- 1Department of Chemical and Biochemical Engineering, Rutgers the State University of New Jersey, Piscataway, NJ, United States
- 2National Renewable Energy Lab, Biosciences Center, Golden, CO, United States
Non-productive binding of cellulolytic enzymes to various plant cell wall components, such as lignin and cellulose, necessitates high enzyme loadings to achieve efficient conversion of pretreated lignocellulosic biomass to fermentable sugars. Protein supercharging was previously employed as one of the strategies to reduce non-productive binding to biomass. However, various questions remain unanswered regarding the hydrolysis kinetics of supercharged enzymes towards pretreated biomass substrates and the role played by enzyme interactions with individual cell wall polymers such as cellulose and xylan. In this study, CBM2a (from Thermobifida fusca) fused with endocellulase Cel5A (from T. fusca) was used as the model wild-type enzyme and CBM2a was supercharged using Rosetta, to obtain eight variants with net charges spanning −14 to +6. These enzymes were recombinantly expressed in E. coli, purified from cell lysates, and their hydrolytic activities were tested against pretreated biomass substrates (AFEX and EA treated corn stover). Although the wild-type enzyme showed greater activity compared to both negatively and positively supercharged enzymes towards pretreated biomass, thermal denaturation assays identified two negatively supercharged constructs that perform better than the wild-type enzyme (∼3 to 4-fold difference in activity) upon thermal deactivation at higher temperatures. To better understand the causal factor of reduced supercharged enzyme activity towards AFEX corn stover, we performed hydrolysis assays on cellulose-I/xylan/pNPC, lignin inhibition assays, and thermal stability assays. Altogether, these assays showed that the negatively supercharged mutants were highly impacted by reduced activity towards xylan whereas the positively supercharged mutants showed dramatically reduced activity towards cellulose and xylan. It was identified that a combination of impaired cellulose binding and lower thermal stability was the cause of reduced hydrolytic activity of positively supercharged enzyme sub-group. Overall, this study demonstrated a systematic approach to investigate the behavior of supercharged enzymes and identified supercharged enzyme constructs that show superior activity at elevated temperatures. Future work will address the impact of parameters such as pH, salt concentration, and assay temperature on the hydrolytic activity and thermal stability of supercharged enzymes.
Introduction
The future circular economy is based on conversion of wastes from a variety of streams to useful products that are currently produced from fossil fuels (Tuck et al., 2012; Ubando et al., 2020). Bioethanol is one such product that can be produced from lignocellulosic biomass such as agricultural residues (e.g., corn stover, wheat/rice straws, sugarcane bagasse) and forest residues (e.g., wood chips) (Chundawat et al., 2011a). The versatility of available biomass sources and the variety of bioproducts that can be generated, lends itself to development of customized conversion strategies tailor-made for various feedstocks in an integrated biorefinery (Kokossis et al., 2014; Maity, 2015). One conversion strategy that has received significant attention is the enzymatic conversion of cellulose and hemicellulose to C6/C5 based mixed sugar streams (Chundawat et al., 2011a), while employing tailored valorization strategies for extracted lignin based on the pretreatment strategy (Ragauskas et al., 2014; Wang et al., 2019). These sugars can be converted to a variety of platform chemicals such as ethanol, organic acids, or polymer-precursors in an integrated biorefinery (Takkellapati et al., 2018).
Various techno-economic analyses have been performed to assess the feasibility of producing bioethanol in a cost-effective and sustainable manner from biomass (Humbird et al., 2011; Scown et al., 2021). These studies have highlighted the role of high enzyme costs prohibiting commercialization of biofuels (Klein-Marcuschamer et al., 2012). Hence, there is a need to develop enzyme engineering strategies to improve the overall conversion of lignocellulosic biomass to reducing sugars, while reducing biomass recalcitrance via thermochemical pretreatment (McCann and Carpita, 2015; Holwerda et al., 2019). Non-productive binding of enzymes to lignin and cellulose along with limited enzyme accessibility to the substrate are considered the key factors that limit enzyme activity towards pretreated biomass substrates (Studer et al., 2011; Zeng et al., 2014; Strobel et al., 2015; Nemmaru et al., 2021). As a result, pretreatment efforts have focused on extraction of lignin for valorization while also improving overall enzyme accessibility to the residual polysaccharides (Narron et al., 2016; Galbe and Wallberg, 2019).
However, most modes of pretreatment technologies (e.g., dilute acid, extractive ammonia, alkaline, deacetylation and mechanical refining or DMR) only extract lignin partially, leaving behind residual lignin that can still deactivate or inhibit enzymes (Chundawat et al., 2011b; Chen et al., 2016). Lignin has been shown to deactivate cellulases through various mechanisms, the most significant of which involves protein conformational changes upon adsorption to lignin driven via hydrophobic interactions (Salas et al., 2013; Guo et al., 2014; Sammond et al., 2014). Broadly speaking, the three strategies that have been employed to reduce cellulase non-productive binding to lignin include: (i) addition of sacrificial proteins such as BSA (Yang and Wyman, 2006) or soy protein (Luo et al., 2019), (ii) inclusion of negatively charged groups such as acetyl groups on the surface of enzymes via chemical conjugation (Nordwald et al., 2014), and (iii) enzyme surface supercharging via computational re-design (Haarmeyer et al., 2017; Whitehead et al., 2017). Although the first two strategies have been shown to reduce lignin inhibition, they require an additional reagent (BSA or soy protein) or treatment procedure (acetylation), which increases the operating or capital cost of the bioconversion process. On the other hand, enzyme supercharging is an inexpensive method of genetically engineering enzymes to alter their surface electrostatic properties (Lawrence et al., 2007; Der et al., 2013).
Protein supercharging has been used to accomplish a variety of useful applications including but not limited to macromolecule or drug delivery into mammalian cells (Thompson et al., 2012), DNA detection and methylation analysis (Lei et al., 2014), complex coacervation with polyelectrolytes (Obermeyer et al., 2016), self-assembly into organized structures (Simon et al., 2019) such as protein nanocages (Sasaki et al., 2017) and Matryoshka-type structures (Beck et al., 2015) and encapsulation of cargo proteins into such higher-order structures (Azuma et al., 2016). Previously, we have utilized a supercharging strategy based on Rosetta (Das and Baker, 2008; Alford et al., 2017) and FoldIt standalone interface (Kleffner et al., 2017) for engineering green fluorescent protein (GFP) (Haarmeyer et al., 2017) and CelE (from Ruminiclostridium thermocellum) (Whitehead et al., 2017). We found that net negative charge was correlated weakly with reduced lignin binding capacity for GFP supercharged mutants, whereas the charge density was not found to have a clear impact on lignin binding capacity (Haarmeyer et al., 2017). In our follow-up study (Whitehead et al., 2017), a cellulase catalytic domain CelE was fused with CBM3a and both domains were individually negatively supercharged. Negatively supercharged CBM3a designs showed relatively improved hydrolysis yields on model amorphous cellulose in the presence of lignin, compared to the wild-type enzyme. However, all tested designs showed reduced absolute activity than wild-type controls on amorphous cellulose substrates (and with no data reported on pretreated lignocellulosic biomass) which was hypothesized to be due to reduced binding to cellulose induced by electrostatic repulsions.
Although these studies show proof-of-concept for the potential beneficial impact of cellulase negative supercharging on biomass hydrolysis, there remain multiple unanswered mechanistic questions to fully leverage the potential of enzyme supercharging for lignocellulosic biomass hydrolysis. Broadly speaking, there are three unanswered questions: (i) how does supercharging impact enzyme kinetics on pretreated biomass substrates? (ii) how can biomass hydrolysis performance of mutant enzymes be rationalized by understanding the activity and binding on individual polymers (cellulose, xylan, and lignin)? (iii) how does supercharging impact thermal stability and cellulase function at elevated temperatures? Here, we sought to address these questions in greater detail, using a model endocellulase enzyme Cel5A from T. fusca (Thermobifida fusca), which has been well-characterized in our lab previously (Liu et al., 2020). T. fusca is a thermophilic microbe that secretes cellulase enzymes belonging primarily to glycosyl hydrolase (GH) families 5, 6, 9, and 48, with most cellulase CDs tethered to a type-A CBM2a (Wilson, 2004). Testing the protein supercharging strategy on a model Cel5A enzyme and its CBM2a from this cellulolytic enzyme system will also allow for extension of these design principles to other enzymes, potentially leading to a supercharged cellulase mixture with superior performance.
More specifically, we computationally designed a library of eight CBM2a designs spanning a net charge range of −14 to +6. These CBM2a designs were fused with the Cel5A catalytic domain and green fluorescent protein (GFP) separately, to study the hydrolysis activity and binding behavior of the constructs on a variety of substrates, respectively. Firstly, we characterized the hydrolysis yields of CBM2a-Cel5A fusion constructs at various reaction times (2–24 h) on ammonia fiber expansion (AFEX) and extractive ammonia (EA) pretreated corn stover substrates. To further rationalize the activity of supercharged enzymes towards pretreated biomass substrates, we assayed enzyme activity towards cellulosic substrates and xylan. Moreover, we performed binding assays to study the binding of GFP-CBM2a fusion constructs to cellulose using previously established QCM-D assay procedures (Haarmeyer et al., 2017; Nemmaru et al., 2021). We followed it up with thermal shift assays to measure melting temperatures of supercharged enzymes and tested enzyme activity upon thermal deactivation at elevated temperatures. Overall, this study presents a rational approach to understand the mechanistic underpinnings of supercharged enzyme action on pretreated biomass substrates by deconvoluting the impact of cellulose and xylan hydrolysis and thermal stability.
