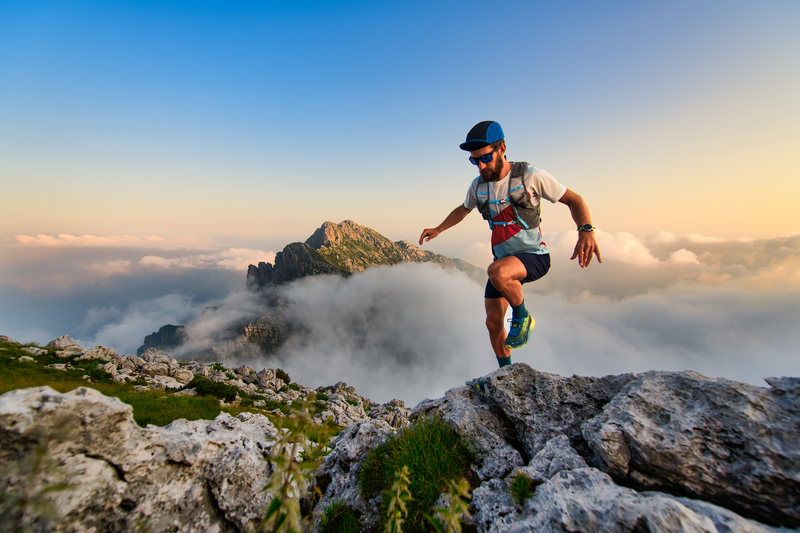
95% of researchers rate our articles as excellent or good
Learn more about the work of our research integrity team to safeguard the quality of each article we publish.
Find out more
ORIGINAL RESEARCH article
Front. Energy Res. , 21 February 2024
Sec. Process and Energy Systems Engineering
Volume 12 - 2024 | https://doi.org/10.3389/fenrg.2024.1364904
This article is part of the Research Topic Design, Simulation, and Optimization of Hybrid Energy Systems View all 5 articles
The surging demand for electricity, fueled by environmental concerns, economic considerations, and the integration of distributed energy resources, underscores the need for innovative approaches to smart home energy management. This research introduces a novel optimization algorithm that leverages electric vehicles (EVs) as integral components, addressing the intricate dynamics of household load management. The study’s significance lies in optimizing energy consumption, reducing costs, and enhancing power grid reliability. Three distinct modes of smart home load management are investigated, ranging from no household load management to load outages, with a focus on the time-of-use (ToU) tariff impact, inclining block rate (IBR) pricing, and the combined effect of ToU and IBR on load management outcomes. The algorithm, a multi-objective approach, minimizes the peak demand and optimizes cost factors, resulting in a 7.9% reduction in integrated payment costs. Notably, EVs play a pivotal role in load planning, showcasing a 16.4% reduction in peak loads and a 7.9% decrease in payment expenses. Numerical results affirm the algorithm’s adaptability, even under load interruptions, preventing excessive increases in paid costs. Incorporating dynamic pricing structures like inclining block rates alongside the time of use reveals a 7.9% reduction in payment costs and a 16.4% decrease in peak loads. In conclusion, this research provides a robust optimization framework for smart home energy management, demonstrating economic benefits, peak load reduction potential, and enhanced reliability through strategic EV integration and dynamic pricing.
The desire to reduce pollution, fight climate change, save fossil fuels, save money, and make the power grid more reliable and stable are all factors that are driving up the consumer demand for electricity (Taner et al., 2019; Jasim et al., 2023; Nejatian et al., 2023). This has led to the adoption of distributed production resources in order to establish a smart and eco-friendly network (Jamil et al., 2022; Almalki et al., 2023). The use of fossil fuels to meet this level of demand without proper energy management will result in the depletion, if not the extinction, of these natural resources (Yin et al., 2022; Nikbakht Naserabad et al., 2023). As a result, today’s humanity is attempting to use renewable resources to help meet this massive and ever-increasing demand (Pachar et al., 2021). Aside from using renewable resources for production, the discussion of how to manage these energy resources can be appealing because there is a significant opportunity to save energy in the building through efficient use (Erixno et al., 2022).
Due to their many advantages over other transportation technologies, electric vehicles (EVs) have recently attracted a lot of interest (Mohammed et al., 2024; Pamidimukkala et al., 2024). This technology has many benefits, including being environmentally friendly, making very little noise, being extremely efficient with energy, reducing operational costs, using local renewable energy sources, and offering additional services through energy exchange between vehicles and the grid (Misbah Inayat et al., 2023; Siddiqui et al., 2024).
The equilibrium between power generation and consumption is a crucial factor for the proper functioning of the power grid (Ourahou et al., 2020; Wei et al., 2024). The disparity between the two results in elevated operational expenses for power corporations or potentially immobilizes the entire power network (He et al., 2023). Power suppliers have installed backup power storage batteries to enhance network stability and reliability. However, typically, this approach in isolation is insufficient. Load response programs are regarded as a tactic to mitigate fluctuations in the demand curve (Ferraro et al., 2020).
By deploying the load response program, the network operator hopes to lower operating expenses by flattening the network load curve. In recent years, it has garnered considerable interest (Lin et al., 2024). The customer is tasked with calculating their own electricity consumption in these programs after being presented with different electricity prices at different times. Pricing should be designed to encourage consumption at the operator’s preferred times. Electricity consumption during peak hours is something that everyone is interested in reducing, including the operator, distribution companies, and producers. The subscriber is thus informed that, according to the load forecast, the price will be higher during peak hours (Kath and Ziel, 2021; Rahman et al., 2024).
