- 1School of Science, Western Sydney University, Penrith, NSW, Australia
- 2Energy Centre, Commonwealth Scientific and Industrial Research Organisation (CSIRO), Mayfield West, NSW, Australia
- 3National Vegetable Protected Cropping Centre, Western Sydney University, Penrith, NSW, Australia
- 4Hawkesbury Institute for the Environment, Western Sydney University, Penrith, NSW, Australia
- 5Global Centre for Land Based Innovation, Western Sydney University, Penrith, NSW, Australia
- 6College of Management, Fu Jen Catholic University, Taipei, Taiwan
High-tech protected cropping holds great potential to improve global food security, but high cooling energy costs in warm climates pose difficulties in propagating the industry. Emerging technologies, such as diffuse glasses fitted with photoselective thin films, have interactions with crops and other cooling technologies which are not well-characterized for warm-climate glasshouses. A light-blocking film (LBF) was chosen as a high-tech, climate-controlled greenhouse cover permitting transmission of 85% of photosynthetically-active light and blocking heat-generating radiation. Two consecutive 7-month trials of two capsicum crops were grown under warm climate conditions partially impacted by bushfire smoke, with 2 cultivars (Gina and O06614) in the first trial, and 2 cultivars (Gina and Kathia) in the second trial. Capsicum fruit yield decreased by 3% in Gina and increased by 3% in O06614 for the first trial, and decreased by 13% in Gina, 26% in Kathia for the second trial. Cooling energy use increased by 11% and 12% for both capsicum crops in AE and SE respectively, with small but insignificant decreases in fertigation demand (2%–5%). Cooling potential was significantly different from material specifications, with indications that convection from LBF interfaces was responsible for higher heat loads. LBF and similar absorptive glasses may still be beneficial for reducing nutrient, water, and energy use in warm climate glasshouses. However, yield is cultivar-dependent and may decrease with below-optimal crop lighting, whereas energy savings are more dependent on LBF orientation and building geometry than outside climate.
1 Introduction
The United Nations projects that by 2050, the global population will reach 9.7 billion people, and in the 2080s it is expected to reach 10.4 billion people (United Nations, Department of Economic and Social Affairs, Population Division, 2022). Rising population and the reduction of arable land per person, combined with the increased frequency of extreme weather events, is putting food security at risk (Benke and Tomkins, 2017; Clercq et al., 2022; Data, 2023). There is an urgent need for agricultural practices to adapt to these conditions (Anderson et al., 2020), noting that to meet 2050 food demands, global food production will need to increase by 70% (Clercq et al., 2022). Thus, agricultural practices need to become more intensive and sustainable. One of the promising cropping methods that can increase food production per land area is protected cropping (Maier et al., 2022).
Protected cropping provides shelter against abiotic and biotic stresses to the crop, and facilities range from low-tech shade houses to highly-automated glasshouses (Chavan et al., 2022; Maier et al., 2022). Greenhouses produce much higher yields than broad acre agriculture as the crop is protected from suboptimal weather events and pests and diseases (Smith, 2011; Hadley, 2017; Maier et al., 2022). The highest yields are achieved in high-tech glasshouses where the climate is continuously monitored and controlled (Chavan et al., 2022; Maier et al., 2022), allowing for the maintenance of optimal growth conditions for the plants to reach their genetic potential of yield and quality (Rabbi et al., 2019). However, high capital investment for high-tech protected cropping facilities is a barrier for many growers as this usually requires a substantial investment for sensor technology and infrastructure (Barbosa et al., 2015). Labor and energy are the two most expensive operating costs after the initial capital investment. For this reason, energy efficient cooling and heating methods are necessary for high-tech protected cropping agriculture to prosper.
Heating and cooling processes to maintain optimal growth conditions account for 65%–85% of energy consumption within a glasshouse (Rabbi et al., 2019; Gorjian et al., 2021). Fossil fuels are still heavily used for heating in glasshouses and renewable energy sources do not consistently produce enough energy to compensate for the energy needed at night for heating (Anifantis et al., 2017). Various renewable energy production methods are being investigated for heating such as photovoltaics paired with ground source heat pumps (Anifantis et al., 2017), ground source heat exchangers (Baddadi et al., 2019), solar air heaters (Baddadi et al., 2019) and Fresnel lens solar collectors with phase change materials as thermal heat storage (Najjar and Hasan, 2008; Baddadi et al., 2019). There are several cooling methods that are used in protected cropping, including active technologies which require continuous energy input to drive cooling and passive technologies (e.g., shade curtains/thermal screens, natural ventilation systems and earth-to-air heat exchangers) which require little or no energy to trigger continuous cooling. Pad and fan technology has been used primarily in this environment along with venting and fogging apparatuses (Soussi et al., 2022). In Australian protected cropping, the primary necessity is for cooling because of abundant solar energy and mild winters (Montagu, K. 2018; Soussi et al., 2022).
In passive systems, heat gain and dissipation are primarily controlled by the shape, orientation, cover material, and the pattern of openings of the glasshouse as solar radiation incident in a glasshouse is the first source of heat gain and the largest contributor to daytime temperature (Soussi et al., 2022). However, these passive cooling systems do not allow for precise control of temperature (Mongkon et al., 2014; Soussi et al., 2022). Combining pad and fan systems with a half-shaded roof has been shown to keep temperatures 10% cooler and to work particularly well in arid climates (Kittas et al., 2000), but also uses a lot of water. The presence of a temperature gradient along the laminar flow path of the greenhouse compartment, with the coolest temperatures closer to the pad and warmest temperatures closer to the fan, creates microclimates within the crop that are not ideal for optimal yield and quality (Soussi et al., 2022). Cooling methods, including semitransparent photovoltaics, novel cover materials, and wavelength selective window tinting (Emmott et al., 2015; Loik et al., 2017), may be viable alternatives to reducing heat gain without using electricity, while allowing the crop to receive adequate light.
Semitransparent photovoltaics and cover materials that help with energy efficiency and thermal heat gain can negatively impact the light environment, thus reducing crop yield. This is especially significant for high-light, fruiting horticultural crops such as tomato, cucumber and capsicum, whereas other crop varies such as lettuce are more tolerant of shading (Cossu et al., 2020). Window tinting reduces the emissivity of the glass and reflects unwanted wavelengths of light that contribute to heat, thus reducing energy usage to maintain optimal indoor temperatures (Chaiyapinunt et al., 2005). Some window tinting films have been developed specifically to have low emissivity to circumvent this issue, such as ULR-80 (Solar Gard, Saint-Gobain Performance Plastics, Sydney, NSW, Australia). Due to ULR-80’s light-blocking properties, it is referred to here as a “light-blocking film” (LBF). The ability that high-tech glasshouses have to tightly control crop microclimates makes them an ideal testing environment to understand the impact of new cover materials and window tinting materials such as ULR-80 on energy efficiency (Zhang et al., 2020). The LBF has been found to reduce energy usage and to maintain optimal environmental conditions for eggplant growing (Chavan et al., 2020; Lin et al. 2022). However, the LBF has only been tested for eggplant, and testing with other crops is necessary given that different crops require different growth parameters and environmental conditions.
Capsicum annuum L. (referred to as capsicum herein) is one of five domesticated pepper varieties (the four others being Capsicum baccatum L., Capsicum chinense Jacq., Capsicum frutescens L., and Capsicum pubescens Ruiz and Pav) and is an important horticultural crop globally (Kraft et al., 2014). Capsicum was first domesticated in Mexico and has a broad-acre yield of 12 kg m-2; however, in greenhouses it may yield 30 kg m-2 (Smith, 2011). For Australia, capsicum historically has been grown in open field situations. However, with the benefit of blemishless fruit and high yields from glasshouse cultivation, capsicum production is increasingly being grown indoors. Casierra-Posada et al. (2014) showed that capsicum growth indices were relatively unaffected by different colored films, suggesting that capsicum may be a resilient crop grown under an array of light environments and may be ideal for production under LBF.
In this study, it was hypothesized that cooling energy, water and nutrient use would be reduced under LBF, given that eggplant and capsicum have similar temperature requirements and are grown over similar crop cycle lengths, with eggplant generally demanding slightly shorter growing periods and utilizing slightly more solar energy (Nonnecke, 1989; Asgharipour et al., 2020). In this study, the energy consumption used for heating and cooling across two capsicum crop cycles was assessed across the LBF and control growth environments. This paper examines the effect of LBF on energy-, water- and nutrient-use of glasshouse capsicum with a focus on operational characterization. Changes in cooling energy usage are highlighted with respect to outside climate variables such as irradiance and air temperature.
