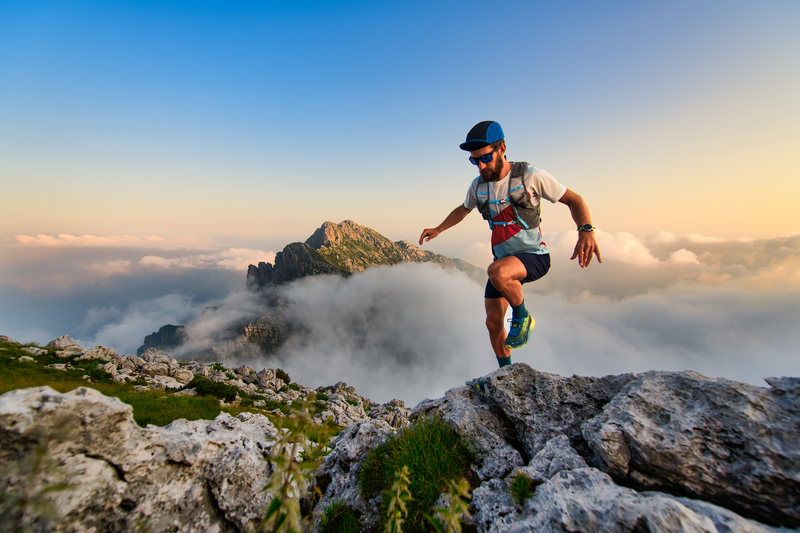
95% of researchers rate our articles as excellent or good
Learn more about the work of our research integrity team to safeguard the quality of each article we publish.
Find out more
ORIGINAL RESEARCH article
Front. Energy Res. , 29 April 2024
Sec. Wind Energy
Volume 12 - 2024 | https://doi.org/10.3389/fenrg.2024.1353388
Introduction: The electricity generated from nuclear plants and petroleum-based products has a negative influence on the environment as a whole. It has shown the utility to search out and promote the utilization of renewable, environmentally friendly, and sustainable energy sources such as solar, wind, and geothermal. Nowadays, Wind energy resource has quickly emerged as the world’s fastest-growing energy source.
Methods: However, the selection of the most suitable places for developing a wind farm is a crucial challenge that can be seen as a problem of site selection, which involves numerous conflicting variables. Therefore, it is classified as an MCDM (multi-criteria decision-making) problem. The main objective of this research is to determine the best locations in Burundi for the installation of wind farms. The Fuzzy Analytic Hierarchy Process (FAHP) was used to weigh the criteria considering their relative importance. This study considers several key factors when determining the optimal location for a wind farm. These factors include wind speed, slope, proximity to the grid network, distance to roads, and land use/land cover (LULC). Furthermore, a geographic information system (GIS) is utilized to generate the final suitability wind farm locations map.
Results and Discussion: The obtained results indicate that 20.91% of the whole study area is suitable nevertheless, only 1.96% is tremendously suitable for wind turbine placement. The western part of Burundi is the optimal area for constructing a wind farm, and the most is in Lake Tanganyika.
Energy is a major factor in any country’s sustainable development and prosperity. However, the growing trend in energy demand is followed by an increase in the global use of fossil fuels, which results in significant climate, environmental, and health costs. (IEA, 2021; Mohammadzadeh et al., 2018). With this rapid increase in energy demand, the development of technologies for harvesting renewable energy has also accelerated. Wind energy, as stated by the Global Wind Energy Council (GWEC), is a renewable energy source that has experienced remarkable and rapid development. The global wind power capacity reached 743 GW by the end of 2020, with an addition of 93 GW in the same year. By 2021, it surpassed 840 GW, and by 2022, it reached 906 GW with 9% growth (Rajendran et al., 2022; Global Wind Energy Council, 2023).
Wind energy represents one of the most promising, mature, and rapidly expanding renewable energy sources (Saraswat et al., 2021; Shahid et al., 2019). Its fastest growth is explained by the fact that it is considered a clean, reliable, and renewable energy resource that positively contributes to minimizing the issue of worldwide concerns about climate change and energy sources (Diffendorfer et al., 2021). Furthermore, the utilization of wind energy not only generates employment opportunities but also contributes to the reduction of CO2 emissions. According to research, wind energy use is anticipated to increase in Canada, Sweden, China, and Germany by 2025 (Sadorsky, 2021). This clean and affordable energy source not only appeals to investors but also provides a practical solution for reducing dependency on fossil fuels. Studies have demonstrated that combining wind turbines with other energy sources can effectively decrease carbon emissions (Yousefi et al., 2022).
Moreover, wind resources are intrinsically unpredictable because of their dependency on geographic and climatic factors as well as the time of the region under consideration. This results in different values from one place to another for daily and seasonal changes. Therefore, there is a clear need for an effective assessment of suitable placements for the installation of wind turbines to maximize wind energy production while minimizing the effects of different challenges (Wu et al., 2018; Damousis et al., 2004).
At a given scale, wind energy is generated by installing wind farms in the windy areas. Selecting the right type of turbine is vital for establishing a sustainable wind farm. It must be able to seamlessly adjust to the diverse topography, climate, and time conditions of the site. The process of making a choice is often influenced by various factors, where multiple decision criteria conflict with each other (Rediske et al., 2021; Seyed et al., 2022; Badi et al., 2023). These factors include the following key technical requirements (Yousefi et al., 2022):
- Sustained wind speed that is adequate for generating substantial power;
- Sufficient area to accommodate wind turbines and related infrastructure;
- Ground conditions that are suitable for constructing and operating the wind farm;
- Access to the electricity grid, enabling the distribution of the generated power;
- Feasibility of providing access for oversized loads that may be required during construction or maintenance;
- Terrain and topography suitable for optimal wind turbine placement and operation.
Several strategies can be employed to effectively tackle the challenges of selecting turbines considering their multi-criteria nature. They fall under the domain of multi-criteria decision-making (MCDM). MCDM is an operation sub-discipline that assesses several operational competing factors in decision-making.
MCDM methods are used to handle decision problems involving multiple factors in the decision-making process. These criteria conflict with each other and are mutually in-commensurable. Incommensurability refers to a scenario where the decision criteria have different units and magnitudes. Numerous MCDMs have been developed by researchers and each has advantages as well as disadvantages; therefore, the choice of which one to use is also an important decision. Common techniques include the weighted sum method, PROMETHEE, TOPSIS, VIKOR, ELECTRE, goal programming, AHP, Grey relation analysis, minimum manhattan distance (MMD) approach and Fuzzy logic, among others. (Mardani et al., 2015; Gr, 2020; Łaska, 2020; Indrajayanthan and Mohanty, 2022).
Numerous research studies have been conducted worldwide to identify the best areas for wind power facilities, using various MCDM methods. To determine the most effective approach for this study, previous works on the subject are thoroughly examined. Table 1 provides a comprehensive overview of prior research on wind farm site selection, prominently showcasing the MCDM-FAHP method as the premier decision-making tool for identifying the best location. The MCDM-FAHP method has not only been widely used for selecting wind farm sites but has also demonstrated its usefulness in other domains like solar farms, urban planning and resource allocation (Seyed et al., 2022; Barakat et al., 2019; Li et al., 2022). What sets it apart from other methods is its capacity to handle intricate decision-making situations and yield dependable outcomes. Therefore, it can be confirmed that the FAHP method rep-resents unrivaled effectiveness and efficiency, making it the ideal choice for implementation in the present study.
