be of help in the foundation of solutions to technical challenges. Battery and photovoltaic systems are RE industry subjects. Furthermore, trust issues appear in the energy industry, be it when making transactions or within management. Otoum and Mouftah (2021) inspected the advantages of AI and blockchain technology in maintaining trust and transparency in an energy infrastructure, answering the question, “How can AI and blockchain be integrated in sustainable systems to add further trust?” Establishing trust in the blockchain is the validators’ job; they maintain the network’s consensus. Hamid and Ganne (2023) mainly focused on discussions about overall energy sector application examples, such as energy trading, demand response optimization, grid optimizations, and forecasting faults, further building the discussion to opportunities and AI’s relation with economy, with a brief touch on blockchain technology. Nonetheless, AI and blockchain were explained side-by-side and are seen as opportunities for the energy sector. On the other hand, Jogunola et al. (2021) discussed consensus mechanisms such as PoW and PoS of blockchain technology and deep reinforcement learning, which is a branch of machine learning of AI in the energy market.
6.2 General AI–blockchain energy architecture
From the previously discussed analysis, apparently there are four general layers of energy management resulting from AI–blockchain amalgamation. Figure 15 summarizes the energy framework architecture of the proposed studies.
Energy consumption layer: This layer is related to data collection from different sources in the DES entities by using smart meters and IoT sensors. The role of the blockchain layer is to help transfer and store data securely in the energy system.
Data forecasting layer: This layer refers to processing the recorded energy data of real-time monitoring from the connected entities in the energy consumption layer.
AI–blockchain data layer: This layer represents the final architecture of AI–blockchain amalgamation in the energy sector. The blockchain module securely transfers energy data from energy distributers to the AI-enabled platform. The platform, in return, utilizes these predicted data (i.e., demand/supply patterns) to optimize the decision-making process in the technical and economic aspects.
Blockchain layer: This is considered an intersecting layer that keeps data transaction between the prosumers, consumers, and other energy system entities secure and private. Similarly, the blockchain layer interacts with all energy data layers and provides a safe resort for individual and corporate data. Furthermore, smart contracts execute payment transactions and validate these transactions through different algorithms.
6.3 Advantages of integrating AI with blockchain technology in the energy sector
This research underscores the significant advantages of integrating AI with blockchain technology, particularly in the energy sector. This integration offers a secure and reliable decentralized platform that enhances data processing, analysis, and decision-making. Blockchain technology serves as a dependable data storage platform, while AI efficiently manages and processes large datasets. Together, AI-powered energy systems and smart contracts enable autonomous learning and adaptation within the energy ecosystem, with decisions validated by blockchain nodes. This combination results in decentralized, secure, and immutable energy systems, where data processed and analyzed by AI are securely stored on the blockchain. AI has a long history and continues to advance through extensive research, impacting various sectors by automating systems and reducing workforce needs. The IoT facilitates this automation by enabling seamless connectivity among smart devices, transitioning from centralized control to collective connectivity. In the energy sector, this is particularly evident in smart city applications, smart grid management, energy trading, and EV charging infrastructure. Without integrating technologies such as AI and blockchain, achieving sustainable and efficient smart city models would be challenging. Blockchain technology, known for its speed, security, and decentralization, eliminates the need for third-party intermediaries and ensures reliable transactions. Since Satoshi Nakamoto introduced Bitcoin’s blockchain with PoW, various consensus mechanisms such as PoS have emerged. These advancements in blockchain technology are expected to drive its application across different industries, proving particularly effective in the energy sector, where traditional centralized systems would fall short.
Blockchain has many advantages, such as secure infrastructure, decentralized, anonymity, and verifiability (Zhu et al., 2020). The traditional electric power transaction model lacks efficiency and ease and is lengthy (Huang et al., 2020). Under blockchain technology, various research terminologies were searched, such as energy trading, smart grids, and smart contracts. Zha et al. (2022) showcased the keywords that were searched on scientific databases and the power of those keywords. It is important to realize that blockchain and IoT are two of the most influential keywords in the energy sector. It can be observed that the advantages of blockchain and AI are one of the most reviewed topics in the field.
Several advantages are explained by Kumari et al. (2020), such as increased security, system accuracy, data tracing, and decentralized intelligence. Furthermore, blockchain technology can enhance cybersecurity and synergy between various systems and technologies (Salama et al., 2023). Encryption in blockchain technology enables the protection of users’ private information, such as transaction details, addresses, and profiles (Hua et al., 2022). Moreover, the emergence of AI and blockchain technology has sped up the process of finding technical solutions in deploying clean energy systems (Yang et al., 2024). Blockchain technology is an ideal solution for IoT platforms that operate in unstable environments, which is ideal for maintaining a reliable and trustworthy environment (Shahinzadeh et al., 2022). Many mathematical models were introduced as part of the research matters being discussed with the readers to support theoretical information. Moreover, diversifying applications in the industry is a sign that the industry will thrive in the foreseeable future. Perhaps it would be suitable to provide a concept of a smart city to clarify AI and blockchain’s integration in the energy sector. At its core, the smart city should be powered by smart grids. SG integration would not only allow supplying energy to public and private lots but also enable smart energy supply to the city. AI works on the efficient delivery of energy, while the blockchain deals with the transaction process. The choice of the consensus mechanism carries significant importance because handling millions of transactions per second can be achieved by choosing a suitable mechanism for the depicted system.
Both blockchain technology and AI empower each other. If blockchain did not exist, transaction models would not have worked; thus, building an energy economy would not have been possible. AI serves as the foundation of an energy ecosystem. In a large-scale application such as a smart city, a smart IoT ecosystem will be created to create an automated and thriving environment.
In conclusion, combining AI with blockchain in the energy sector offers significant benefits. As shown in Figure 16, blockchain’s decentralized ledger ensures that data remain secure and unchangeable, boosting transparency in energy transactions and operations. This trustworthiness is vital for fostering confidence among stakeholders and effectively managing distributed energy resources. AI, leveraging the data stored on the blockchain, enhances the accuracy of energy forecasts and optimizes real-time energy consumption. This collaboration supports the implementation of flexible pricing models and facilitates direct P2P energy trading, eliminating the need for intermediaries. Ultimately, integrating AI and blockchain creates a robust framework that promotes innovation, ensures compliance with regulations, and enhances overall system reliability in the energy industry.
6.4 Open research challenges
The integration of blockchain technology with AI in the energy sector leads to the following challenges and limitations.