2 Materials and methods
2.1 Experimental design and LBF characteristics
The experiment was conducted over two crop cycles using red and orange varieties of Capsicum annuum L. The first crop cycle was initiated on 5 April 2019 and completed on 5 December 2019 using Gina (red) and O06614 (orange). The second crop was initiated on 17 January 2020 and completed on 23 September 2020 using Gina (red) and Kathia (orange). These two crop cycles are differentiated by season (Autumn Experiment; AE, and Summer Experiment; SE). All crop varieties were sourced from Syngenta Australia (Macquarie Park, NSW, Australia). Both crop trials took place in the National Vegetable Protected Cropping Centre (NVPCC), established jointly by Western Sydney University (WSU) and Horticultural Innovation Australia as an educational and research facility located at WSU’s Hawkesbury Campus in Richmond NSW (Latitude: -33.61° S, Longitude: 150.75° E).
The facility is an 1800 m2 high-tech, multi-span gable roof hydroponic glasshouse which is environmentally monitored and controlled by Priva hardware and software (Priva, De Leir, Netherlands). The facility is comprised of 8 × 105 m2 research compartments and 1 × 400 m2 training/education compartment; 4 research compartments are utilized in this experiment. There is also a temperature-controlled walkway for greenhouse workers which additionally serves as a buffer zone against pests. All research compartments were fitted with HD1AR 70% hazed glass, and the walls were fitted with tempered clear floatglass; two of these research compartments were controls. In the LBF treatment rooms, two research compartments had ULR-80 window film (Solar Gard, Saint-Gobain Performance Plastics, Sydney, NSW, Australia) applied to the glass on the underside of ceilings and inner side of walls. ULR-80 [light-blocking film, Saint-Gobain] is a low emissivity, commercially available residential and industrial window tinting which blocks ≈88% of heat-generating light in 780–2,500 nm (infrared and far-infrared) region and >99% of light in 300–400 nm (ultraviolet) region, while allowing most light in the photosynthetically active radiation (400–700 nm) region (Chavan et al., 2020). Wavelength-averaged absorptance and reflectance were 54% and 6% respectively, compared to 9% and 8% for control floatglass. The solar heat gain coefficient was 0.57 compared to 0.86 for floatglass, whereas the winter U-factor was 6.0 compared to 5.9 W/m2 °C according to manufacturer specifications.
Seedlings were grown on 1 m long Grodan Grotop Expert rockwool slabs (Roermond, Limburg, Netherlands), which are chemically inert. Four plants were transplanted onto each slab and each gutter had 10 slabs equaling a total of 240 plants across 6 gutters per compartment. Two weeks after transplantation, two stems were selected from each plant and trellised using a supported high-wire setup with strings. Commercial hydroponic practices were followed throughout the growth cycle using the following conditions: electric conductivity (EC): 2.5–3.0 dS m-1; pH: 5.5–6.0; [CO2]: 489.6 and 476.6 μL L−1 daytime average; air temperature: 25.3/19.3 and 25.2/19.3°C day/night average; relative humidity (RH): 74.2/72.9 and 74.2/77.5% day/night average, AE and SE respectively, and natural light.
2.2 Glasshouse cooling and heating devices
Each compartment (both LBF and Control) was cooled through both active and passive cooling methods. Active cooling used two fan coil units per room (Kruger KDD 9/9 550 W 4P-1 3SY, Kruger Engineering, Singapore), each processing up to 800 L s-1 of air at low static pressure. These units were fed with ≈6 °C water from HFC-134a chillers (Model AD028 CE.1BH06·F4AEBA.A006AA.E10, Smardt Chillers, Australia), and were equipped with energy meters (Siemens UH50-G50P-AU06-F, Siemens, Germany). Passive cooling consisted of two rooftop vents that spanned the length of each room. These vents released hot air through external and internal pressure differences generated by leeward wind movement and air buoyancy. In all rooms, water fogging systems were installed above canopy height (5 m above ground level) and were used to maintain RH set points. The foggers dispersed heat through evaporative cooling; hence, removing this humid air from the glasshouse via ventilation was expected to impact energy usage of the facility. For this reason, the cooling energy usage was evaluated based on energy consumption of the chiller and outside climate variables. Shade screens (Harmony 5,045, Svenssen, Sweden) were installed in each room. All rooms were controlled using the same shading strategy which was automated by the Priva system.
Each room was heated using hot water pipes installed at ground level and which ran the length of the floor in between the gutters These pipes also served as the support for the trolleys and lifts for maintenance activities. The hot water was heated and distributed via a gas boiler (Rendamax R600, Elco B·V, Netherlands). All rooms were heated via this method. However, only the C3 research bay (LBF treatment room) had an energy meter installed to track representative heating energy usage (SCYLAR DE-10-MI004-PTB004, Diehl, Germany), which was calculated for other rooms based on the hot water flow rate and temperature changes. All relevant control devices and sensors are outlined in Figure 1.
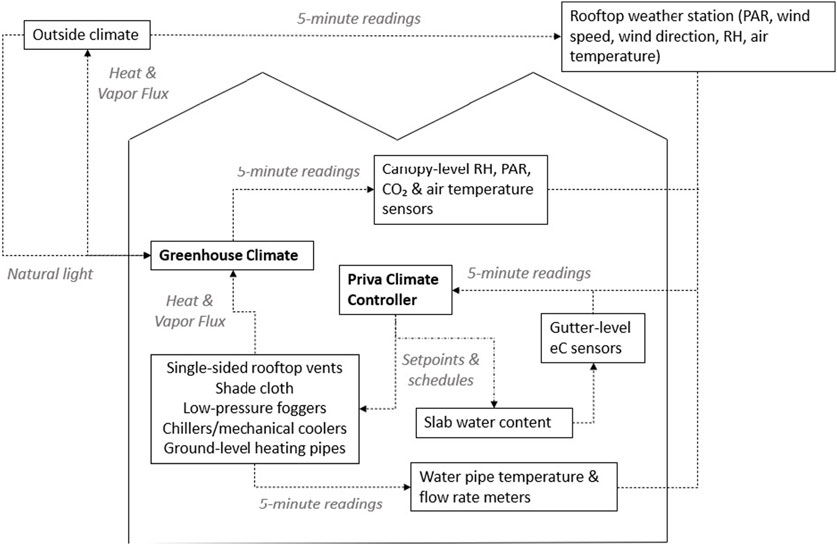
FIGURE 1. Basic control diagram for the greenhouse outlining all relevant control devices and sensors in this study.
2.3 Data collection and statistical analysis
Fruit weight and number for mature fruit were measured weekly beginning from the middle of week 10 in AE and the start of week 11 in SE. Bud-, flower-, and fruit-count were conducted on 20 stems per cultivar at 2 weeks frequency, though these have not been included in this study. Side gutters were excluded from yield calculations to remove edge effects, leaving 160 plants (80/cultivar) per room.
The Priva system software and hardware managed all aspects of environmental control, including ventilation, cooling, heating, and fertigation in the NVPCC glasshouse. Plants were provided non-limiting fertigation by the grower and the Priva system based on slab electroconductivity and water content percentage (Grosens sensor, Grodan, Roermond, Netherlands) in each compartment. Canopy-level temperature, humidity, and PAR were measured by the Priva E-measuring box (Priva E-Measuring Box; Priva, Ontario, Canada). All such greenhouse microclimate data was logged by Priva at 5-min intervals. Outside climate data, including air temperature, global horizontal radiation, humidity, wind speed and wind direction was also logged by the greenhouse rooftop weather station at 5-min intervals. Rainfall data was acquired from the Bureau of Meteorology (Bureau of Meteorology, 2024). Aerosol optical depth, UV/VIS aerosol index, and terrain reflectivity for the site location were acquired from the Ozone Monitoring Instrument (OMI) on board NASA’s Dutch-Finnish Aura satellite via Earthdata (OMI-Aura, Goddard Earth Science Data and Information Service Centre [GES DISC]).
Matlab 2019 (Mathworks, Natick, Massachusetts, United States) was used to perform all data processing and statistical analysis, including cleaning, pre-processing, fitting, predicting, interpolating, and plotting data. Priva data were analyzed on daily and hourly scales with missing values of sizes from 1 – 5 timepoints replaced by linear interpolations; days with larger gaps were discarded. This discarded data amounted to 2 days (0.67%) in AE and 9 days (3.2%) in SE. Cooling and heating energy use were calculated by energy meters based on supply and return water temperatures, pump activity, and water flowrates. Predictions of seasonal performance were based on linear regression analyses. One-way ANOVA was used to quantify statistical significance for bar plots, whereas p-values were used in cases of multiple regression.