Experimental section
Reagents
AFEX and EA pretreated corn stover were prepared and provided in kind by Dr. Rebecca Ong’s lab (Michigan Technological University, Houghton) and Bruce Dale’s lab (Michigan State University, East Lansing), according to previously established protocols (Da Costa Sousa et al., 2016; Sousa et al., 2019; Chundawat et al., 2020). Avicel (PH 101, Sigma-Aldrich, St Louis) was used to prepare cellulose-III allomorph with the following pretreatment conditions (90°C, 6:1 anhydrous liquid ammonia to cellulose loading, and 30 min of total residence time) and phosphoric acid swollen cellulose (PASC) as described previously (Chundawat et al., 2011c). Sarvada Chipkar from the Ong lab kindly prepared and provided cellulose-III used in this study. Lignin extracted from corn stover was prepared using the organosolv extraction process (Bozell et al., 2011) and kindly provided by Stuart Black of the National Renewable Energy Laboratory (NREL). All other chemicals and analytical reagents were procured either from Fisher Scientific or Sigma Aldrich, or as noted in the relevant experimental section.
Mutant energy scoring using rosetta
Creation of computational designs carrying a certain net charge necessitated computing the change in energy scores upon mutation of a native amino acid residue to either a positively charged (K, R) or a negatively charged residue (D, E). These mutations were scored using Rosetta. The wild-type protein PDB file is obtained either via homology modeling using Rosetta CM49 or via the protein data bank (Burley et al., 2021). PyMOL (Schrodinger) was used to generate the desired mutation in amino acid sequence of a given protein and exported as a PDB file that represents the mutated protein. Customized scripts were developed in Rosetta to perform fast relax (Khatib et al., 2011) of any input PDB file. PDB files of both the wild-type and mutated proteins were relaxed separately using ten fast relax operations at a time. Each round of energy minimization enabled by ten fast relax operations was repeated until the Rosetta energy score of protein equilibrated and did not vary by more than 0.1 Rosetta Energy Units (REU) between one round of energy minimization (comprising of 10 fast relaxes) to another. The mutation energy score for a given mutation was calculated by measuring the difference between Rosetta energy scores of the wild-type protein and the mutant after energy minimization.
Plasmid generation, protein expression and purification
Thermobifida fusca native Cel5A (Watson et al., 2002; Jung et al., 2003) (UniprotKB–Q01786) gene was cloned into pET28a(+) (Novagen) and was kindly provided by Nathan Kruer-Zerhusen (from late Prof. David Wilson’s lab at Cornell University). An N-terminal 8X His tag was inserted and the native signal peptide removed from the original gene construct. The gene was then cloned into our in-house expression vector pEC to optimize protein expression yields as described previously (Blommel et al., 2009; Lim et al., 2014). The plasmid maps for pEC-CBM2a-Cel5A and pEC-GFP-CBM2a are provided in Supplementary Figures S1, S2 respectively. The full nucleotide sequences with color coding for each gene segment are reported in the Supplementary Material titled SI_Appendix_Sequences.docx. CBM2a mutant designs were ordered from Integrated DNA Technologies, Inc (IDT) as custom-synthesized gBlocks. These CBM2a design gBlocks were then swapped with wild-type CBM2a to generate mutant CBM2a-Cel5A fusion constructs using standard sequence and ligation independent cloning (SLIC) protocols. A similar approach was used to insert CBM2a designs into previously reported pEC-GFP-CBM vector (Lim et al., 2014). Molecular cloning for T. fusca β-glucosidase (UniprotKB–Q9LAV5) gene These colonies were then inoculated in LB medium and grown overnight to prepare 20% glycerol stocks for long-term storage at −80°C. These glycerol stocks were then used to inoculate 25 mL of LB media with 50 μg/mL kanamycin and incubated at 37°C, 200 rpm for 16 h. These overnight cultures were then transferred to 500 mL auto-induction medium (TB + G) (Studier, 2005) and incubated at 37°C, 200 rpm for 6 h to allow optical density to reach the exponential regime. Protein expression was then induced by reducing the temperature to 25°C for 24 h at 200 rpm. Cell pellets were then harvested using Beckman Coulter centrifuge and JA-14 rotor by spinning the liquid cultures in 250 mL plastic bottles at 30,100 g for 10 min at 4°C. All the cell culturing experiments were performed using an Eppendorf Innova™ incubator shaker. Cell pellets were lysed using 15 mL cell lysis buffer (20 mM phosphate buffer, 500 mM NaCl, 20% (v/v) glycerol, pH 7.4), 0.5 mM Benzamidine (Calbiochem 199,001), 200 µL protease inhibitor cocktail (1 µM E-64 (Sigma Aldrich E3132), 15 µL lysozyme (Sigma Aldrich, USA) and 1 mM EDTA (Fisher Scientific BP1201)) for every 3 g wet cell pellet. The cell lysis mixture was sonicated using Misonix™ sonicator 3,000 for 5 min of total process time at 4.5 output level and specified pulse settings to avoid sample overheating (pulse-on time: 10 s and pulse-off time: 30 s). An Eppendorf centrifuge (5810R) with F-34-6-28 rotor was then used to separate the cell lysis extract from insoluble cellular debris at 15,500 g, 4°C for 45 min. Immobilized metal affinity chromatography (IMAC) using His-Trap FF Ni+2-NTA column (GE Healthcare) attached to BioRad™ NGC system, was then performed to purify the his-tagged proteins of interest from the background of cell lysate proteins. Briefly, there were three steps involved during IMAC purification: 1. equilibration of column in buffer A (100 mM MOPS, 500 mM NaCl, 10 mM imidazole, pH 7.4) at 5 mL/min for five column volumes, 2. Soluble cell lysate loading at 2 mL/min, and 3. His-tagged protein elution using buffer B (100 mM MOPS, 500 mM NaCl, 500 mM imidazole, pH 7.4). The purity of eluted proteins was validated using SDS-PAGE before buffer exchange into 10 mM sodium acetate (pH 5.5) buffer for long-term storage after flash freezing at −80 °C and/or follow-on activity characterization.
Pretreated lignocellulosic biomass hydrolysis assays
AFEX and EA corn stover (milled to 0.5 mm) were suspended in deionized water to obtain slurries of 25 g/L total solids concentration. All biomass hydrolysis assays were performed in 0.2-mL round-bottomed microplates (PlateOne™), with at least four replicates for each reaction condition. Reactions quenched at different time points (2, 6 and 24 h) were performed in different microplates. Each reaction was composed of 80 μL biomass slurry (25 g/L), 20 μL sodium acetate buffer (0.5 M), 50 μL cellulase enzyme (at appropriate concentration), 25 μL β-glucosidase (at appropriate concentration), and 25 μL of deionized water to make up the total reaction volume to 200 μL. For reaction blanks, the enzyme solutions were replaced with deionized water while biomass slurry and buffer volumes remained the same. The cellulase enzyme loading was maintained at 120 nmol per Gram biomass substrate and the β-glucosidase enzyme loading was maintained at 12 nmol per Gram biomass substrate (leading to 10% of cellulase enzyme concentration). Since supercharged constructs have varying molecular weights, a molar basis was used for all hydrolysis assays to keep concentrations between enzymes normalized. A conversion of enzyme loading for each concentration to a mass basis can be viewed in the Supplementary Appendix SA1. Upon addition of all the requisite reaction components, the microplates were covered with a plate mat, sealed with packaging tape, and incubated at 60°C for the specified time duration (2, six or 24 h) with end-over-end mixing at 5 rpm in a VWR hybridization oven. Upon reaction completion, the microplates were centrifuged at 3,900 rpm for 10 min at 4°C to separate the soluble supernatant (comprised of soluble reducing sugars) from insoluble biomass substrate. The supernatants were then recovered and dinitrosalicylic acid (DNS) assays were performed as previously described to estimate total soluble reducing sugars (Liu et al., 2020). This data was fitted to a two-parameter kinetic model that was previously deployed to study reaction kinetics of T. fusca cellulases on biomass substrates (Kostylev and Wilson, 2013). Origin software was used to perform the curve fitting analysis and obtain the pseudo-kinetic time-dependent parameters ‘A’ and ‘b’ which represent the net activity of bound enzyme and the time-dependent ability of enzyme to overcome recalcitrance, respectively. An increase in b might indicate the ability of enzyme to sample new substrate sites as reaction progresses, thereby reducing substrate recalcitrance.
Cellulose hydrolysis assays and lignin inhibition assays
The cellulose hydrolysis assays were performed in a similar manner as biomass hydrolysis assays, except for the reaction composition. Avicel PH101 derived cellulose-I and cellulose-III were suspended in deionized water to form slurries of 100 g/L total solids concentration. A 0.2-mL round-bottomed microplate (PlateOne™) was used for each discrete reaction timepoint (2, 6 and 24 h) and each reaction was performed with at least four replicates. Each reaction was composed of 40 μL cellulose slurry (100 g/L), 20 μL sodium acetate buffer (0.5 M, pH 5.5), 50 μL cellulase enzyme (at appropriate concentration), 25 μL β-glucosidase (at appropriate concentration) and 65 μL of deionized water to make up the total reaction volume to 200 μL. The cellulase enzyme loading was maintained at 120 nmol per Gram biomass substrate and the β-glucosidase enzyme loading was maintained at 12 nmol per Gram biomass substrate (leading to 10% of cellulase enzyme concentration). Upon reaction completion, supernatants were removed, and DNS assays were performed as described in the previous section on biomass hydrolysis assays. The reaction mixture for lignin inhibition assays was composed of 20 μL cellulose slurry (100 g/L), 40 µL lignin slurry (20 g/L), 20 μL sodium acetate buffer (0.5 M, pH 5.5), 50 μL cellulase enzyme (at appropriate concentration), 25 μL β-glucosidase (at appropriate concentration) and 65 μL of deionized water to make up the total reaction volume to 200 μL. The enzyme loadings and all the follow-on steps were conducted in a similar manner to cellulose hydrolysis assays. 24 h was used as the preferred reaction time for lignin inhibition assays, owing to the prevalence of lignin and cellulose non-productive binding at longer reaction times.