A smart building is one in which all of its internal components interact with one another via integrated systems and logical creations that are environmentally friendly (Al Dakheel et al., 2020; Apanaviciene et al., 2020; Eini et al., 2021; Li et al., 2021). It goes without saying that the preceding definition does not imply that this structure has decided to respond to any incident. Being intelligent means that the system can make appropriate decisions based on past events and defined logic. In other words, a smart building will not be able to be creative in the face of various factors but will instead behave logically. Energy management, on the other hand, is defined as the economic and efficient use of energy (Hakimi and Hasankhani, 2020; Aguilar et al., 2021).
A multi-objective algorithm introduced by Shirazi and Jadid (2015) for the optimal scheduling of electricity and heat with optimal management is used to provide a trade-off between minimizing peak demand and the cost of using the smart home. The use of distributed energy sources has been proposed. Nojavan and Zare (2013) investigated the optimal bidding strategy with uncertainty modeling of the day-ahead market price. Nojavan et al. (2015) utilized a combined approach based on particle optimization to maximize production profit in the presence of market price uncertainty. Brahman et al. (2015) investigated the residential energy hub with the goal of minimizing operating costs by taking the customer’s priorities into account. The minimum cost of operations in the GAMS model was obtained by programming a residential microgrid incorporating a smart heat load-micro-CHP-battery meter (Tasdighi et al., 2014). The management of an energy hub with the goal of minimizing energy costs and risks incorporating a wind turbine, heat generator, and CHP system has been investigated (Bozchalui et al., 2012). Yan et al. (2023) investigated an economically optimized home energy management system for smart buildings, incorporating electric vehicles and considering risk factors. They employed an advanced whale optimization algorithm to address practical constraints, revealing substantial cost reductions with positive consumer attitudes toward energy consumption and adaptive strategies based on price risk indicators. Manivannan (2024) explored the impact of EVs on the electrical system and emphasized the importance of the EV’s energy management system (EMS) in smart transportation within smart cities. The study proposed a machine learning-based Smart Energy Management System for Hybrid Electrical Vehicles (SEMS-HEV) to optimize energy usage, enhance battery lifespan, and prevent electrical supply disruptions. Integrating IoT-based smart charging, the research focuses on improving EMS performance and efficiency through dynamic scheduling of vehicle-to-grid (V2G) connections for hybrid electrical vehicles. Alghassab (2024) introduced a fuzzy-based smart energy management system for Saudi residential buildings, optimizing energy use through fuzzy logic. The system adapts devices based on user preferences, occupancy, and real-time energy prices, demonstrating superior performance in a comparative study. This approach aligns with Saudi Arabia’s goal of sustainable energy practices, marking a promising step toward a more efficient and eco-friendly future.
By employing EVs as a sophisticated model and a viable solution to economic concerns, this study also provides a strategy for load planning. In smart homes, the presence of EVs is critical for reducing shared costs and powering household loads with energy discharged during periods of high tariff. The significance of EVs in household load management, however, has been neglected. Consequently, the purpose of this research is to achieve this goal through the implementation of a suitable optimization algorithm. Additionally, the examination of relevant literature studies unveiled that the household load management procedure implemented in the edifice failed to consider the matter of a definite load. The following sections will provide an overview of the subject before proceeding to the mathematical modeling of home load management. This study is grounded in the data regarding a smart home in the urban area of Najran, Kingdom of Saudi Arabia.
In this study, a novel framework for the management of buildings will also be connected to the network. The study also has an EV parking lot and can use electrical car reserves for energy management. In addition, it is assumed that the building has a battery energy store. It will not be a battery charge and discharge, reducing energy losses and the cost of investment because the number of energy converters decreases. By employing precise modeling of the issue grounded in household load management and simulation through the GAMS model, the acquired outcomes are scrutinized and assessed.
There are numerous approaches to EMS in buildings. These techniques fall into the following two categories: information-based predictive methods and real-time-based methods. In this study, real-time-based methods are used. Energy management operates using real-time data, thereby eliminating the necessity for forecasting. In contrast to their predecessors, these methods offer numerous advantages, such as algorithm structure simplification and the elimination of the requirement for intensive calculations. Sechilariu et al. (2013) stated that an approach to energy management for structures housing PV products involves considering two factors: the DC building load and the charging of EVs. Additionally, for the purpose of energy management, they made an effort to prevent network reception during peak hours. Additionally, the load response method was implemented utilizing a variable price prosecution mechanism.
The pricing structure is based on the time of use (ToU) and the inclining block rate (IBR). This depends on the real-time situation and the specific moment during which it has had the capability to accomplish it. In the forthcoming years, however, real-time is anticipated to be mandatory for the majority of end-users, encompassing the intelligent capabilities of the smart network that rely on suitable communication. According to the results presented in Sechilariu et al. (2013), Wi et al. (2013), and Byeon et al. (2013), EMS based on real-time information is more suitable for buildings because it is easier to understand and manage.