Elastic net regression was employed to examine the relationship between outside climate variables as predictors, and cooling energy usage as a response variable over daylight periods from 6 a.m. to 9 pM. Elastic net is a regularized ordinary least squares (OLS) regression combining both L1 (LASSO) and L2 (Ridge) penalties during regularization to handle multicollinearity and provide feature selection. The objective is to encourage sparsity in the model by shrinking some of the estimated coefficients towards zero, allowing selection of the most relevant variables for prediction by pooling contributions of highly correlated predictors together. Given the expectation of correlation between environmental variables such as temperature and light, upon causally constraining input features, this approach improves the interpretability of the regression coefficients. Thus, it is expected to afford limited causal inference by contrasting relative magnitudes of regression coefficients for treatment and control (Pichler and Hartig, 2023 PREPRINT). To determine the optimal value for λ, the tuning hyperparameter controlling the bias-variance tradeoff in the penalty function, 10-fold cross-validation was performed and repeated 100 times to ensure robustness. The decision tree size with the lowest mean squared error (MSE) plus one standard deviation was chosen as the optimal criterion for λ selection. The average wind direction was handled as a circular variable by taking the four-quadrant inverse tangent of instantaneous wind directions and speeds.
3 Results
3.1 Smart glass heating and cooling performance variation with climate
Table 1 compares selected mean external conditions to greenhouse conditions taken over the course of both experiments, for control and LBF rooms. There were no significant differences in air temperature between control and LBF compartments due to heating, ventilation, and air conditioning (HVAC) regulation, as the Priva system adjusted temperature set-points throughout the day to accommodate changes in cooling needs (Table 1). However, CO2 and RH levels were lower in LBF compartments across both experiments, and RH especially in SE LBF compartments (−13.3%). This reflects a reduction in evapotranspiration under LBF for the two capsicum crops.
Compared to control glass, LBF reduced overall PAR by an average of 27.1% (95% CI [15.45 mol m-2, 17.12 mol m-2]) in AE and 27.8% (95% CI [13.09 mol m-2, 14.52 mol m-2]) in SE (Supplementary Figure S1). However, LBF increased cooling energy use by 10.6% (95% CI [209.90 kWh, 238.98 kWh]) in AE and 11.6% (95% CI [166.32 kWh, 190.94 kWh]) in SE (Figure 2). For periods when shade screens were not operating, LBF was instead found to increase cooling energy use by 10.7% (95% CI [184.92 kWh, 209.74 kWh]) in AE and by 19.2% (95% CI [132.14 kWh, 154.57 kWh]) in SE, indicating that shading lowered temperatures to a greater degree under LBF.
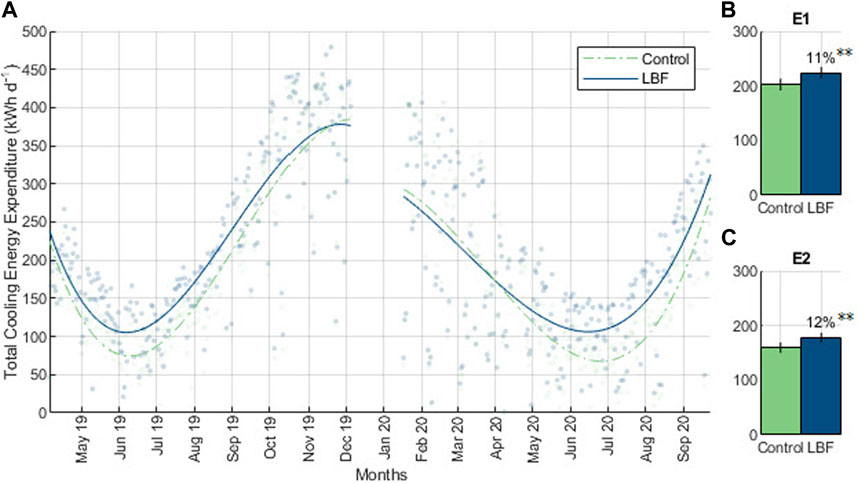
FIGURE 2. Comparison of total cooling energy expenditure between the Control and LBF during two capsicum crop cycles. LBF was found to increase cooling energy use during both experimental periods, with a larger disparity during cooler months. (A) Cooling energy use for both LBF and control groups as a scatter plot, with curves fitted via kernel smoothing. (B,C) Bar plot of mean daily cooling energy use for AE and SE respectively. Error bars represent 95% confidence intervals. **p < 0.05.
The peak for outside air temperature occurred at approximately 2 p.m., whereas the peaks for cooling energy usage were slightly before 12 p.m. for LBF compartments and slightly after 12 p.m. for control compartments (Figure 3). Additionally, LBF rooms displayed increased cooling load primarily before noon, with cooling profiles more similar after noon. LBF rooms also used less energy on heating in SE outside of cooling periods, which may be indicative of increased heat retention under LBF in this period. Similarly, SE compartments used less energy on cooling compared to AE compartments despite being presented with higher outside air temperatures, which is related to decreased irradiance in the same season (Supplementary Figure S1).
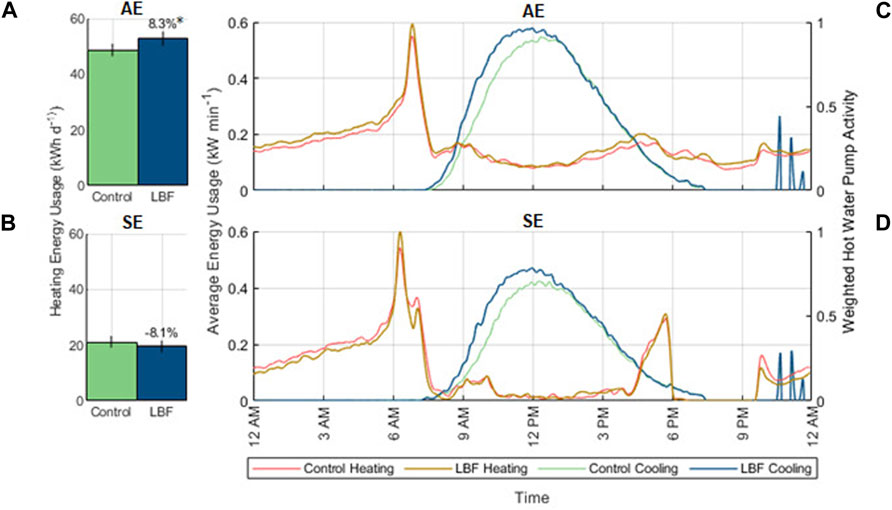
FIGURE 3. Comparison of daily average cooling and heating energy usage between the Control and LBF during two capsicum crop cycles. Heating energy usage was significantly higher in AE, and lower in SE (but not significantly lower). Cooling energy usage was higher in both AE and SE. (A,B) Daily heating energy usage for LBF and control groups, calculated from hot water pump activity and supply/return water temperatures. Error bars represent 95% confidence intervals. *p < 0.05 (C,D) Cooling energy usage and weighted hot water pump activity per minute in AE and SE, with smoothed spline curve fitting, averaged for LBF and control groups to describe a typical experiment day. Heating uses second y-axis.
LBF compartments also required more energy for heating in AE (8.3%) and less energy in SE (−8.1%) compared to control compartments, with the discrepancy between seasons largely due to warmer temperatures in SE. A comparison of robust Cohen’s d for LBF and control groups reveals that the magnitudes of the differences between heating loads and cooling loads was very large in AE (d = 1.21) and SE (d = 0.94) respectively, such that heating loads could be considered relatively unimportant. However, it must be noted that the cooling system was refrigeration-based and prioritized thermal control over energy efficiency. If a commercial greenhouse cooling system such as fan-pad were used, and the current heating system were to remain installed, then heating energy use would form a larger portion of the greenhouse energy profile and may require greater focus.