MCDM methods have a significant limitation in their applicability, particularly when it comes to decision-making based on both ‘AND’ and ‘OR’ operations between criteria. In such situations, these approaches become ineffective. However, the Fuzzy logic theory provides a viable solution as it can handle decision rules involving ‘AND’ or ‘OR’ operations and effectively manage complex scenarios that combine ‘AND-OR’ type rules. This is why the implementation of Fuzzy logic is highly advantageous in developing and executing multiple decision criteria for wind farm site selection.
The fuzzy logic tool is based on Zadeh’s fuzzy set theory, which he proposed in 1965 (Zimmermann, 2010). The fuzzy set theory offers a framework in which several conceptual phenomena can be precisely and rigorously analyzed. Furthermore, it can also be considered as a modelling language, fitting for cases in which fuzzy relations, criteria, and phenomena exist. A fuzzy logic tool is an essential tool in risk assessment in the way it compensates for the lack of knowledge and ambiguity faced when assessing the risks related to complex technological systems. It is also more useful when working with fuzzy linguistic concepts like low, medium, high, etc. (Gr, 2020; Nguyen et al., 2022).
The main steps in the fuzzy-logic method procedure are depicted in Figure 1(Badran et al., 2011; Abdelmassih et al., 2021; Mustafa and Barabadi, 2022):
- Rule base: it contains all the rules and conditions defined by experts to control the decision-making system;
- Fuzzification process: in this step, the input variable values are provided by experts. It permits to convert crisp inputs into fuzzy sets that correspond to the intuitive perception of the system’s status;
- Inference engine: this component helps to determine the degree of match between fuzzy inputs and the rules. It is a main step because all information is treated in the inference engine. Based on the percentage match, it determines what control to perform in response to the different combinations of the input variables;
Defuzzification: this process is performed to convert significant fuzzy outputs into specific output variables.
The economic viability of a wind project greatly depends on identifying the most suitable areas for wind farm development. There is a need to find a systematic approach to determine where these potential locations exist as well as the cost of power production. With this objective, a fuzzy GIS-MCDM-based approach is recommended as the best tool for wind farm sitting based on prior research (Li et al., 2020; Cavazzi and Dutton, 2016; Villacreses et al., 2017; Ayodele et al., 2018; Kaya et al., 2019; Aliyev et al., 2020).
The F-AHP process is a combination of fuzzy logic theory and the classical AHP process. The analytic hierarchy process (AHP) provides a precise technique for determining the importance of different decision criteria. It is a highly systematic approach to evaluating complex judgments, allowing for effective organization and analysis of multiple factors. This process involves the construction of a pairwise matrix to assess the relative importance of decision criteria. The matrix is further normalized to establish the relative significance of each criterion, a vital component in the decision-making process. By integrating fuzzy theory, the AHP method effectively tackles scenarios where criterion weighting entails partial truth or uncertainty (Baalousha et al., 2023).
The FAHP method was selected for this study for site-selection problems due to several compelling reasons. Firstly, it is a highly regarded and widely used method known for its high computational efficiency and practicality. Moreover, it seamlessly integrates with GIS, a widely used tool for land analysis and site selection. Another advantage is its ability to conduct hierarchical modelling, enabling the incorporation of subjective judgments and consistency verification. Additionally, the FAHP method takes into consideration both quantitative and qualitative criteria, offering a comprehensive approach to problem interpretation. With the capability to perform various sensitivity analyses, FAHP provides flexibility in handling different criteria. Furthermore, it streamlines the decision-making process by facilitating pairwise comparisons among the criteria. Lastly, the method takes into account the consistency and inconsistency of alternatives, making it an invaluable tool (Mosadeghi et al., 2015; Perkin et al., 2015; Rehman and Khan, 2016; Sedaghat et al., 2019; Yunia and Rahmawati, 2020; Pang et al., 2021; Sánchez-Lozano et al., 2021).
In Burundi, the exploration of wind resource assessment remains relatively unmapped. This particular topic has been underexplored in previous studies, making it a significant gap that needs to be addressed. Although limited studies have been conducted in Burundi, including one conducted by (Bashahu et al., 2022), which examined 1 year of hourly wind speed data from two stations. This research offers invaluable insights for strategically organizing and executing wind energy conversion systems (WECS) in these areas. Furthermore, another study, (Bashahu and Buseke, 2016), performed a statistical analysis on a 4-year span of hourly wind speed data from four additional Burundian stations. Hence, it is of utmost importance to conduct further research on this matter to obtain general information on Burundi wind resource potential and its distribution throughout the territory. Unleashing the power of wind energy will revolutionize Burundi’s energy landscape, providing a sustainable solution to the country’s energy scarcity.
Furthermore, no research has focused on the selection of optimal wind farm placements and their classification. This will help decision-makers to know which type of wind farms fit well in Burundi and at which scale they can contribute to future projects.
The main objective of this research is to assess the feasibility of establishing wind farms in various locations across Burundi. The study utilized the MCDM methodology, focusing on the fuzzy-AHP technique, to identify the optimal locations for wind farm projects using GIS software. Different criteria are taken into account to identify the best locations. These include factors like wind speed, slope, aspect, distance from transmission lines and power grids, distance from protected areas, distance from airports, elevation, and distance from major roads.
Finally, this study’s main contributions and originality may be stated as follows: A cutting-edge wind farm suitability mapping method based on Fuzzy Analytic Hierarchy Process (FAHP) and Geographic Information System (GIS) methodologies is presented. This innovative approach takes into consideration technical, environmental, social, and spatial factors in the Burundi’s territory. This research investigation is the first of its kind to identify the optimal locations for constructing wind power plants (WPPs) in Burundi.
The remainder of this paper is divided into the following sections: the second section is the research background, the third section describes the methodology used to solve the considered problem; the fourth section provides a comprehensive description, analysis, and discussion of both the GIS and the proposed decision problem; and the fifth section presents the results and engages in a thorough discussion of these findings. Finally, the sixth section succinctly outlines the main conclusions of this study.
Before development, manufacture, installation, commissioning and operation there is a need to process site selection at an early stage to assess the feasibility of wind farms in light of environmental concerns, economic feasibility, and other criteria. This study’s methodology framework was developed in three key stages:
(a) The FAHP-MCDM approach was utilized in the initial stage to establish and prioritize the criteria for wind farm site suitability. A comprehensive evaluation of restriction and exclusion factors was conducted, leading to the definition of specific criteria for wind farms. These criteria were then ranked according to their level of importance.
(b) To advance to the next stage, we conducted a comprehensive GIS analysis in order to identify the necessary GIS operations. This enabled the creation of classified digital map layers for each criterion, setting a solid foundation for the subsequent analysis.
(c) In the final stage, wind farm suitability map was generated to identify the most suitable locations for wind farm development. Wind data collected over a 10-year period from several meteorological stations owned by the Geographical Institute of Burundi (IGE BU) was used to create an extensive wind map of the country of Burundi. Data from the World Bank and REGIDESO are used to create digital map layers for GIS software. These sources provided Burundi’s main roads and electrical network maps (Devex, 2013).
The main focus of this study lies on Burundi, Figure 2, an East African country that is entirely landlocked. Burundi shares borders with Tanzania to the south and east, the Democratic Republic of Congo to the west, and Rwanda to the north. Its precise geographical coordinates are between 2° 15′– 4° 30′S and 28° 58′– 30° 53′E. Burundi is 27,834 square kilometers and is located in two major watersheds: the Nile basin (
GIS-MCDM combination allows evaluation of the criteria and their factors by the mean of characteristics within a specific range of decision rules and assessment. The technique is commonly used to select ideal site processes in a huge variety of scientific fields (Gr, 2020). Lots of the problems people have in suitable site selection are geographic and, thus, coupling GIS with the MCDM method has the potential to solve such complex decision-making problems (Saraswat et al., 2021; Li et al., 2020). Concerning optimal wind farm site selection, the GIS-MCD methods have been standardized to effectively search for and select the best wind sites (Yousefi et al., 2022).