Data privacy: In many energy systems, a public blockchain ledger is used (despite using private consortiums in some cases), where data are openly available on all node networks. This raises the question of how vulnerable the data are. Moreover, some study cases use IoT devices such as smart meters to collect personal data, which are stored in the blockchain platform, raising more privacy concerns.
Data security: Particularly in energy trading systems, Ethereum is a largely deployed blockchain-based platform. Yet, the platform has been the target of critical cyberattacks in the past. As mentioned before, a public blockchain can be compromised as miners carry out the consensus algorithm. Although it has been proven that using a private blockchain such as Hyperledger provides more security than when using a public blockchain, there is less tendency to deploy it in the energy sector.
Data fluctuation: Input data that come from IoT devices such as sensors are fluctuating in nature. Subsequently, random and approximate results are generated as a result of the execution of the smart contract, which leads to inaccurate decision-making output results. Therefore, finding a consensus protocol that sets output data as precise as deterministic is necessary.
Data scalability: As the number of transactions increase, there is a higher limitation to process these transactions due to the time required for confirmation, making scalability a major concern, especially when adding more DERs to the whole energy system. These initiate thousands of transactions and would affect the efficiency of training and the accuracy of prediction capability, leading to potential inaccurate decision-making results. Therefore, there is a need to develop a feature in the blockchain in order to scale up transaction numbers in parallel with the number of connected devices in the energy system. An example of a challenge encountered especially in energy P2P trading is the required power and demand in microgrids (Xu et al., 2020).
However, one of the main purposes of this research is to explore challenges, combined with the advantages that Blockchain and AI provides. As such, challenges related to handling data can be solved by utilizing the advantages of each technology. For instance, scalability appears to be a major issue in implementing such systems. However, blockchain’s different block processing mechanisms greatly assist in easing scalability issues, presenting a challenge that is solvable with today’s advantageous stance in blockchain scalability methods. Furthermore, challenges present us with numerous opportunities to explore solutions to existing issues, begetting the need to delve deeper into theory and technical grounds. The mass adoption of a unique technology can present with a question of “scalability.” The opportunity arises to tackle the adoption challenge when we develop a system that can increase block production, or vice versa. Conversely, adoption would be a failure if issues are not properly addressed. With Blockchain and AI, we expect that data provided will be private and secure. One of the opportunities blockchain provides is that it is decentralized, however a main challenge is that it is prone to cyberattacks. A challenge arises from here, such as: how can blockchain security be maintained? Indeed, the blockchain has protocols that protect the layer from attacks, and blockchain still remains a powerful place to build energy systems, regardless of the challenges it is prone to. AI’s optimization throughout energy processes provides assurance to users that operations would be carried out intelligently. Current existing challenges pave the way for new research and development, such that new opportunities in existing technologies would be built. In a broader sense, the potential benefits of the challenges of both technologies will only contribute to further studies and lead to successful outcomes.
7 Conclusion
Integrating AI with blockchain technology in the energy sector holds an unlimited number of potential opportunities such that deploying these emerging technologies would resolve many issues faced in the energy systems, such as data security and privacy, and help make reliable decisions with more accurate data. In addition, it would facilitate better transaction methods using decentralized platforms. Challenges arise in every technical concept that empowers technical systems; it is only typical to encounter issues when implementing powerful technologies. Onboarding a wide range of users requires rigorous scalability that enables the mass adoption of energy trading. We mentioned a wide range of applications in AI and Blockchain, such as the integration of renewable energy, which were coupled with concerns that demand swift resolution. As much as ensuring system longevity, we reinstated that handling data has become of great significance, which has its own challenges. However, with the combination of AI and Blockchain those challenges can be greatly diminished. On the other hand, the integration would face many challenges, particularly when adding more energy components to the system with more energy users; the need to initiate a countless number of transactions would adversely affect the prediction capability, and hence, it would cause potential inaccuracy in the decision-making process. Furthermore, in this paper, we briefly introduced the basics of blockchain and AI, including their structures, taxonomies, and fundamental features. We reviewed some major applications of the integration of blockchain and AI in multiple areas with some use cases. We discussed the applications of blockchain and AI in the energy sector and how this can help in forming a new ecosystem in the energy domains. We also discussed the major benefits incurred from the integration. We reported and discussed many novel use cases and systematically reviewed studies of blockchain–AI applications in the energy sector, including the review methodology of the novel research work on blockchain–AI integration and its applications in the energy sector. Then, we analyzed the research outcomes, highlighting study similarities and the general structure/framework of integrating AI with blockchain. Finally, we identified the outcomes of 40 novel studies, shedding light on the technologies and contributions of each study. Moreover, we identified the major advantages of combining AI with blockchain in different energy systems. Finally, we identified the main challenges and the limitations associated with adopting AI–blockchain integration in the energy sector.
Author contributions
AA: conceptualization, data curation, formal analysis, funding acquisition, investigation, methodology, project administration, resources, software, supervision, validation, visualization, writing–original draft, and writing–review and editing. SS: investigation, project administration, supervision, and writing–review and editing. BE: conceptualization and writing–review and editing. HA: writing–review and editing and project administration.
Funding
The author(s) declare that no financial support was received for the research, authorship, and/or publication of this article.
Acknowledgments
We acknowledge the use of advanced generative technologies for producing the images in this article. In this study, images were created using generative technology (version GPT-4). This tool was utilized to produce images in Figure 5. “Store transaction of power information in three different microgrid modes,” contributing to the efficiency and clarity of the visual components of our research.
Conflict of interest
The authors declare that the research was conducted in the absence of any commercial or financial relationships that could be construed as a potential conflict of interest.
Publisher’s note
All claims expressed in this article are solely those of the authors and do not necessarily represent those of their affiliated organizations, or those of the publisher, the editors, and the reviewers. Any product that may be evaluated in this article, or claim that may be made by its manufacturer, is not guaranteed or endorsed by the publisher.