3.2 Modelling the performance of LBF under diverse climate conditions
Variations in energy-saving performance observed across both experiments highlighted the LBF film’s sensitivity to different climate conditions. Two separate elastic net regression models were developed for control and LBF rooms to further investigate the cause of this sensitivity. The α hyperparameter (α = 0.5) was chosen to equally enforce ridge and LASSO penalties. The tuning hyperparameters (λControl = 0.1148; λLBF = 0.1162) were chosen after averaging λ from 10-fold cross-validation repeated 100 times, with minimal mean squared error (MSE) plus one standard deviation chosen as a standard metric of classifier accuracy (Krstajic et al., 2014). Elastic net regression is not commonly used for causal inference owing to the regularization bias applied to predictors from penalized regression which is stronger for collinear than independent features (Pichler and Hartig, 2023 PREPRINT). Nevertheless, the regression coefficients may still be analyzed in terms of sign and magnitude if information is provided about their appearance rate during variable selection for bootstrapped samples, whereby the penalization is useful for grouping the contributions of highly correlated predictors (Hüls et al., 2017). The estimated parameters and their p-values listed in Table 2 were selected after re-sampling with bootstrapping (n = 500) and are valid for p < 0.05, where p relates to the rate of selection by lasso during training and thus directly addresses type 1 error.
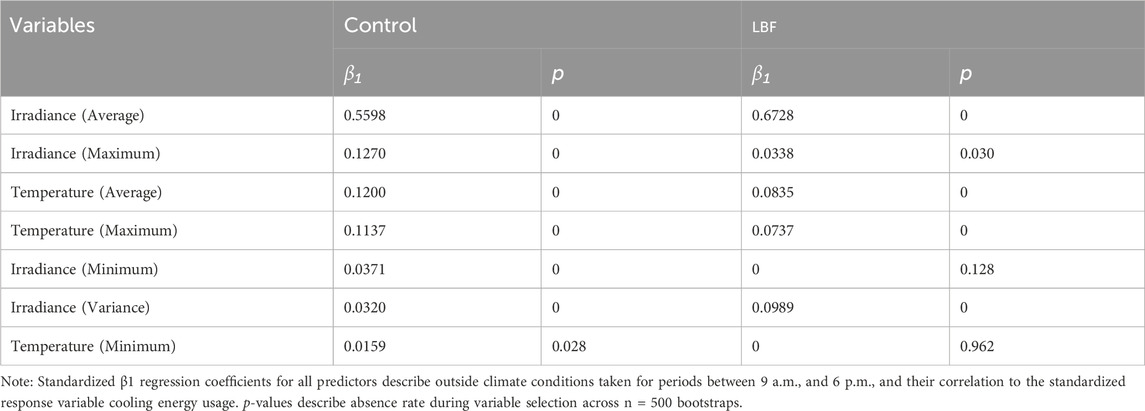
TABLE 2. Significant elastic net regression coefficients for cooling energy usage in control and LBF rooms across combined and standardized AE and SE data.
Each model was first built by partitioning AE and SE data into training and testing datasets before validation. Model validation indicated no significant change in fit in all cases (R2 > 0.90). Both full models described high positive correlations to cooling energy usage, with R2 = 0.93 for control rooms and R2 = 0.92 for LBF rooms. Training and testing sets were combined for the full models.
It was noted that ridge regression aims to improve the stability of β1 coefficient estimates in the presence of highly correlated predictors by distributing their effect across sets of correlated predictors. Therefore, the contributions of several predictors listed in Table 2, such as average irradiance which is typically considered a highly impactful predictor for energy usage, have altered magnitudes as they are described by the contributions of more strongly-correlated predictors. The predictors in Table 2 were then used to predict energy savings for equivalent daylight periods in other climates using 1-h resolution typical meteorological year (TMY) climate data (Table 3).
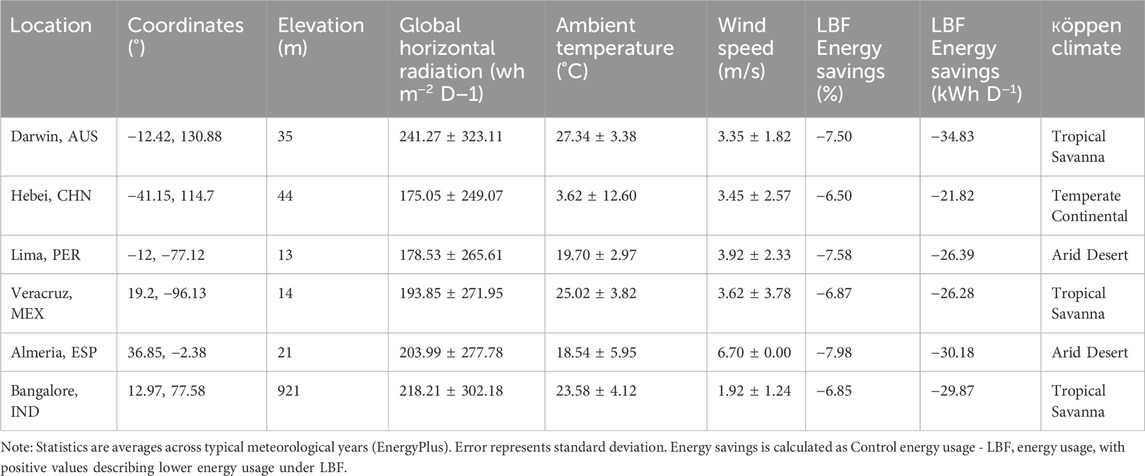
TABLE 3. Descriptive statistics and predicted cooling energy savings for different climates across the world.
It was also of interest to examine whether the energy savings (ΔE) under LBF, calculated as the difference score for energy usage ΔE = Coolingcontrol–CoolingLBF, could be evaluated directly by another elastic net regression model. Elastic net regression models aim to prune out all zero-valued β1 coefficients, complicating significance testing between individual models. Conversely, as chosen coefficients are non-zero, correlations to ΔE can describe the significance and direction of differences in energy usage between rooms. The tuning parameter λ = 0.0607 was chosen as described for previous models. This reduced elastic net regression model retained an R2 of 0.60 compared to 0.70 from a least-squares regression with bi-square weighting, the latter using 66 main effects and interaction terms as predictors. The estimated parameters and their p-values in this model are presented in Table 4.
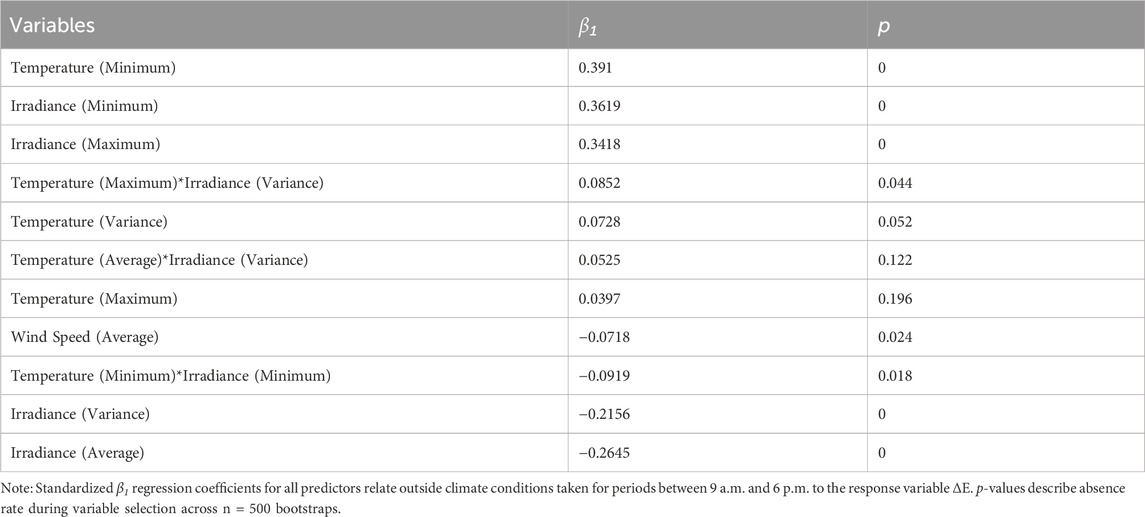
TABLE 4. Significant elastic net regression coefficients for LBF energy savings across combined and standardized AE and SE data.
3.3 Capsicum cultivar performance under LBF is differentially impacted by climate
LBF compartments demanded increased fertigation in AE and decreased fertigation in SE, wherein the quantity of nutrient water pumped was based on measured slab electroconductivity (EC). However, overall nutrient water use, calculated as the difference between pumped and drained nutrient water, did not change significantly compared to control compartments in AE (−2.17%) and in SE (−5.17%). It is difficult to state whether this slight reduction in nutrient water use is related to higher room temperatures or to altered plant photosynthesis under LBF (Figure 4).