In this study, an EMS is presented that assists building energy operators in achieving a logical and automated operation of DC systems by utilizing real-time cost and consumption information. This algorithm is provided for EMS-owned reserves, which include buildings such as the EV parking lot and incur expenses for the EMS to purchase, repair, and other related matters. Battery technology has been implemented for storage. The EV method employed in this study represents a sophisticated approach to energy management within the realm of smart buildings. Central to this methodology is the integration of electric vehicles as pivotal components in the optimization of energy consumption. EVs act as dynamic entities capable of not only drawing power from the grid but also contributing to the grid through strategic discharge during peak demand periods. Unlike conventional methods, our EV method goes beyond mere load balancing and incorporates a multi-objective model that considers not only economic factors but also risk considerations. This entails a robust framework where electric vehicles are not just passive consumers but active participants in the overall energy ecosystem.
The uniqueness of our EV method lies in its ability to adapt to real-world constraints, including production limits, flexible loads, and intricate cost-risk indicators. By leveraging a developed whale optimization algorithm, we ensure a more efficient and adaptive energy management system. This algorithm has been specifically enhanced to avoid converging to local optima, offering a higher likelihood of finding globally optimal solutions. Moreover, our EV method stands out by considering practical and nonlinear constraints, making it more aligned with the complexities of modern energy scenarios.
Before proceeding via this method, the required information is gathered. The load value, distribution network conditions, battery state of charge (SoCBatt), and EV state of charge (SoCEV) are included in these data. The primary distribution network is categorized into three modes of load, namely, low load, medium load, and high load, depending on its load level. Energy costs vary for each load level. If the combined power demand for charging the EV and the amount of load exceed the generated power, this approach utilizes either the energy stored in the battery or purchases power from the distribution network. It is possible that the battery’s stored power is inadequate to offset the fraction. In this instance, the necessary energy is procured concurrently from the distribution network and the battery. At the times that the SoC has been in the order SoCmin < SoC < SoCmax, the power of the work should be used. SoCmin and SoCmax are the minimum and maximum values, which specify the allowed amount of the storage level.
Constant connectivity exists between the building distribution system and the primary distribution network. The EMS is obligated to reduce the power it purchases from the distribution network and, if it possesses power generation sources such as renewable energy sources, sell the excess power to the national distribution network during periods of high electricity prices (typically during peak hours). Therefore, the utilization of an EV is employed in this particular scenario. The EV will be operational to the extent that the EMS will be able to discontinue charging or commence discharging the EV at specific times. If EV charging is halted, the EV will enter the standby mode; otherwise, it will enter the discharge mode.
To encourage EV owners to participate in energy management and utilize EVs as energy storage devices, the EMS operator must provide incentives. The charging and discharging model for EVs presented is predicated on the subsequent principles.
• Engagement in energy management activities is not required. The proprietor of an EV is free to sign a participation contract in energy management and configure SOCmin and SOCdischarging to their liking at any time. However, if they wish to cancel the contract prior to its expiration, they will incur a fine.
• While the EV is parked in the lot and being charged, the EMS operator is obligated to maintain the SOC of the EV battery above the SOCmin value specified in the contract.
• If the SOC of the EV battery reaches the SOCmax value mentioned in the contract, the EMS operator can stop charging the EV.
• To enforce energy management strategies, such as peak load reduction, the EMS operator may suspend the charging process for vehicles with a SOC exceeding the SOCmin. The term for this state is the standby mode.
• It will be feasible to discharge vehicles whose SOC exceeds SOCdischarging in situations involving a substantial load. It is referred to as the discharge mode.
In accordance with the prescribed regulations governing EV involvement in energy management, the charging expense for every EV is computed using Eqs 1, 2, which can be utilized to compute the energy cost of all EVs when multiple EVs are engaged in energy management (Ghosh et al., 2022).
Here, ECtotal_EV represents the total cost of charging all EVs, while ECi,EV represents the energy cost for charging the ith EV. The costs associated with charging and discharging 1 kW of power are denoted by CEV_C and CEV_D, respectively. Additionally, Pi,j,EV_C and Pi,j,EV_D represent the EV’s charge and discharge powers, respectively. If the EV is parked in the lot and is connected to the system, it will adhere to the EMS-provided charging and discharging procedure. The EV owner is responsible for the cost of all power consumed by the EV battery (
The EMS operator must determine the CEV_D value. This task necessitates the consideration of numerous variables, including charging losses, EV battery life, and the amount of money saved by reducing the peak load (EV battery efficiency). If the CEV_D value is accurately determined, field EVs will be able to manage and benefit from energy support.
The price of electricity influences the performance of EVs, and the price of electricity can impact the degree to which EV owners participate in energy management. Given the fluctuating electricity prices between peak and off-peak hours, the conduct of EV owners will likewise vary during these periods. The charging and discharging patterns of EVs, in relation to the load, are presented in Table 1.
The system is divided into three load modes, as shown in Table 1: high load, normal or medium load, and low load. Charge and discharge patterns of EVs vary between the three scenarios. When power levels are high or low, an EV is only charged if its SOC is below SOCmin. If the SOC value for any EV during charging falls within the range of SOCmin and SOCdischarging, the vehicle enters the standby mode, and neither charging nor discharging occurs. Moreover, the discharge of EVs is exclusively permitted during productive periods, provided that their SOC exceeds that of the SOCdischarging.
In Figure 1, the presented algorithm is shown for three modes: low load, medium load, and high load. The SOCSmin and SOCSmax parameters denote the minimum and maximum permissible values for the battery’s storage level, respectively. PUG represents power exchangeable units. PS and PEV represent the power and storage capacity of an EV, respectively; positive values signify a charging state.