Supplementary material
The Supplementary Material for this article can be found online at: https://www.frontiersin.org/articles/10.3389/fenrg.2024.1377950/full#supplementary-material
References
Ahmad, T., Zhang, D., Huang, C., Zhang, H., Dai, N., Song, Y., et al. (2021). Artificial intelligence in sustainable energy industry: Status Quo, challenges and opportunities. J. Clean. Prod. 289, 125834. doi:10.1016/j.jclepro.2021.125834
Ahmad, T., Zhu, H., Zhang, D., Tariq, R., Bassam, A., Ullah, F., et al. (2022). Energetics Systems and artificial intelligence: applications of industry 4.0. Energy Rep. 8, 334–361. doi:10.1016/j.egyr.2021.11.256
Ahmed, I., Zhang, Y., Jeon, G., Lin, W., Khosravi, M. R., and Qi, L. (2022a). A Blockchain- and artificial intelligence-enabled smart IoT framework for sustainable city. Int. J. Intelligent Syst. 37, 6493–6507. doi:10.1002/int.22852
Ahmed, I., Zhang, Y., Jeon, G., Lin, W., Khosravi, M. R., and Qi, L. (2022b). A Blockchain-and artificial intelligence-enabled smart IoT framework for sustainable city. Int. J. Intelligent Syst. 37 (9), 6493–6507. doi:10.1002/int.22852
Al-Abri, T., Onen, A., Al-Abri, R., Hossen, A., Al-Hinai, A., Jung, J., et al. (2022). Review on energy application using Blockchain technology with an introductions in the pricing infrastructure. IEEE 10 (1), 80119–80137. doi:10.1109/access.2022.3194161
Al-Fatlawi, H. H., Khayoon, Q. M., Albhadly, A. J., and Ibrahim, A. K. (2024). AI-enhanced demand response strategies in smart grids: toward sustainable energy future. J. Electr. Syst., 1054–1064.
Ali, M., Prakash, K., Hossain, Md. A., and Pota, H. R. (2021). Intelligent energy management: evolving developments, current challenges, and research directions for sustainable future. J. Clean. Prod. 314, 127904. doi:10.1016/j.jclepro.2021.127904
Al-Saif, N., Ahmad, R., Salah, K., Yaqoob, I., Jayaraman, R., and Omar, M. (2021). Blockchain for electric vehicles energy trading: requirements, opportunities, and challenges. IEEE Access 9 (1), 156947–156961. doi:10.1109/access.2021.3130095
Altin, N., and Eyimaya, S. E. (2023). “Artificial intelligence applications for energy management in microgrid,” in 2023 11th International Conference on Smart Grid (icSmartGrid), Paris, France, 1–6. doi:10.1109/icsmartgrid58556.2023.10170860
Andoni, M., Robu, V., Flynn, D., Abram, S., Geach, D., Jenkins, D., et al. (2019a). Blockchain technology in the energy sector: a systematic review of challenges and opportunities. Renew. Sustain. Energy Rev. 100, 143–174. doi:10.1016/j.rser.2018.10.014
Andoni, M., Robu, V., Flynn, D., Abram, S., Geach, D., Jenkins, D., et al. (2019b). Blockchain technology in the energy sector: a systematic review of challenges and opportunities. Renew. Sustain. Energy Rev. 100, 143–174. doi:10.1016/j.rser.2018.10.014
ArulRaj, K., Karthikeyan, M., and Narmatha, D. (2021). A view of artificial neural network models in different application areas. EDP Sciences. Available at: https://www.researchgate.net/publication/353143135.
Badidi, E. (2022). Edge AI and Blockchain for smart sustainable cities: promise and potential. Sustainability 14 (13), 7609. doi:10.3390/su14137609
Baltrušaitis, T., Ahuja, C., and Morency, L.-P. (2018). Multimodal machine learning: a survey and taxonomy. IEEE Trans. Pattern Analysis Mach. Intell. 41, 423–443. doi:10.1109/tpami.2018.2798607
Belotti, M., Božić, N., Pujolle, G., and Secci, S. (2019). A vademecum on Blockchain technologies: when, which, and how. IEEE Commun. Surv. and Tutorials 21 (4), 3796–3838. doi:10.1109/comst.2019.2928178
Bishaw, F. (2024). Review artificial intelligence applications in renewable energy systems integration. J. Electr. Syst. 20, 566–582. doi:10.52783/jes.2983
Bodemer, O. (2024). Empowering prosumers in the energy sector: integrating AI and Blockchain for enhanced photovoltaic and battery systems. Eng. OA 2 (1), 01–07.
Bouckaert, S., Pales, A., Mekhilef, S., McGlade, C., Remme, U., Wanner, B., et al. (2021). Net zero by 2050: a roadmap for the global energy sector. Paris, France: IEA. Available at: https://www.iea.org/reports/net-zero-by-2050.
Boumaiza, A., and Sanfilippo, A. (2022). “AI for energy: a blockchain-based trading market,” in Annual Conference of the IEEE Industrial Electronics Society, 1–6. 978-1-6654-8025-3/22. doi:10.1109/iecon49645.2022.9968686
Brundo, R., and De Nicola, R. (2018). Blockchain-based decentralized cloud/fog solutions: challenges, opportunities, and standards. IEEE Commun. Stand. Mag. 2 (3), 22–28. doi:10.1109/mcomstd.2018.1800020
Cai, W., Wang, Z., Ernst, J. B., Hong, Z., Feng, C., and Leung, V. C. (2018). Decentralized applications: the Blockchain-empowered software system. IEEE Access 6, 53019–53033. doi:10.1109/access.2018.2870644
Chatterjee, R., and Chatterjee, Ra. (2017). “An overview of the emerging technology: Blockchain,” in International Conference on Computational Intelligence and Networks, 126–127. 978-1-5386-2529-3/17. doi:10.1109/cine.2017.33
Chen, H. Y., Shamra, K., Sharma, C., and Sharma, S. (2023). “Integrating explainable artificial intelligence and Blockchain to smart agriculture: research prospects for decision making and improved security,” in Smart agricultural technology (Elsevier).
Chen, S., Ping, J., Yan, Z., Li, J., and Huang, Z. (2022). Blockchain in energy systems: values, opportunities, and limitations. Front. Energy 16 (1), 9–18. doi:10.1007/s11708-022-0818-8
Chenli, C., Li, B., Shi, Y., and Jung, T. (2021). “Energy-recycling Blockchain with proof-of-deep-learning,” in IEEE, International Conference on Blockchain and Cryptocurrency (ICBC). 978-1-7281-1328-9/19.
Christidis, K., and Devetsikiotis, M. (2016). Blockchains and smart contracts for the internet of things. Ieee Access 4, 2292–2303. doi:10.1109/access.2016.2566339
Cinar, Z., Nuhu, A., Zeeshan, Q., Korhan, O., Asmael, M., and Safaei, B. (2020). Machine learning in predictive maintenance towards sustainable smart manufacturing in industry 4.0. Sustainability: MDPI.
