- 1U.S.-Pakistan Center for Advanced Studies in Energy (USPCAS-E), National University of Sciences and Technology (NUST), Islamabad, Pakistan
- 2Department of Electrical Engineering, College of Engineering, Majmaah University, Al-Majmaah, Saudi Arabia
- 3Engineering and Applied Science Research Center, Majmaah University, Riyadh, Saudi Arabia
- 4School of Computing and Engineering, University of Huddersfield, Huddersfield, United Kingdom
- 5Department of Electrical Engineering, Mirpur University of Science and Technology, Mirpur, Pakistan
- 6Department of Electrical Engineering, College of Engineering, University of Ha’il, Ha’il, Saudi Arabia
- 7Department of Engineering Sciences, University of Oxford, Oxford, United Kingdom
This paper developed an intelligent multi-agent system (MAS) with a multi-layer framework for multi-microgrids (MMGs) using robust and modern communication patterns for deployed agents to achieve distributed tasks. The MMG paradigm introduces three microgrids (MGs) based on the type of load, working environment, and living habitat: residential, commercial, and industrial. In addition, a day-ahead and real-time model is proposed for day-ahead and real-time signals. Intelligent agents in the multi-layer MAS framework make smart decisions based on multiple algorithms to optimize schedule power and minimize costs, considering demand dispatch and demand response as core components. Maximum renewable energy utilization aims to increase user comfort and reduce greenhouse gas (GHG) emissions. Load agents deployed in each MG ensure maximum efficiency. The proposed framework recommends various tariff rates and tariff adjustment strategies to promote and offer an economic evaluation across the respective indices. To minimize the monopoly of the energy market, an efficient energy market model is developed for the proposed MMG paradigm to maximize the competition by incorporating future and spot-market trading schemes for day-ahead and real-time signals. The comparative analysis indicates optimized results based upon the cost-benefit analysis, cost reduction, power transaction in the market, and maximum utilization of renewable energy resources (RERs).
1 Introduction
1.1 Background
According to the International Energy Agency, the renewable energy utilization rate increased by 3.7% in 2019, a minor increase compared to that of the previous year. Wind (150 TWh) and solar (140 TWh) power accounted for 27% of the global electricity supply and contributed to 85% of renewable energy growth. The main factors contributing to such growth are extensive support in policy and a reduction in technological prices. Consequently, the power sector lowered greenhouse gas (GHG) emissions by nearly 170 Mt in 2019 (IEA, 2020). Furthermore, in a microgrid, to tackle the resiliency and reliability issues arising from the intermittent nature of renewable energy resources (RERs), this concept has been introduced to maximize energy utilization and the power system’s credibility. According to the U.S. Department of Energy, a microgrid (MG) is a group of interconnected loads and distributed generation resources (DERs) within clearly defined electrical boundaries that act as a single controllable entity concerning the grid (Ton and Smith, 2012).
The microgrid's broader concept revolves around the deployment of self-sufficient distributed electricity generation resources on a small scale along with the energy storage systems (ESSs) near the consumers with adequate controllability over consumption demand (Hirsch et al., 2018). The generation resources may also include conventional resources along with clean energy. Microgrids can operate in two modes: grid-connected and islanded. The decentralized and distributed architecture of the resources in microgrids completely negates the transmission losses. Microgrid ecosystems allow automation, controllability, and monitoring of distributed assets within a cost-effective economic operation, making them less expensive than centralized and main-grid systems (Cortes et al., 2017). Multi-microgrids (MMGs) can be referred to as a networked cluster of adjoining microgrids for coordination of energy management and interactive assistance among each other (Xu et al., 2018).
The interconnectivity of microgrids uses different factors, including the more effective use of renewable energy sources, lower operating costs, black-out support, increased stability, and resiliency (Alam et al., 2020). All the benefits mentioned above are achievable through effective energy management in both the microgrid and multi-microgrid paradigms. Energy management is widely applicable to achieve optimal load scheduling based on available energy and market considerations. Energy interactions across the grids under the multi-microgrid paradigm boost stakeholder engagement, resulting in more competitive energy markets. As a result, the MMGs’ power and economic stability have improved (Yang et al., 2019). According to Khalid et al. (2019), energy management improves grid dependability and stability while lowering customer costs and usage. Zlatkovic et al. (2019) applied demand side management using real-time pricing and an inclining block rate (IBR) tariff to schedule a load for homes effectively.
Demand side management (DSM) is a method of energy management that benefits both customers and utilities. Liang et al. (2019) implemented the demand response on a commercial building to achieve satisfactory user comfort within an optimal operating cost range. Demand response is one of the techniques of demand side management, which allows users to change their consumption patterns at peak hours in response to the pricing signal. In addition to residential and commercial MGs, industrial microgrids have also gained popularity in recent years. Naderi et al. (2017) shows the planning of industrial estate for maximizing renewable resource utilization, keeping in view the reliable and sustainable operation of sensitive loads. Choobineh and Mohagheghi (2016) proposed a framework for the industrial microgrid that can achieve optimal energy usage, reduce the cost, and delay the lifetime of assets. The number of MGs in distribution networks has increased dramatically due to the fast adoption of DERs. As a result, MG interaction and energy exchange are significant concerns in multi-microgrid systems.
Tan and Chen (2020) aimed to concurrently minimize the system’s cost, emissions, and loss through multi-objective optimization. Ahmadi and Rezaei (2020) decreased the cost and increased the generation performance of the multi-microgrid framework using the proposed the demand response program (DRP) strategy in energy management. To deploy a microgrid, it is necessary to undertake an economic study of the project, allowing investors to relate to it. Husein and Chung (2018) conducted an economic analysis for designing an optimal microgrid considering the tax benefits and incentives of integrating RERs. Oueid (2019) discussed the financial information required for planning MGs to facilitate evaluating similar projects. The Microgrid Decision Support Tool (MDSTool) was used in Vu et al. (2019) to conduct a detailed technical and economic analysis of grid-connected PVs with the energy storage system to achieve an optimal university microgrid. The net present value (NPV) is used as a metric for economic evaluation of the project. The MMG system’s dependability and economic evaluations are studied in Wang et al. (2018).
1.2 Literature review and research gap
In multi-microgrids, an energy management system (EMS) is used to optimize the scheduling of power resources and energy storage devices to ensure supply–demand equilibrium through exchanging power among grids by adopting different trading mechanisms. During the last few years, there has been a surge of attention on the EMS among researchers for interconnected multi-microgrids. A hierarchical Stackelberg game model in an MAS with three separate stack-holders was proposed in Dong et al. (2020). The suggested energy trading model maximizes each participant’s economic rewards while achieving energy management via the incentive demand response. However, existing research does not include a full sensitivity analysis of this model nor provides detailed information about the load type in microgrids. Furthermore, the model considers only day-ahead operation for all the three stack-holders and ignores real-time operation. Within the multi-microgrid paradigm, Zhuo et al. (2019) suggested an independent MG for battery energy storage systems (BESSs). Parallel to demand response initiatives, the independent BESS will maximize the share of renewable energy sources while reducing grid power dependency. However, the studies cannot provide a detailed study of the suggested framework’s financial report and scheduled load. Bui et al. (2018) proposed the concept of adjustable power in a multi-agent framework for a multi-microgrid paradigm. Mixed-integer linear programming (MILP) underpins all established models, making them simple to apply and computationally inexpensive. The proposed methodology reduces the MMG’s operational costs while ignoring GHG emissions.