FIGURE 1. Model presented for energy management for (A) low-load, (B) medium-load, and (C) high-load hour.
During the low-load mode, the EV’s SOC value is evaluated in order to ascertain the necessary course of action. The Pe value represents the EV’s charging rate. If the SOC value of the EV exceeds SOCEVmin during the medium load stage, the system will enter the standby mode. If not, the EV enters the charging mode; this cycle will continue until its state of charge reaches SOCEVmin. In this scenario, should the network encounter a power deficit, EVs with the highest SOC will enter the discharge mode (SOCEVdischarging). Once the SOC of a vehicle reaches SOCdischarging, it will transition to the standby mode.
To conduct an economic analysis of the proposed energy management algorithm, it is imperative to derive a correlation that facilitates the computation of energy expenses. The determination of energy supply costs can be approached from three perspectives: perspective of the building manager, who may serve as the load collector or energy management operator; perspective of the consumers residing in the building; or from the standpoint of the distribution network. This study operates under the assumption that the building manager is responsible for EMS control and is tasked with reducing the overall cost of energy supply for the structure. Energy costs can be calculated using Eq. 3.
The total cost of energy supply for the building is denoted by ECtotal. Additionally, ECUG represents the expense incurred to procure electricity from the distribution network, while ECtotal_EV represents the cost incurred to charge and discharge all EVs. The cost of purchasing energy from the distribution network is calculable with the aid of Eq. 4.
Here, Ptotal_load is the total load of the building and calculated using Eq. 5.
CUG represents the electricity purchased from the grid that costs one hundred dollars per kilowatt-hour. PD_load represents the building load demand expressed in kilowatt-hours. Pi,EV_C and Pi,EV_D represent the quantity of charged power and discharged power, respectively, in ith EV in kW hours.
The evaluation of the proposed innovation method encompassed diverse smart home configurations, incorporating responsive and non-responsive devices, along with the integration of an EV. Three distinct load management modes were considered, including the initial condition with no household load management, a subsequent condition implementing load management without outages, and a final condition simulating load outages akin to the second condition. The assessment was conducted primarily with ToU tariffs as the baseline, with a detailed exploration of the impact of IBR pricing and the combined influence of ToU and IBR on load management outcomes, which are comprehensively discussed in the conclusion section.
In the context of smart home load management, the proposed approach demonstrated robust efficacy in regulating the household load for a 24-h period, employing a daily timestep count of 96, where each time step represented 15 min. Non-operational appliances, such as personal laptops, televisions, and vacuum cleaners, were considered baseline non-responsive devices. The load management program effectively transformed the washing machine, dishwasher, and coffee maker into responsive devices by regulating their usage time, as illustrated in the daily average data presented in Table 2.
Further considerations included a permissible deviation of 0.30 kW-hours, with subscribers being offered one permitted interval for non-responsive devices. It was assumed that the subscriber would accept one of the three intervals specified in the result announcement. A uniform equivalent lost load charge of 25 cents/kWh was applied to all appliances. Table 3 presents a detailed breakdown of various time-of-use tariffs, providing comprehensive insights into the pricing structures considered during the evaluation.
Assumed within the confines of this scenario are several critical parameters. First and foremost, the heating system is presumed to consistently operate at peak efficiency, ensuring optimal energy utilization. Simultaneously, the EV is envisioned to be charged to its maximum capacity upon connection to the charger, and all responsive devices are activated within their designated permitted operating range. Despite these proactive measures, due to the inherent uncontrollability of the load, the integration of EVs into the household load is presently deemed unfeasible. Figure 2 provides a comprehensive illustration of the building load demand diagram for this residential scenario, encapsulating the intricate dynamics of the load under the assumed conditions. The basis for the ToU pricing model is established at 268.87 cents, reflecting the electricity consumption throughout the day, as depicted by the load demand in Figure 2. Furthermore, the peak load consumption during this period is recorded at 6.80 kW.
On the same day, it is postulated that the house’s electricity consumption occurs within the timeframe spanning the 78th to the 85th time steps. Consequently, electrical service is anticipated to be intentionally disconnected from the residence at incremental intervals corresponding to these time steps. Notably, during these cut-off periods, both the television and personal laptop experience forced shutdowns, enduring a blackout period of 105 min. Additionally, specific measures are implemented to curtail energy consumption during this scenario.
In this particular scenario, the assumption is made that the household load remains uninterrupted, thereby framing load management as an exercise focused on minimizing co-payments throughout the day. Table 4 provides a comprehensive breakdown of performance intervals for responsive appliances, suggested performance intervals for non-responsive appliances, and the corresponding consumption levels of the heating system within each interval. These values are derived from solving the optimization problem, considering both scenarios with and without accounting for the capability of EVs to supply household loads.
An analysis of the outcomes reveals that device functionality adheres to the permitted time intervals during periods of reduced tariffs, showcasing the effectiveness of load management in minimizing costs. Table 5 elucidates the planning involved in EV charging and discharging during different time steps. For instance, during time steps 1–12, if the EV cannot supply the load, it is charged to ensure a fully charged battery before departing the residence. At time step 65, when the EV enters the residence, the battery is 2.7 kWh full, prompting a recharge at the end of each day to attain a pre-established battery charge level of 3.9 kWh.