Daniel, J., Sargolzaei, A., Abdelghani, M., Sargolzaei, S., and Amaba, B. (2017). Blockchain technology, cognitive computing, and healthcare innovations. J. Adv. Inf. Technol. 8 (3), 194–198. doi:10.12720/jait.8.3.194-198
Dargan, J., Gupta, N., and Singh, L. (2021). “Blockchain based energy management system: a proposed model,” in IEEE, International Conference on Technological Advancements and Innovations (ICTAI), 510–514. 978-1-6654-2087-7/21. doi:10.1109/ictai53825.2021.9673233
Dinh, T. T. A., Liu, R., Zhang, M., Chen, G., Ooi, B. C., and Wang, J. (2018). Untangling Blockchain: a data processing view of Blockchain systems. IEEE Trans. Knowl. Data Eng. 30 (7), 1366–1385. doi:10.1109/tkde.2017.2781227
Di Silvestre, M. L., Gallo, P., Ippolito, M. G., Sanseverino, E. R., and Zizzo, G. (2018). A technical approach to the energy Blockchain in microgrids. IEEE Trans. Industrial Inf. 14 (11), 4792–4803. doi:10.1109/tii.2018.2806357
Djavanshir, G., Chen, X., and Yang, W. (2021). A review of artificial intelligence’s neural networks (Deep learning) applications in medical diagnosis and prediction. IEEE, 1520–9202.
Dobrev, D. (2012). A definition of artificial intelligence. ArXiv Cornell Univ. Comput. Sci. Artif. Intell.
Effah, D., Yu, G., Lu, Q., and Xu, X. (2021). Carbon emission monitoring and credit trading: the Blockchain and IOT approach. IEEE. 978-1-6654-1364-0/21.
ElHusseini, H., Assi, C., Moussa, B., Attallah, R., and Ghrayeb, A. (2020). Blockchain, AI and smart grids: the three musketeers to a decentralized EV charging infrastructure. IEEE Internet Things Mag. 3 (2), 24–29. doi:10.1109/iotm.0001.1900081
Fernández-Caramés, T. M., and Fraga-Lamas, P. (2018). A review on the use of Blockchain for the internet of things. IEEE Access 6, 32979–33001. doi:10.1109/access.2018.2842685
Ferrag, M., and Maglaras, L. (2020). DeepCoin: a novel Deep learning and blockchain-based energy exchange framework for smart grids. IEEE Trans. Eng. Manag. 67 (4), 1285–1297. doi:10.1109/tem.2019.2922936
Fioretto, F., Pontelli, E., and Yeoh, W. (2016). Distributed constraint optimization problems and applications: a survey. arXiv Prepr. arXiv:1602.06347. doi:10.1613/jair.5565
Gawusu, S., Zhang, X., Ahmed, A., Jamatutu, S. A., Miensah, E. D., Amadu, A. A., et al. (2022). Renewable energy sources from the perspective of Blockchain integration: from theory to application. Sustain. Energy Technol. Assessments 52, 102108. doi:10.1016/j.seta.2022.102108
Goli, A., Bozanic, D., Zhou, F., and Ali, I. (2024). Editorial: the role of Blockchain technology toward a sustainable energy future. Front. Energy Res. 12, 1361080. doi:10.3389/fenrg.2024.1361080
Goodarzian, F., Ghasemi, P., Gonzalez, E. D. R., and Tirkolaee, E. (2023). A sustainable-circular citrus closed-loop supply chain configuration: pareto-based algorithms. J. Environ. Manag. 328, 116892. doi:10.1016/j.jenvman.2022.116892
Gupta, Y., Javorac, M., Cyr, S., and Yassine, A. (2021). “HELIUS: a Blockchain based renewable energy trading system,” in IEEE 4th International Seminar on Research of Information Technology and Intelligent Systems (ISRITI). 978-1-6654-0151-7/21.
Gusc, J., Bosma, P., Jarka, S., and Biernat-Jarka, A. (2022). The big data, artificial intelligence, and Blockchain in true cost accounting for energy transition in europe. Energies 15, 1089. doi:10.3390/en15031089
Hamid, M., and Ganne, A. (2023). Artificial intelligence in energy markets and power systems. J. Eng. Technol. Manag. 5, 2582–5208. doi:10.3390/en15031089
Hamidi, M., Raihani, A., Bouattane, O., and Al-Olama, S. S. (2023). “Analyzing microgrid energy profile behavior to study a shared energy management system based on AI: Dubai buildings case study,” in Middle East and North Africa Solar Conference (MENA-SC), Dubai, United Arab Emirates, 1–7. doi:10.1109/mena-sc54044.2023.10374504
Hazari, S. S., and Mahmoud, Q. H. (2020). Improving transaction speed and scalability of Blockchain systems via parallel proof of work. Future Internet 12 (8), 125. doi:10.3390/fi12080125
Hu, W., and Li, H. (2021). A Blockchain-based secure transaction model for distributed energy in Industrial Internet of Things. Alexandria Eng. J. 60 (1), 491–500. ISSN 1110-0168. doi:10.1016/j.aej.2020.09.021
Hua, W., Chen, Y., Qadrdan, M., Jiang, J., Sun, H., and Wu, J. (2022). Applications of Blockchain and artificial intelligence technologies for enabling prosumers in smart grids: a review. ’’ Renew. Sustain. Energy Rev. 161, 112308. doi:10.1016/j.rser.2022.112308
Huang, L. Y., Cai, J. F., Lee, T. C., and Weng, M. H. (2020). A study on the development trends of the energy system with Blockchain technology using patent analysis. Sustainability 12 (5), 2005. doi:10.3390/su12052005
Jabarulla, M., and Lee, H. (2021). A Blockchain and artificial intelligence-based, patient-centric healthcare system for combating the COVID-19 pandemic: opportunities and applications. Healthcare 9, 1019. doi:10.3390/healthcare9081019
Jadav, N., Rathod, T., Gupta, R., Tanwar, S., Kumar, N., and Alkhayyat, A. (2023). Blockchain and artificial intelligence-empowered smart agriculture framework for maximizing human life expectancy. Comput. Electr. Eng. 105, 108486. doi:10.1016/j.compeleceng.2022.108486
Jain, A. K., Mao, J., and Mohiuddin, K. M. (1996). Artificial neural networks: a tutorial. Computer 29 (3), 31–44. doi:10.1109/2.485891