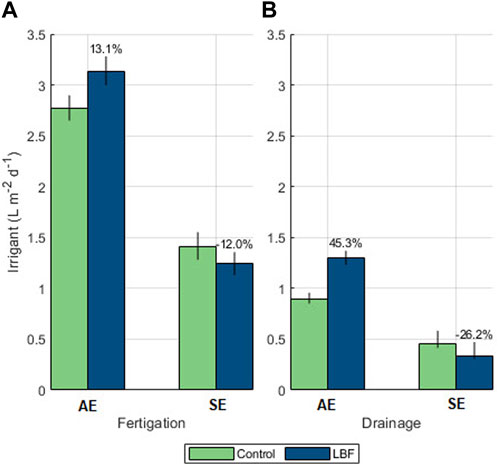
FIGURE 4. LBF compartments saw altered fertigation demands compared to control compartments, but differences in actual fertigant use were not significant. Bar plots for measured fertigation, averaged for LBF and control groups. (A) Total amount of nutrient and water mix allocated to each 105 m2 room each day. (B) Total amount drained each day. Error bars represent 95% confidence intervals.
Fruit yield for LBF rooms was not significantly different for either capsicum cultivar in AE. However, yield was significantly lower in SE LBF rooms, with a larger reduction in the orange cultivar (−25.9%) compared to the red cultivar (−12.6%) indicating cultivar-specific reactions to the changes in light intensity and quality under LBF (Figure 5). Additionally, fruit number was lower under LBF in both AE and SE for all cultivars, with a greater disparity during SE. SE involved cloudier conditions and warmer air temperatures which may have been influenced by bushfires in the downtime between experiments. As compartment air temperatures were stabilized by HVAC (Table 1), it is possible that light-limiting climate conditions coinciding with young crop development may have impacted crop performance.
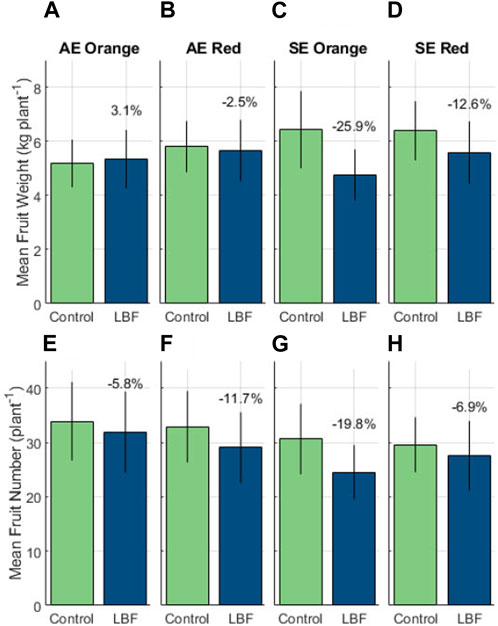
FIGURE 5. LBF reduced fruit weight in SE across both cultivars, and reduced fruit number in both AE and SE across both cultivars, excluding side gutters. Bar plot of means for both cultivars; fruit weight in AE (A,B) and SE (C,D); fruit number in AE (E,F) and SE (G,H). All error bars represent standard error of mean, noting that high error was greatly influenced by the final harvest where all fruits were collected. (n = 80 plants/cultivar/gutter).
4 Discussion
4.1 Interaction of the greenhouse energy use and crop performance under LBF
A range of crops including capsicum, eggplants, cucumbers, and lettuces have been tested in the NVPCC glasshouse facility using the LBF. Comparing the effect of LBF on greenhouse energy use and performance of different crops will provide important insight into their profound interactions and useful guidance to growers. A previous energy analysis on the LBF was conducted across two crop cycles of eggplant (Solanum melongena, referred to as eggplant herein) (Lin et al., 2022). In this case, cooling energy usage was 4.4% lower under LBF for both warm and cold weather conditions, with significant decreases in fertigation demand (18%–29%) and fruit yield (21%–25%). Both capsicum and eggplant belong to the Solanaceae family and require a DLI of 20–30 mol m-2 d-1, a relative humidity range of 50%–70%, and optimal temperature of 22°C–30 °C during the day and 14°C–16 °C at night (Rabbi et al., 2019; Cossu et al., 2020; Morgan, 2021; Soussi et al., 2022). While eggplant and capsicum have similar growth parameter requirements, they differ in plant phenology with much bigger leaf size for eggplant (Morgan, 2021). Capsicum also has a much higher leaf reflectance than eggplant that may impact heat gain within a glasshouse. Furthermore, the typical planting density for capsicum (5-6 stem m-2) is also different from eggplant (4-5 stems/m2) which will impact the thermal mass within the growing compartment and light penetration throughout the canopy. These crop related differences between eggplant and capsicum production impact the amount of energy needed to maintain optimal growth conditions (Samaranayake et al., 2020; He et al., 2021; Samaranayake et al., 2021). Climate parameter modeling within a glasshouse becomes especially important from the perspective of lifecycle costs as all climate parameters are linked and influence one another and more energy is required for glasshouse production compared to in the field (Zhang et al., 2020).
Significant differences were identified in the crop performance between AE and SE (Figure 5), which could be partially affected by the coincidence of bushfires over the 2019–2020 summer at the experiment location. It was initially hypothesized that air pollution due to bushfires may have affected yield during SE, as smoke and other atmospheric absorbents have been shown to significantly reduce PAR (-45%–95%) and ambient temperatures, and increase RH, ultimately reducing photosynthetic rate (Darrall et al., 1989; Davies and Unam, 1999; Fu et al., 2010; O'Carrigan et al., 2014). However, a cursory examination of the site location revealed that it was largely unaffected by smoke aside from near the end of AE and during the experimental downtime between AE and SE. Instead, it is more likely that changes in light intensity between AE and SE, combined with the shading effect of LBF, were responsible for yield disparities.
Several studies have investigated the effect of shading upon capsicum, though there does not appear to be a literary consensus. Díaz-Pérez. (2014) and Kabir et al. (2020) estimated optimal capsicum yield to occur on days with light intensity between 1,365–1,470 μmol m−2 s−1 at midday (1,200–1,500 h), with net photosynthesis maximal between 1,470–2,100 μmol m−2 s−1. Conversely, Ilić et al. (2017) reported increasing capsicum yields with decreasing light intensity between 700–1,000 μmol m−2 s−1 (1,300–1,400 h) and under light-shifting, light-diffusing, and light-blocking covers. Nevertheless, in all cases, yield improved because of increased fruit size, despite reduced fruit numbers under light-limiting conditions. It was evident in this study that only a small percentage of the full experimental duration was spent within the range of 700–1,500 μmol m−2 s−1 light intensity (1,200–1,500 h) for both AE (control: 32.9%, LBF: 24.7%) and SE (control: 25.5%, LBF: 16.0%) (Supplementary Figure S3). The experimental conditions for capsicum growth could be described as highly light-limiting, particularly during SE under LBF. Accordingly, though individual fruit weights improved with shading, high reductions in fruit number were observed during SE which account for the larger discrepancy seen in total yield. AE was characterized by a well-documented lack of precipitation alongside hot and dry conditions which served as a catalyst for the 2019-20 Australian bushfires, whereas SE instead experienced above average rainfall (Table 1) (Abram et al., 2021).
These findings are supported by a statistical evaluation of the difference in terrain reflectivity, equivalent to surface reflectance, between AE, SE, and preceding years (2011–2018). The significance of terrain reflectivity is that reflected light may be absorbed by aerosols or altered by cloud cover, such that decreasing surface albedo (given an original ground albedo >0.4) may serve as an indicator of fires and/or rainfall (Herman and Browning, 1975). The 2019-20 Australian bushfire season largely persisted from the first week of November 2019 before subsiding towards late January 2020, whereby smoke and other aerosols may have affected the final weeks of AE and the early weeks of SE. A cursory examination of the UV-Vis aerosol indices and aerosol optical thickness from 2019 through to 2020 was inconclusive regarding whether the site was affected by bushfire smoke within the experimental period, which is sensible given the timing of the experimental downtime period was during peak bushfire intensity (6 December 2019–16 January 2020). However, terrain reflectivity did decrease from the recent historical average of 26% down to ≈20.6% in the final weeks of AE, and from the recent historical average of 30% down to ≈24.6% in the early weeks of SE (Supplementary Figure S4). Nevertheless, AE may represent yield under more standard climate conditions as bushfire smoke could have only affected the mature crop near the end of AE, compared to torrential rain causing larger decreases in PAR throughout SE.
4.2 LBF performance increases with higher minimum temperatures
The lower correlation (R2 = 0.60) for the energy savings model is impacted by error propagation at the day scale as variance in the difference score is less predictable. Nevertheless, this model provided detail about the most significant factors influencing energy savings under LBF. The β1-values in Table 4 then describe the relative magnitudes of the differences observed in the individual room-level models in Table 2.