Using model predictive control (MPC) and two-stage stochastic programming with recourse, Systems et al. (2020) proposed an efficient control technique for a hierarchal framework-based MMG system with integrated energy management. The authors claim to have achieved a reduction in grid power exchange as well as an optimal solution technique for power scheduling. Yin and Li (2021) focused on developing an energy management framework for achieving decentralized autonomous microgrids that operate in an MMG system with optimal operation coordination. The hybrid metaheuristic multi-layer reinforcement learning technique is implemented to lower the peak-to-average ratios, reduce power fluctuations, lower operational costs, lower computational strain, and improve load forecasting. By deploying fuzzy-based peer-to-peer energy exchange in interconnected multi-microgrids, the energy management technique presented in Thirugnanam et al. (2021) minimizes the cost of consumer energy use. The random vector functional link network (RVFLN) technique models load demand and distributed generators (DGs). For peer-to-peer energy exchange, dynamic pricing is used. This work does not go through the different loads or how they are scheduled. Additionally, the availability of historical data is a barrier to deploying such models.
A unique online consensus alternating direction method of multipliers (OC-ADMM) algorithm is presented in Guo et al. (2021) to handle P2P trading mechanisms in real-time. This mechanism lessens the computational burden with precise assessment and maximizes online social welfare. The distributed power management system discussed in Rajaei et al. (2020) is based on the alternating direction method of multipliers (ADMM) in the distributed MMG hierarchy for day-ahead operational scheduling considering the technical constraints of the distribution network. Unlike a conventional centralized controller, a transactive energy signal initiates ADMM and MG scheduling processes for distributed microgrid coordination. Liu et al. (2021) optimized energy scheduling and the trading of microgrid and electric vehicles with nearby microgrids by considering the availability of fast charging services in the MAS framework. The authors used convex optimization to solve the problem of time-flexible EV driving. Purage et al. (2022) aimed at achieving market equilibrium, global optimality, and microgrid constraint without invading market participants’ privacy in a decentralized P2P energy trading mechanism.
The ADMM is applied to each market participant for coordination in the proposed market framework. Energy management for four nanogrids comprising two residential, one commercial, and one industrial building was presented in Ramadan et al. (2019). The authors viewed nanogrids as prosumers or aggregators, resulting in an effective demand response (DR) and lower operational costs. Using the MATLAB and JADE framework for Multi-Agent Network (MAN) deployment, a hybridized reinforcement learning and powerful machine learning algorithm accomplished optimal trading and scheduling of nanogrids. The authors, however, neglect to specify the load details for each nanogrid. Rezaei et al. (2022) introduced conditional value-at-risk (CVaR) into the scheduling problem of the MMG system to reduce the low-profit risk even in the worst-case situation. The findings show that raising the emission factor and risk does not influence the predicted profit decrease. Karimi and Jadid (2023) proposed a tri-layer integrated energy system framework in the MG that minimizes costs and maximizes the integration of RERs. However, the proposed work has not considered the economic analysis and device-level load scheduling. Furthermore, the proposed framework fails to minimize the robustness of an operation at each layer.
Karimi et al. (2023) suggested the Average Power Flexibility during Peak Period Index (APFDPPI) to reduce the occurrence of new peaks and maximize the reliability of the MMG system due to the uncoordinated DR. Real-time (RT) MMG operation and economic evaluation for independent investors are not presented in the proposed research. A bi-layer predictive energy management system (PEMS) is deployed (Xu et al., 2023) in uncertain renewable multi-energy systems for reliable operation of microgrids and to reduce operational costs. The authors have not discussed the energy transaction among grids and the details of the MG load nature and its scheduling. Ali et al. (2023) proposed the energy management system for the residential load only but have neglected the priorities to be assigned to the appliances during low generation power. Furthermore, the economic viability of the MG for power transactions in the energy market is not highlighted. To ensure the reliable and stable operation of the MG cluster, a centerless control strategy is employed in Shi et al. (2023). The proposed work has not discussed the energy management strategies for efficient operation of MGs. Furthermore, the techniques to use renewables and load scheduling are also neglected. Zhong et al. (2022) used the ADMM in day-ahead scheduling to reduce the MMG’s operating expenses and increase energy while maintaining confidentiality. For each MG, the rolling horizon method is employed for intra-day scheduling to lower the charge and expenses.
1.3 Contributions and organization of the paper
The contribution of the proposed work is two-fold. First, the technical and economic perspectives of the proposed work are covered. This paper’s contributions are summarized as follows:
• This paper proposes a cost-effective and energy-efficient solution through energy management modeling of three different load nature multi-layered agent-based hierarchical interconnected and grid-connected microgrids: smart residential microgrid, smart commercial microgrid, and smart industrial microgrid.
• The proposed scheme provides optimal control over personal comfort, pricing, and bilateral power transactions in energy markets by deploying a robust and secure multi-agent system framework in the Python environment.
• The proposed framework develops a deregulated market for real-time and day-ahead signals by providing spot-market and future market environments, respectively.
• User comfort, power efficiency, maximum clean energy utilization, and cost minimization objectives are achieved simultaneously for both models through proposed modified multi-objective gray wolf optimization and modified multi-objective prioritized plug-and-play and knapsack algorithms.
• A cost–benefit analysis of each microgrid in the multi-microgrid paradigm is performed using parameters such as net present value, internal rate of return, return on investment, payback period, and profitability index through various tariff adjustment techniques for renewable energy resources and battery energy storage systems.
The proposed paper is organized as follows: Section 3 provides the approach adopted by researchers to model the suggested framework for each MG and implementation of the proposed study. The results of the proposed work and discussion on it are given in Section 4, and Section 5 presents the conclusion of the proposed work.
2 Methodology
2.1 Modeling of the complete MMG setup
The devices deployed as the fixed load in each MG are energy-efficient, and their financial and functional requirements determine their quantity. The simplex mode of communication (uni-directional communication) occurs between the fixed load device agent and the controller of the respective MGs. Table 1 shows the base load of smart residential microgrids (SRMGs), smart commercial microgrids (SCMGs), and smart industrial microgrids (SIMGs). Time flexible devices (TFDs) are traditionally moved away from peak times to prevent excessive prices and load constraints on their utility with maximum user comfort. These load devices are relocated to the period of extra renewable generation power in the proposed model to achieve maximum RER utilization and reduce energy curtailment. Furthermore, power flexible devices (PFDs) are optimally set in a rated range of power through an intelligent thermostat. According to the American Society of Heating, Refrigerating, and Air-Conditioning Engineers (ASHRAE) standard 55-2010, intelligent thermostats ensure that AC and HVAC systems function between 20°C and 27°C based on
In PFDs, an intelligent thermostat in HVAC and AC is fed with the
where
From Figures 1A, B, the thermostat’s set point oscillates between the permitted limits of temperature,
To ascertain the value of
In the proposed paper, it has been observed from Eq. 8 that every
2.2 Multi-layer MAS infrastructure for smart MMG setup
A smart residential MG is a basic model for different categories of homes based on its standard of living and load demand based on the personal comfort and poor, rich, and middle classes, as demonstrated in Figure 2A. The configuration is designed for both real-time and day-ahead (DA) MG operations. The real-time approach operates based on hourly signals such as load demand, generation from RERs, and energy price. In contrast, the day-ahead model operates entirely on forecasted data for 24 h. The power needs of the occupants of the mentioned homes with respect to the economic diversity are increased by cost-effective alternatives subject to their affordability and various living patterns. Similarly, a smart commercial MG framework comprises different classifications depending upon the level of power consumed by each scale.