Jain, V., Chaudhary, G., Luthra, N., Rao, A., and Walia, S. (2019). Dynamic handwritten signature and machine learning based identity verification for keyless cryptocurrency transactions. J. Discrete Math. Sci. Cryptogr. 22 (2), 191–202. doi:10.1080/09720529.2019.1582867
Jamil, F., Iqbal, N., Imran, , Ahmad, S., and Kim, D. (2021). Peer-to-Peer energy trading mechanism based on Blockchain and machine learning for sustainable electrical power supply in smart grid. IEEE 9 (1), 39193–39217. doi:10.1109/access.2021.3060457
Jha, S. K., Bilalovic, J., Jha, A., Patel, N., and Zhang, H. (2017). Renewable energy: present research and future scope of Artificial Intelligence. Renew. Sustain. Energy Rev. 77, 297–317. doi:10.1016/j.rser.2017.04.018
Jiang, K., and Lu, X. (2021). Natural Language processing and its applications in machine translation: a diachronic review. IEEE. 978-1-7281-7738-0. doi:10.1109/IICSPI51290.2020.9332458
Jin, A., Li, C., Su, J., and Tan, J. (2022). Fundamental studies of smart distributed energy resources along with energy Blockchain. Energies 15, 8067. doi:10.3390/en15218067
Jin, A., Li, C., Su, J., Tan, J., Mamun, K. A., Islam, F., et al. (2020). Distributed energy resources and the application of AI, IoT, and Blockchain in smart grids. Energies 13, 5739. doi:10.3390/en13215739
Jogunola, O., Adebisi, B., Ikpehai, A., Popoola, S., Gui, G., Gacanin, H., et al. (2021). Consensus algorithms and Deep reinforcement learning in energy market: a review. IEEE Internet Things J. 8 (6), 4211–4227. doi:10.1109/jiot.2020.3032162
Junaidi, N., Abdullah, M. P., Alharbi, B., and Shaaban, M. (2024). Blockchain-based management of demand response in electric energy grids: a systematic review. Energy Rep. 9, 5075–5100. doi:10.1016/j.egyr.2023.04.020
Kang, M., and Jameson, N. (2018) “Machine learning fundamentals,” in Prognostics and health management in electronics: fundamentals, machine learning, and Internet of Things. Willey Online Library.
Khan, A., Laghari, A., Rashid, M., Li, H., Javed, A., and Gadekallu, T. (2023a). Artificial intelligence and Blockchain technology for secure smart grid and power distribution automation: a state-of-the-art review. Sustain. Energy Technol. Assessments 57, 103282. doi:10.1016/j.seta.2023.103282
Khan, M., Saleh, A., Waseem, M., and Sajjad, I. (2023b). Artificial intelligence enabled demand response: prospects and challenges in smart grid environment. IEEE 11 (1), 1477–1505. doi:10.1109/access.2022.3231444
Khanzode, C., and Sarode, R. (2020). Advantages and disadvantages of artificial intelligence and machine learning: a literature review. Int. J. Libr. and Inf. Sci. (IJLIS) 9 (1).
Khezami, N., Gharbi, N., Neji, B., and Braiek, N. (2022). Blockchain technology implementation in the energy sector: comprehensive literature review and mapping. Sustainability 15826. doi:10.3390/su142315826
Kumari, A., Gupta, R., and Tanwar, S. (2021). “PRS-P2P: a prosumer recommender system for secure P2P energy trading using Q-learning towards 6G,” in IEEE International Conference on Communications Workshops, ICC, 1–6. 978-1-7281-9441-7/21. doi:10.1109/iccworkshops50388.2021.9473888
Kumari, A., Gupta, R., Tanwar, S., and Kumar, N. (2020). Blockchain and AI amalgamation for energy cloud management: challenges, solutions, and future directions. J. Parallel Distributed Comput. 1 (123), 148–166. doi:10.1016/j.jpdc.2020.05.004
Kuzior, A., Sira, M., and Brozek, P. (2022). Using Blockchain and artificial intelligence in energy management as a tool to achieve energy efficiency. Virtual Econ. 5, 69–90. doi:10.34021/ve.2022.05.03(4)
Lee, D., and Yoon, S. (2021). Application of artificial intelligence-based technologies in the healthcare industry: opportunities and challenges. J. Adv. Inf. Technol. 18 (1), 271. doi:10.3390/ijerph18010271
Li, J., Herdem, M., Nathwani, J., and Wen, J. Z. (2023a). Methods and applications for artificial intelligence, big data, internet of things, and Blockchain in smart energy management. Energy AI 11, 100208. doi:10.1016/j.egyai.2022.100208
Li, J., Herdem, M. S., Nathwani, J., and Wen, J. Z. (2023b). Methods and applications for artificial intelligence, big data, internet of things, and Blockchain in smart energy management. Energy AI 11, 100208. doi:10.1016/j.egyai.2022.100208
Li, X., Jiang, P., Chen, T., Luo, X., and Wen, Q. (2017). A survey on the security of Blockchain systems. Future Gener. Comput. Syst. 107, 841–853. doi:10.1016/j.future.2017.08.020
Li, Z., Xu, J., Xie, Y., Jiang, J., Zhu, Y., and Yang, X. (2021). “Integration of Blockchain and machine learning for microgrids,” in IEEE the 6th International Conference on Computer and Communication Systems, 211–216. 978-0-7381-2604-3/21. doi:10.1109/icccs52626.2021.9449300
Lin, X., Wu, J., Bashir, A., Li, J., Yang, W., and Piran, M. (2022b). Blockchain-based incentive energy-knowledge trading in IoT: joint power transfer and AI design. IEEE Internet Of Things J. 9 (16), 14685–14698. doi:10.1109/jiot.2020.3024246
Lin, Y., Cheng, Y., Zheng, J., Chu, D., Shao, D., and Yang, H. (2022a). Blockchain power trading and energy management platform. IEEE Power and Energy Soc. Sect. 10 (1), 75932–75948. doi:10.1109/access.2022.3189472
Lu, B., Shen, X., and Ping, J. (2020) “Energy storage sharing mechanism based on Blockchain,” in IEEE, student conference on electric machines and systems. 978-1-7281-5622-4/20.