Xylan hydrolysis assays
The xylan hydrolysis assays were performed in a similar manner as biomass hydrolysis assays, with a slight change to the reaction composition. Beechwood xylan suspended in deionized water to form slurries of 100 g/L total solids concentration. Equipment, procedures and reaction timepoint remained the same. Each reaction was composed of 20 μL xylan slurry (100 g/L), 20 μL sodium acetate buffer (0.5 M, pH 5.5), 50 μL cellulase enzyme (at appropriate concentration), 25 μL β-glucosidase (at appropriate concentration) and 85 μL of deionized water to make up the total reaction volume to 200 μL. The cellulase enzyme loading was maintained at 120 nmol per Gram xylan substrate and the β-glucosidase enzyme loading was maintained at 12 nmol per Gram biomass substrate (leading to 10% of cellulase enzyme concentration). All the follow-on steps were conducted in a similar manner to biomass hydrolysis assays.
pNPC kinetic hydrolysis assays
The pNPC hydrolysis assays were adapted from previously established protocols laid out in Whitehead et al. (2017). The assay was conducted in a 0.2-mL flat-bottomed clear microplate (PlateOne™) and the enzyme activity was tested at pH 5.5 and pH 7.5. Each reaction was composed of 100 µL pNPC slurry (2 mM), 7.5 µL 1 M sodium acetate buffer pH 5.5 or 7.5 µL 1 M MOPS (pH 7.5), 42.5 µL cellulase enzyme (at an appropriate concentration to constitute five ug of enzyme per g pNPC). The reaction was performed for a duration of up to 700 min and the progress of hydrolysis reaction was tracked via pNP absorbance through a UV-vis spectrophotometer.
Quartz crystal microbalance with dissipation (QCM-D) based binding assays
Preparation of cellulose and lignin films for characterization of GFP-CBM binding, was performed as described elsewhere (Brunecky et al., 2020; Nemmaru et al., 2021). Quartz sensors functionalized with nanocrystalline cellulose or lignin were mounted on the sensor holder of QSense E4 instrument and equilibrated with buffer (50 mM sodium acetate, pH 5.5 with 100 mM NaCl) for 10 min at a flow rate of 100 μL/min using a peristaltic pump. The films were left to swell in buffer overnight and the films were considered stable if the third harmonic reached a stable baseline after overnight incubation. GFP-CBM2a protein stocks were then diluted to a concentration of 2.5 μM using 50 mM sodium acetate (pH 5.5) and flown over the sensors at a flow rate of 100 μL/min for 10–15 min until the system reached saturation, as observed by the third harmonic. The system was then allowed to equilibrate for at least 30 min and protein unbinding was then tracked by flowing buffer (50 mM sodium acetate, pH 5.5 with 100 mM NaCl) over the sensors at a flow rate of 100 μL/min for at least 30 min. Data analysis for QCM-D traces was performed as described previously (Nemmaru et al., 2021). However, for lignin, binding was observed to be mostly irreversible (Gao et al., 2014) and hence, only the maximum number of binding sites and percent irreversible protein bound, calculated based on the maximum number of binding sites and the amount of protein bound towards the end of unbinding regime.
Pretreated biomass/cellulose hydrolysis assays with thermally treated enzymes
This assay was performed in a similar way to the pretreated lignocellulosic biomass hydrolysis assays and the cellulose hydrolysis assays described above. However, the enzyme dilution used in those assay procedures was exposed to 70°C in an Eppendorf thermocycler for 30 min followed by 10°C for 10 min directly before being added into the microplate for reaction. The reaction was incubated for 60°C for 24 h only. The initial assay used all the enzyme designs with a denaturation temperature of 70°C. From this the thermally stable enzyme designs, D1, D2 and the WT were exposed to temperatures of 73°C, 76°C, and 79°C for 30 min prior to incubation at 60°C for 24 h.
Cellulase thermal shift assay
The protocol for thermal shift assays was similar to that reported previously (Whitehead et al., 2017). Briefly, 5 µL 200X SYPRO reagent, 5 µL 0.5 M sodium acetate buffer (pH 5.5), enzyme dilution to make up an effective concentration of 5 µM and deionized water to make up the total volume to 50 µL were added to MicroAmp™ EnduraPlate™ 96-well clear microplate (Applied Biosystems™). QuantStudio3 (Applied Biosystems™) was then used to measure the fluorescence using the channel allocated to FAM dye (excitation: 470 nm, emission: 520 nm) under a temperature ramp from 25°C to 99°C at a rate of 0.04°C per second. The melting curves obtained were then analyzed using an open-source tool called SimpleDSFViewer (Sun et al., 2020).
Results and discussion
Selection of a wild-type construct from CBM family two for supercharging
CBM family two comprises a large collection of mostly bacterial CBMs, with ∼11,000 entries and 10 solved structures. T. fusca, an industrially relevant cellulolytic microbe, secretes multi-modular cellulase enzymes comprised of CBMs from family 2. Cel5A (endocellulase from GH5) was chosen as the model cellulase and tested for expression and activity, both with its native CBM2a and CBM2a from exocellulase Cel6B. The objective was to fuse each CBM to the Cel5A catalytic domain and identify the fusion enzyme that shows greater thermal stability as a target for supercharging. These two fusion cellulases are labeled as CBM2a (native) Cel5A and CBM2a (Cel6B) Cel5A from hereon. Supplementary Figure S3 shows the hydrolytic activity of both enzyme constructs towards AFEX corn stover and cellulose-I. Surprisingly, CBM2a (Cel6B) Cel5A showed 1.8 to 2.5-fold improvement in activity towards both substrates compared to CBM2a (native) Cel5A, across all timepoints considered. This experiment was followed up with a measurement of enzyme activity upon thermal treatment at 70°C, as reported in Supplementary Figure S4. CBM2a (Cel6B) Cel5A loses ∼60% of activity towards both substrates (AFEX corn stover and cellulose-I) whereas CBM2a (native) Cel5A loses up to ∼90% activity. The fusion of the cellulose binding module CBM2a from Cel6B with the catalytic domain from Cel5A showed the greatest hydrolytic activity and thermal resistance. As a result, we chose CBM2a (Cel6B) Cel5A as the wild-type construct to be engineered in this study. CBM2a (Cel6B) Cel5A will be referred to as wild-type CBM2a Cel5A or WT for the remainder of this paper.
Design of supercharged CBM2a library
A homology model was constructed for the target CBM2a (Cel6B) wild-type protein using Rosetta CM tool (Song et al., 2013) based on templates from CBM family 2a with at least 50% sequence identity. Surface residues were then identified using an appropriate residue selector in Rosetta. Previous studies have shown that 10% of the total amino acid sequence length of globular proteins can be mutated using the supercharging strategy, while still allowing the proteins to fold properly (Lawrence et al., 2007). Given that CBM2a is 100 amino acids long and has a net charge of −4, we sought to generate designs that spanned a net charge range of −14 to +6 using 10 mutations of polar uncharged amino acid residues. Overall, 31 polar uncharged amino acid residues were identified on the protein surface and these residues were scored individually for mutations to lysine (K), arginine (R), aspartic acid (D) and glutamic acid (E).
The mutation energy scores were then averaged for any given position and surface polar uncharged residues were sorted based on these average mutation energy scores. From the original pool of 31 polar uncharged residues, three categories of residues were considered immutable due to their potential implications for protein folding or interaction with cellulose as follows: 1. residues within 10 Å distance from evolutionarily conserved planar aromatic residues (Georgelis et al., 2012) essential for CBM function, 2. residues on the CBM binding face (Nimlos et al., 2012), and 3. residues with a positive average mutation energy score (predicting structural instability upon mutation). Upon exclusion of these three categories of residues, 11 mutable polar uncharged residues were identified and sorted into two spatially distinct clusters and sorted based on their mutation energy scores from highest to lowest. The individual and average mutation energy scores of mutable residues are reported in Supplementary Table S1. Eight designs were then generated to have net charges of −14 (D1), −12 (D2), −10 (D3), −8 (D4), −6 (D5), −2 (D6), +2 (D7) and +6 (D8) as shown in Figure 1. Negatively supercharged space was sampled more granularly because negative supercharging has been shown to reduce lignin inhibition in our previous work (Whitehead et al., 2017). The mutations used to generate each design, are reported in Supplementary Table S2 whereas the full amino acid sequence for wild-type CBM2a with these mutable residues highlighted in red font, are reported in a separate file titled ‘SI_Appendix_Sequences.docx’.
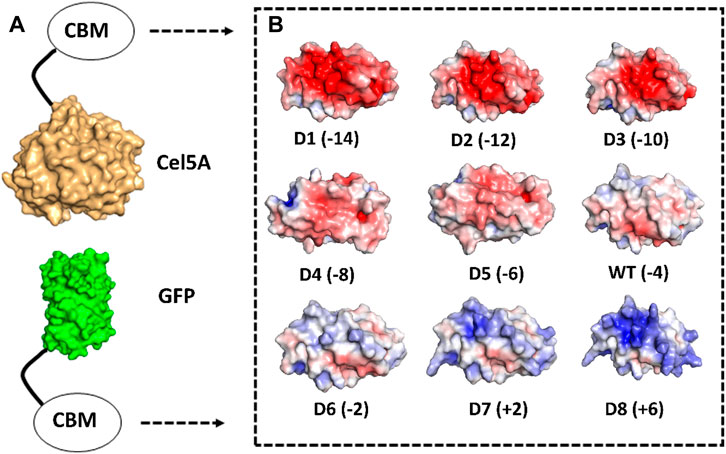
Figure 1. Computational design of supercharged CBM2a mutants and generation of fusion protein constructs. Rosetta was used to identify amino acids on the surface of CBM2a wild-type protein which are amenable to positively charged (K, R) or negatively charged (D, E) amino acid mutations to achieve a target net charge spanning the −14 to +6 range. (A) CBM2a designs were fused with Cel5A and GFP separately. (B) Electrostatic potential maps of the 8 CBM2a designs and their wild-type (represented as WT) are generated using APBS Electrostatics Tool in PyMOL. The name of each construct (D1–D8 and WT) is followed by the net charge of each design in parenthesis.