In the proposed work, three commercial-scale, namely, small, medium, and large, setups are considered for both real-time and day-ahead signals, as shown in Figure 2B. With intelligent scheduling of power and time-adaptable devices, commercial modeling addresses the priority assigned to the devices of each commercial scale, cost, and power efficiency. The schedule is done so that the work of each MG category is not disrupted. Shops, markets, buildings, agricultural land, educational institutions, and hospitals (emergency and outpatient door (OPD)) make up the commercial grid load.
In the proposed work, the smart industrial MG setup consists of high-energy-intensive cement and medium-energy-intensive olive oil industries, as shown in Figure 2C. The industrial load is intelligently scheduled through the active participation of agents in the MAS framework. It is a common observation that industries are more vulnerable to the penalties imposed on them for maximum power usage at peak times. Depending upon the feasibility, the industries are not prepared to schedule their load if the cost of not producing at a particular hour exceeds the penalties. However, in our research, industries are allowed to increase production at peak times for clean generation to fulfill the demand. Consequently, both industries achieved cost minimization, reduced production risk, and increased clean energy utilization. Furthermore, the intelligent actions of smart agents assist in achieving the industry’s routine productivity and profitability matters. The MAS framework deploys advanced communication patterns and low latency rates which edify the agents to achieve their distributed objectives in a complex and secure environment. In addition, a secure transport protocol, namely, inter-process communication (IPC), is deployed for connecting agents. The modeled MAS in the proposed paper for each of the three MGs is of a hierarchical type divided into three levels.
2.2.1 Layer 3
A home agent controller (HAC) employs a request–reply communication pattern for asking about the details from the load agent devices in SRMGs. Load agent devices use the push–pull communication pattern to send the necessary information for scheduling to the HAC. Similarly, a Commercial agent controller (CAC) and an industrial zone controller (IZC) in SCMGs and SIMGs send and receive queries regarding the load details to categorized areas and zones using the request–reply and push–pull communication patterns. The HAC, CAC, and IZC in SRMGs, SCMGs, and SIMGs deploy MMGWO and modified multi-objective-prioritized plug-and-play (MMPPnP) algorithms for day-ahead and real-time signals, respectively, to achieve the defined objectives, . From Eqs 9–11,
Load-device agent controllers intelligently transition the mode of connected devices based on the defined restrictions in Eqs 13, 14.
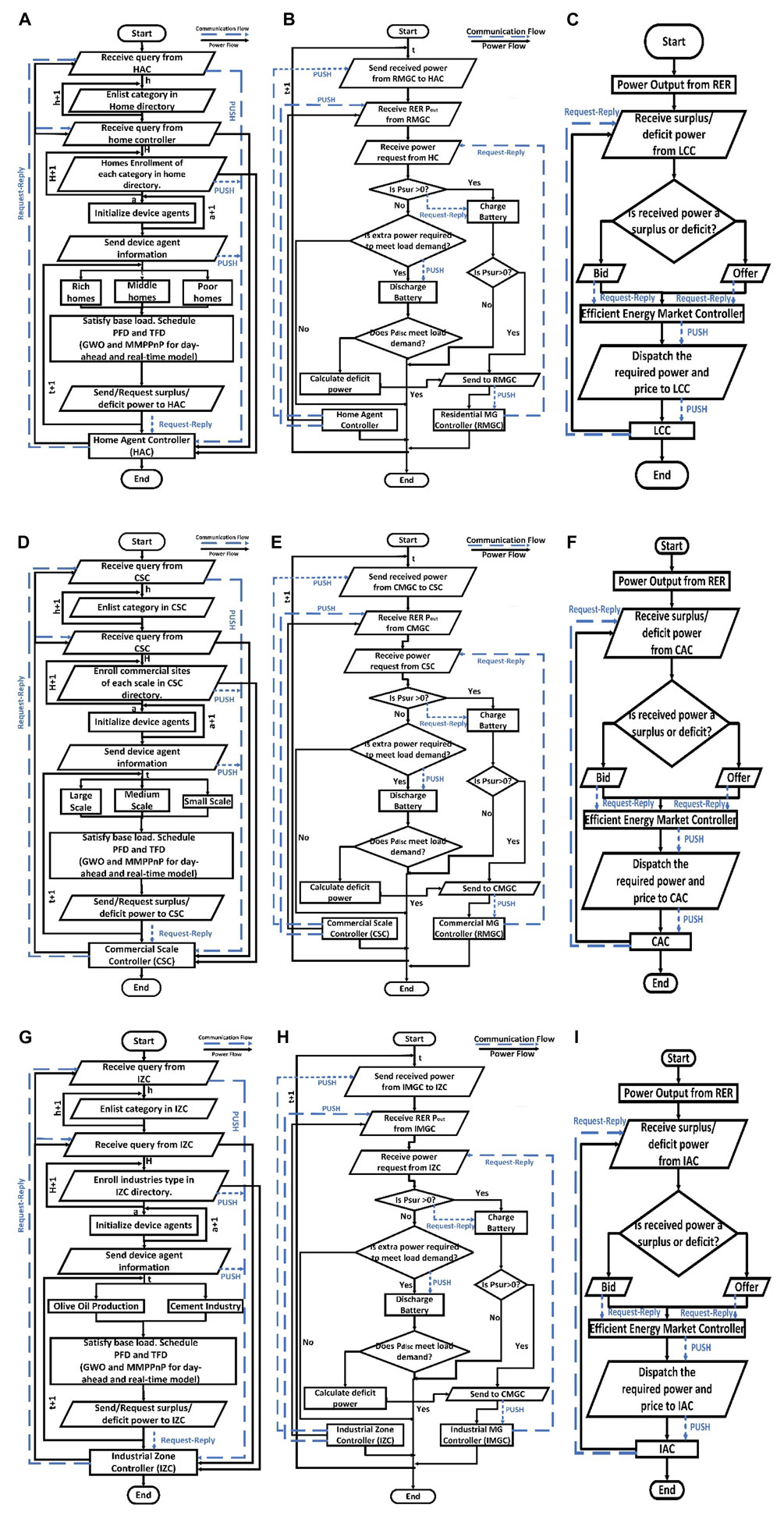
FIGURE 3. Workflow of SRMGs: (A) layer 3; (B) layer 2; (C) layer 1. Workflow of SCMGs: (D) layer 3; (E) layer 2; (F) layer 1. Workflow of SIMGs: (G) layer 3; (H) layer 2; (I) layer 1.