The main steps followed by the GIS-MCDM technique are (Ali et al., 2017):
• Problem definition. Try to define and understand the problem by fixing the goal or objective as clearly as possible;
• Criteria and limitations determination through a combination of experts’ opinions and information from various sources;
• Transform values onto a relative scale to allow comparison between each of the criteria;
• Weight assignment to criteria in regards to the fixed objective and respect for each other;
• Combine, synthesize and aggregate criteria together;
• Analyze and then approve results.
After the criteria determination, their hierarchy must be defined to calculate corresponding weights. All criteria are converted into standard values and combined using the weighted linear combination method, a widely used method (Balogun et al., 2017) that is based on the factors’ weighted aggregation, to finally generate a suitability map.
The optimal wind farm site selection problem depends on several criteria that include attributes and objectives. They can differ from the different areas and should have some properties to adequately represent the multi-criteria. Criteria must also be comprehensive and measurable (Yalcin et al., 2017; Dhunny et al., 2019). Consequently, to assess well the criteria, it is important to identify such relevant factors affecting the optimal wind farm site selection typically including topographic, geographic, climatic, socio-environmental, economic, location and political aspects.
For this study, the choice of evaluation criteria is based on previous various studies and is categorized into two main categories, restriction and exclusion criteria (See Table 3). The exclusion criteria comprise legally protected areas such as natural reserves, seaports, airports, and tourist sites. The areas dropping under this category are rejected and consequently not eligible for wind farm sites. Conversely, restriction criteria define a distance to be respected from the selected zone for the implementation of wind farms. The distance can be determined in terms of a buffer zone inside which the wind farm cannot be constructed. Some examples of those zones are found around settlements, water bodies, natural parks, airports, and aircraft landing corridors, among others (Refer to Figure 3 for this study).
The construction of wind energy facilities must consider that the selected location is not subject to any legal restrictions but also has a set of factors influencing the decision. The choice of those factors is primarily dictated by the study area. Therefore, based on studies so far done, this study classified these factors into three general groups (geographical factors, climatological factors, and geographical location factors) (Xu et al., 2020; Sánchez-Lozano et al., 2014; Aksoy and San, 2019; Taoufik and Fekri, 2021). The factors are divided into a set of criteria that impact the selection of the wind turbine location and will make one site preferable rather than another (Caceoğlu et al., 2022; Shafiee, 2022).
The local wind speed (intensity, direction, consistency, and uniformity) is the most important factor which plays a key role in wind farm project performance. A wind turbine’s energy generation is directly proportional to the cube of the wind speed, and it is given by Eq. 1:
where
Land slope influences the cost involved in wind farm implementation due to the difficulties in access and groundwork on sloppy lands. In many studies, reference is taken in the range of
Wind resource varies with altitude and is better at high altitudes but decreases at very high altitudes due to air density decrease. Elevation above 2000 m, is sometimes considered the cut-off altitude (Noorollahi Y. et al., 2016). Hence, ground elevation is also considered a factor in the choice of the best wind farm location. Therefore, flat terrain will be favourable for wind farm project construction, operation, and maintenance (Hoang et al., 2022).
The better the aspect of the wind farm’s location, the more straightforward it is to erect. As a result, the slope at the location of the wind farm should be oriented toward the prevailing wind blow (Villacreses et al., 2017; Feng, 2021). In terms of wind potential, the slope orientation concerning wind direction is one of the crucial factors (Pamucar et al., 2017).
It is recommended to install wind farms at a distance from airports/seaports and similar facilities. It is highly recommended to install wind farms in locations that are at a considerable distance from airports and seaports (Sánchez-Lozano et al., 2014).
Being close to the main road network is crucial in reducing transportation costs and facilitating material transport for wind turbine implementation (Hoang et al., 2022). The distance from the main roads is a significant economic factor, and therefore, the best location for a wind farm should be one that is near existing roads, rather than building new ones to access the site (Pamucar et al., 2017).
Since wind energy must be connected to the grid, the proximity to the national grid affects the cost of a wind farm. Increasing the distance between the wind farm and the electrical grid will inevitably lead to higher transmission costs (Alhuyi et al., 2020; Rehman and Khan, 2016). Hence, the power transport to consumers using the existing power transmission lines hugely reduces the project cost. Figure 4 illustrates a variety of maps that depict the restriction factors that affect the process of selecting suitable sites for wind farms. The Burundi maps of high voltage electric lines and national roads were obtained from the official reports published in Burundi country and are digitalized using ArcGIS software (Balogun et al., 2017; Yalcin et al., 2017; Dhunny et al., 2019).
Figure 4. Restriction factors that influence the selection of wind farms in Burundi (DIVA-GIS, 2023; logcluster, 2022; ENERGYDATA, 2007; Dreamstime, 2023).
The wind speed map is the outcome of the ArcGIS process obtained using weather research and forecasting model (WRF) output simulations. Wind speed data collected at a height of 12 m above the ground level was used for a wind potential simulation using the WRF model. However, modern wind turbines have hub heights ranging from 25 to over 100 m. Therefore, the WRF wind speed outcome was extrapolated to 60 m above ground level for a more comprehensive analysis (Baalousha et al., 2023; Van Sark et al., 2019; Benti et al., 2023). The slope, aspect and elevation map factors for this work were obtained using ArcGIS software and digital elevation model (DEM) data. The DEM is downloaded from the ‘U.S. Geological Survey (USGS) EarthExplorer tool’ (US Geological Survey, 2019).
The AHP methodology, first established by Saat in 1980 (Cavazzi and Dutton, 2016), is widely acknowledged as a robust and flexible MCDM method for dealing with complex decision problems (Sánchez-Lozano et al., 2016). The decision maker can utilize it to effectively analyze complex decision problems that involve various criteria and obtain accurate results. The AHP enables a more comprehensive examination of the intricate facets of objective aspects related to a problem. Furthermore, it helps ensure the accuracy and constancy of their evaluation, thereby minimizing potential human errors during the decision-making procedure.
The AHP process through three main stages to get the final decision (Yousefi et al., 2022; Zalhaf et al., 2022):
• Develop complex problems in a hierarchy of goals, alternatives, and criteria to assess those alternatives;
• Comparison of alternatives at each level of the hierarchy, one criterion at a time;
• At last, generate a vertically oriented decision-making matrix that spans across the multiple levels of the hierarchy.
Despite its strengths, AHP does have some weaknesses. For instance, there is a mutual dependency among alternatives, which can occasionally result in relatively less accurate outcomes. Additionally, when multiple decision-makers with different perspectives are involved, defining criterion weights can become more complex. Furthermore, AHP relies on opinions and experience-based data collection, which may introduce some limitations. The main problem encountered when utilizing the AHP lies in the presence of uncertain, biased, and vague opinions expressed by experts (Gr, 2020; Sánchez-Lozano et al., 2021; Zalhaf et al., 2022).