Hydrolytic activities of supercharged CBM2a-Cel5A constructs towards pretreated biomass
All CBM2a-Cel5a designs were cloned, expressed, and purified as described in the experimental procedures section. The hydrolytic activity of the supercharged and wild type cellulases were tested against pretreated biomass, namely, AFEX corn stover. The hydrolysis yields are reported in the form of glucose equivalent reducing sugars released at three time points (2 h, 6 h and 24 h) resulting in reaction progress curves shown in Figure 2. Based on the raw hydrolysis data reported in Figure 2A, the negatively supercharged and positively supercharged mutants were separated into two groups and their average hydrolysis yields were reported in Figure 2B.
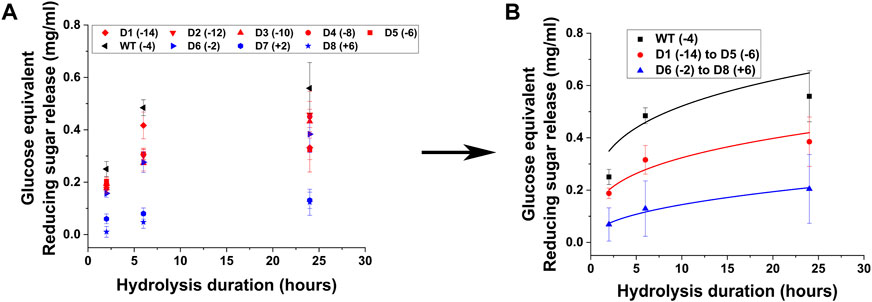
Figure 2. Hydrolytic activity of supercharged cellulases towards AFEX Corn Stover. 80 μL of 25 g/L AFEX Corn Stover was hydrolyzed using an enzyme loading of 120 nmol CBM2a Cel5A fusion enzyme per Gram biomass substrate with 12 nmol β-glucosidase enzyme (10% of cellulase loading) per Gram biomass substrate for reaction times of 2, 6, and 24 hrs. The solubilized reducing sugar concentrations in the supernatant after hydrolysis were determined by the DNS assay (A) Glucose equivalent reducing sugar release (mg/mL) as a function of time (2, 6, and 24 h) for the hydrolysis of AFEX Corn Stover by D1–D8 CBM2a Cel5A and WT CBM2a Cel5A. Error bars represent standard deviation from the mean, based on at least four replicates. (B) Based on the data reported in (A), CBM2a Cel5A fusion constructs with negatively supercharged CBMs (D1–D5) were grouped together and average hydrolysis yields were obtained for the group, with the error bars representing standard deviation from the mean. Similarly, CBM2a-Cel5A fusion constructs with positively supercharged CBMs (D6–D8) were grouped together and average hydrolysis yields were obtained. Trend curves have been added to represent the kinetic profiles of the hydrolysis reaction. Wild-type CBM2a-Cel5A is referred to as WT throughout this figure.
From Figure 2A, it is evident that the wild-type enzyme (WT) has the highest activity compared to any supercharged mutant. The wild-type showed ∼1.2 to 1.8-fold greater activity compared to negatively supercharged mutants and ∼1.5 to 24.1-fold greater activity compared to positively charged mutants. D6 was an outlier amongst the positively supercharged group, showing higher activity compared to D7 and D8 across all timepoints considered. Similar trends were observed with EA corn stover, another model pretreated biomass substrate, for which the hydrolysis yields are reported in a similar format in Supplementary Figure S5. To further compare the hydrolysis yields of mutants on AFEX and EA corn stover, T-tests were performed between each mutant pair within the negatively supercharged (D1-D5) and positively supercharged (D6-D8) groups, as reported in Supplementary Table S3. On AFEX corn stover, mutants within D1–D5 group were found to not show statistically significant differences at 2 h although certain mutant pairs showed p < 0.05 at 6 h and 24 h. Within D6–D8, D6 showed statistically significant differences from D7 and D8 at most timepoints. To understand the behavior of each group compared to the wild-type, the activities of negatively supercharged mutants (D1–D5) and positively supercharged mutants (D6–D8) were averaged separately and reported in Figure 2B. Positively supercharged mutants ranked the least as a group, at every time point considered, followed by negatively supercharged mutants with the wild type consistently ranking higher than both.
The reaction kinetic data on AFEX and EA corn stover for each individual mutant was then fit to a two-parameter model as described previously (shown in Supplementary Table S4). Parameter ‘A’ represents the net activity of the bound enzyme whereas parameter ‘b’ represents the enzyme’s ability to reduce biomass recalcitrance over time. On AFEX corn stover, the wild-type showed ∼0.8 to 1.5-fold improvement in parameter ‘A’ over the negatively supercharged enzymes and ∼2 to 6-fold improvement over positively supercharged enzymes. D1 was the only mutant to show an improvement in ‘A’ over wild-type indicating that the net activity of bound enzyme for this mutant may have been greater than the wild-type but the mutant perhaps lacks the ability to access new binding sites that can reduce recalcitrance of the enzyme. Similar trends were observed for EA corn stover, with D1 being the only mutant to show improvement in A.
Since electrostatic interactions between supercharged mutants and biomass may be influenced by the presence of salt, a hydrolysis assay was run at the 2-h timepoint in the presence of 100 mM NaCl (see Supplementary Figure S6). The presence of salt showed little to no impact for most mutants, except in the case of D7 on AFEX corn stover for which the presence of salt improved activity by more than 2-folds.
Overall, the wild type showed improved activity compared to all the supercharged mutants. The trends observed for the different cellulases towards pretreated biomass could arise from a combination of various factors: (i) cellulolytic activity, (ii) xylanolytic activity, (iii) lignin interactions, or (iv) thermal stability. We designed specific assays to understand each of these contributions to pretreated biomass hydrolysis as discussed below.
Hydrolytic activities of supercharged CBM2a-Cel5A constructs towards cellulosic substrates
Hydrolysis yields for cellulose-I were measured in terms of reducing sugar release at three time points (2 h, 6 h and 24 h) using 120 nmol enzyme per Gram substrate loading, resulting in reaction progress curves shown in Figure 3A for Avicel Cellulose-I and Supplementary Figure S7A for Avicel Cellulose-III. Cellulose-I and Cellulose-III were chosen as the target substrates because these are the predominant cellulose allomorphs that comprise AFEX corn stover and EA corn stover respectively. The wild-type showed activity that was ∼0.8 to 1.2-folds compared to negatively supercharged mutants (D1–D5) and ∼0.9 to 4.5-folds that of positively supercharged mutants (D6–D8). Unlike the trends observed towards pretreated biomass in the previous section, negatively supercharged mutants (D1–D5) show either increased or comparable activities to the wild type. On cellulose-III, most negatively supercharged mutants performed better than the wildtype, as observed in Supplementary Figure S7A. T-tests revealed that there were no statistically significant differences between each mutant pair in the negatively supercharged group (D1–D5) or within the negatively supercharged group (D6–D8), with a few exceptions (see Supplementary Table S5). To understand the behavior of each group compared to the wild-type, the activities of negatively supercharged mutants (D1–D5) and positively supercharged mutants (D6–D8) were averaged separately and reported in Figure 3B and Supplementary Figure S7B for Cellulose-I and Cellulose-III respectively. The two-parameter kinetic model fits, achieved as described for biomass, are reported for cellulose-I and cellulose-III in Supplementary Table S6.
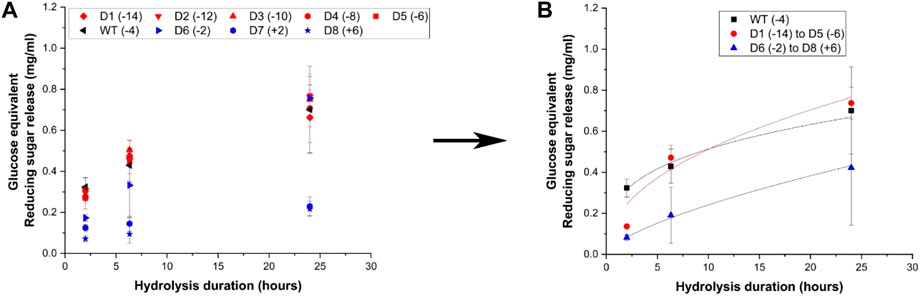
Figure 3. Hydrolytic activity of supercharged cellulases towards Avicel cellulose-I. 40 μL of 100 g/L Avicel cellulose-I substrate was hydrolyzed using an enzyme loading of 120 nmol CBM2a Cel5A fusion enzyme per Gram cellulose substrate supplemented with 12 nmol of β-glucosidase enzyme (10% of cellulase loading) per Gram cellulose substrate for reaction times of 2, 6, and 24 hrs. The solubilized reducing sugar concentrations in the supernatant after hydrolysis were determined by the DNS assay (A) Glucose equivalent reducing sugar release (mg/mL) as a function of time (2, 6, and 24 h) for the hydrolysis of Cellulose-I by D1–D8 CBM2a Cel5A and WT CBM2a Cel5A. Error bars represent standard deviation from the mean, based on at least four replicates. (B) Based on the data reported in (A), CBM2a Cel5A fusion constructs with negatively supercharged CBMs (D1–D5) were grouped together and average hydrolysis yields were obtained for the group, with the error bars representing standard deviation from the mean. Similarly, CBM2a-Cel5A fusion constructs with positively supercharged CBMs (D6–D8) were grouped together and average hydrolysis yields were obtained. Trend curves have been added to represent the kinetic profiles of the hydrolysis reaction. Wild-type CBM2a-Cel5A is referred to as WT throughout this figure.
Overall, positively supercharged mutants (D6–D8) consistently ranked below wild type and negatively supercharged mutants (D1–D5) across both substrates. On the other hand, negatively supercharged mutants showed comparable performance to wild type in the case of cellulose-I and outperformed the wild type in the case of Cellulose-III. Combining these results along with trends observed towards pretreated biomass substrates in the previous section, it is evident that the reduced activity of negatively supercharged mutants towards AFEX and EA corn stover may be resulting from one of the other factors such as xylanolytic activity, interactions with lignin or thermal stability. Similar results were obtained in previous works where a decrease in hydrolytic activity towards PASC was observed for negatively supercharged mutants (Whitehead et al., 2017). However, the lower activity of positively supercharged mutants towards cellulose may be one of the causal factors behind their overall lowered activity towards pretreated biomass.