In analyzing main effects for the individual room models, the strongest predictor of cooling energy usage in either room was average outside irradiance. The lower coefficient for average outside irradiance in control rooms (β1 = 0.5598) compared to LBF rooms (β1 = 0.6728) suggests that changes in outside irradiance have a relatively larger effect on cooling energy usage in LBF rooms, i.e., the presence of the LBF increases the impact of light fluctuations on the indoor environment relative to control glass. Energy usage under LBF was less correlated to minimum irradiance (β1 = 0) and maximum irradiance (β1 = 0.0338) compared to control. In the dataset, these days were characterized by relatively higher irradiance than normal at the start, end, or immediate peak of the daylight period, and relatively lower irradiance than the sample mean immediately before or after the peak of the daylight period, but with no net change in average irradiance from the population mean. Hence, minimum and maximum irradiance β1 coefficients primarily describe changes in daily energy usage which depend on variations in sunlight distribution throughout the daylight period, being less appropriate for interpretation and with no strict correlation to cloud cover.
Energy usage was positively correlated to variance in irradiance over the same period for both control (β1 = 0.0320) and LBF (β1 = 0.0989), with the stronger correlation for LBF suggesting that LBF rooms cool poorly compared to control rooms under intermittently cloudy conditions and independently of maximum and minimum irradiance. This is unexpected given that if the LBF is absorptive and thus hotter, a more potent cooling effect under LBF could be expected during periods of inconsistent sunlight and associated atmospheric excitation. In such instances, heated glass should be cooled proportionately by higher outside fluid velocities under rain or wind (Mirsadeghi et al., 2013).
The higher coefficients for average and maximum outside temperatures in the case of control rooms indicate that the LBF may provide some insulation or thermal buffering effect, reducing the impact of conductive and convective heat transfer from outside air into rooms. Considering that the LBF has high absorptance compared to control floatglass, it is likely that the heating mechanism described here is not direct radiative transfer, but rather re-emission of absorbed light as heat from LBF interfaces to room air through convection and conduction (Ismail et al., 2008). Minimum outside temperature was a significant determinant of cooling in control rooms, but with a low magnitude (β1 = 0.0159), and not significant in LBF rooms. Maximum outside temperature was a significant determinant of cooling under both control (β1 = 0.1137) and LBF (β1 = 0.0737), but the difference between treatments was not significant given the lower selection rate in the energy savings model (p = 0.196).
Several interaction effects were found to be significant for differences in energy usage between rooms, but largely did not contribute to energy usage compared to main effects, as evidenced in Table 2. The interaction of maximum temperature * variance in irradiance describes a positive correlation (β1 = 0.0802) suggesting that periods with higher maximum temperatures alongside higher intermittent cloudiness observed improved energy savings under LBF. The interaction of minimum temperature * minimum irradiance appeared to decrease energy savings (β1 = −0.1124), suggesting that higher minimum temperatures accompanied by higher minimum irradiance increased energy usage under LBF relative to control.
Energy usage was not correlated with wind direction; it is hypothesized that this is because the LBF was strongly absorptive rather than reflective towards infrared, which determines much of the glass’s temperature (Palomar and Enríquez, 2022). The greenhouse compartments in this study were bounded by a temperature-controlled walkway (He et al., 2021) such that only roofs would have been exposed to outside winds, decreasing any potential correlations. LBF surfaces would have generally been warmer than control glass surfaces due to high absorbance, thus convective losses from LBF would have been higher given the similar U-factors (Ismail et al., 2008). Importantly, the external heat transfer coefficient of a glazing is typically dependent on the wind velocity close to the glazing and partially determines the inward-flowing fraction of absorbed radiation. The internal heat transfer coefficient, i.e., the heat transfer coefficient towards the inside, is commonly considered to be dependent on free convection rather than wind speed within a room (ASHRAE, 2021). This is a source of error in greenhouse applications, where the structures are well-ventilated by necessity.
For the multi-span greenhouse compartments in this study, activating a fan unit produced a strong horizontal air current, or axial free jet, across the room from the entrance to the back wall. This free jet, parallel to and approximately 2 m below the roof, produced a radial wall jet at the back wall. The correlation between radial velocity fluctuations and heat transfer peaks is not simple to extrapolate at the distances between fans and back walls presented in this study, though it is expected that there will be an increase in convective heat transfer within axial and radial wall jets (Kosutova et al., 2019; O'Donovan and Murray, 2007). The free jet is also expected to become more turbulent in the presence of a taller or denser crop, as in the case of denser capsicum crops when compared to eggplant crops, which may increase the effective size of the airstream and associated heat transfer from walls (Seidel et al., 2001; Naqavi et al., 2017). Hence, in the case where LBFs re-emit absorbed light as heat, convective heat transfer at LBF interfaces to the LBF room is likely to be much greater than observed in normal buildings, which may account for the discrepancies in observed and expected energy usage.
A weak, negative, but significant correlation between energy savings and wind speed was also found (β1 = −0.0718). However, wind speed was also not a chosen predictor of energy usage in either room for the individual room models described Table 2. The low correlation to wind direction indicated in Table 4 likely results from how walls between control, treatment, and walkway compartments were shared rather than distinct and separate. These thermal boundaries were not included as part of the building envelope and hence could not be affected by outside winds. Chavan et al. (2022) described significant crop- and season-dependent changes in transpiration for capsicum under control and LBF. This suggests that the magnitude of cooling by evapotranspiration was non-linear for this crop, and the relationship to wind speed, wind direction, and vent aperture is not simple. It has also been previously demonstrated that eggplant transpiration under LBF is hindered in a light-limited fashion (Chavan et al., 2020), suggesting that additional measurements of canopy transpiration over the season would be required to capture the cooling potential of evapotranspiration.
Ultimately, it was apparent that no other simulated climates described positive energy savings under LBF despite variation in multiple climate parameters (Table 3). Energy usage under LBF appeared less dependent on outside temperatures, and more dependent on irradiance compared to control. Furthermore, LBF used more energy usage on cooling despite being manufactured for a similar winter U-factor and lower solar heat gain coefficient. The weak correlation of energy savings to outside precipitation indicates that this greenhouse design, whereby only the roof LBF is exposed to outside wind, may not be ideal for generating energy savings. Thus, an enhanced cooling effect may be observed when LBF walls are included as part of the building envelope, and hence influenced by outside climate. This effect could be further improved with double-glazed glass whereby re-radiation of absorbed heat from the exterior LBF and from gas in the gap cavity may determine over half of the difference in heat transfer coefficients for exterior and interior glass panes (Ismail et al., 2008; Akhtar and Mullick, 2012). However, where such absorptive glasses are utilized in the interior greenhouse environment rather than the building envelope, reflective films may be a better option for cooling as more light can escape the building envelope or is reflected at this interface.
5 Conclusion
The climate-dependent reductions in yield under LBF demonstrate that absorptive glasses may impact crop performance across different capsicum cultivars by reducing fruit number, particularly when less than optimal light is provided. Furthermore, highly absorptive glasses may not be suitable cover materials for improving glasshouse energy savings given the possibility of increased heat retention under strongly ventilated conditions. In a greenhouse configuration where LBF is installed as part of the internal structure rather than as part of the building envelope, wind speed and intermittent cloud cover are much weaker factors of cooling compared to light intensity and temperature. The ideal situation of LBF and other absorptive glasses within landscape of protected cropping will benefit from a re-examination of which thermal processes should be exploited to reduce energy usage.
Data availability statement
The original contributions presented in the study are included in the article/Supplementary Material, further inquiries can be directed to the corresponding authors.
Author contributions
TL: Conceptualization, Data curation, Formal Analysis, Investigation, Methodology, Validation, Visualization, Writing–original draft, Writing–review and editing. CM: Formal Analysis, Investigation, Writing–original draft. WL: Investigation, Methodology, Writing–review and editing. NK: Investigation, Writing–review and editing. JH: Data curation, Formal Analysis, Writing–review and editing. DT: Funding acquisition, Project administration, Writing–review and editing. Y-CL: Funding acquisition, Supervision, Writing – review and editing. SS: Funding acquisition, Project administration, Supervision, Writing–review and editing. MG: Conceptualization, Funding acquisition, Project administration, Supervision, Writing–review and editing. Z-HC: Conceptualization, Funding acquisition, Project administration, Resources, Supervision, Writing–review and editing.