The classical AHP method was extended to the fuzzy sets theory and the fuzzy AHP (FAHP) has been developed to handle complex decision problems accurately and more systematically than the simple AHP scheme. This technique integrates the fuzzy set theory concept with basic AHP. It displays the elaboration of a standard AHP into a fuzzy domain by applying fuzzy numbers rather than real numbers (Ali et al., 2017).
The FAHP application process is summarized below (Havle and Kılıç, 2019; Özdemir et al., 2018):
Step 1: This step consists of defining a fuzzy pairwise comparison matrix. This matrix consists of the main criteria that may have an impact on the problem under investigation. Diagonal matrices are built using the hierarchical structure’s sub-criteria.
Decision-makers utilize fuzzy linguistic terms to assign the significance of one criterion over another. To achieve this, the decision-makers refer to Table 2 (In Table 2, TFN stands for Triangular Fuzzy Number). The conversion scale displayed illustrates the nine-point scale utilized in converting responses into fuzzy numbers. The resulting pairwise comparison matrix is given by Eq. 2:
Table 2. The nine-point fuzzy conversion scale (Tripathi et al., 2022).
Where
Step 2: The comprehensive assessment is generated by combining the pairwise comparison matrices in this stage. This investigation employs fuzzy triangular numbers to calculate the judgment matrix. The results of this calculation can be found in Table 3. To accurately calculate the TFNs, Excel software has been employed. Each triple value represents the preference score of the considered criterion relative to other criteria as defined in Table 2.
Land use and land cover (LULC) refers to the standard restriction areas such as archaeological sites, forests, wetlands, seaports, aviation zones, military zones, water bodies, and urban areas from which wind turbines should be placed at a specific distance.
The decision-makers are aggregated using Eq. 3:
where
Step 3: This computes the fuzzy weights matrix. The fuzzy comparison values are first calculated in this stage using Eq. 4:
Next, fuzzy weights
Where
Step 4: The center-of-area approach is used to defuzzify fuzzy weights using Eq. 6:
Step 5: Crisp weights normalization using Eq. 7:
Figure 5 displays the six steps that are integral to the F-AHP phase process. These steps guide the way in defining the problem accurately and ultimately establishing the solution that is most desired. These steps are computed using R-4.3.2 (R package for fuzzy AHP computation) and Excel software in this study.
Figure 6 provides a concise summary of the proposed research methodology, incorporating all the previously mentioned details.
This section will present and discuss the FAHP-GIS results in terms of spatial analysis of optimal wind turbine locations in Burundi. This includes the numerical values representing the optimal weight of the evaluation factors and the suitability map for the optimal wind sites.
In this study, the optimal weights of restriction factors mentioned in section Figure 5 are obtained using the FAHP-based MCDM method. Tables 4, 5 provides the numerical weights for each criterion (CRI), obtained through the use of Fuzzy pairwise comparison matrices. After obtaining the weight values for each criterion from the F-AHP pairwise comparison matrix presented in Table 3, this study utilized Buckley’s algorithm to calculate the geometric mean of fuzzy values (ri) and fuzzy weights (Wi) (Abdullah et al., 2021; Wang et al., 2021). To refine the results further, the averaged weight criterion (Mi) was normalized, resulting in the final normalized weight (Ni) for each criterion. The obtained weights corresponding to the criteria are given in Table 4.
According to the findings, the wind potential carries the greatest weight among other factors, amounting to an impressive 41% percentage. This means the wind potential has a high priority in the selection of the optimal areas for the implementation of wind farms. It is followed by the slope, proximity to the grids, distance from the roads, aspect, LULC and elevation with 24%, 13%, 8%, 7.3%, 4% and 4% respectively.
Mapping wind suitability involves a meticulous and systematic process, where each criterion is classified into specific categories (Benti et al., 2023). The approach thoroughly evaluates each criterion’s suitability level and ranks them accurately, from highest to lowest, to ensure precise results. This section introduces the classification of criteria and factors that are essential for evaluating suitable locations for wind farms. In this work, “Counts” represents the number of records of each class.
As mentioned on the map, Figure 7, the wind speed is classified into four scales: very good, good, moderate, and poor. About 2.10% of the land area registered very high, 30.67% high, 47.52% moderate and 19.72% low wind speed. The cut-in wind speed typically ranges between 2.5 and 4 m/s (Loukatou et al., 2018; Satymov et al., 2022; Wind-Energy, 2023). Approximately 32.77% of total land area is considered highly suitable for wind turbines, which are classified as class 3 and 4 exclusively based on wind speed.
According to the map of the DEM of Burundi, Figure 8, 13%, 30% and 50% are situated in the elevation ranging from 739 to 1000 m, 1000–1500 m and 1,500–2000 m a.g.l respectively. These regions correspondingly represent extremely, very strongly, strongly preferred places for the implementation of wind farms (Cunden et al., 2020; Chikoto et al., 2015). As it has been mentioned in section 2.3.3, the altitude of 2000m is considered the cut-off favourite elevation for wind resources. Accordingly, for Burundi, about 7% of the land area has an elevation above 2000 m making it an unsuitable or less preferred place for wind farm placement. More than 90% of Burundi’s land is suitable for wind farms due to its elevation being less than 2000 m.
Figure 9 displays the distance in km from the main national electric network. The area within a distance less than 5 km from the electrical lines is strongly suitable and the land areas between 5 km and 15 km are relatively suitable for wind site selection. However, it is considered impractical to implement wind farms in areas that are more than 15 km away from the national electric grid.
The maps of connectivity to main roads, Figure 10, show different categories of the distance from the national roads (logcluster, 2022). The land area situated at a distance of less than 0.5 km and a distance greater than 20 km is considered an exclusion zone for wind farm installation and represents 36% of Burundi. The Burundi land areas located at a distance in the range of 0.5–10 km away from the main roads are considered a strongly suitable location for wind farms. Furthermore, the land areas located at a distance ranging from 10 to 20 km are considered suitable for wind farm projects. In contrast, the land areas sited at a distance of 20 km or farther away from the main road network are unsuitable or less preferred for the implementation of wind farms.
Figure 11 displays the slope value distribution through Burundi. More than 70%of the Burundi, area is dominated by slope values below 15° with about 57% from 0°–10° and 18% from10°-15°. These areas are considered to be the most excellent places for wind farm installation considering the construction cost (Zalhaf et al., 2022; Baalousha et al., 2023). Places with slopes varying between 15° and 25° represent 19% and they are considered relatively favourable for wind turbine siting. The remaining areas which have slopes >25° are not appreciated for the wind farm locations.
The two main attributes of wind are its speed and direction. These attributes impact the wind farm implementation to optimize its energy production. The prevalent wind blows direction and aspect orientation are two linked factors influencing wind turbine production. As it has been mentioned before, flat terrains are highly recommended when choosing the locations of wind turbines. Therefore, in this work, the aspect of Burundi is evaluated and the percentage aspect distribution is given for each direction. The map of the aspect model of Burundi, Figure 12A, revealed that the aspect orientation consists of flat terrain by 8.1%, the north by 10.13%, the northeast by 10.53%, the east by 12.08%, the southeast by 13.31%, the south by 11.23%, the southwest by 10.81%, the west by 11.61% and the northwest by 12.2%. The wind rose of Burundi, Figure 12B is produced using on-field data obtained from the meteorological institution Institut Géographique du Burundi (IGEBU) through Matlab software. As shown in previous studies, Burundi’s predominant wind blow is from southwest to southeast (Placide et al., 2021). Consequently, the southeast, south and southwest aspect orientations are the fitting direction for wind farm implementation in Burundi. To this is added the flat land to totalize 43.45% of potential locations for wind farm construction in Burundi in terms of direction consideration.