Hydrolytic activities of supercharged CBM2a-Cel5A constructs towards xylan and pNPC
Certain cellulases like Cel5A are multifunctional and exhibit activity on xylan, thus the mutants were screened for their activity towards beechwood xylan and the raw data is reported in Figure 4A These results show that the negatively supercharged mutants show reduced activity compared to the wild-type, with the difference becoming more prominent at longer hydrolysis durations such as 24 h. Upon averaging the activities of all supercharged mutants of the same type (negative (D1–D5) vs. positive (D6–D8)) as shown in Figure 4B, it is evident that the negatively supercharged mutants collectively show greater than 1.5-fold reduction in activity. The positively supercharged mutants also show a reduction in activity compared to the wild-type but their activity is very similar to that of negatively supercharged mutants. This is unlike the case of insoluble substrates such as AFEX corn stover or cellulose-I where the positively supercharged mutants showed demonstrably reduced activity compared to the wildtype and negatively charged subgroup. Surprisingly, the mutant D8 showed improved activity compared to the wild-type, despite showing drastically activity amongst the cohort, towards AFEX corn stover and cellulose-I. This trend could likely be due to the reduced significance of CBM function for soluble substrates such as Xylan. Summarizing the results of activity towards biomass, cellulose and xylan, it can be inferred that the reduced activity of negatively supercharged mutants (D1–D5) towards biomass arises predominantly from reduced activity towards Xylan. Positively supercharged mutants D7 and D8 show consistently reduced activity towards all substrates tested although they show activity similar to that of the.
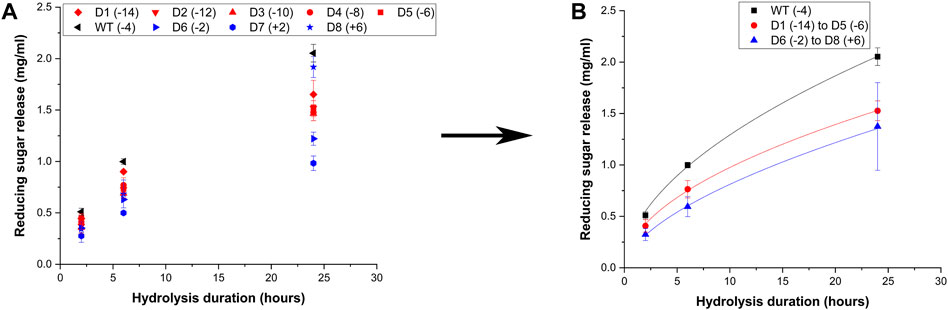
Figure 4. Hydrolytic activity of supercharged cellulases towards Beechwood xylan. 20 μL of 100 g/L Beechwood xylan substrate was hydrolyzed using an enzyme loading of 120 nmol CBM2a Cel5A fusion enzyme per Gram xylan substrate supplemented with 12 nmol of β-xylosidase enzyme (10% of cellulase loading) per Gram xylan substrate for reaction times of 2, 6, and 24 hrs. The solubilized reducing sugar concentrations in the supernatant after hydrolysis were determined by the DNS assay (A) Reducing sugar release (mg/mL) as a function of time (2 h, 6 h and 24 h) for the hydrolysis of Beechwood xylan by D1–D8 CBM2a Cel5A and WT CBM2a Cel5A. Error bars represent standard deviation from the mean, based on at least four replicates. (B) Based on the data reported in (A), CBM2a Cel5A fusion constructs with negatively supercharged CBMs (D1–D5) were grouped together and average hydrolysis yields were obtained for the group, with the error bars representing standard deviation from the mean. Similarly, CBM2a-Cel5A fusion constructs with positively supercharged CBMs (D6–D8) were grouped together and average hydrolysis yields were obtained. Trend curves have been added to represent the kinetic profiles of the hydrolysis reaction. Wild-type CBM2a-Cel5A is referred to as WT throughout this figure.
To validate these trends towards another model soluble substrate, we tested hydrolytic activity towards pNPC (see Supplementary Figure S8). This assay was originally designed to test activity at pH 7.5 (as reported by Whitehead et al. (2017)); however, we adapted the assay to pH 5.5 to keep the pH consistent across all substrates tested in this study. The raw data reported in Supplementary Figure S8A was analyzed further to obtain averages for each individual group (D1–D5 and D6–D8), which is reported in Supplementary Figure S8B. At pH 5.5, all mutants show a negligible reduction in activity towards pNPC (Supplementary Figure S8B) whereas at pH 7.5, negatively supercharged mutants on average showed improved activity compared to the wild-type (Supplementary Figure S8D). Mutant D8 was amongst the top performers in the pNPC assay at pH 7.5, performing distinctly better than the other positively supercharged enzymes. Overall, the activity towards pNPC shows that in the case of positively supercharged mutants (D7 and D8 specifically), the structural integrity of cellulase enzyme may not have been affected in an adverse manner and that the reduced activities observed towards pretreated corn stover or cellulosic substrates may be a result of reduced binding interactions of CBMs to cellulose/lignin or an impact to thermal stability caused due to supercharging.
Binding of mutants to cellulose and lignin
To fully understand the role of CBM binding interactions on the activities of supercharged mutants towards cellulose and thereby pretreated biomass, we performed QCM-D assays (see Supplementary Figure S9 for raw data in the form of sensorgrams) which capture the total number of binding sites and the desorption rate constant (
Lignin is a key polymer in pretreated biomass, which has the potential to restrict access to cellulose binding sites and thereby reduce overall biomass hydrolysis yields. QCM-D assays were performed to understand the binding of supercharged CBM2a mutants to lignin (see Supplementary Figure S10 for raw data). As reported in Supplementary Table S7, all mutants show an improvement in the percentage of protein recovered, indicating that supercharging may have resulted in increased reversibility of interactions between lignin and the CBM. Interestingly, D6 shows the highest percentage of protein recovered amongst all mutants, in stark contrast to D7 and D8, which could partially explain the reason for D6 outperforming its positively supercharged peers (D7 and D8) towards pretreated biomass and cellulose. Lignin inhibition assays were then performed to understand the inhibitory potential of lignin towards hydrolysis of cellulose-I and cellulose-III (see Supplementary Figure S11 for hydrolysis results and Supplementary Table S8 for T-tests comparing mutant activities in lignin inhibition assays). Results from lignin inhibition assays were not too instructive due to the high level of error observed in this assay although the overall trends of negatively supercharged mutants outperforming positively supercharged mutants (with the exception of D6) still remained the same.
Overall, the binding assays to cellulose and lignin shed some light on the behavior of D6 as an outliar from the rest of the positively supercharged sub-group, due to increased binding to cellulose-I and reduced irreversible binding to lignin. In addition, D8 shows a dramatic reduction (∼9-fold) in desorption rate constant towards cellulose-I, indicating that the mutant may struggle with accessing binding sites on cellulose during hydrolysis. However, these results do not explain the reduced activity of D7 toward cellulose-I and AFEX corn stover. It is to be noted that these binding assays are performed at 25 °C as opposed to the hydrolysis assays which are performed at 60 °C. Hence, to understand the potential role of thermal stress on enzyme activity, we subjected these constructs to thermal exposure at elevated temperatures (70 °C), followed by testing of hydrolytic activities at 60 °C.
Hydrolysis of pretreated biomass/cellulose by CBM2a-Cel5A mutants upon thermal treatment
Exposure of the enzymes to 70°C prior to hydrolysis of AFEX corn stover or cellulose-I revealed differences in thermal stability of the cellulase variants (see Figure 5 for AFEX Corn Stover and Cellulose-I and Supplementary Figure S12 for EA Corn Stover and Cellulose-III). To quantify this trend, we calculated the percent reduction in activity caused due to thermal treatment which is reported in Supplementary Table S9. While all enzymes showed decreased reducing sugar release, D3, D6 and D7 showed the most reduction in activity (∼80–99%) towards all substrates tested. On the other hand, D1 and D2 showed better activity upon thermal treatment compared to the wildtype and show the least percent reduction in activity compared to all other mutants.
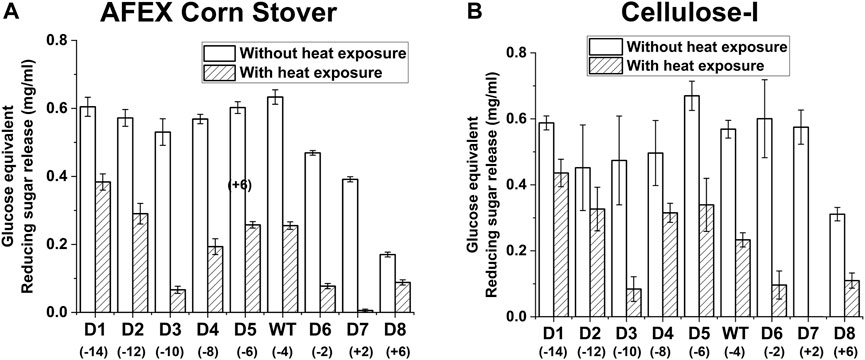
Figure 5. Impact of enzyme thermal denaturation at 70°C on reducing sugar yields from AFEX corn stover (A) and Avicel cellulose-I (B) Cellulase enzyme (0.0048 nmol/μL concentration) was thermally denatured at 70°C for 30 min using an Eppendorf thermocycler. 50 μL of denatured cellulase enzyme was added to either 80 µL of 25 g/L AFEX Corn Stover (A) or 40 µL of Cellulose-I (B) to establish an effective enzyme loading of 120 nmol enzyme per Gram AFEX Corn Stover or Cellulose-I substrate. 12 nmol of β-glucosidase enzyme (10% of cellulase loading) per Gram substrate was added to the reaction mixture and incubated at 60°C for 24 h. A control reaction was performed with enzyme that was incubated on an ice bath (0°C) for 30 min. The results of enzyme activity upon thermal denaturation at 70°C are labelled as ‘With heat exposure’ and those without thermal denaturation are labelled as ‘Without heat exposure’. At least three replicates were run for each condition and the error bars represent standard deviation from the mean. Reducing sugar yields from hydrolysis of AFEX Corn Stover are reported in (A) and those from Avicel cellulose-I are reported in (B).