Ma, R., Yi, Z., Xiang, Y., Shi, D., Xu, C., and Wu, H. (2022). A blockchain-enabled demand management and control framework driven by Deep reinforcement learning. IEEE Trans. Industrial Electron. 70 (1), 430–440. doi:10.1109/tie.2022.3146631
Ma, Y., Chen, X., Wang, L., and Yang, J. (2021). Study on smart home energy management system based on artificial intelligence. J. Sensors 2021 (1), 9101453. doi:10.1155/2021/9101453
Megha, R., Madhura, A., and Sneha, Y. (2017). Cognitive computing and its applications. IEEE 2, 1168–1172. doi:10.1109/icecds.2017.8389625
Mengidis, N., Tsikrika, T., Vrochidis, S., and Kompatsiaris, I. (2019). Blockchain and AI for the next generation energy grids: cybersecurity challenges and opportunities. Inf. and Secur. 43 (1), 21–33. doi:10.11610/isij.4302
Miao, Y., Zhou, M., and Ghoneim, A. (2020). Blockchain and AI-based natural gas industrial IoT system: architecture and design issues. IEEE Netw. 34 (5), 84–90. doi:10.1109/mnet.011.1900532
Mischos, S., Dalagdi, E., and Vrakas, D. (2023). Intelligent energy management systems: a review. Artif. Intell. Rev. 56, 11635–11674. doi:10.1007/s10462-023-10441-3
Mohammadi, E., Alizadeh, M., Asgarimoghaddam, M., Wang, X., and Simões, M. (2022). A review on application of artificial intelligence techniques in microgrids. IEEE 3 (4), 878–890. doi:10.1109/jestie.2022.3198504
Momenitabar, M., Ebrahimi, Z., and Ghasemi, P. (2022b) “Designing A sustainable bioethanol supply chain network: a combination of machine learning and meta-heuristic algorithms,” in Industrial crops and products. Elsevier.
Momenitabar, M., Ebrahimi, Z. D., Arani, M., Mattson, J., and Ghasemi, P. (2022a). “Designing A sustainable closed-loop supply chain network considering lateral resupply and backup suppliers using Fuzzy inference system”. Environment. Dev. Sustain. Springer Nat. 1–34. doi:10.1007/s10668-022-02332-4
Momenitabar, M., Ebrahimi, Z. D. D., Abdollahi, A., Helmi, W., Bengtson, K., and Ghasemi, P. (2023). An integrated machine learning and quantitative optimization method for designing sustainable bioethanol supply chain networks. Decis. Anal. J. 7, 100236. doi:10.1016/j.dajour.2023.100236
Monett, D., Lewis, C., and Thorisson, K. (2019). On defining artificial intelligence. J. Artif. General Intell. 10 (2), 1–37. doi:10.2478/jagi-2019-0002
Mureddu, M., Ghiani, E., and Pilo, F. (2020). Smart GridOptimization with Blockchain based decentralized genetic algorithm. IEEE General Meeting Power& Energy Society. 978-1-7281-5508-1/20.
Musamih, A., Jayaraman, R., Salah, K., Hasan, H., Yaqoob, I., and Al-Hammadi, Y. (2021). Blockchain-based solution for distribution and delivery of COVID-19 vaccines. IEEE 9, 71372–71387. doi:10.1109/access.2021.3079197
Nagarhalli, T., Mhatre, S., Patil, S., and Patil, S. (2022). “The review of Natural Language Processing applications with emphasis on machine learning implementations,” in Proceedings of the International Conference on Electronics and Renewable Systems (IEEE). 978-1-6654-8425-1/22.
Nakamoto, S. (2008). Bitcoin, A peer-to-peer electronic cash system. Available at: https://bitcoin.org/bitcoin.pdf32.N.Ramadani,E.Ramadani,F.Idrizi,V.Misimi,“Blockchain:generaloverviewoftheArchitecture,SecurityandReliability”.JournalofNaturalSciencesandMathematicsofUT.
Neudecker, T., and Hartenstein, H. (2018). Network layer aspects of permissionless Blockchains. IEEE Commun. Surv. and Tutorials 21, 838–857. doi:10.1109/comst.2018.2852480
Nguyen, D. (2020). Electric vehicle – wireless charging-discharging lane decentralized peer-to-peer energy trading. IEEE 8, 179616–179625. doi:10.1109/access.2020.3027832
Otoum, S., and Mouftah, H. (2021). Enabling trustworthiness in sustainable energy infrastructure through Blockchain and AI-assisted solutions. IEEE Wirel. Commun., 1536–1284/21. doi:10.1109/MWC.018.2100194
Panarello, A., Tapas, N., Merlino, G., Longo, F., and Puliafito, A. (2018). Blockchain and iot integration: a systematic survey. Sensors 18 (8), 2575. doi:10.3390/s18082575
Patel, R., Sethia, A., and Patil, S. (2018). “Blockchain – future of decentralized systems,” in International Conference on Computing, Power and Communication Technologies (GUCON), India, 369–374. doi:10.1109/gucon.2018.8675091
Pilkington, M. (2016). “Blockchain technology: principles and applications (september 18, 2015),” in Research handbook on digital transformations. Editors F. Xavier Olleros, and M. Zhegu (E. Elgar).
Ray, S. (2019). “A quick review of machine learning algorithms,” in IEEE, International Conference on Machine Learning, Big Data, Cloud and Parallel Computing (Com-IT-Con). 978-1-7281-0211-5/19. doi:10.1109/COMITCon.2019.8862451
Reebadiya, D., and Gupta, R. (2022). Blockchain and AI-integrated vehicle-based dynamic parking pricing scheme. IEEE. 978-1-7281-9441-7/21.
Rincy, T., and Gupta, R. (2020). “A survey on machine learning approaches and its techniques,” in IEEE International Students' Conference on Electrical, Electronics and Computer Science. 978-1-7281-4862-5/20.
Rocha, H. R., Honorato, I. H., Fiorotti, R., Celeste, W. C., Silvestre, L. J., and Silva, J. A. (2021). An Artificial Intelligence based scheduling algorithm for demand-side energy management in Smart Homes. Appl. Energy 282, 116145. doi:10.1016/j.apenergy.2020.116145
Sabri, Y., El Kamoun, N., and Lakrami, F. (2019). “A survey: centralized, decentralized, and distributed control scheme,” in Smart grid systems (IEEE). 978-1-7281-4420-7/19.