This study used GIS to locate optimal sites for wind farms using raster and vector data sets as inputs. The weighted values are utilized to determine the degree of suitability for the wind turbine site selection of Burundi. Based on the annual average wind values and other factors, the wind farm site suitability map, Figure 13, displays three classes of sites. Locations with an average wind speed of less than 2.5 m/s are deemed inappropriate for the installation of wind farms. On the map, the land area in green colour is unsuitable to be a candidate for a wind farm at any scale.
The area in yellow colour represents the relatively suitable locations for wind farm sitting. The region in red colour is highly recommended as a suitable area to be a candidate for wind turbine sitting.
The main part of the suitable region is located in Lake Tanganyika, in the western part of Burundi. This area encompasses both the prime locations for wind farm sitting and the majority of the relatively suitable locations. This region is ideal for harnessing wind energy due to its strong and consistent ideal conditions for wind farm project development. Wind farms in this region could greatly boost renewable energy production in Burundi and aid in sustainable development. The region’s ample wind resources also have the potential to support global efforts against climate change. Apart from unsuitable areas, there also exist exclusion zones which cannot be eligible for wind farm installation. Such zones are found as protected areas on the Burundi wind farm site suitability map (Figure 12). They include forests, an airport, port, natural reserves and north lakes.
The GIS generates a useful attribute table that displays the area corresponding to each class. According to Figure 14, the outcomes show that just 1.96% (
The suitable area experiences wind speeds exceeding 7 m/s, which leads to a significant amount of potential energy generation. Table 6 further strengthens this point by showcasing the projected energy output for three selected wind turbines.
The estimated wind energy is for a single turbine, yet envisioning a wind farm comprised of hundreds of turbines, it becomes clear that deploying wind farms in appropriate locations can substantially enhance energy access in Burundi. For example, the Kenyan wind farm, boasting 365 wind turbines, each with an outstanding 850-kW capacity, holds a total capacity of 310 MW. In Burundi, a similar wind farm with Vestas V63 (1.5 MW) turbine type could generate 240 MW of power. The estimated capacity greatly exceeds the capacity of the largest existing power plant, Rwegura Hydropower, which has a capacity of only 18 MW (Nsabimana, 2020). With wind turbine implementation in place, total potential energy generation could increase exponentially, making it possible to meet a significant portion of Burundi’s energy demands. This would not only ensure a more reliable power supply but also contribute to reducing carbon emissions and promoting sustainable development in the country.
Burundi’s power supply is largely dominated by hydropower plants, making it heavily reliant on seasonal rainfall. This dependence results in major shortages during dry seasons, from June to August and December to January. To overcome this energy shortage, REGIDESO, a Burundi company in charge of electricity supply, owns diesel generators used as a backup power source to supplement the hydroelectric supply during dry seasons. The use of diesel has negative environmental impacts due to its high carbon emissions and contribution to air pollution.
The unit
The Estimated potential wind energy (EPWE) is determined by the following equation, Eq. 8:
Where A represents the area swept by the wind turbine blades, and
Every wind turbine generator has a maximum efficiency limit when it comes to converting the energy of the wind into useable energy. Albert Betz, a renowned German physicist, discovered this limit. He calculated that no wind turbine could convert more than 59.3% of its kinetic energy into mechanical energy. This is known as the Betz Limit and represents the theoretical maximum coefficient of power that any wind turbine can achieve (Ranjbar et al., 2019; De et al., 2018). Considering the Betz Limit, Eq. 8 can be refined as follows, Eq. 9:
The annual EPWE (AEPWE) is given by Eq. 10:
This study used the MCDM system coupled with GIS to produce the wind suitability map of Burundi. The WRF model simulation outputs over Burundi territory were employed to classify the regions in terms of wind speed potential. Topographical, climatological, and location restriction factors were established. These restrictions such as aspect, slope, elevation, distance from major electrical transmission lines, distance from main roads and the protected zones divided the Burundi country into suitable and unsuitable areas for wind power facilities placement.
The results indicate that the Burundi territory has highly favourable areas for extracting wind energy, making it suitable for implementing medium-scale wind farms. The western part of the country has the highest potential for wind farms. The results signify about 1.96% of the hall country with the highest wind farm potential. At the same time, approximately 19% of the study area registers relatively suitable wind farm potential.
As it is the first time this kind of study has been used to evaluate the optimal wind site selection over Burundi, it is recommended that further research focus on additional methods for optimal wind site selection to compare the results. Additionally, the study did not distinguish between onshore and offshore wind farm site assessments. To achieve this, the authors plan to conduct a techno-economic analysis to evaluate the unit cost of wind energy in various locations throughout Burundi.
The raw data supporting the conclusion of this article will be made available by the authors, without undue reservation.
GP: Conceptualization, Writing–review and editing. ML: Supervision, Writing–review and editing.
The author(s) declare that no financial support was received for the research, authorship, and/or publication of this article.
The authors want to express gratitude to the African Centre of Excellence in Energy for Sustainable Development ACEESD), University of Rwanda for providing a suitable academic environment.
The authors declare that the research was conducted in the absence of any commercial or financial relationships that could be construed as a potential conflict of interest.
All claims expressed in this article are solely those of the authors and do not necessarily represent those of their affiliated organizations, or those of the publisher, the editors and the reviewers. Any product that may be evaluated in this article, or claim that may be made by its manufacturer, is not guaranteed or endorsed by the publisher.
Abdelmassih, G., Al-Numay, M., and El Aroudi, A. (2021). Map optimization fuzzy logic framework in wind turbine site selection with application to the USA wind farms. Energies 14 (19), 1–15. doi:10.3390/en14196127
Abdullah, A. G., Setiorini, A. H., Dwitasari, N. A., Hakim, D. L., and Aziz, M. (2021). Location suitability analysis for wind farm exploitation using fuzzy analytic hierarchy process. Indones. J. Sci. Technol. 6 (3), 523–534. doi:10.17509/ijost.v6i3.38957
Ajanaku, B. A., Strager, M. P., and Collins, A. R. (2022). GIS-based multi-criteria decision analysis of utility-scale wind farm site suitability in West Virginia. GeoJournal 87 (5), 3735–3757. doi:10.1007/s10708-021-10453-y
Aksoy, E., and San, B. T. (2019). Geographical information systems (GIS) and Multi-Criteria Decision Analysis (MCDA) integration for sustainable landfill site selection considering dynamic data source. Bull. Eng. Geol. Environ. 78 (2), 779–791. doi:10.1007/s10064-017-1135-z
Alhuyi Nazari, A., Assad, M., Haghighat, M. E. H., and Maleki, S. (2020). “Applying TOPSIS method for wind farm site selection in Iran,” in Adv. Sci. Eng. Technol. Int. Conf. (ASET), Dubai, United Arab Emirates, June 2020, 4.