To build on this trend further, D1, D2 and WT were analyzed further by exposing them to a wider range of temperatures (70°C, 73°C, 76°C and 79 °C) as shown in Figure 6. D1 and D2 outshine the wild type especially at higher temperatures such as 76°C and 79°C towards AFEX corn stover (see Figure 6A) where the wildtype shows practically no activity. For instance, after thermal treatment at 73°C, D1 and D2 showed ∼ 3-fold and ∼ 4-fold improvement in activity compared to WT respectively. This difference is even starker at 76°C although the wild-type activity is closer to the detection limit thereby making activity improvements harder to quantify. However, on cellulose-I (see Figure 6B), D1 and D2 showed a steep decrease in activity when the thermal exposure temperature was reduced from 70°C to 73°C, which continues to decline at higher temperatures. This may likely be caused by the fact that the reducing sugar release from biomass may be arising from xylan at higher temperatures, which is easily accessible compared to cellulose which requires CBM binding for the catalytic domain to be engaged.
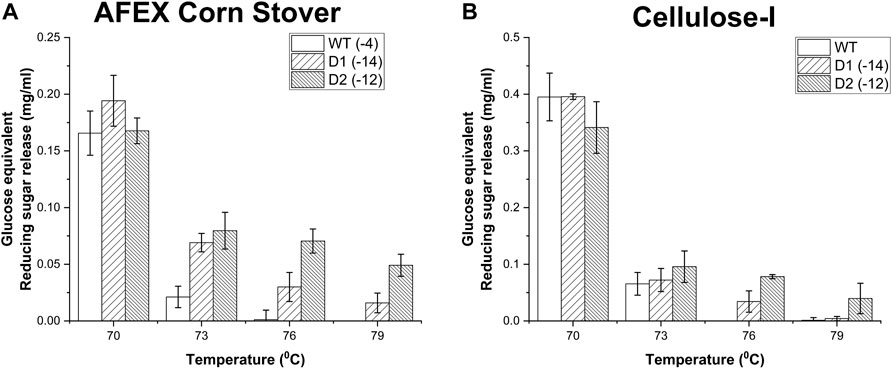
Figure 6. Impact of thermal denaturation at varying temperatures (70°C, 73°C, 76°C and 79°C) on reducing sugar yields from AFEX Corn Stover (A) and Avicel cellulose-I (B). Cellulase enzyme (0.0048 nmol/μL concentration) was thermally denatured for 30 min at one of the following temperatures (70°C, 73°C, 76°C, 79°C) using an Eppendorf thermocycler. 50 μL of denatured cellulase enzyme was added to either 80 µL of 25 g/L AFEX Corn Stover (A) or 40 µL of Cellulose-I (B) to establish an effective enzyme loading of 120 nmol enzyme per Gram AFEX Corn Stover or Cellulose-I substrate. 12 nmol of β-glucosidase enzyme (10% of cellulase loading) per Gram substrate was added to the reaction mixture and incubated at 60°C for 24 h. A control reaction was performed with enzyme that was incubated on an ice bath (0°C) for 30 min. At least three replicates were run for each condition and the error bars represent standard deviation from the mean. Reducing sugar yields from hydrolysis of AFEX Corn Stover are reported in (A) and those from Avicel cellulose-I are reported in (B) under each of the denaturation temperatures (70°C, 73°C, 76°C and 79°C).
The thermal stability was also measured using a thermal shift assay with SYPRO reagent, and the melting temperatures are reported in Supplementary Table S10 (the raw thermal shift assay data is reported in Supplementary Figure S13). There was not an appreciable difference seen in the melting temperatures. This assay measures the melting temperature of the enzyme as a whole and hence these results may be more biased towards the melting temperature of the catalytic domain as opposed to that of the CBM.
Overall, functional hydrolysis assays after thermal treatment of enzymes indicated that supercharging strategy gave rise to thermally stable mutants (D1 and D2) that find direct applications in industry in high-temperature biomass conversion processes. The structural basis of what renders certain supercharged mutants superior to others, still needs to be understood at greater detail which will be the subject of future studies.
Conclusion
Carbohydrate-binding modules (CBMs) play a crucial role in targeting appended glycoside hydrolase enzymes to plant cell wall polymers such as cellulose and hemicellulose (McLean et al., 2002; Herve et al., 2010; Fox et al., 2013; Reyes-Ortiz et al., 2013). However, recent studies have shown that CBMs can also play a role in non-productive binding of appended cellulase catalytic domains to cellulose surface (Karuna and Jeoh, 2017; Nill and Jeoh, 2020; Nemmaru et al., 2021). In addition, CBMs can bind non-productively to lignin via hydrophobic interactions, leading to deactivation of the enzymes on biomass surface (Haarmeyer et al., 2017). To address these bottlenecks, a previous study from our lab has used selective supercharging of cellulase enzymes to reduce lignin inhibition although the mechanistic details were yet to be elucidated (Whitehead et al., 2017). In this study, we expanded the supercharging approach to address mechanistic questions surrounding the impact of CBM supercharging on the hydrolysis of real-world pretreated biomass substrates.
This study is the first comprehensive study to test the impact of enzyme supercharging on activity towards various pretreated biomass substrates and systematically deconvolute the interactions of supercharged enzymes with cellulose, xylan and lignin. Although negatively and positively supercharged enzymes showed reduced activity compared to the wild-type, it was identified that some of these mutants show up to 4-fold improved activity upon exposure to higher temperatures. The reduced activity for negatively supercharged mutants was found to be predominantly driven by reduced activity towards xylan, whereas positively supercharged mutants showed reduced activity towards both cellulose and xylan. Future studies should focus on understanding the structural basis of hydrolytic activity and binding of supercharged mutants to lignocellulosic substrates. Recent work has shown that supercharging CBMs may improve catalytic activity on cellulosic biomass due to improved binding, but this outcome may be dependent on supercharging design strategy and the choice of enzymes (DeChellis et al., 2024). Moreover, the role of solution pH and salt concentration also need to be studied in greater detail due to their outsized impact on the net charge of the protein and alteration of electrostatic potential of supercharged proteins.
Data availability statement
The original contributions presented in the study are included in the article/Supplementary Material, further inquiries can be directed to the corresponding author.
Author contributions
BN: Conceptualization, Formal Analysis, Investigation, Methodology, Validation, Visualization, Writing–original draft, Writing–review and editing. JD: Investigation, Validation, Visualization, Writing–review and editing. JY: Investigation, Methodology, Resources, Writing–review and editing. AD: Investigation, Validation, Writing–review and editing. SS: Investigation, Validation, Writing–review and editing. AT: Investigation, Validation, Writing–review and editing. AW: Investigation, Validation, Writing–review and editing. SC: Conceptualization, Funding acquisition, Project administration, Supervision, Writing–review and editing.
Funding
The author(s) declare that financial support was received for the research, authorship, and/or publication of this article. The authors acknowledge support from the NSF CBET (Award 1604421 and Career Award 1846797), ORAU Ralph E. Powe Award, Rutgers Global Grant, Rutgers Division of Continuing Studies, Rutgers School of Engineering, and the Great Lakes Bioenergy Research Center (DOE BER Office of Science DE-FC02-07ER64494).
Acknowledgments
The authors thank Nathan Kruer-Zerhusen (from late Prof. David Wilson’s lab) for kindly providing the Thermobifida fusca strain and original Cel5A plasmid DNA. Special thanks to Sarvada Chipkar and Dr. Rebecca Ong at Michigan Technological University, for conducting ammonia pretreatment to provide us with cellulose-III and AFEX corn stover. SPSC would also like to thank Dr. Leonardo Da Sousa (Dale lab at Michigan State University) for conducting ammonia pretreatment to generate cellulose III and EA corn stover that was used here in this study as well. BN would like to thank Patrick Doran (Boston University) for his help initially with setting up protocols for computational protein design using Rosetta. JY acknowledges support from the Department of Energy, Office of Energy Efficiency and Renewable Energy (EERE) under agreement no. 28598.
Conflict of interest
The authors declare that the research was conducted in the absence of any commercial or financial relationships that could be construed as a potential conflict of interest.
The author(s) declared that they were an editorial board member of Frontiers, at the time of submission. This had no impact on the peer review process and the final decision.
Publisher’s note
All claims expressed in this article are solely those of the authors and do not necessarily represent those of their affiliated organizations, or those of the publisher, the editors and the reviewers. Any product that may be evaluated in this article, or claim that may be made by its manufacturer, is not guaranteed or endorsed by the publisher.