2.2.2 Layer 2
This layer comprises a local community controller (LCC) in SRMGs, CAC in SCMGs, and IAC in SIMGs. Each MG constitutes the BESS with different capacities in the proposed paper. Because of the intricate numerical computations and intelligent decisions executed by the agents in this layer, it has core importance. Layer 2 serves as a link between the consumer and generational levels. Consequently, this layer acts both as a sink and a source across the 24-h operation of agents. Layer 2 controllers for each MG use a request–reply pattern for communication with the BESS, layer 1, and layer 3. At each time period (per hour), the layer 2 controllers obtain power consumption and power generation signals simultaneously from the respective MGs. Controllers calculate the demand/generation power difference from the received power signals and decide the power status using a knapsack algorithm. Priorities are assigned to receiving power utilization by the LCC, CAC, and IAC, as shown in Eq. 15, in the proposed research. The operation of devices is explained based on a four-tiered priority list assigned by the PPnP. Figures 3B, E, H show the workflow of layer 2 in SRMGs, SCMGs, and SIMGs, respectively. The mathematical representation of power at layer 2 is represented in Eqs 16, 17:
2.2.3 Layer 1
Layer 1, as shown in Figures 3C, F, I, acts as a gateway among RERs, MGs through the energy market, and level 2 systems. Layer 1 constitutes the Residential micro-grid controller (RMGC) in SRMGs, Commercial micro-grid controller (CMGC) in SCMGs, and Industrial micro-grid controller (IMGC) in SIMGs. Additionally, solar agents (SAs) and wind agents (WAs) are also deployed in layer 1. RMGC, CMGC, and IMGC are the primary controllers in layer 1 and are involved in maximizing the efficiency and utilization of RERs of the respective MGs. Furthermore, it actively participates in energy trading with the primary grid and other nearby microgrids on the surplus and deficit power. In the case of
where
From Eq. 25, the speed of wind at real-time
2.3 BESS
To encounter the volatile nature and ensure maximum utilization of the output power of RERs, we accommodate the BESS to supply supplementary services. The BESS stores surplus energy at the time of maximum or surplus generation and uses it afterward to satisfy the required demand during minimal generation or emergencies. Additionally, the cost of power is minimized by ignoring the power import from another microgrid or the primary grid. The BESS communicates with the layer 2 controller of each MG through a secure and fast channel using the push–pull communication pattern. As given in Eq. 15, the proposed paper has given the BESS the third priority for employing the RER output power. The battery deployed in the proposed paper is a lithium-ion battery (LIB). Lithium-ion batteries are most popular because of their high energy density, extended lifespan, and high cost and performance efficiency (Hannan et al., 2017). The agent associated with the BESS effectively monitors the performance measuring the parameters such as state-of-charge (SOC), depth-of-discharge (DoD), and round-trip-efficiency (RTE) and ensures the safe and reliable operation of the battery. For per hour along with 24 h, the state-of-charge of the battery is determined from the net power
Only the power capacity
2.4 Framework and algorithms
The proposed algorithm intends to achieve goals such as prioritizing and optimizing green energy utilization, increasing user comfort, and lowering consumer bills and greenhouse gas emissions. This suggested framework is unique, in that it achieves all of the objectives at the same time, delivering a futuristic and practical approach to the multi-microgrid paradigm.
2.4.1 Environment for modeling and developing the MAS framework
A Python-based unique and adaptive environment is developed for operating the MAS in the current work. osBrain is an OpenSistemas-designed Python-based library employed for creating and communicating agents in an MAS. osBrain facilitates the configuration of agents to adopt durable, innovative, and dynamic complex multi-hierarchical formations. The agents deployed using this library operate in real-time, autonomously, with vital intelligence, effective control, and safe communication capabilities. For agents to communicate information in an MAS, the brain provides basic communication patterns such as push–pull, request–reply, and publish–subscribe, as well as sophisticated communication patterns such as the asynchronous request–reply channel and the synced publish–subscribe channel. The developed MAS framework deploys scenario-based communication, as mentioned above. Inter-process communication is a transport protocol for communication and data or power interactions among agents. Furthermore, osBrain uses many Python packages such as NumPy, SciPy, time, and Matplotlib for visual analysis and numerical data computation. To avoid unwanted organizations from compromising the MAS, osBrain provides secure and trusted networks for the agents to share information. The proposed MAS in the MMG paradigm is developed with the assistance of the osBrain module by employing multiple communication methods and networks depending on circumstances. The concept of object-oriented programming (OOP) in Python is also deployed for accessing programmed functions of agents in real-time scenarios.
2.4.2 Gray wolf optimization
Gray wolf optimization (GWO), introduced in Mirjalili et al. (2016), is one of the most effective meta-heuristic optimization algorithms. Because of the extreme precision of the solution, the reduced computing strain, and the minimization of slow convergence, GWO leads to other meta-heuristic algorithms such as the genetic algorithm (GA), African buffalo optimization (ABO), particle swarm optimization (PSO), binary particle swarm optimization (BPSO), and central force optimization (CFO) (Haseeb et al., 2020). This meta-heuristic algorithm mimics gray wolves’ natural command structure and hunting procedure. The alpha wolf position is regarded as the fittest and best solution in the proposed MMGWO with the following objective functions:
2.4.3 Modified multi-objective prioritized plug-and-play
Modified multi-objective-prioritized plug-and-play is an iterative method that, at each iteration, produces the best result under the defined limitations, which is then fed to the controllers for deployment. The objective of this approach is to effectively utilize the RER generation, reduce consumer bills, maximize the user’s comfort, and increase the power efficiency of the devices. For achieving these objectives, certain conditions are imposed on the controller. Consequently, an intelligent controller makes autonomous decisions while considering the constraints that lead to plug-in/plug-out devices. The proposed research implements MMPPnP in a real-time model for 24 h with per-hour signals. Figure 4A explains the working flow of MMPPnP. The objectives of the proposed work are given below.
2.4.4 Knapsack
To reduce costs and emissions while increasing UC and RER utilization, generation and storage in the algorithm are carried out according to the demand dispatch and DR pattern scheduling at the chosen periods using the multiple knapsack problem (MKP) criteria (Zhong et al., 2022). MKP deployment for resource allocation with “m” resources (capacities), a set of “n” objects, and “j” number of knapsack maps projected the problem accordingly (Karimi et al., 2023). The proposed work develops a constraint to limit the power usage based on the load profile and its states given in Eqs 34, 35:
where
where
2.4.5 Efficient energy management market
The conventional infrastructure only enables a one-direction power flow from the generator to the end user, without any response. On the other hand, modern advanced grids provide bi-directional power and communication flow to adjust the load and generation. Consequently, the cost is reduced, thus achieving one of the primary goals of the proposed work. Different strategies have been explored in the literature review for energy transactions with the MG and main grid within the MMG paradigm. The proposed work in this paper provides a realistic alternative to accomplish a solution concerning the technical, economical, and environmental concerns. Because each MG in the MMG paradigm retains RERs, the efficient energy management market controller (EEMMC) primarily transacts clean energy. For this reason, importing of energy from the primary grid is minimized and GHG emission is reduced likewise. The main grid is the least priority for transactive energy in the proposed work. The EEMMC asks each MG in the MMG about the status of energy and cost. Each MG GAC provides information according to the need of an hour or day. The provided information is secured with the EEMMC and is simultaneously shared by each MG. Depending upon the proposed model in this paper, the agent adopts a uniform clearing price and bilateral trading for real-time and day-ahead models in spot-market and future market environments, respectively. In the current project, a double-sided bidding method is being used to make the market more competitive. The proposed efficient energy market workflow is shown in Figure 4B.