Ali, S., Lee, S. M., and Jang, C. M. (2017). Determination of the most optimal on-shore wind farm site location using a GIS-MCDM methodology: evaluating the case of South Korea. Energies 10 (12), 2072. doi:10.3390/en10122072
Aliyev, R., Temizkan, H., and Aliyev, R. (2020). Fuzzy analytic hierarchy process-based multi-criteria decision making for universities ranking. Symmetry (Basel). 12 (8), 1–15. doi:10.3390/sym12081351
Ayodele, T. R., Ogunjuyigbe, A. S. O., Odigie, O., and Munda, J. L. (2018). A multi-criteria GIS based model for wind farm site selection using interval type-2 fuzzy analytic hierarchy process: the case study of Nigeria. Appl. Energy 228, 1853–1869. June. doi:10.1016/j.apenergy.2018.07.051
Baalousha, H. M., Younes, A., Yassin, M. A., and Fahs, M. (2023). Comparison of the fuzzy analytic hierarchy process (F-AHP) and fuzzy logic for flood exposure risk assessment in arid regions. Hydrology 10 (7), 136. doi:10.3390/hydrology10070136
Badi, I., Pamučar, D., Stević, Ž., and Muhammad, L. J. (2023). Wind farm site selection using BWM-AHP-MARCOS method: a case study of Libya. Sci. Afr. 19, e01511. doi:10.1016/j.sciaf.2022.e01511
Badran, O., Abdulhadi, E., and El-Tous, Y. (2011). Fuzzy logic controller for predicting wind turbine power generation. Int. J. Mech. Mat. Eng. 6 (1), 51–66.
Balogun, A. L., Matori, A. N., Hamid-Mosaku, A. I., Umar Lawal, D., and Ahmed Chandio, I. (2017). Fuzzy MCDM-based GIS model for subsea oil pipeline route optimization: an integrated approach. Mar. Georesources Geotechnol. 35 (7), 961–969. doi:10.1080/1064119X.2016.1269247
Barakat, A., Ouargaf, Z., Khellouk, R., El Jazouli, A., and Touhami, F. (2019). Land use/land cover change and environmental impact assessment in béni-mellal district (Morocco) using remote sensing and GIS. Earth Syst. Environ. 3 (1), 113–125. doi:10.1007/s41748-019-00088-y
Bashahu, M., and Buseke, M. (2016). Statistical analysis of hourly wind speed data from some Burundian stations using beta probability density functions. Mod. Environ. Sci. Eng. 2 (11), 740–746. doi:10.15341/mese(2333-2581)/11.02.2016/005
Bashahu, M., Nsabimana, P., Barakamfitiye, J., and Niyukuri, F. (2022). Assessment of the wind energy potential of two Burundian sites. Energy Power Eng. 14 (05), 181–200. doi:10.4236/epe.2022.145010
Benti, N. E., Alemu, Y. B., Balta, M. M., Gunta, S., Chaka, M. D., Semie, A. G., et al. (2023). Site suitability assessment for the development of wind power plant in Wolaita area, Southern Ethiopia: an AHP-GIS model. Sci. Rep. 13 (1), 19811–19815. doi:10.1038/s41598-023-47149-x
Caceoğlu, E., Yildiz, H. K., Oğuz, E., Huvaj, N., and Guerrero, J. M. (2022). Offshore wind power plant site selection using Analytical Hierarchy Process for Northwest Turkey. Ocean. Eng. 252, 111178. doi:10.1016/j.oceaneng.2022.111178
Cavazzi, S., and Dutton, A. G. (2016). An Offshore Wind Energy Geographic Information System (OWE-GIS) for assessment of the UK’s offshore wind energy potential. Renew. Energy 87 (2016), 212–228. doi:10.1016/j.renene.2015.09.021
Chikoto, M., Gwenzi, J., Maruziva, R., and Buka, L. (2015). Mapping suitable sites for setting up wind farms: a case study of nyanga district. Ethiop. J. Environ. Stud. Manag. 8 (4), 351. doi:10.4314/ejesm.v8i4.1
Cunden, T. S. M., Doorga, J., Lollchund, M. R., and Rughooputh, S. D. D. V. (2020). Multi-level constraints wind farms siting for a complex terrain in a tropical region using MCDM approach coupled with GIS. Energy 211, 118533. doi:10.1016/j.energy.2020.118533
Damousis, I. G., Alexiadis, M. C., Theocharis, J. B., and Dokopoulos, P. S. (2004). A fuzzy model for wind speed prediction and power generation in wind parks using spatial correlation. IEEE Trans. Energy Convers. 19 (2), 352–361. doi:10.1109/TEC.2003.821865
De Lellis, M., Reginatto, R., Saraiva, R., and Trofino, A. (2018). The betz limit applied to airborne wind energy. Renew. Energy 127, 32–40. doi:10.1016/j.renene.2018.04.034
Devex (2013). Ministère de l ’ Energie et des Mines Etude diagnostique du secteur de l ’ Energie au Burundi dans le cadre de l ’ Initiative du Secrétaire Général des Nations Unies sur l ’ Energie durable pour tous (Sustainable Energy for All) Juin 2013 Réalisé avec l.
Dhunny, A. Z., Doorga, J. R. S., Allam, Z., Lollchund, M. R., and Boojhawon, R. (2019). Identification of optimal wind, solar and hybrid wind-solar farming sites using fuzzy logic modelling. Energy 188, 116056. doi:10.1016/j.energy.2019.116056
Diffendorfer, J. E., Stanton, J. C., Beston, J. A., Thogmartin, W. E., Loss, S. R., Katzner, T. E., et al. (2021). Demographic and potential biological removal models identify raptor species sensitive to current and future wind energy. Ecosphere 12 (6). doi:10.1002/ecs2.3531
DIVA-GIS (2023). Download data by country | DIVA-GIS. Available at: https://www.diva-gis.org/gdata (Accessed October 28, 2023).
Dreamstime (2023). Burundi map stock vector. Illustration of ocean, geographic - 27837876. Available at: https://www.dreamstime.com/royalty-free-stock-image-burundi-map-image27837876 (Accessed October 28, 2023).
Ecer, F. (2021). Sustainability assessment of existing onshore wind plants in the context of triple bottom line: a best-worst method (BWM) based MCDM framework. Environ. Sci. Pollut. Res. 28 (16), 19677–19693. doi:10.1007/s11356-020-11940-4
ENERGYDATA (2007). Burundi - electricity transmission network - dataset - ENERGYDATA.INFO. Available at: https://energydata.info/dataset/burundi-electricity-transmission-network-2007.
Feng, J. (2021). Wind farm site selection from the perspective of sustainability: a novel satisfaction degree-based fuzzy axiomatic design approach. Int. J. Energy Res. 45 (1), 1097–1127. doi:10.1002/er.6256
Gao, J., Guo, F., Ma, Z., Huang, X., and Li, X. (2020). Multi-criteria group decision-making framework for offshore wind farm site selection based on the intuitionistic linguistic aggregation operators. Energy 204, 117899. doi:10.1016/j.energy.2020.117899
Gil-García, I. C., Ramos-Escudero, A., García-Cascales, M. S., Dagher, H., and Molina-García, A. (2022). Fuzzy GIS-based MCDM solution for the optimal offshore wind site selection: the Gulf of Maine case. Renew. Energy 183, 130–147. doi:10.1016/J.RENENE.2021.10.058
Havle, C. A., and Kılıç, B. (2019). A hybrid approach based on the fuzzy AHP and HFACS framework for identifying and analyzing gross navigation errors during transatlantic flights. J. Air Transp. Manag. 76 (2018), 21–30. doi:10.1016/j.jairtraman.2019.02.005
Heckmann, M., Vassolo, S., and Tiberghien, C. (2016). Groundwater vulnerability map (COP) for the nyanzari catchment, gitega, Burundi. doi:10.13140/RG.2.2.36199.80802
Hoang, T. N., Ly, T. T. B., and Do, H. T. T. (2022). A hybrid approach of wind farm site selection using Group Best-Worst Method and GIS-Based Fuzzy Logic Relations. A case study in Vietnam. Environ. Qual. Manag. 32, 251–267. doi:10.1002/tqem.21839
Hussaini, M. S., Farahmand, A., Shrestha, S., Neupane, S., and Abrunhosa, M. (2022). Site selection for managed aquifer recharge in the city of Kabul, Afghanistan, using a multi-criteria decision analysis and geographic information system. Hydrogeol. J. 30 (1), 59–78. doi:10.1007/s10040-021-02408-x
Ifkirne, M., El Bouhi, H., Acharki, S., Pham, Q. B., Farah, A., and Linh, N. T. T. (2022). Multi-criteria GIS-based analysis for mapping suitable sites for onshore wind farms in southeast France. Land 11 (10), 1839. doi:10.3390/land11101839
Indrajayanthan, V., and Mohanty, N. K. (2022). Assessment of clean energy transition potential in major power-producing states of India using multi-criteria decision analysis. Sustain 14 (3), 1166. doi:10.3390/su14031166
JAPAN INTERNATIONAL COOPERATION AGENCY (2010). Final report of the preparatory Survey on the project for Japan international cooperation agency. Available at: http://open_jicareport.jica.go.jp/pdf/12003828_01.pdf.