Supplementary material
The Supplementary Material for this article can be found online at: https://www.frontiersin.org/articles/10.3389/fenrg.2024.1372916/full#supplementary-material
References
Alford, R. F., Leaver-Fay, A., Jeliazkov, J. R., O’Meara, M. J., DiMaio, F. P., Park, H., et al. (2017). The Rosetta all-atom energy function for macromolecular modeling and design. J. Chem. Theory Comput. 13, 3031–3048. doi:10.1021/acs.jctc.7b00125
Azuma, Y., Zschoche, R., Tinzl, M., and Hilvert, D. (2016). Quantitative packaging of active enzymes into a protein cage. Angew. Chem.–Int. Ed. 55, 1531–1534. doi:10.1002/anie.201508414
Beck, T., Tetter, S., Künzle, M., and Hilvert, D. (2015). Construction of matryoshka-type structures from supercharged protein nanocages. Angew. Chem. 127, 951–954. doi:10.1002/ange.201408677
Blommel, P. G., Martin, P. A., Seder, K. D., Wrobel, R. L., and Fox, B. G. (2009). “Flexi vector cloning,” in High throughput protein expression and purification: methods and protocols. Editor S. A. Doyle (Humana Press), 55–73. doi:10.1007/978-1-59745-196-3_4
Bozell, J. J., Black, S. K., Myers, M., Cahill, D., Miller, W. P., and Park, S. (2011). Solvent fractionation of renewable woody feedstocks: organosolv generation of biorefinery process streams for the production of biobased chemicals. Biomass Bioenergy 35, 4197–4208. doi:10.1016/j.biombioe.2011.07.006
Brunecky, R., Subramanian, V., Yarbrough, J. M., Donohoe, B. S., Vinzant, T. B., Vanderwall, T. A., et al. (2020). Synthetic fungal multifunctional cellulases for enhanced biomass conversion. Green Chem. 22, 478–489. doi:10.1039/c9gc03062j
Burley, S. K., Bhikadiya, C., Bi, C., Bittrich, S., Chen, L., Crichlow, G. V., et al. (2021). RCSB Protein Data Bank: powerful new tools for exploring 3D structures of biological macromolecules for basic and applied research and education in fundamental biology, biomedicine, biotechnology, bioengineering and energy sciences. Nucleic Acids Res. 49, D437–D451. doi:10.1093/nar/gkaa1038
Chen, X., Kuhn, E., Jennings, E. W., Nelson, R., Tao, L., Zhang, M., et al. (2016). DMR (deacetylation and mechanical refining) processing of corn stover achieves high monomeric sugar concentrations (230 g L−1) during enzymatic hydrolysis and high ethanol concentrations (>10% v/v) during fermentation without hydrolysate purification or concentration. Energy Environ. Sci. 9, 1237–1245. doi:10.1039/c5ee03718b
Chundawat, S. P. S., Beckham, G. T., Himmel, M. E., and Dale, B. E. (2011a). Deconstruction of lignocellulosic biomass to fuels and chemicals. Annu. Rev. Chem. Biomol. Eng. 2, 121–145. doi:10.1146/annurev-chembioeng-061010-114205
Chundawat, S. P. S., Bellesia, G., Uppugundla, N., da Costa Sousa, L., Gao, D., Cheh, A. M., et al. (2011c). Restructuring the crystalline cellulose hydrogen bond network enhances its depolymerization rate. J. Am. Chem. Soc. 133, 11163–11174. doi:10.1021/ja2011115
Chundawat, S. P. S., Donohoe, B. S., da Costa Sousa, L., Elder, T., Agarwal, U. P., Lu, F., et al. (2011b). Multi-scale visualization and characterization of lignocellulosic plant cell wall deconstruction during thermochemical pretreatment † ‡. Energy & Environ. Sci. 4, 973. doi:10.1039/c0ee00574f
Chundawat, S. P. S., Sousa, L. d. C., Roy, S., Yang, Z., Gupta, S., Pal, R., et al. (2020). Ammonia-salt solvent promotes cellulosic biomass deconstruction under ambient pretreatment conditions to enable rapid soluble sugar production at ultra-low enzyme loadings. Green Chem. 22, 204–218. doi:10.1039/c9gc03524a
Da Costa Sousa, L., Jin, M., Chundawat, S. P. S., Bokade, V., Tang, X., Azarpira, A., et al. (2016). Next-generation ammonia pretreatment enhances cellulosic biofuel production. Energy Environ. Sci. 9, 1215–1223. doi:10.1039/c5ee03051j
Das, R., and Baker, D. (2008). Macromolecular modeling with Rosetta. Annu. Rev. Biochem. 77, 363–382. doi:10.1146/annurev.biochem.77.062906.171838
DeChellis, A., Nemmaru, B., Sammond, D., Douglass, J., Patil, N., Reste, O., et al. (2024). Supercharging carbohydrate binding module alone enhances endocellulase thermostability, binding, and activity on cellulosic biomass. ACS Sustain. Chem. Eng. 12, 3500–3516. doi:10.1021/acssuschemeng.3c06266
Der, B. S., Kluwe, C., Miklos, A. E., Jacak, R., Lyskov, S., Gray, J. J., et al. (2013). Alternative computational protocols for supercharging protein surfaces for reversible unfolding and retention of stability. PLoS One 8, e64363. doi:10.1371/journal.pone.0064363
Fox, J. M., Jess, P., Jambusaria, R. B., Moo, G. M., Liphardt, J., Clark, D. S., et al. (2013). A single-molecule analysis reveals morphological targets for cellulase synergy. Nat. Chem. Biol. 9, 356–361. doi:10.1038/nchembio.1227
Galbe, M., and Wallberg, O. (2019). Pretreatment for biorefineries: a review of common methods for efficient utilisation of lignocellulosic materials. Biotechnol. Biofuels 12, 294. doi:10.1186/s13068-019-1634-1
Gao, D., Haarmeyer, C., Balan, V., Whitehead, T. A., Dale, B. E., and Chundawat, S. P. (2014). Lignin triggers irreversible cellulase loss during pretreated lignocellulosic biomass saccharification. Biotechnol. Biofuels 7, 175. doi:10.1186/s13068-014-0175-x
Georgelis, N., Yennawar, N. H., and Cosgrove, D. J. (2012). Structural basis for entropy-driven cellulose binding by a type-A cellulose-binding module (CBM) and bacterial expansin. Proc. Natl. Acad. Sci. U. S. A. 109, 14830–14835. doi:10.1073/pnas.1213200109
Guo, F., Shi, W., Sun, W., Li, X., Wang, F., Zhao, J., et al. (2014). Differences in the adsorption of enzymes onto lignins from diverse types of lignocellulosic biomass and the underlying mechanism. Biotechnol. Biofuels 7, 38–10. doi:10.1186/1754-6834-7-38
Haarmeyer, C. N., Smith, M. D., Chundawat, S. P. S., Sammond, D., and Whitehead, T. A. (2017). Insights into cellulase-lignin non-specific binding revealed by computational redesign of the surface of green fluorescent protein. Biotechnol. Bioeng. 114, 740–750. doi:10.1002/bit.26201
Herve, C., Rogowski, A., Blake, A. W., Marcus, S. E., Gilbert, H. J., and Knox, J. P. (2010). Carbohydrate-binding modules promote the enzymatic deconstruction of intact plant cell walls by targeting and proximity effects. Proc. Natl. Acad. Sci. 107, 15293–15298. doi:10.1073/pnas.1005732107
Holwerda, E. K., Worthen, R. S., Kothari, N., Lasky, R. C., Davison, B. H., Fu, C., et al. (2019). Multiple levers for overcoming the recalcitrance of lignocellulosic biomass. Biotechnol. Biofuels 12, 15–12. doi:10.1186/s13068-019-1353-7
Humbird, D., et al. (2011). “Process design and economics for biochemical conversion of lignocellulosic biomass to ethanol: dilute-acid pretreatment and enzymatic hydrolysis of corn stover,” in NREL/TP-5100-47764 (Golden, CO, USA: National Renewable Energy Laboratory).
Jung, H., Wilson, D. B., and Walker, L. P. (2003). Binding and reversibility of Thermobifida fusca Cel5A, Cel6B, and Cel48A and their respective catalytic domains to bacterial microcrystalline cellulose. Biotechnol. Bioeng. 84, 151–159. doi:10.1002/bit.10743
Karuna, N., and Jeoh, T. (2017). The productive cellulase binding capacity of cellulosic substrates. Biotechnol. Bioeng. 114, 533–542. doi:10.1002/bit.26193
Khatib, F., Cooper, S., Tyka, M. D., Xu, K., Makedon, I., Popović, Z., et al. (2011). Algorithm discovery by protein folding game players. Proc. Natl. Acad. Sci. U. S. A. 108, 18949–18953. doi:10.1073/pnas.1115898108
Kleffner, R., Flatten, J., Leaver-Fay, A., Baker, D., Siegel, J. B., Khatib, F., et al. (2017). Foldit Standalone: a video game-derived protein structure manipulation interface using Rosetta. Bioinformatics 33, 2765–2767. doi:10.1093/bioinformatics/btx283
Klein-Marcuschamer, D., Oleskowicz-Popiel, P., Simmons, B. A., and Blanch, H. W. (2012). The challenge of enzyme cost in the production of lignocellulosic biofuels. Biotechnol. Bioeng. 109, 1083–1087. doi:10.1002/bit.24370
Kokossis, A. C., Tsakalova, M., and Pyrgakis, K. (2014). Design of integrated biorefineries. Comput. Chem. Eng. 81, 40–56. doi:10.1016/j.compchemeng.2015.05.021
Kostylev, M., and Wilson, D. (2013). Two-parameter kinetic model based on a time-dependent activity coefficient accurately describes enzymatic cellulose digestion. Biochemistry 52, 5656–5664. doi:10.1021/bi400358v
Lawrence, M. S., Phillips, K. J., and Liu, D. R. (2007). Supercharging proteins can impart unusual resilience. J. Am. Chem. Soc. 129, 10110–10112. doi:10.1021/ja071641y
Lei, C., Huang, Y., Nie, Z., Hu, J., Li, L., Lu, G., et al. (2014). A supercharged fluorescent protein as a versatile probe for homogeneous DNA detection and methylation analysis. Angew. Chem.–Int. Ed. 53, 8358–8362. doi:10.1002/anie.201403615
Lim, S., Chundawat, S. P. S., and Fox, B. G. (2014). Expression, purification and characterization of a functional carbohydrate-binding module from streptomyces sp. SirexAA-E. Protein Expr. Purif. 98, 1–9. doi:10.1016/j.pep.2014.02.013