Safaei, S., Ghasemi, P., Goodarzian, F., and Momenitabar, M. (2022a). Designing a new multi-echelon multi-period closed-loop supply chain network by forecasting demand using time series model: a genetic algorithm. Environ. Sci. Pollut. Res. 29, 79754–79768. doi:10.1007/s11356-022-19341-5
Safaei, S., Ghasemi, P., Goodarzian, F., and Momenitabar, M. (2022b). “Designing A new multi-echelon multi-period closed-loop supply chain network by forecasting demand using time series model: a genetic algorithm,” in Environmental science and pollution research (Springer).
Said, D., Elloumi, M., and Khoukhi, L. (2022). Cyber-attack on P2P energy transaction between connected electric vehicles: a false data injection detection based machine learning model. IEEE 10 (1), 63640–63647. doi:10.1109/access.2022.3182689
Salah, K., Rehman, M. H. U., Nizamuddin, N., and AlFuqaha, A. (2019). Blockchain for AI: review and open research challenges. IEEE Access 7, 10127–10149. doi:10.1109/access.2018.2890507
Salama, R., Altrjman, C., and Al-Turjman, F. (2023). Smart grid applications and Blockchain technology in the AI era. NEU J. Artif. Intell. Internet Things 1 (1), 59–63.
Salman, T., Zolanvari, M., Erbad, A., Jain, R., and Samaka, M. (2018). Security services using Blockchains: a state-of-the-art survey. IEEE Commun. Surv. and Tutorials 21, 858–880. doi:10.1109/comst.2018.2863956
Samuel, O., Javaid, N., Alghamdi, T., and Kumar, N. (2022). Towards sustainable smart cities: a secure and scalable trading system for residential homes using Blockchain and artificial intelligence. Sustain. Cities Soc. 76 (1), 103371. doi:10.1016/j.scs.2021.103371
Sanseverino, E. R., Di Silvestre, M. L., Gallo, P., Zizzo, G., and Ippolito, M. (2017). “The Blockchain in microgrids for transacting energy and attributing losses,” in 2017 IEEE International Conference on Internet of Things (iThings) and IEEE Green Computing and Communications (GreenCom) and IEEE Cyber, Physical and Social Computing (CPSCom) and IEEE Smart Data (Exeter, UK: SmartData), 925–930.
Sedlmeir, J., Buhl, H. U., Fridgen, G., and Keller, R. (2021). Recent developments in Blockchain technology and their impact on energy consumption. arXiv Prepr. arXiv:2102.07886.
Shafiullah, M., Refat, A. M., Haque, M. E., Chowdhury, D. M. H., Hossain, M. S., Alharbi, A. G., et al. (2022). Review of recent developments in microgrid energy management strategies. Sustainability 14, 14794. doi:10.3390/su142214794
Shahinzadeh, H., Zanjani, S., and Moradi, J. (2022). The transition toward merging big data analytics, IoT, and artificial intelligence with Blockchain in transactive energy markets. IEEE, Glob. Energy Conf. (GEC). 978-1-6654-9751-0/22. doi:10.1109/GEC55014.2022.9986604
Sharma, A., Podoplelova, E., Shapovalov, G., Tselykh, A., and Alexander, T. (2021). Sustainable smart cities: convergence of artificial intelligence and Blockchain. Sustainability 13, 13076. doi:10.3390/su132313076
Sherule, A., and Dudhe, R. (2021). “Disruptive technologies in energy and environment,” in International Conference on Computational Intelligence and Knowledge Economy (ICCIKE) (IEEE). 37–41. 978-1-6654-2921-4/21. doi:10.1109/iccike51210.2021.9410785
Shinde, P., and Shah, S. (2018). A review of machine learning and Deep learning applications. IEEE. 978-1-5386-5257-2/18. doi:10.1109/ICCUBEA.2018.8697857
Soto, E., Bosman, L., Wollega, E., and Salas, W. (2020). Peer-to-peer energy trading: a review of the literature. Appl. Energy 283, 116268. doi:10.1016/j.apenergy.2020.116268
Teng, F., Zhang, Q., Wang, G., Liu, J., and Li, H. (2021). A comprehensive review of energy Blockchain: application scenarios and development trends. Int. J. Energy Res. 45 (12), 17515–17531. doi:10.1002/er.7109
Teufel, B., Sentic, A., and Barmet, M. (2019). Blockchain energy: Blockchain in future energy systems. J. Electron. Sci. Technol. 17 (4), 100011. doi:10.1016/j.jnlest.2020.100011
Torky, M., and Hassanein, A. E. (2020). “Integrating Blockchain and the internet of things in precision agriculture: analysis, opportunities, and challenges,” in Computers and electronics in agriculture (Elsevier).
Trejo, D. (2020) “Blockchain-based peer-to-peer energy trading,” in IEEE, PES transactive energy systems conference (TESC) (IEEE). 978-1-6654-2935-1/20.
Viriyasitavat, W., and Hoonsopon, D. (2018). Blockchain characteristics and consensus in modern business processes. J. Industrial Inf. Integration 13, 32–39. doi:10.1016/j.jii.2018.07.004
Wang, J. (2022). “Analyzing the application of Blockchain and artificial intelligence in new energy vehicle transactions from a data security perspective,” in IEEE, Sixth International Conference on Trends in Electronics and Informatics (ICOEI). 978-1-6654-8328-5/22.
Wang, Q., and Su, M. (2020). Integrating Blockchain technology into the energy sector — from theory of Blockchain to research and application of energy Blockchain. Comput. Sci. Rev. 37, 100275. doi:10.1016/j.cosrev.2020.100275
Wang, Z., Ogbodo, M., Huang, H., Qiu, C., Hisada, M., and Ben Abdallah, A. (2020). AEBIS: AI-enabled blockchain-based electric vehicle integration system for power management in smart grid platform. IEEE 8 (1), 226409–226421. doi:10.1109/access.2020.3044612
Wegrzyn, K., and Wang, E. (2021). “Blockchain in supply chain: article 2”. Manufacturing industry, advisor, innovative technology insights. Dashboard Insights. Available at: https://www.foley.com/en/insights/publications/2021/08/types-of-Blockchain-public-private-between/.