Considering the EV’s capacity to supply the household load, the battery is strategically discharged during high tariff periods and charged during low tariff periods (as detailed in Table 5), resulting in an overall reduction in payment amounts. A comparative analysis between the two cases, one accounting for the ability of the EV to provide load and the other without, reveals a shared payment cost of 227.26 cents per day in the former and 238.16 cents per day in the latter.
Furthermore, Figure 3 visually depicts the household load dynamics both with and without the capability of energy supply via the EV. Notably, when EVs possess the ability to supply the household load, they are discharged during high tariff hours and charged more during low tariff hours, leading to an increase in the peak load at the end of the night, an effect absent in cases where the load supply capability is lacking. This nuanced exploration of load management strategies provides a comprehensive understanding of the interplay between EV capabilities and household load dynamics.
In this scenario, the household load experiences a planned interruption from time step 77 for a duration of 90 min, spanning 6 time steps, until time step 84. Prior to this interruption, up to time step 76, the household load management results mirrored those observed in the preceding scenario. At time step 77, the schedule undergoes an update to accommodate the planned interruption, and following the cessation of the load at time step 84, the household load is re-evaluated based on the most recent information regarding device operations. Noteworthy in this context is the consideration of a lost energy value for devices at 22 cents/kWh, surpassing the electricity tariff at any given time. Consequently, in the second mode, encompassing time steps 77 to 84, the EV strives to supply maximum electricity to devices that remain operational during this interval.
Given a switching time of 30 s, the cost associated with load interruption is calculated at 1.8 cents. This deviation in outage cost, as opposed to the cost calculated in the initial scenario, exemplifies the impact of the proposed household load management methodology on the reduction of outage-related expenses. Figure 4 visually represents the household load diagram in this third state, offering insights into the load dynamics during and after the interruption.
Based on the outcomes, the expected household peak load in this scenario is determined to be 6.29 kW, which is less than the peak load observed in the initial case. Moreover, the payment fee registers at 246.11 cents, slightly higher than that in the second case. This outcome underscores that, despite the occurrence of load interruptions and deviations from the optimal plan in terms of paid costs observed in the second case, the household load management strategy in the third case effectively prevented an excessive increase in overall payment costs. This nuanced analysis highlights the resilience and effectiveness of the proposed methodology under challenging conditions, contributing to a comprehensive understanding of its practical implications.
In this section, the effects of IBR pricing, as well as the combination of IBR and ToU, which have been considered in previous references, on the results of household load management, are attempted to be studied. Table 6 displays the results of the paid fee and peak load when comparing different tariffs.
TABLE 6. Results of the implementation of building load management considering different electricity tariffs.
The results show that when the IBR is used, the load is distributed throughout the day, and, as can be seen, the peak load is significantly reduced. As a result, the payment fee and peak load will be reduced by 7.9% and 16.4%, respectively, when the ToU tariff is considered.
To summarize the findings, the first scenario is considered in the ToU to be a state without household load management due to the existence of power outages and the occurrence of outages for various devices, as well as the uncontrollability of the load and the impossibility of supplying the household load by the EV. Regardless of the pricing method used, it results in the imposition of high costs on the subscriber. In the second scenario, unlike the first, it is not certain, owing to the result of load management planning by defining performance intervals for all devices, as well as checking the conditions of ability and inability to supply the household load by the EV, which shows that the supply ability is optimal. In this scenario, the load is supplied by an EV.
In comparison to scenario 1, the result is that the co-payment cost with the ToU tariff has been reduced even if the household load cannot be supplied by the EV. Domestic peak load has also declined since the first state. Outage is considered in the third scenario, but unlike the first, there is optimal planning for load management with the presence of the EV, which allows for a reduction in the outage cost when compared to the first. Furthermore, when compared to the first scenario, the domestic peak load and the cost of paying with the ToU tariff are lower in this case. However, despite the presence of load interruption in this case, the payment fee is only marginally higher than in the second scenario, owing to the effect of the proposed household load management. Finally, the goal is to compare different pricing on household load management planning, demonstrating that the combination of IBR and ToU results in a reduction in the payment cost and peak load when compared to the ToU pricing method.
To validate the outcomes of various tariffs, a qualitative comparison is conducted in this section. The multiple users and load priority (MULP) scenario is utilized by Abushnaf et al. (2015) to compare the outcomes of the multi-user algorithm and ToU. In the initial instance of this scenario, a comparison is made between the flat rate and ToU tariffs (without energy consumption control). The alterations in Figure 5A constitute a summer day, while the modifications in Figure 5B represent a winter day. The graphs additionally illustrate the load for both flat rate and ToU tariffs, along with the curves representing the costs incurred under each tariff option (flat rate and ToU).
FIGURE 5. Effect of using ToU transpiration on the consumption load and cost in (A) summer and (B) winter.
As shown in Figure 5, the cost of payment is reduced by 0.83% when the ToU tariff is utilized during the summer, as opposed to the flat rate tariff. Conversely, when compared to the ToU tariff, the flat rate tariff will result in an 8% reduction in payment costs during the winter season. Conversely, energy consumption remains constant throughout the day and night, with no measures implemented to transfer superfluous loads to more cost-effective ToU periods. Nevertheless, during peak hours, the cost of electricity is considerably greater under this tariff compared to the flat rate tariff. This necessitates the promotion of a method for regulating peak-hour electricity consumption.