Liu, Y., Nemmaru, B., and Chundawat, S. P. S. (2020). Thermobifida fusca cellulases exhibit increased endo − exo synergistic activity, but lower exocellulase activity, on cellulose-III. ACS Sustain. Chem. Eng. 8, 5028–5039. doi:10.1021/acssuschemeng.9b06792
Luo, X., Liu, J., Zheng, P., Li, M., Zhou, Y., Huang, L., et al. (2019). Promoting enzymatic hydrolysis of lignocellulosic biomass by inexpensive soy protein. Biotechnol. Biofuels 12, 51–13. doi:10.1186/s13068-019-1387-x
Maity, S. K. (2015). Opportunities, recent trends and challenges of integrated biorefinery: Part i. Renew. Sustain. Energy Rev. 43, 1427–1445. doi:10.1016/j.rser.2014.11.092
McCann, M. C., and Carpita, N. C. (2015). Biomass recalcitrance: a multi-scale, multi-factor, and conversion-specific property: fig. 1. J. Exp. Bot. 66, 4109–4118. doi:10.1093/jxb/erv267
McLean, B. W., Boraston, A. B., Brouwer, D., Sanaie, N., Fyfe, C. A., Warren, R. A. J., et al. (2002). Carbohydrate-binding modules recognize fine substructures of cellulose. J. Biol. Chem. 277, 50245–50254. doi:10.1074/jbc.m204433200
Narron, R. H., Kim, H., Chang, H. M., Jameel, H., and Park, S. (2016). Biomass pretreatments capable of enabling lignin valorization in a biorefinery process. Curr. Opin. Biotechnol. 38, 39–46. doi:10.1016/j.copbio.2015.12.018
Nemmaru, B., Ramirez, N., Farino, C. J., Yarbrough, J. M., Kravchenko, N., and Chundawat, S. P. S. (2021). Reduced type-A carbohydrate-binding module interactions to cellulose I leads to improved endocellulase activity. Biotechnol. Bioeng. 118, 1141–1151. doi:10.1002/bit.27637
Nill, J., and Jeoh, T. (2020). The role of evolving interfacial substrate properties on heterogeneous cellulose hydrolysis kinetics. ACS Sustain. Chem. Eng. 8, 6722–6733. doi:10.1021/acssuschemeng.0c00779
Nimlos, M. R., Beckham, G. T., Matthews, J. F., Bu, L., Himmel, M. E., and Crowley, M. F. (2012). Binding preferences, surface attachment, diffusivity, and orientation of a family 1 carbohydrate-binding module on cellulose. J. Biol. Chem. 287, 20603–20612. doi:10.1074/jbc.M112.358184
Nordwald, E. M., Brunecky, R., Himmel, M. E., Beckham, G. T., and Kaar, J. L. (2014). Charge engineering of cellulases improves ionic liquid tolerance and reduces lignin inhibition. Biotechnol. Bioeng. 111, 1541–1549. doi:10.1002/bit.25216
Obermeyer, A. C., Mills, C. E., Dong, X. H., Flores, R. J., and Olsen, B. D. (2016). Complex coacervation of supercharged proteins with polyelectrolytes. Soft Matter 12, 3570–3581. doi:10.1039/c6sm00002a
Ragauskas, A. J., Beckham, G. T., Biddy, M. J., Chandra, R., Chen, F., Davis, M. F., et al. (2014). Lignin valorization: improving lignin processing in the biorefinery. Science 344, 1246843. doi:10.1126/science.1246843
Reyes-Ortiz, V., Heins, R. A., Cheng, G., Kim, E. Y., Vernon, B. C., Elandt, R. B., et al. (2013). Addition of a carbohydrate-binding module enhances cellulase penetration into cellulose substrates. Biotechnol. Biofuels 6, 93. doi:10.1186/1754-6834-6-93
Salas, C., Rojas, O. J., Lucia, L. a., Hubbe, M. A., and Genzer, J. (2013). On the surface interactions of proteins with lignin. ACS Appl. Mater. Interfaces 5, 199–206. doi:10.1021/am3024788
Sammond, D. W., Yarbrough, J. M., Mansfield, E., Bomble, Y. J., Hobdey, S. E., Decker, S. R., et al. (2014). Predicting enzyme adsorption to lignin films by calculating enzyme surface hydrophobicity. J. Biol. Chem. 289, 20960–20969. doi:10.1074/jbc.m114.573642
Sasaki, E., Böhringer, D., van de Waterbeemd, M., Leibundgut, M., Zschoche, R., Heck, A. J. R., et al. (2017). Structure and assembly of scalable porous protein cages. Nat. Commun. 8, 14663–14710. doi:10.1038/ncomms14663
Scown, C. D., Baral, N. R., Yang, M., Vora, N., and Huntington, T. (2021). Technoeconomic analysis for biofuels and bioproducts. Curr. Opin. Biotechnol. 67, 58–64. doi:10.1016/j.copbio.2021.01.002
Simon, A. J., Zhou, Y., Ramasubramani, V., Glaser, J., Pothukuchy, A., Gollihar, J., et al. (2019). Supercharging enables organized assembly of synthetic biomolecules. Nat. Chem. 11, 204–212. doi:10.1038/s41557-018-0196-3
Song, Y., DiMaio, F., Wang, R. R., Kim, D., Miles, C., Brunette, T., et al. (2013). High-resolution comparative modeling with RosettaCM. Structure 21, 1735–1742. doi:10.1016/j.str.2013.08.005
Sousa, L. da C., Humpula, J., Balan, V., Dale, B. E., and Chundawat, S. P. S. (2019). Impact of ammonia pretreatment conditions on the cellulose III allomorph ultrastructure and its enzymatic digestibility. ACS Sustain. Chem. Eng. 7, 14411–14424. doi:10.1021/acssuschemeng.9b00606
Strobel, K. L., Pfeiffer, K. A., Blanch, H. W., and Clark, D. S. (2015). Structural insights into the affinity of Cel7A carbohydratebinding module for lignin. J. Biol. Chem. 290, 22818–22826. doi:10.1074/jbc.m115.673467
Studer, M. H., DeMartini, J. D., Davis, M. F., Sykes, R. W., Davison, B., Keller, M., et al. (2011). Lignin content in natural populus variants affects sugar release. Proc. Natl. Acad. Sci. U. S. A. 108, 6300–6305. doi:10.1073/pnas.1009252108
Studier, F. W. (2005). Protein production by auto-induction in high-density shaking cultures. Protein Expr. Purif. 41, 207–234. doi:10.1016/j.pep.2005.01.016
Sun, C., Li, Y., Yates, E. A., and Fernig, D. G. (2020). SimpleDSFviewer: a tool to analyze and view differential scanning fluorimetry data for characterizing protein thermal stability and interactions. Protein Sci. 29, 19–27. doi:10.1002/pro.3703
Takkellapati, S., Li, T., and Gonzalez, M. A. (2018). An overview of biorefinery-derived platform chemicals from a cellulose and hemicellulose biorefinery. Clean. Technol. Environ. Policy 20, 1615–1630. doi:10.1007/s10098-018-1568-5
Thompson, D. B., Cronican, J. J., and Liu, D. R. (2012). Engineering and identifying supercharged proteins for macromolecule delivery into mammalian cells. Methods Enzymol. 1067, 293–319. doi:10.1016/b978-0-12-396962-0.00012-4
Tuck, C. O., Perez, E., Horvath, I. T., Sheldon, R. A., and Poliakoff, M. (2012). Valorization of biomass: deriving more value from waste. Science 338, 604. doi:10.1126/science.1218930
Ubando, A. T., Felix, C. B., and Chen, W. H. (2020). Biorefineries in circular bioeconomy: a comprehensive review. Bioresour. Technol. 299, 122585. doi:10.1016/j.biortech.2019.122585
Wang, H., Pu, Y., Ragauskas, A., and Yang, B. (2019). From lignin to valuable products–strategies, challenges, and prospects. Bioresour. Technol. 271, 449–461. doi:10.1016/j.biortech.2018.09.072
Watson, D. L., Wilson, D. B., and Walker, L. P. (2002). Synergism in binary mixtures of Thermobifida fusca cellulases Cel6b, Cel9a, and Cel5a on BMCC and Avicel. Appl. Biochem. Biotechnol.–Part A Enzym. Eng. Biotechnol. 101, 097–112. doi:10.1385/abab:101:2:097
Whitehead, T. A., Bandi, C. K., Berger, M., Park, J., and Chundawat, S. P. S. (2017). Negatively supercharging cellulases render them lignin-resistant. ACS Sustain. Chem. Eng. 5, 6247–6252. doi:10.1021/acssuschemeng.7b01202
Wilson, D. B. (2004). Studies of Thermobifida fusca plant cell wall degrading enzymes. Chem. Rec. 4, 72–82. doi:10.1002/tcr.20002
Yang, B., and Wyman, C. E. (2006). BSA treatment to enhance enzymatic hydrolysis of cellulose in lignin containing substrates. Biotechnol. Bioeng. 94, 611–617. doi:10.1002/bit.20750
Keywords: cellulase, carbohydrate-binding module, computational protein design, thermal stability, enzyme supercharging, lignin inhibition, non-productive binding, cellulosic biomass hydrolysis
Citation: Nemmaru B, Douglass J, Yarbrough JM, DeChellis A, Shankar S, Thokkadam A, Wang A and Chundawat SPS (2024) Supercharged cellulases show superior thermal stability and enhanced activity towards pretreated biomass and cellulose. Front. Energy Res. 12:1372916. doi: 10.3389/fenrg.2024.1372916
Received: 18 January 2024; Accepted: 04 June 2024;
Published: 01 July 2024.
Edited by:
Haiping Yang, Huazhong University of Science and Technology, ChinaReviewed by:
Zhaoxian Xu, Nanjing University of Science and Technology, ChinaCaoxing Huang, Nanjing Forestry University, China
Copyright © 2024 Nemmaru, Douglass, Yarbrough, DeChellis, Shankar, Thokkadam, Wang and Chundawat. This is an open-access article distributed under the terms of the Creative Commons Attribution License (CC BY). The use, distribution or reproduction in other forums is permitted, provided the original author(s) and the copyright owner(s) are credited and that the original publication in this journal is cited, in accordance with accepted academic practice. No use, distribution or reproduction is permitted which does not comply with these terms.
*Correspondence: Shishir P. S. Chundawat, c2hpc2hpci5jaHVuZGF3YXRAcnV0Z2Vycy5lZHU=
†ORCID: Shishir P. S. Chundawat, orcid.org/0000-0003-3677-6735
‡These authors have contributed equally to this work