Funding
The author(s) declare financial support was received for the research, authorship, and/or publication of this article. This work was supported by Horticulture Innovation Australia (VG16070, VG17003, LP18000) to DT and Z-HC. TL is supported by a CSIRO and Western Sydney University (WSU) joint PhD scholarship and by the CRC Future Food Systems (P2-018). SS and MG were supported by CSIRO and Z-HC was funded by Australian Research Council (DE140101143; FT210100366).
Acknowledgments
The authors thank greenhouse technical assistants at the National Vegetable Protected Cropping Centre (NVPCC) Western Sydney University for their support in crop maintenance.
Conflict of interest
The authors declare that the research was conducted in the absence of any commercial or financial relationships that could be construed as a potential conflict of interest.
The author(s) declared that they were an editorial board member of Frontiers, at the time of submission. This had no impact on the peer review process and the final decision.
Publisher’s note
All claims expressed in this article are solely those of the authors and do not necessarily represent those of their affiliated organizations, or those of the publisher, the editors and the reviewers. Any product that may be evaluated in this article, or claim that may be made by its manufacturer, is not guaranteed or endorsed by the publisher.
Supplementary material
The Supplementary Material for this article can be found online at: https://www.frontiersin.org/articles/10.3389/fenrg.2024.1360536/full#supplementary-material
References
Abram, N. J., Henley, B. J., Sen Gupta, A., Lippmann, T. J., Clarke, H., Dowdy, A. J., et al. (2021). Connections of climate change and variability to large and extreme forest fires in southeast Australia. Commun. Earth Environ. 2 (1), 8. doi:10.1038/s43247-020-00065-8
Akhtar, N., and Mullick, S. C. (2012). Effect of absorption of solar radiation in glass-cover (s) on heat transfer coefficients in upward heat flow in single and double glazed flat-plate collectors. Int. J. Heat Mass Transf. 55 (1-3), 125–132. doi:10.1016/j.ijheatmasstransfer.2011.08.048
Anderson, R., Bayer, P. E., and Edwards, D. (2020). Climate change and the need for agricultural adaptation. Curr. Opin. Plant Biol. 56, 197–202. doi:10.1016/j.pbi.2019.12.006
Anifantis, A. S., Colantoni, A., and Pascuzzi, S. (2017). Thermal energy assessment of a small scale photovoltaic, hydrogen and geothermal stand-alone system for greenhouse heating. Renew. Energy 103, 115–127. doi:10.1016/j.renene.2016.11.031
Asgharipour, M. R., Amiri, Z., and Campbell, D. E. (2020). Evaluation of the sustainability of four greenhouse vegetable production ecosystems based on an analysis of emergy and social characteristics. Ecol. Model. 424, 109021. doi:10.1016/j.ecolmodel.2020.109021
Baddadi, S., Bouadila, S., Ghorbel, W., and Guizani, A. (2019). Autonomous greenhouse microclimate through hydroponic design and refurbished thermal energy by phase change material. J. Clean. Prod. 211, 360–379. doi:10.1016/j.jclepro.2018.11.192
Barbosa, G., Gadelha, F., Kublik, N., Proctor, A., Reichelm, L., Weissinger, E., et al. (2015). Comparison of land, water, and energy requirements of lettuce grown using hydroponic vs. Conventional agricultural methods, Int. J. Environ. Res. Public Health 12 (6), 6879–6891. doi:10.3390/ijerph120606879
Benke, K., and Tomkins, B. (2017). Future food-production systems: vertical farming and controlled-environment agriculture. Sustain. Sci. Pract. Policy 13 (1), 13–26. doi:10.1080/15487733.2017.1394054
Bureau of Meteorology (2024). Daily rainfall - Richmond UWS Hawkesbury. http://www.bom.gov.au/jsp/ncc/cdio/weatherData/av?p_nccObsCode=136&p_display_type=dailyDataFile&p_startYear=2019&p_c=−898362055&p_stn_num=067021 (February 9, 2024).
Casierra-Posada, F., Matallana-Díaz, Y. A., and Zapata-Casierra, E. (2014). Growth of bell pepper plants (Capsicum annuum) affected by coloured covers. Gesunde Pflanz. 66 (4), 149–155. doi:10.1007/s10343-014-0328-7
Chaiyapinunt, S., Phueakphongsuriya, B., Mongkornsaksit, K., and Khomporn, N. (2005). Performance rating of glass windows and glass windows with films in aspect of thermal comfort and heat transmission. Energy Build. 37 (7), 725–738. doi:10.1016/j.enbuild.2004.10.008
Chavan, S. G., Chen, Z.-H., Ghannoum, O., Cazzonelli, C. I., and Tissue, D. T. (2022). Current technologies and target crops: a review on Australian protected cropping. Crops 2 (2), 172–185. doi:10.3390/crops2020013
Chavan, S. G., Maier, C., Alagoz, Y., Filipe, J. C., Warren, C. R., Lin, H., et al. (2020). Light-limited photosynthesis under energy-saving film decreases eggplant yield. Food Energy Secur. 9 (4), SE45. doi:10.1002/fes3.245
Clercq, M. D., Vats, A., and Biel, A. (2018). Agriculture 4.0: the future of farming technology,” in Proceedings of the World Government Summit, World Government Summit, Dubai, UAE, February 2, 2018. https://www.bollettinoadapt.it/wp-content/uploads/2019/12/OliverWyman-Report_English-LOW.pdf
Cossu, M., Yano, A., Solinas, S., Deligios, P. A., Tiloca, M. T., Cossu, A., et al. (2020). Agricultural sustainability estimation of the European photovoltaic greenhouses. Eur. J. Agron. 118, 126074. doi:10.1016/j.eja.2020.126074
Darrall, N. M. (1989). The effect of air pollutants on physiological processes in plants. Plant, Cell and Environ. 12 (1), 1–30. doi:10.1111/j.1365-3040.1989.tb01913.x
Data (2022). indicator. Retrieved September 14, 2022, from https://data.worldbank.org/indicator/AG.LND.ARBL.HA.PC?end=2018&start=1961.
Davies, S. J., and Unam, L. (1999). Smoke-haze from the 1997 Indonesian forest fires: effects on pollution levels, local climate, atmospheric CO2 concentrations, and tree photosynthesis. For. Ecol. Manag. 124 (2), 137–144. doi:10.1016/S0378-1127(99)00060-2
Díaz-Pérez, J. C. (2014). Bell pepper (Capsicum annum L.) crop as affected by shade level: fruit yield, quality, and postharvest attributes, and incidence of phytophthora blight (caused by Phytophthora capsici Leon.). HortScience 49 (7), 891–900. doi:10.21273/HORTSCI.49.7.891
Emmott, C. J. M., Röhr, J. A., Campoy-Quiles, M., Kirchartz, T., Urbina, A., Ekins-Daukes, N. J., et al. (2015). Organic photovoltaic greenhouses: a unique application for semi-transparent PV? Energy and Environ. Sci. 8 (4), 1317–1328. doi:10.1039/C4EE03132F
Fu, Q. S., Zhao, B., Wang, Y. J., Ren, S., and Guo, Y. D. (2010). Stomatal development and associated photosynthetic performance of capsicum in response to differential light availabilities. Photosynthetica 48, 189–198. doi:10.1007/s11099-010-0024-5
Gorjian, S., Calise, F., Kant, K., Ahamed, M. S., Copertaro, B., Najafi, G., et al. (2021). A review on opportunities for implementation of solar energy technologies in agricultural greenhouses. J. Clean. Prod. 285, 124807. doi:10.1016/j.jclepro.2020.124807
Hadley, D. D. (2017). Controlled environment horticulture industry potential in NSW. Armidale, Australia: University of New England.