In this particular situation, the algorithm for home energy management transfers superfluous loads from periods of high demand to periods of lower cost with the intention of diminishing electricity expenses and overall consumption. In order to accomplish this, it is assumed that the demand limit of 1.7 kW during peak hours is constant for both seasons. The home energy management algorithm’s adaptable architecture enables subscribers to exercise diverse discretion in order to mitigate their daily energy usage and subsequently lower their electricity expenses.
The desire to reduce pollution, combat climate change, conserve fossil fuels, save money, and improve power grid reliability has led to the utilization of distributed production resources to build a smart, environmentally friendly network. If the demand for fossil fuels is not met properly, they will deplete or disappear. Thus, humanity relies on renewable resources to meet this massive and growing demand. Beyond production, discussing renewable energy management is appealing because the building can save a significant amount of energy through efficiency. This study underscores the efficacy of the proposed optimization approach for managing household load in smart homes, particularly in integrating EVs into the energy management framework. The numerical results obtained from various scenarios and pricing models provide tangible evidence of the approach’s effectiveness in achieving cost savings, peak load reduction, and improved reliability.
This study addresses the escalating demand for electricity by presenting a methodology that not only optimizes energy consumption but also aligns with environmental sustainability goals. The numerical outcomes reveal a 7.9% reduction in integrated payment costs through the regulation of device usage durations, demonstrating a tangible impact on financial savings. EVs, hailed for their environmental compatibility and energy efficiency, emerge as crucial components in the proposed algorithm. The numerical results emphasize the potential of EVs to contribute to load planning, displaying a 16.4% reduction in peak loads and a 7.9% decrease in payment expenses when incorporating IBR pricing. The dynamic pricing structures, including ToU and IBR, exhibit significant impacts on load management. The numerical analysis illustrates a 7.9% reduction in payment costs and a 16.4% decrease in peak loads when IBR pricing is implemented, underscoring the effectiveness of such pricing models in influencing consumer behavior.
Scenario-based results demonstrate the adaptability and robustness of the proposed algorithm under various conditions, including uninterrupted loads, load interruptions, and load management strategies. The outcomes showcase not only cost reduction but also the prevention of excessive increases in paid costs, reinforcing the practical utility of the algorithm. The findings provide clear insights into the economic benefits, peak load reduction potential, and reliability improvements achievable through the proposed optimization approach. The results further underscore the importance of considering dynamic pricing structures and strategically integrating electric vehicles for a holistic and effective smart home energy management system.
The original contributions presented in the study are included in the article/Supplementary material; further inquiries can be directed to the corresponding authors.
YD: conceptualization, data curation, methodology, software, writing–original draft, and writing–review and editing. AI: conceptualization, methodology, software, validation, writing–original draft, and writing–review and editing. MA: conceptualization, data curation, methodology, software, validation, writing–original draft, and writing–review and editing. ZB: data curation, investigation, software, writing–original draft, and writing–review and editing. AM: data curation, formal analysis, methodology, software, visualization, writing–original draft, and writing–review and editing. AN: data curation, formal analysis, resources, writing–original draft, and writing–review and editing.
The author(s) declare financial support was received for the research, authorship, and/or publication of this article. The authors are thankful to the Deanship of Scientific Research at Najran University for funding this work under the Research Priorities and Najran Research Funding Program, grant code (NU/NRP/SERC/12/9).
The authors declare that the research was conducted in the absence of any commercial or financial relationships that could be construed as a potential conflict of interest.
All claims expressed in this article are solely those of the authors and do not necessarily represent those of their affiliated organizations, or those of the publisher, the editors, and the reviewers. Any product that may be evaluated in this article, or claim that may be made by its manufacturer, is not guaranteed or endorsed by the publisher.
Abushnaf, J., Rassau, A., and Górnisiewicz, W. (2015). Impact of dynamic energy pricing schemes on a novel multi-user home energy management system. Electr. Power Syst. Res. 125, 124–132. doi:10.1016/j.epsr.2015.04.003
Aguilar, J., Garces-Jimenez, A., R-Moreno, M. D., and García, R. (2021). A systematic literature review on the use of artificial intelligence in energy self-management in smart buildings. Renew. Sustain. Energy Rev. 151, 111530. doi:10.1016/j.rser.2021.111530