3 Results and discussion
A proposed MMG paradigm in the current work incorporates residential, commercial, and industrial MGs. SRMGs comprise five rich-, seven middle-, and 12 poor-class homes, categorized on the basis of appliances. The SCMG is classified into large, medium, and small-scale commercial areas covering different appliances installed in commercial markets, educational institutes, hospitals, offices, and agricultural land. Cement and olive oil production are the sectors of the industrial grid that use high and medium energy levels, respectively. All the three MGs are integrated within an MAS environment through modern communication patterns. Two models, day-ahead and real-time models, are presented based on the time signal received. The model proposed in this paper is designed and programmed so that it tends to be effectively adopted according to each MG’s needs and planning. The business-as-usual (BAU) load represents the consumer’s unscheduled device usage or not optimized load pattern.
3.1 RTM for the MMG
3.1.1 RTM cases for SRMGs
The proposed model in the paper is built by considering different installed RER capacities, as given in Table 2, for SRMGs. Agents in the real-time model (RTM) receive hourly signals for the MMG paradigm’s operation. In an uncertain environment, the agents perform an intelligent action to optimize the operation of each MG and accomplish the defined objectives. Figure 5A represents a base case of hourly operation of SRMGs. Figure 5A depicts that as the peak generation of the introduced RER is more prominent than the maximum load, the imported power by SRMGs is low in contrast to its exported power. The initial hours of power deficiency are satisfied by the BESS. Due to the non-availability of power in the energy market, power is imported from the main grid to satisfy the load demand at hours 19–23 by the bidding price mentioned in Table 2. Similarly, surplus power is exported to SCMGs at hour 5 and the main grid at hours 5–17 through the energy market, with offer price shown in Table 2. Furthermore, Figure 5A demonstrates the effective scheduling of the total load by MMPPnP considering the generation curve and incoming tariff as compared to the unscheduled BAU load. As shown in Figures 5B, C, the TFD and PFD agents turn ON/OFF devices at the ON-peak and OFF-peak hours of generation. The device status, ON and OFF, is represented by 0 and 1, respectively. Status 1 and status 2 for PFDs correspond to the minimum and maximum thermostat levels, respectively. The thermostat is set at the maximum level at ON-peak hours of generation, resulting in maximum user comfort with minimum cost. In contrast, the thermostat level is changed to status 1 at OFF-peak RER generation hours.
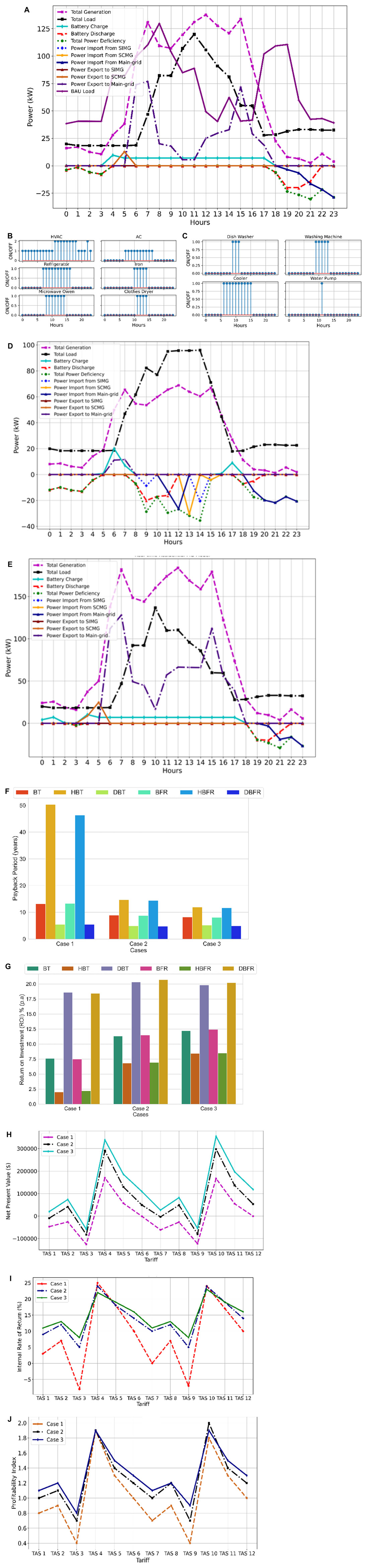
FIGURE 5. Illustration of the RTM of SRMGs: (A) base case power flow; (B) and (C) base case status of schedule devices; (D) case 1; (E) case 3; CBA of the proposed tariff; (F) PBP; (G) ROI; CBA of the proposed tariff adjustment strategy (TAS); (H) NPV; (I) IRR; (J) PI.
Figure 5D is the graphical representation of case 1 of SRMGs. Figure 5D depicts that RERs cannot meet the scheduled load demand during OFF-peak generation hours. As a result, initially, limited power is taken from batteries. Then, the SRMG approaches the energy market to meet the remaining load demand. Due to the volatile nature of the market, power import from the main grid is more than that of other MGs. Agents deployed in SRMGs intelligently optimize thermostat levels for PFDs at low generation hours. Due to the high installed capacity, as given in Table 2, the SRMG exports more energy in case 3, resulting in negligible energy import as compared to case 1 and case 2. Because of enough surplus power, as shown in Figure 5E, the thermostat of PFDs is positioned to a maximum level, reflecting the efficient working of agents. The energy transactions for case 3 can be visualized in Figure 5E. Due to the unavailability of power in the market, the load demand from hours 21 to 23 is satisfied by the main grid. The monthly bill for the schedule load and BAU load pattern is calculated by considering the cost of the power taken from the RER, battery, and other MGs. It can be seen from Table 2 that the monthly bill for scheduled load is lower than that for the unscheduled load in all three cases of renewable power generation. This reflects the compelling and optimal load scheduling by deploying the proposed MMPPnP in RTM SRMGs. The increasing pattern of the schedule load cost in case 2 and case 3 shows the intelligent setting of the thermostat by agents for PFDs. Similarly, the decreasing trend of the BAU load shows the availability of more surplus power in subsequent cases.
At any hour, power is taken from RERs, batteries, and other renewable MGs (SCMGs and SIMGs) to meet the load demand by avoiding the grid, equivalent to reducing carbon emissions. Table 2 shows the emissions of SRMGs for the three cases of renewable energy generation. Table 2 depicts a tremendous decrease in carbon emissions if a load is satisfied from renewable sources compared to meeting the same. The cost–benefit analysis of SRMGs is determined by considering different parameters which are calculated to reference the investors or IPPs for implementing the proposed framework. The proposed tariff breakdown for the proposed model is given in Table 11. The Base TOU tariff is decided based on the solar FiT set for local residential areas in Pakistan. Half-base and double-base TOUs represent the half and double tariff of the base TOU, respectively. The base flat rate is the average sum of the base TOU ON and OFF-peak generation tariff.