He, X., Chavan, S. G., Hamoui, Z., Maier, C., Ghannoum, O., Chen, Z.-H., et al. (2022). Smart glass film reduced ascorbic acid in red and orange capsicum fruit cultivars without impacting shelf life. Plants Basel, Switz. 11 (7), 985. doi:10.3390/plants11070985
He, X., Maier, C., Chavan, S. G., Zhao, C. C., Alagoz, Y., Cazzonelli, C., et al. (2021). Light-altering cover materials and sustainable greenhouse production of vegetables: a review. Plant Growth Regul. 95 (1), 1–17. doi:10.1007/s10725-021-00723-7
Herman, B. M., and Browning, S. R. (1975). The effect of aerosols on the earth-atmosphere albedo. J. Atmos. Sci. 32 (7), 1430–1445. doi:10.1175/1520-0469(1975)032<1430:TEOAOT>2.0.CO;2
Hüls, A., Ickstadt, K., Schikowski, T., and Krämer, U. (2017). Detection of gene-environment interactions in the presence of linkage disequilibrium and noise by using genetic risk scores with internal weights from elastic net regression. BMC Genet. 18 (1), 55–14. doi:10.1186/s12863-017-0519-1
Ilić, Z. S., Milenković, L., Šunić, L., Barać, S., Mastilović, J., Kevrešan, Ž., et al. (2017). Effect of shading by coloured nets on yield and fruit quality of sweet pepper. Zemdirbyste-Agriculture 104 (1), 53–62. doi:10.13080/Z-A.2017.104.008
Ismail, K. A., Salinas, C. T., and Henriquez, J. R. (2008). Comparison between PCM filled glass windows and absorbing gas filled windows. Energy Build. 40 (5), 710–719. doi:10.1016/j.enbuild.2007.05.005
Kabir, M. Y., Díaz-Pérez, J. C., and Nambeesan, S. U. (2020). Effect of shade levels on plant growth, physiology, and fruit yield in bell pepper (Capsicum annuum L.). Acta Hortic. 1268, 311–318. doi:10.17660/ActaHortic.2020.1268.42
Kittas, C., Bartzanas, Th., and Jaffrin, A. (2000). Greenhouse evaporative cooling: measurement and data analysis. Acta Hortic. 534, 67–74. doi:10.17660/ActaHortic.2000.534.6
Kosutova, K., Vanderwel, C., van Hooff, T., Blocken, B., and Hensen, J. (2019). “Analysis of convective heat transfer coefficient correlations for ventilative cooling based on reduced-scale measurements,” in Proceedings of 40th AIVC conference, Air Infiltration and Ventilation Centre, Ghent, Belgium, October, 2019.
Kraft, K. H., Brown, C. H., Nabhan, G. P., Luedeling, E., Luna Ruiz, J. de J., Coppens d’Eeckenbrugge, G., et al. (2014). Multiple lines of evidence for the origin of domesticated chili pepper, Capsicum annuum, in Mexico. Proc. Natl. Acad. Sci. 111 (17), 6165–6170. doi:10.1073/pnas.1308933111
Krstajic, D., Buturovic, L. J., Leahy, D. E., and Thomas, S. (2014). Cross-validation pitfalls when selecting and assessing regression and classification models. J. cheminformatics 6, 10–15. doi:10.1186/1758-2946-6-10
Lin, T., Goldsworthy, M., Chavan, S., Liang, W., Maier, C., Ghannoum, O., et al. (2022). A novel cover material improves cooling energy and fertigation efficiency for glasshouse eggplant production. Energy 251, 123871. doi:10.1016/j.energy.2022.123871
Loik, M. E., Carter, S. A., Alers, G., Wade, C. E., Shugar, D., Corrado, C., et al. (2017). Wavelength-selective solar photovoltaic systems: powering greenhouses for plant growth at the food-energy-water nexus. Earth’s Future 5 (10), 1044–1053. doi:10.1002/2016EF000531
Maier, C. R., Chen, Z.-H., Cazzonelli, C. I., Tissue, D. T., and Ghannoum, O. (2022). Precise phenotyping for improved crop quality and management in protected cropping: a review. Crops 2 (4), 336–350. doi:10.3390/crops2040024
Meyer, R. S., Karol, K. G., Little, D. P., Nee, M. H., and Litt, A. (2012). Phylogeographic relationships among Asian eggplants and new perspectives on eggplant domestication. Mol. Phylogenetics Evol. 63 (3), 685–701. doi:10.1016/j.ympev.2012.02.006
Mongkon, S., Thepa, S., Namprakai, P., and Pratinthong, N. (2014). Cooling performance assessment of horizontal earth tube system and effect on planting in tropical greenhouse. Energy Convers. Manag. 78, 225–236. doi:10.1016/j.enconman.2013.10.076
Montagu, K. (2018). Protected Cropping – review of research and identification of R&D gaps for levied vegetables. HortInnovation Australia. https://www.horticulture.com.au/globalassets/laserfiche/assets/project-reports/vg16083/vg16083---final-report-complete.pdf.
Morgan, L. (2021). Hydroponics and protected cultivation: a practical guide. Wallingford, United Kingdom: CAB International.
Najjar, A., and Hasan, A. (2008). Modeling of greenhouse with PCM energy storage. Energy Convers. Manag. 49 (11), 3338–3342. doi:10.1016/j.enconman.2008.04.015
Naqavi, I. Z., Tyacke, J. C., and Tucker, P. G. (2017). A numerical study of a plane wall jet with heat transfer. Int. J. Heat Fluid Flow 63, 99–107. doi:10.1016/j.ijheatfluidflow.2016.07.012
O’Carrigan, A., Hinde, E., Lu, N., Xu, X. Q., Duan, H., Huang, G., et al. (2014). Effects of light irradiance on stomatal regulation and growth of tomato. Environ. Exp. Bot. 98, 65–73. doi:10.1016/j.envexpbot.2013.10.007
O’Donovan, T. S., and Murray, D. B. (2007). Jet impingement heat transfer–Part I: mean and root-mean-square heat transfer and velocity distributions. Int. J. heat mass Transf. 50 (17-18), 3291–3301. doi:10.1016/j.ijheatmasstransfer.2007.01.044
Palomar, T., and Enríquez, E. (2022). Evaluation of the interaction of solar radiation with colored glasses and its thermal behavior. J. Non-Crystalline Solids 579, 121376. doi:10.1016/j.jnoncrysol.2021.121376
Pichler, M., and Hartig, F. (2023). Can predictive models be used for causal inference? https://arxiv.org/abs/2306.10551.
Rabbi, B., Chen, Z.-H., and Sethuvenkatraman, S. (2019). Protected cropping in warm climates: a review of humidity control and cooling methods. Energies 12 (14), 2737. doi:10.3390/en12142737
Samaranayake, P., Liang, W., Chen, Z. H., Tissue, D., and Lan, Y. C. (2020). Sustainable protected cropping: a case study of seasonal impacts on greenhouse energy consumption during capsicum production. Energies 13 (17), 4468. doi:10.3390/en13174468
Samaranayake, P., Maier, C., Chavan, S., Liang, W., Chen, Z. H., Tissue, D. T., et al. (2021). Energy minimisation in a protected cropping facility using multi-temperature acquisition points and control of ventilation settings. Energies 14 (19), 6014. doi:10.3390/en14196014
Seidel, J., and Fasel, H. F. (2001). Numerical investigations of heat transfer mechanisms in the forced laminar wall jet. J. Fluid Mech. 442, 191–215. doi:10.1017/S0022112001005079
Smith, G. (2011). Overview of the Australian protected cropping industry. https://www.graemesmithconsulting.com/.
Soussi, M., Chaibi, M. T., Buchholz, M., and Saghrouni, Z. (2022). Comprehensive review on climate control and cooling systems in greenhouses under hot and arid conditions. Agronomy 12 (3), 626. doi:10.3390/agronomy12030626
United Nations Department of Economic and Social Affairs, Population Division (2022). World population prospects 2022: summary of results. https://www.un.org/development/desa/pd/content/World-Population-Prospects-2022.
Keywords: protected cropping, energy modelling, sustainable crop production, greenhouse technology, Capsicum annuum L.
Citation: Lin T, Maier CR, Liang W, Klause N, He J, Tissue DT, Lan Y-C, Sethuvenkatraman S, Goldsworthy M and Chen Z-H (2024) A light-blocking greenhouse film differentially impacts climate control energy use and capsicum production. Front. Energy Res. 12:1360536. doi: 10.3389/fenrg.2024.1360536
Received: 05 January 2024; Accepted: 19 February 2024;
Published: 07 March 2024.
Edited by:
Seung Jin Oh, Korea Institute of Industrial Technology, Republic of KoreaReviewed by:
Jesus Alejandro Franco Piña, Universidad Nacional Autónoma de México, MexicoChiara Burattini, Unitelma Sapienza University, Italy
Copyright © 2024 Lin, Maier, Liang, Klause, He, Tissue, Lan, Sethuvenkatraman, Goldsworthy and Chen. This is an open-access article distributed under the terms of the Creative Commons Attribution License (CC BY). The use, distribution or reproduction in other forums is permitted, provided the original author(s) and the copyright owner(s) are credited and that the original publication in this journal is cited, in accordance with accepted academic practice. No use, distribution or reproduction is permitted which does not comply with these terms.
*Correspondence: Zhong-Hua Chen, ei5jaGVuQHdlc3Rlcm5zeWRuZXkuZWR1LmF1; Mark Goldsworthy, bWFyay5nb2xkc3dvcnRoeUBjc2lyby5hdQ==
†These authors share senior authorship