Al Dakheel, J., Del Pero, C., Aste, N., and Leonforte, F. (2020). Smart buildings features and key performance indicators: a review. Sustain. Cities Soc. 61, 102328. doi:10.1016/j.scs.2020.102328
Alghassab, M. A. (2024). Fuzzy-based smart energy management system for residential buildings in Saudi Arabia: a comparative study. Energy Rep. 11, 1212–1224. doi:10.1016/j.egyr.2023.12.039
Almalki, F. A., Alsamhi, S. H., Sahal, R., Hassan, J., Hawbani, A., Rajput, N. S., et al. (2023). Green IoT for eco-friendly and sustainable smart cities: future directions and opportunities. Mob. Netw. Appl. 28, 178–202. doi:10.1007/s11036-021-01790-w
Apanaviciene, R., Urbonas, R., and Fokaides, P. A. (2020). Smart building integration into a smart city: comparative study of real estate development. Sustainability 12, 9376. doi:10.3390/su12229376
Bozchalui, M. C., Hashmi, S. A., Hassen, H., Canizares, C. A., and Bhattacharya, K. (2012). Optimal operation of residential energy hubs in smart grids. IEEE Trans. Smart Grid 3, 1755–1766. doi:10.1109/TSG.2012.2212032
Brahman, F., Honarmand, M., and Jadid, S. (2015). Optimal electrical and thermal energy management of a residential energy hub, integrating demand response and energy storage system. Energy Build. 90, 65–75. doi:10.1016/j.enbuild.2014.12.039
Byeon, G., Yoon, T., Oh, S., and Jang, G. (2013). Energy management strategy of the DC distribution system in buildings using the EV service model. IEEE Trans. Power Electron. 28, 1544–1554. doi:10.1109/TPEL.2012.2210911
Eini, R., Linkous, L., Zohrabi, N., and Abdelwahed, S. (2021). Smart building management system: performance specifications and design requirements. J. Build. Eng. 39, 102222. doi:10.1016/j.jobe.2021.102222
Erixno, O., Rahim, N. A., Ramadhani, F., and Adzman, N. N. (2022). Energy management of renewable energy-based combined heat and power systems: a review. Sustain. Energy Technol. Assessments 51, 101944. doi:10.1016/j.seta.2021.101944
Ferraro, M., Brunaccini, G., Sergi, F., Aloisio, D., Randazzo, N., and Antonucci, V. (2020). From Uninterruptible Power Supply to resilient smart micro grid: the case of a battery storage at telecommunication station. J. Energy Storage 28, 101207. doi:10.1016/j.est.2020.101207
Ghosh, A., Zapata, M. Z., Silwal, S., Khurram, A., and Kleissl, J. (2022). Effects of number of electric vehicles charging/discharging on total electricity costs in commercial buildings with time-of-use energy and demand charges. J. Renew. Sustain. Energy 14. doi:10.1063/5.0086924
Hakimi, S. M., and Hasankhani, A. (2020). Intelligent energy management in off-grid smart buildings with energy interaction. J. Clean. Prod. 244, 118906. doi:10.1016/j.jclepro.2019.118906
He, W., Huang, G., and Markides, C. N. (2023). Synergies and potential of hybrid solar photovoltaic-thermal desalination technologies. Desalination 552, 116424. doi:10.1016/j.desal.2023.116424
Jamil, K., Dunnan, L., Awan, F. H., Jabeen, G., Gul, R. F., Idrees, M., et al. (2022). Antecedents of consumer’s purchase intention towards energy-efficient home appliances: an agenda of energy efficiency in the post COVID-19 era. Front. Energy Res. 10. doi:10.3389/fenrg.2022.863127
Jasim, M. A., Ahmed, O. K., and Alaiwi, Y. (2023). Performance of solar stills integrated with PV/Thermal solar collectors: a review. NTU J. Renew. Energy 4, 97–111. doi:10.56286/ntujre.v4i1.456
Kath, C., and Ziel, F. (2021). Conformal prediction interval estimation and applications to day-ahead and intraday power markets. Int. J. Forecast. 37, 777–799. doi:10.1016/j.ijforecast.2020.09.006
Li, P., Lu, Y., Yan, D., Xiao, J., and Wu, H. (2021). Scientometric mapping of smart building research: towards a framework of human-cyber-physical system (HCPS). Automation Constr. 129, 103776. doi:10.1016/j.autcon.2021.103776
Lin, G., Qi, B., Ma, C., and Rostam, F. (2024). Intelligent electric vehicle charging optimization and horse herd-inspired power generation for enhanced energy management. Energy 291, 130395. doi:10.1016/j.energy.2024.130395
Manivannan, R. (2024). Research on IoT-based hybrid electrical vehicles energy management systems using machine learning-based algorithm. Sustain. Comput. Inf. Syst. 41, 100943. doi:10.1016/j.suscom.2023.100943
Misbah Inayat, S., Rafay Zaidi, S. M., Ahmed, H., Ahmed, D., Kausar Azam, M., and Ahmad Arfeen, Z. (2023). Risk assessment and mitigation strategy of large-scale solar photovoltaic systems in Pakistan. Int. J. Industrial Eng. Manag. 14, 105–121. doi:10.24867/IJIEM-2023-2-327
Mohammed, A., Saif, O., Abo-Adma, M., Fahmy, A., and Elazab, R. (2024). Strategies and sustainability in fast charging station deployment for electric vehicles. Sci. Rep. 14, 283. doi:10.1038/s41598-023-50825-7
Nejatian, N., Abbaspour, M., Javidan, P., Nia, M. Y., Shacheri, F., Azizi, H., et al. (2023). Evaluation of the vulnerability and pathways of groundwater pollution in the Zanjanrud river basin by an integrated modeling approach. Model. Earth Syst. Environ. doi:10.1007/s40808-023-01897-x
Nikbakht Naserabad, S., Akbari Vakilabadi, M., and Ahmadi, M. H. (2023). Commercial building integrated energy system: sizing and energy-economic assessment. Int. J. Low-Carbon Technol. 18, 714–726. doi:10.1093/ijlct/ctad050