Kaya, İ., Çolak, M., and Terzi, F. (2019). A comprehensive review of fuzzy multi criteria decision making methodologies for energy policy making. Energy Strateg. Rev. 24, 207–228. March. doi:10.1016/j.esr.2019.03.003
Łaska, G. (2020). Wind energy and multicriteria analysis in making decisions on the location of wind farms: a case study in the North-eastern of Poland. Model. Simul. Optim. Wind Farms Hybrid. Syst., 1–18. no. Mcdm. doi:10.5772/intechopen.90450
Li, C., Zhang, T., Wang, X., and Lian, Z. (2022). Site selection of urban parks based on fuzzy-analytic hierarchy process (F-AHP): a case study of nanjing, China. Int. J. Environ. Res. Public Health 19 (20), 13159. doi:10.3390/ijerph192013159
Li, M., Xu, Y., Guo, J., Li, Y., and Li, W. (2020). Application of a GIS-based fuzzy multi-criteria evaluation approach for wind farm site selection in China. Energies (MDPI) 13 (10), 2426. doi:10.3390/en13102426
logcluster (2022). 2.3 Burundi road network | digital logistics capacity assessments. Available at: https://dlca.logcluster.org/23-burundi-road-network.
Loukatou, A., Howell, S., Johnson, P., and Duck, P. (2018). Stochastic wind speed modelling for estimation of expected wind power output. Appl. Energy 228, 1328–1340. May. doi:10.1016/j.apenergy.2018.06.117
Mardani, A., Jusoh, A., Nor, K. M. D., Khalifah, Z., Zakwan, N., and Valipour, A. (2015). Multiple criteria decision-making techniques and their applications - a review of the literature from 2000 to 2014. Econ. Res. Istraz. 28 (1), 516–571. doi:10.1080/1331677X.2015.1075139
Mohammadzadeh Bina, S., Jalilinasrabady, S., Fujii, H., and Farabi-Asl, H. (2018). A comprehensive approach for wind power plant potential assessment, application to northwestern Iran. Energy 164, 344–358. doi:10.1016/j.energy.2018.08.211
Mosadeghi, R., Warnken, J., Tomlinson, R., and Mirfenderesk, H. (2015). Comparison of Fuzzy-AHP and AHP in a spatial multi-criteria decision making model for urban land-use planning. Comput. Environ. Urban Syst. 49, 54–65. doi:10.1016/j.compenvurbsys.2014.10.001
Mustafa, A. M., and Barabadi, A. (2022). Criteria-based fuzzy logic risk analysis of wind farms operation in cold climate regions. Energies 15 (4), 1335. doi:10.3390/en15041335
Nasery, S., Matci, D. K., and Avdan, U. (2021). GIS-based wind farm suitability assessment using fuzzy AHP multi-criteria approach: the case of Herat, Afghanistan. Arab. J. Geosci. 14 (12), 1091. doi:10.1007/s12517-021-07478-5
Nguyen, V. T., Hai, N. H., Thi, N., and Lan, K. (2022). Spherical fuzzy multicriteria decision-making model for wind, 1–12.
Nkunzimana, A., Bi, S., Jiang, T., Wu, W., and Abro, M. I. (2019). Spatiotemporal variation of rainfall and occurrence of extreme events over Burundi during 1960 to 2010. Arab. J. Geosci. 12 (5), 176. doi:10.1007/s12517-019-4335-y
Noorollahi, E., Fadai, D., Shirazi, M. A., and Ghodsipour, S. H. (2016a). Land suitability analysis for solar farms exploitation using GIS and fuzzy analytic hierarchy process (FAHP) - a case study of Iran. Energies 9 (8), 1–24. doi:10.3390/en9080643
Noorollahi, Y., Yousefi, H., and Mohammadi, M. (2016b). Multi-criteria decision support system for wind farm site selection using GIS. Sustain. Energy Technol. Assessments 13, 38–50. doi:10.1016/j.seta.2015.11.007
Nsabimana, R. (2020). Electricity sector organization and performance in Burundi. Proceedings 58 (1), 26. doi:10.3390/wef-06938
Özdemir, ónal, Altinpinar, İ., and Demirel, F. B. (2018). A MCDM approach with fuzzy AHP method for occupational accidents on board. TransNav, Int. J. Mar. Navig. Saf. Sea Transp. 12 (1), 93–98. doi:10.12716/1001.12.01.10
Pamucar, D., Gigovic, L., Bajic, Z., and Janoševic, M. (2017). Location selection for wind farms using GIS multi-criteria hybrid model: an approach based on fuzzy and rough numbers. Sustain 9 (8), 1315. doi:10.3390/su9081315
Pang, N., Nan, M., Meng, Q., and Zhao, S. (2021). Selection of wind turbine based on fuzzy analytic network process: a case study in China. Sustain 13 (4), 1792. doi:10.3390/su13041792
Perkin, S., Garrett, D., and Jensson, P. (2015). Optimal wind turbine selection methodology: a case-study for Búrfell, Iceland. Renew. Energy 75, 165–172. doi:10.1016/j.renene.2014.09.043
Placide, G., Lollchund, M. R., and Dalso, G. A. (2021). Wind energy potential assessment of some sites in Burundi using statistical modelling. IEEE PES/IAS PowerAfrica, PowerAfrica (1), 1–5. doi:10.1109/PowerAfrica52236.2021.9543186
Rajendran, S., Diaz, M., Cárdenas, R., Espina, E., Contreras, E., and Rodriguez, J. (2022). A review of generators and power converters for multi-MW wind energy conversion systems. Process 10 (11), 2302. doi:10.3390/PR10112302
Ranjbar, M. H., Nasrazadani, S. A., Kia, H. Z., and Gharali, K. (2019). Energy Equipment and Systems Reaching the betz limit experimentally and numerically. Energy Equip. Sys 7 (3), 271–278.
Rediske, G., Burin, H. P., Rigo, P. D., Rosa, C. B., Michels, L., and Siluk, J. C. M. (2021). Wind power plant site selection: a systematic review. Renew. Sustain. Energy Rev. 148, 111293. September. doi:10.1016/j.rser.2021.111293
Rehman, S., and Khan, S. A. (2016). Fuzzy logic based multi-criteria wind turbine selection strategy - a case study of Qassim, Saudi Arabia. Energies 9 (11), 872. doi:10.3390/en9110872
Sadorsky, P. (2021). Wind energy for sustainable development: driving factors and future outlook. J. Clean. Prod. 289, 125779. doi:10.1016/j.jclepro.2020.125779
Sánchez-Lozano, J. M., Escudero, A. R., Gil-García, I. C., García-Cascales, M. S., and Molina-García, A. (2021). Comparative analysis analysis based based on GIS and fuzzy MCDM approaches. Case Study Offshore Offshore Wind Wind Site Site Sel. Sel. Gulf Gulf Maine 3, 211–218. Mcdm.