Xiao, W., Liu, C., Wang, H., Zhou, M., Hossain, M., Alrashoud, M., et al. (2021). Blockchain for secure-GaS: blockchain-powered secure natural gas IoT system with AI-enabled gas prediction and transaction in smart city. IEEE Internet Of Things J. 8 (8), 6305–6312. doi:10.1109/jiot.2020.3028773
Xinyi, Y., Yi, Z., and He, Y. (2018). “Technical characteristics and model of Blockchain,” in 10th International Conference on Communication Software and Networks, 562–566. 978-1-5386-7223-5/18. doi:10.1109/controlo.2018.8439793
Xu, M., Chen, X., and Kou, G. (2019). A systematic review of Blockchain. Financ. Innov. 5, 27. doi:10.1186/s40854-019-0147-z
Xu, Y., Yu, L., Bi, G., Zhang, M., and Shen, C. (2020). “Deep reinforcement learning and Blockchain for peer-to-peer energy trading among microgrids,” in International Conferences on Internet of Things (iThings) and IEEE Green Computing and Communications (GreenCom) and IEEE Cyber, Physical and Social Computing (CPSCom) and IEEE Smart Data (SmartData). 978-1-7281-7647-5/20.
Yan, Y., Zhao, J., Wen, F., and Chen, X. (2017). Blockchain in energy systems: concept, application and prospect. Electr. Power Constr. 38 (2). doi:10.3969/j.issn.1000-7229.2017.02.002
Yang, Q., and Wang, H. (2021). Privacy-preserving transactive energy management for IoT-aided smart homes via Blockchain. IEEE Internet Of Things J. 8 (14), 11463–11475. doi:10.1109/jiot.2021.3051323
Yang, S., Zhu, M. N., and Yu, H. (2024). Are artificial intelligence and Blockchain the key to unlocking the box of clean energy? Energy Econ. 134, 107616. doi:10.1016/j.eneco.2024.107616
Yap, K. Y., Chin, H. H., and Klemeš, J. J. (2023). Blockchain technology for distributed generation: a review of current development, challenges and future prospect. Renew. Sustain. Energy Rev. 175, 113170. doi:10.1016/j.rser.2023.113170
Yeow, K., Gani, A., Ahmad, R. W., Rodrigues, J. J., and Ko, K. (2018a). Decentralized consensus for edge-centric internet of things: a review, taxonomy, and research issues. IEEE Access 6, 1513–1524. doi:10.1109/access.2017.2779263
Yeow, K., Gani, A., Ahmad, R. W., Rodrigues, J. J. P. C., and Ko, K. (2018b). Decentralized consensus for edge-centric internet of things: a review, taxonomy, and research issues. IEEE Access 6, 1513–1524. doi:10.1109/access.2017.2779263
Yildizbasi, A. (2021). Blockchain and renewable energy: integration challenges in circular economy era. Renew. Energy 176, 183–197. doi:10.1016/j.renene.2021.05.053
Yin, S., Langenheldt, H., Harlev, K., Mukkamala, M., and Vatrapu, R. (2019). Regulating cryptocurrencies: a supervised machine learning approach to de-anonymizing the bitcoin Blockchain. J. Manag. Inf. Syst. 36 (1), 3773. doi:10.1080/07421222.2018.1550550
Yong, B., Shen, J., Liu, X., Li, F., Chen, H., and Zhou, Q. (2020). An intelligent Blockchain-based system for safe vaccine supply and supervision. Int. J. Inf. Manag. 52, 102024. doi:10.1016/j.ijinfomgt.2019.10.009
Zha, D. S., Feng, T. T., Gong, X. L., and Liu, S. Y. (2022). When energy meets Blockchain: a systematic exposition of policies, research hotspots, applications, and prospects. Int. J. Energy Res. 46 (3), 2330–2360. doi:10.1002/er.7398
Zhang, Z., Song, X., Liu, L., Yin, J., Wang, Y., and Lan, D. (2021). Recent advances in Blockchain and artificial intelligence integration: feasibility analysis, research issues, applications, challenges, and future work. Secur. Commun. Netw. 2021 (1), 1–15. doi:10.1155/2021/9991535
Zhao, S., Wang, B., Li, Y., and Li, Y. (2018). Integrated energy transaction mechanisms based on Blockchain technology. Energies 11 (9), 2412. doi:10.3390/en11092412
Zheng, X., Zhu, M., Li, Q., Chen, C., and Tan, Y. (2018a). Finbrain: when finance meets ai 2.0. arXiv Prepr. arXiv:1808.08497. doi:10.1631/FITEE.1700822
Zheng, Z., Xie, S., Dai, H. N., Chen, W., Chen, X., Weng, J., et al. (2020). An overview on smart contracts: challenges, advances and platforms. Future Gener. Comput. Syst. 105, 475–491. doi:10.1016/j.future.2019.12.019
Zheng, Z., Xie, S., Dai, H. N., and Chen, X. (2018b). Blockchain challenges and opportunities: a survey. Int. J. web grid Serv. 14 (4), 352–375. doi:10.1504/ijwgs.2018.10016848
Zheng, Z., Xie, S., Dai, H.-N., and Wang, H. (2016). Blockchain challenges and opportunities: a survey. Work Pap. 2016. doi:10.1504/IJWGS.2018.095647
Keywords: energy sector, blockchain, artificial intelligence, decentralized, smart contract, transaction
Citation: Al Shareef AM, Seçkiner S, Eid B and Abumeteir H (2024) Integration of blockchain with artificial intelligence technologies in the energy sector: a systematic review. Front. Energy Res. 12:1377950. doi: 10.3389/fenrg.2024.1377950
Received: 28 January 2024; Accepted: 30 September 2024;
Published: 23 October 2024.
Edited by:
Haris M. Khalid, University of Dubai, United Arab EmiratesReviewed by:
Peiman Ghasemi, University of Vienna, AustriaLeo Raju, SSN College of Engineering, India
Copyright © 2024 Al Shareef, Seçkiner, Eid and Abumeteir. This is an open-access article distributed under the terms of the Creative Commons Attribution License (CC BY). The use, distribution or reproduction in other forums is permitted, provided the original author(s) and the copyright owner(s) are credited and that the original publication in this journal is cited, in accordance with accepted academic practice. No use, distribution or reproduction is permitted which does not comply with these terms.
*Correspondence: Al Mothana Al Shareef, ZW5ncjFzdEBnbWFpbC5jb20=
†Present address: Hasan Abumeteir, Department of Electrical-Electronic Engineering, Gaziantep İslam Bilim ve Teknoloji Üniversitesi, Gaziantep, Türkiye