As demonstrated in Figures 5A, F, the sensitivity analysis for the proposed model has determined the payback period for different cases. Figure 5F depicts that case 1 has a more extended payback period for different tariffs than cases 2 and 3. Due to the lowest tariff charge by the investor for selling power, as given in Table 3, the half-base TOU and half-base flat rate tariffs in each scenario have a more extended payback period. Because of the significant investment and low demand fulfillment from RERs, these two tariffs are considered invalid for case 1. On the contrary, double-base TOU and double-base flat rate tariffs exhibit the best results for selecting tariffs in each case. The best outcome for all the instances is provided by case 3, which has a short payback period for all tariffs. This results from RER electricity being used to its fullest potential to meet the load demand.
The annual return-on-investment for each of the three RTM SRMG cases is shown in Figure 5G. The ROI for half-base TOU and half-base flat rate tariff, observed in Figure 5G, is considered to be the lowest in all cases. In contrast, double-base TOU and the double-base flat rate have the highest ROI values for different capacities of RERs. Case 1 in Figure 5G is assessed as infeasible for the investors as it has the lowest ROI. This is due to the increase in power import from other MGs and the main grid to meet the demand. On the other hand, case 3 is considered the most feasible as it has the highest values of ROIs for different tariffs. The current work proposes different tariff adjustment strategies given in Table 3 to calculate the NPV, PI, and IRR for different installed capacities of RERs. Figure 5H illustrates the NPV of SRMGs by adopting various tariff adjustment strategies (TASs) for different cases. It can be seen in Figure 5H that TAS 3 and TAS 9 have the lowest NPV, but TAS 10 has the best NPV among all situations. Additionally, as seen in Figure 5I, IRR is kept to a minimum by adhering to TAS 3 and 9. The adoption of TAS 4, however, results in the greatest IRR. The highest and lowest IRRs between these cases can be seen in case 1. Case 2 has the highest PI through implementing TAS 10, as shown in Figure 5J. However, RER installation, as in case 1, is infeasible to pursue as its PI is less than 1 in most TASs.
3.1.2 RTM cases for SCMGs
The RTM of SCMGs is evaluated through an acquaintance of different scenarios and their economic assessment. Therefore, the load demand is maintained throughout. Different cases are taken into consideration based on the capacity of the installed RER, as represented in Table 4. RERs with 1000 kW capacity are installed for the base case of SCMGs. It can be seen from the graphical representation of SCMGs, in Figure 6A, that power export is maximum at the ON-peak hour of generation. In contrast, power is initially imported from the battery and then the SCMG approaches to the energy for meeting the scheduled load. At power deficit hours 3, 4, 5, 18, and 19, power is imported from SRMGs and SIMGs by bidding the price given in Table 4. The corresponding signal is communicated to the efficient energy market in the RTM for every hour with excess or lack of power. The SCMG places an offer and bid for the corresponding power through the communicated signal.
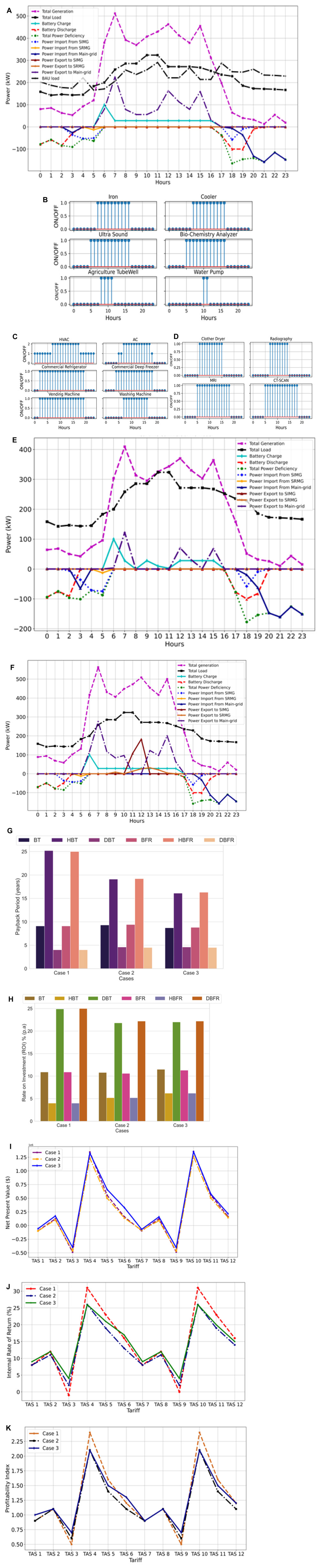
FIGURE 6. Illustration of the RTM of SCMGs: (A) base case power flow; (B), (C), and (D) base case status of schedule devices; (E) case 1; (F) case 3; CBA of the proposed tariff; (G) PBP; (H) ROI; CBA of the proposed TAS; (I) NPV; (J) IRR; (K) PI.
Figure 6E demonstrates that the load curve is more dominant than the generation curve at off-peak hours for case 1. As a result, initially, limited power is taken from batteries. Then, SCMGs approach the energy market to meet the remaining load demand. As shown in Figure 6E, deficiency is satisfied by taking power from the SIMG at hours 3, 4, 5, 18, and 19. Similarly, the SRMG provides energy to the SCMG at hour 5, but the market clears at an industrial bid. Thus, the SRMG experiences social welfare benefit from the market environment. Power is taken from the main grid at 3, 4, 18, 19, 20, 21, 22, and 23 h due to insufficient power to satisfy the load. Due to the volatile nature of the market, power import from the main grid is more than that of the other MGs.
Case 3 consists of RERs with the highest capacity as provided in Figure 6F. Consequently, the thermostat is tuned to a maximum level and maximum energy is exported to other MGs as compared to other cases. Consequently, less electricity is purchased from the energy market than in the other scenarios. At load surplus hours of 9, 11, 12, 13, and 14, power is exported to SIMGs and SRMGs at the offer price shown in Table 4. The cost of the electricity used from RERs, batteries, and other MGs is considered while calculating the monthly bill for the schedule load and the BAU load. Scheduled load cost in case 3 is the lowest, as shown in Table 4. This is due to the minimum power import from other MGs and the maximum utilization of clean energy. The low cost of the schedule load shows the efficient and effective load scheduling achieved by implementing the suggested MMPPnP and knapsack in RTM SCMGs. Carbon dioxide emission by taking power from the main grid is minimized by importing power from RERs, batteries, and other MGs with RERs. Emissions from SCMGs satiating the same demand from the main grid and RERs are demonstrated in Table 4. It can be seen from Table 4 that there is a prominent decrease in emissions that can be achieved through the proposed work.