Sánchez-Lozano, J. M., García-Cascales, M. S., and Lamata, M. T. (2014). Identification and selection of potential sites for onshore wind farms development in region of murcia, Spain. Energy 73 (2014), 311–324. doi:10.1016/j.energy.2014.06.024
Sánchez-Lozano, J. M., García-Cascales, M. S., and Lamata, M. T. (2016). GIS-based onshore wind farm site selection using Fuzzy Multi-Criteria Decision Making methods. Evaluating the case of Southeastern Spain. Appl. Energy 171 (2016), 86–102. doi:10.1016/j.apenergy.2016.03.030
Saraswat, S. K., Digalwar, A. K., Yadav, S. S., and Kumar, G. (2021). MCDM and GIS based modelling technique for assessment of solar and wind farm locations in India. Renew. Energy 169, 865–884. doi:10.1016/j.renene.2021.01.056
Satymov, R., Bogdanov, D., and Breyer, C. (2022). Global-local analysis of cost-optimal onshore wind turbine configurations considering wind classes and hub heights. Energy 256, 124629. doi:10.1016/J.ENERGY.2022.124629
Sedaghat, A., Alkhatib, F., Eilaghi, A., Sabati, M., Borvayeh, L., and Mostafaeipour, A. (2019). A new strategy for wind turbine selection using optimization based on rated wind speed. Energy Procedia 160 (2018), 582–589. doi:10.1016/j.egypro.2019.02.209
Seyed Alavi, S. M., Maleki, A., and Khaleghi, A. (2022). Optimal site selection for wind power plant using multi-criteria decision-making methods: a case study in eastern Iran. Int. J. Low-Carbon Technol. 17, 1319–1337. November. doi:10.1093/ijlct/ctac009
Shafiee, M. (2022). Wind energy development site selection using an integrated fuzzy ANP-TOPSIS decision model. Energies 15 (12), 4289. doi:10.3390/en15124289
Shahid Ali, J., untakan Taweekun, S. G., Techato, K., Waewsak, J., and Gyawali, S. (2019). GIS based site suitability assessment for wind and solar farms in Songkhla, Thailand. Renew. Energy 132, 1360–1372. doi:10.1016/j.renene.2018.09.035
Tabutin, D., and Schoumaker, B. (2020). La démographie de l'Afrique subsaharienne au XXI e siècle: Bilan des changements de 2000 à 2020, perspectives et défis d’ici 2050. Popul. Paris. 75 (2–3), 169–295. doi:10.3917/popu.2002.0169
Taoufik, M., and Fekri, A. (2021). GIS-based multi-criteria analysis of offshore wind farm development in Morocco. Energy Convers. Manag. X 11, 100103. doi:10.1016/j.ecmx.2021.100103
Tripathi, A. K., Agrawal, S., and Gupta, R. D. (2022). Comparison of GIS-based AHP and fuzzy AHP methods for hospital site selection: a case study for Prayagraj City, India. GeoJournal 87 (5), 3507–3528. doi:10.1007/s10708-021-10445-y
US Geological Survey (2019). USGS earth explorer tool. Available at: https://earthexplorer.usgs.gov/.
Van Sark, W. G. J. H. M., Van der Velde, H. C., Coelingh, J. P., and Bierbooms, W. A. A. M. (2019). Do we really need rotor equivalent wind speed? Wind Energy 22 (6), 745–763. doi:10.1002/we.2319
Villacreses, G., Gaona, G., Martínez-Gómez, J., and Jijón, D. J. (2017). Wind farms suitability location using geographical information system (GIS), based on multi-criteria decision making (MCDM) methods: the case of continental Ecuador. Renew. Energy 109, 275–286. doi:10.1016/j.renene.2017.03.041
Wang, C. N., Dang, T. T., and Nguyen, N. A. T. (2021). Location optimization of wind plants using dea and fuzzy multi-criteria decision making: a case study in vietnam. IEEE Access 9, 116265–116285. doi:10.1109/access.2021.3106281
Wind-Energy (2023). Wind turbine basics and data (A feasibility study of renewable energy solutions). Available at: https://www.ese.wustl.edu/∼nehorai/NaitikJessica/sites.google.com/site/wugreenenergy/wind-energy.html (Accessed October 15, 2023).
Wu, B., Yip, T. L., Xie, L., and Wang, Y. (2018). A fuzzy-MADM based approach for site selection of offshore wind farm in busy waterways in China. Ocean. Eng. 168, 121–132. August. doi:10.1016/j.oceaneng.2018.08.065
Xu, Y., Li, Y., Zheng, L., Cui, L., Li, S., Li, W., et al. (2020). Site selection of wind farms using GIS and multi-criteria decision making method in Wafangdian, China. Energy 207, 118222. doi:10.1016/j.energy.2020.118222
Yalcin, M., and Kilic Gul, F. (2017). A GIS-based multi criteria decision analysis approach for exploring geothermal resources: akarcay basin (Afyonkarahisar). Geothermics 67, 18–28. doi:10.1016/j.geothermics.2017.01.002
Yousefi, H., Motlagh, S. G., and Montazeri, M. (2022). Multi-criteria decision-making system for wind farm site-selection using geographic information system (GIS): case study of semnan province, Iran. Sustainability 14 (13), 7640. doi:10.3390/su14137640
Zalhaf, A. S., Elboshy, B., Kotb, K. M., Han, Y., Almaliki, A. H., Aly, R. M. H., et al. (2022). A high-resolution wind farms suitability mapping using gis and fuzzy ahp approach: a national-level case study in Sudan. Sustain 14 (1), 358. doi:10.3390/su14010358
Keywords: fuzzy theory, wind farm location, decision-making, fuzzy-analytic hierarchy process, restriction factors
Citation: Placide G and Lollchund MR (2024) Wind farm site selection using GIS-based mathematical modeling and fuzzy logic tools: a case study of Burundi. Front. Energy Res. 12:1353388. doi: 10.3389/fenrg.2024.1353388
Received: 10 December 2023; Accepted: 15 April 2024;
Published: 29 April 2024.
Edited by:
Harish Puppala, SRM University, IndiaReviewed by:
Nagababu Garlapati, Pandit Deendayal Energy University, IndiaCopyright © 2024 Placide and Lollchund. This is an open-access article distributed under the terms of the Creative Commons Attribution License (CC BY). The use, distribution or reproduction in other forums is permitted, provided the original author(s) and the copyright owner(s) are credited and that the original publication in this journal is cited, in accordance with accepted academic practice. No use, distribution or reproduction is permitted which does not comply with these terms.
*Correspondence: Gatoto Placide, cGxhY2lkZWdhQHlhaG9vLmZy
Disclaimer: All claims expressed in this article are solely those of the authors and do not necessarily represent those of their affiliated organizations, or those of the publisher, the editors and the reviewers. Any product that may be evaluated in this article or claim that may be made by its manufacturer is not guaranteed or endorsed by the publisher.
Research integrity at Frontiers
Learn more about the work of our research integrity team to safeguard the quality of each article we publish.