Nojavan, S., and Zare, K. (2013). Risk-based optimal bidding strategy of generation company in day-ahead electricity market using information gap decision theory. Int. J. Electr. Power & Energy Syst. 48, 83–92. doi:10.1016/j.ijepes.2012.11.028
Nojavan, S., Zare, K., and Ashpazi, M. A. (2015). A hybrid approach based on IGDT–MPSO method for optimal bidding strategy of price-taker generation station in day-ahead electricity market. Int. J. Electr. Power & Energy Syst. 69, 335–343. doi:10.1016/j.ijepes.2015.01.006
Ourahou, M., Ayrir, W., El Hassouni, B., and Haddi, A. (2020). Review on smart grid control and reliability in presence of renewable energies: challenges and prospects. Math. Comput. Simul. 167, 19–31. doi:10.1016/j.matcom.2018.11.009
Pachar, S., Singh, R., and Wahid, M. A. (2021). Implication of renewable energy in sustainable development in India: future strategy. IOP Conf. Ser. Mater. Sci. Eng. 1149, 012020. doi:10.1088/1757-899X/1149/1/012020
Pamidimukkala, A., Kermanshachi, S., Rosenberger, J. M., and Hladik, G. (2024). Barriers and motivators to the adoption of electric vehicles: a global review. Green Energy Intelligent Transp., 100153. doi:10.1016/j.geits.2024.100153
Rahman, T., Othman, M. L., Mohd Noor, S. B., Binti Wan Ahmad, W. F., and Sulaima, M. F. (2024). Methods and attributes for customer-centric dynamic electricity tariff design: a review. Renew. Sustain. Energy Rev. 192, 114228. doi:10.1016/j.rser.2023.114228
Sechilariu, M., Wang, B., and Locment, F. (2013). Building-integrated microgrid: advanced local energy management for forthcoming smart power grid communication. Energy Build. 59, 236–243. doi:10.1016/j.enbuild.2012.12.039
Shirazi, E., and Jadid, S. (2015). Optimal residential appliance scheduling under dynamic pricing scheme via HEMDAS. Energy Build. 93, 40–49. doi:10.1016/j.enbuild.2015.01.061
Siddiqui, O., Ishaq, H., Khan, D. A., and Fazel, H. (2024). Social cost-benefit analysis of different types of buses for sustainable public transportation. J. Clean. Prod. 438, 140656. doi:10.1016/j.jclepro.2024.140656
Taner, T., Naqvi, S. A. H., and Ozkaymak, M. (2019). Techno-economic analysis of a more efficient hydrogen generation system prototype: a case study of pem electrolyzer with Cr-C coated SS304 bipolar plates. Fuel Cells 19, 19–26. doi:10.1002/fuce.201700225
Tasdighi, M., Ghasemi, H., and Rahimi-Kian, A. (2014). Residential microgrid scheduling based on smart meters data and temperature dependent thermal load modeling. IEEE Trans. Smart Grid 5, 349–357. doi:10.1109/TSG.2013.2261829
Wei, X., Chammam, A., Feng, J., Alshammari, A., Tehranian, K., Innab, N., et al. (2024). Power system monitoring for electrical disturbances in wide network using machine learning. Sustain. Comput. Inf. Syst. 42, 100959. doi:10.1016/j.suscom.2024.100959
Wi, Y.-M., Lee, J.-U., and Joo, S.-K. (2013). Electric vehicle charging method for smart homes/buildings with a photovoltaic system. IEEE Trans. Consumer Electron. 59, 323–328. doi:10.1109/TCE.2013.6531113
Yan, Z., Duan, X., Chang, Y., Xu, Z., and Sobhani, B. (2023). Optimal energy management in smart buildings with electric vehicles based on economic and risk aspects using developed whale optimization algorithm. J. Clean. Prod. 415, 137710. doi:10.1016/j.jclepro.2023.137710
Yin, Q., Anser, M. K., Abbas, S., Ashraf, J., Ahmad, M., Jamshid, J., et al. (2022). Integrating the role of green fiscal policies with energy prices volatility and energy efficiency: presenting a COVID-19 perspective. Front. Energy Res. 9. doi:10.3389/fenrg.2021.838307
Keywords: smart home, electricity energy, energy management, electric vehicle, time of use, inclining block rate
Citation: Dodo YA, Ibrahim AO, Abuhussain MA, Baba Girei ZJ, Maghrabi A and Naibi AU (2024) An innovative method for building electricity energy management in smart homes based on electric vehicle energy capacity. Front. Energy Res. 12:1364904. doi: 10.3389/fenrg.2024.1364904
Received: 03 January 2024; Accepted: 06 February 2024;
Published: 21 February 2024.
Edited by:
Mohammad Hossein Ahmadi, Shahrood University of Technology, IranReviewed by:
Maher Dhahri, University of Sousse, TunisiaCopyright © 2024 Dodo, Ibrahim, Abuhussain, Baba Girei, Maghrabi and Naibi. This is an open-access article distributed under the terms of the Creative Commons Attribution License (CC BY). The use, distribution or reproduction in other forums is permitted, provided the original author(s) and the copyright owner(s) are credited and that the original publication in this journal is cited, in accordance with accepted academic practice. No use, distribution or reproduction is permitted which does not comply with these terms.
*Correspondence: Mohammed Awad Abuhussain, bWFhYnVodXNzYWluQG51LmVkdS5zYQ==; Ahmed Osman Ibrahim, YWguaWJyYWhpbUB1b2guZWR1LnNh
Disclaimer: All claims expressed in this article are solely those of the authors and do not necessarily represent those of their affiliated organizations, or those of the publisher, the editors and the reviewers. Any product that may be evaluated in this article or claim that may be made by its manufacturer is not guaranteed or endorsed by the publisher.
Research integrity at Frontiers
Learn more about the work of our research integrity team to safeguard the quality of each article we publish.