For executing the proposed framework for SCMGs, specific metrics of CBA are calculated to pave the way for investors or IPPs. Three scenarios are put into consideration based on the capacity of the installed RER. As shown in Figure 6G, the suggested model’s sensitivity analysis was used to evaluate the payback period for the defined cases. It can be analyzed from Figure 6G that there is a prominent decrease in PB for different cases by adopting half-base TOU and the half-base flat rate tariff. Furthermore, by introducing additional tariffs, PB also reduces the ascendingly arranged cases. The primary factor behind the decline in PB is the maximum use of clean energy. However, the capital and operating costs of a large number of RERs cause the PB of the installed setup to be delayed, even though clean energy usage is constant. Case 1 in Figure 6H has the lowest and highest ROI by deploying half-base TOU, half-base flat rate, double-base TOU, and double-base flat rate tariffs, respectively. This is because of the low initial and operation costs and maximum utilization of clean energy. It can be seen from Figure 6I that adopting TAS 4 and TAS 10, given in Table 4, achieves the highest NPV for SCMGs. Moreover, case 1 has the highest IRR for TAS 4 and TAS 10, as demonstrated in Figure 6J. The PI for different cases by deploying various TASs is illustrated in Figure 6K. The analysis in Figure 6K reveals that case 1 achieved the highest PI by using TAS 4 and TAS 10 in the RTM for SCMGs.
3.1.3 RTM cases for SIMGs
Table 5 shows the values of installed RERs, which are taken into consideration for the evaluation of different cases of the RTM for SIMGs. The capacity of the installed RER for case 3 is the highest among other cases. Due to the highest capacity of the installed RER in SIMGs, the generation curve is highly dominant along 24 h, as shown in Figure 7A. Consequently, the SIMG experiences no power deficiency as the total scheduled load demand is met by the installed RER. Due to high surplus power, the maximum power is exported to nearby MGs and the main grid by offering it in the energy market at the price given in Table 5. Figure 7 (B–D) shows the optimal scheduling of devices in SIMGs by deploying MMPPnP.

FIGURE 7. Illustration of the RTM of SIMGs: (A) base case power flow; (B), (C), and (D) base case status of schedule devices; (E) case 1; (F) case 2; CBA of the proposed tariff; (G) PBP; (H) ROI; CBA of the proposed TAS; (I) NPV; (J) IRR; and (K) PI.
The energy flow in SIMGs for case 1 by deploying the RTM is represented in Figure 7E. It can be seen from Figure 7E that most of the load is satisfied by clean energy, and the remaining surplus power is communicated to the energy market for trading. However, at hours 11 and 12, the SIMG approaches SCMGs to satisfy the scheduled load. Further power deficiency is satisfied by the main grid at a high tariff. Case 2 has the lowest capacity for the installed RER and is illustrated in Figure 7F. Due to the low power provided by RERs at hours 11 and 12, the SIMG approaches the energy market where the SCMG is the only provider of energy. As a result, the SIMG imports power from the SCMG at a low price by avoiding the high price main-grid energy import, as given in Table 5. The monthly bill of the scheduled and BAU load for SIMGs is provided in Table 5. Case 2 has the lowest monthly bill due to the low price of power import from the SCMG and SIMG. While in other cases, the low power is satisfied by importing power from the main grid mostly. Furthermore, PFDs in the SIMG operate at a low-rated power at hours of low generation. Consequently, the PFD in case 3 operates at the maximum rated power and increases the bill cost.
By importing energy from RERs, batteries, and other MGs with RERs, carbon dioxide emissions caused by using the power from the main grid are reduced to a minimum. Table 5 shows emissions from the SIMG satisfying the same demand from the main grid and RERs. As given in Table 5, the proposed work has the potential to reduce emissions significantly. Specific CBA parameters are estimated based on the capacities of the installed RERs to provide an idea for investors or IPPs in executing the suggested framework for SIMGs. Different CBA parameters are calculated for the proposed tariff given in Table 5.
The payback period for different cases of the SIMG is illustrated in Figure 7G. It can be seen from Figure 7G that case 3 has the shortest PB. This is due to the maximum utilization of RERs by the SIMG and low power import. Consequently, ROIs for different tariffs of case 3 are maximum compared to other cases shown in Figure 7H. The NPV for the RTM SIMG, given in Figure 7I, shows that case 3 shows a dominant difference among different cases for various TASs suggested in Table 5. Figure 7J shows that case 1 has a high IRR compared to case 3 by adopting TAS 4 and 10. However, case 3 gives a high IRR for the rest of TASs. As seen in Figure 7K, case 3 is the most feasible, whereas case 2 is the least feasible to pursue for different TASs.
3.2 Day-ahead model for the MMG paradigm
The DAM’s operation is based upon the forecasted data where DA signals are communicated only once to the agents at the start of each day. The monthly bill for the base case of the DAM in the MMG paradigm is calculated and is given in Table 6, i.e., MMGWO for the DAM efficiently minimizes the cost of load consumption by effective scheduling of the load as compared to the BAU. Deployed intelligent agents efficiently optimize the signals and plans intelligently for achieving defined objectives. Figure 8A visualizes the energy flow operation by deploying the DAM in the SRMG, and it is observed that the maximum load is scheduled at signal hours of low tariff and maximum renewable energy generation. At hours of maximum surplus, the battery is charged first, and then the remaining surplus power is sent to the market for energy transactions. Furthermore, Figures 8B, C show the power flow of SCMGs and SIMGs over 24 h, respectively. During hours 5–17, the RER generation is greater than the load scheduled, and the thermostat is adjusted to a maximum level, and maximum number of devices are turned ON to minimize the cost and maximize the UC. The comparative analysis of proposed tariffs and their adjustments are shown in Figures 8D–E and Figures 8F–H, respectively.
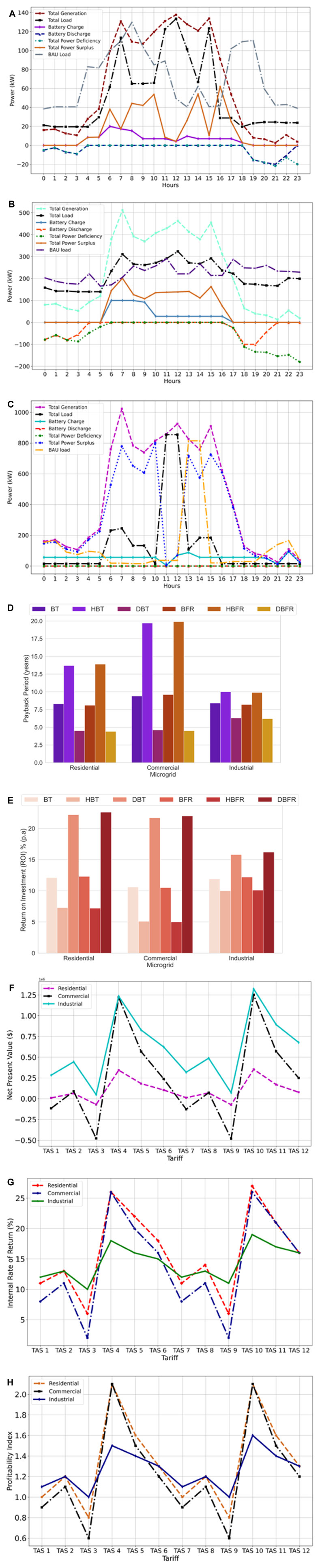
FIGURE 8. Power flow of the DAM base case of (A) the SRMG; (B) SIMG; (C) SCMG, CBA of the proposed tariff for MMGs; (D) PBP; (E) ROI, CBA of the proposed TAS for MMGs; (F) NPV; (G) IRR; and (H) PI.
4 Conclusion
The proposed work develops an intelligent and efficient MAS framework in three different load-based MGs in the MMG paradigm. An adequate communication infrastructure among agents is implemented to complete the distributed tasks smartly. The proposed work aims to maximize RER utilization, reduce monthly consumer bill, maximize user-comfort, and reduce emissions. MMPPnP, MMGWO, and knapsack are deployed concurrently with the demand dispatch and demand response techniques for real-time and day-ahead signals to obtain optimum results for the defined objectives. Intelligent thermostat agents set the optimal temperature of the HVAC and AC according to the ASHRAE standard 55-2010 depending upon the incoming
Data availability statement
The original contributions presented in the study are included in the article/Supplementary Material; further inquiries can be directed to the corresponding authors.
Author contributions
KM: formal analysis, investigation, software, and writing–original draft. AA: funding acquisition, methodology, resources, supervision, validation, and writing–original draft. SK: conceptualization, formal analysis, methodology, resources, and writing–original draft. ZK: conceptualization, data curation, formal analysis, funding acquisition, supervision, and writing–review and editing. BA: investigation, project administration, software, validation, visualization, and writing–review and editing. HAla: investigation, project administration, resources, validation, visualization, and writing–review and editing. HAls: data curation, project administration, resources, visualization, and writing–review and editing. AM: data curation, funding acquisition, methodology, and writing–review and editing.
Funding
The author(s) declare that financial support was received for the research, authorship, and/or publication of this article. The authors extend their appreciation to the Deputyship for Research and Innovation, Ministry Education in Saudi Arabia, for funding this research work through the project number (IFP-2022-44).
Conflict of interest
The authors declare that the research was conducted in the absence of any commercial or financial relationships that could be construed as a potential conflict of interest.
Publisher’s note
All claims expressed in this article are solely those of the authors and do not necessarily represent those of their affiliated organizations, or those of the publisher, the editors, and the reviewers. Any product that may be evaluated in this article, or claim that may be made by its manufacturer, is not guaranteed or endorsed by the publisher.
Abbreviations
MM, modified multi-objective; DD, demand dispatch; DR, demand response; MAS, multi-agent system; L, M, and S: large, medium, and small; SRMG, smart residential microgrid; SCMG, smart commercial microgrid; SIMG, smart industrial microgrid; R, M, and P: rich, middle, and poor;
References
Ahmadi, S. E., and Rezaei, N. (2020). A new isolated renewable based multi microgrid optimal energy management system considering uncertainty and demand response. Int. J. Electr. Power and Energy Syst. 118, 105760. doi:10.1016/j.ijepes.2019.105760
Alam, M. N., Chakrabarti, S., and Liang, X. (2020). A benchmark test system for networked microgrids. IEEE Trans. Ind. Inf. 16 (10), 6217–6230. doi:10.1109/tii.2020.2976893
Ali, S. A., Hussain, A., Haider, W., Rehman, H. U., and Kazmi, S. A. A. (2023). Optimal energy management system of isolated multi-microgrids with local energy transactive market with indigenous PV-Wind-and biomass-based resources. Energies (Basel) 16, 1667. doi:10.3390/en16041667
Bui, V., Member, S., Hussain, A., and Member, S. (2018). A multiagent-based hierarchical energy management strategy for multi-microgrids considering adjustable power and demand response. IEEE Trans. Smart Grid 9 (2), 1323–1333. doi:10.1109/tsg.2016.2585671
Choobineh, M., and Mohagheghi, S. (2016). A multi-objective optimization framework for energy and asset management in an industrial Microgrid. J. Clean. Prod. 139, 1326–1338. doi:10.1016/j.jclepro.2016.08.138
Cortes, C. A., Contreras, S. F., and Shahidehpour, M. (2017). Microgrid topology planning for enhancing the reliability of active distribution networks. IEEE Trans. Smart Grid 9 (6), 6369–6377. doi:10.1109/tsg.2017.2709699
Dong, X., Li, X., and Cheng, S. (2020). Energy management optimization of microgrid cluster based on multi-agent-system and hierarchical stackelberg game theory. IEEE Access 8, 206183–206197. doi:10.1109/ACCESS.2020.3037676
Guo, Z., Pinson, P., Chen, S., Yang, Q., and Yang, Z. (2021). Online optimization for real-time peer-to-peer electricity market mechanisms. IEEE Trans. Smart Grid 12 (5), 4151–4163. doi:10.1109/tsg.2021.3075707
Hannan, M. A., Lipu, M. S. H., Hussain, A., and Mohamed, A. (2017). A review of lithium-ion battery state of charge estimation and management system in electric vehicle applications: challenges and recommendations. Renew. Sustain. Energy Rev. 78, 834–854. doi:10.1016/j.rser.2017.05.001
Haseeb, M., Kazmi, S. A. A., Malik, M. M., Ali, S., Bukhari, S. B., and Shin, D. R. (2020). Multi objective based framework for energy management of smart micro-grid. IEEE Access 8, 220302–220319. doi:10.1109/ACCESS.2020.3041473
Hirsch, A., Parag, Y., and Guerrero, J. (2018). Microgrids: a review of technologies, key drivers, and outstanding issues. Renew. Sustain. Energy Rev. 90, 402–411. doi:10.1016/j.rser.2018.03.040
Husein, M., and Chung, I.-Y. (2018). Optimal design and financial feasibility of a university campus microgrid considering renewable energy incentives. Appl. Energy 225, 273–289. doi:10.1016/j.apenergy.2018.05.036
IEA (2020). Global Energy Review 2019 - the latest trends in energy and emissions in 2019. Glob. Energy Trends, 1–50.
Karimi, H., Gharehpetian, G. B., Ahmadiahangar, R., and Rosin, A. (2023). Optimal energy management of grid-connected multi-microgrid systems considering demand-side flexibility: a two-stage multi-objective approach. Electr. Power Syst. Res. 214, 108902. doi:10.1016/j.epsr.2022.108902
Karimi, H., and Jadid, S. (2023). Multi-layer energy management of smart integrated-energy microgrid systems considering generation and demand-side flexibility. Appl. Energy 339 (Jun), 120984–122023. doi:10.1016/j.apenergy.2023.120984
Khalid, R., Javaid, N., Rahim, M. H., Aslam, S., and Sher, A. (2019). Fuzzy energy management controller and scheduler for smart homes. Sustain. Comput. Inf. Syst. 21, 103–118. doi:10.1016/j.suscom.2018.11.010
Liang, Z., Bian, D., Zhang, X., Shi, D., Diao, R., and Wang, Z. (2019). Optimal energy management for commercial buildings considering comprehensive comfort levels in a retail electricity market. Appl. Energy 236, 916–926. doi:10.1016/j.apenergy.2018.12.048
Liu, Y., Wang, Y., Li, Y., Gooi, H., and Xin, H. (2021). Multi-agent based optimal scheduling and trading for multi-microgrids integrated with urban transportation networks. IEEE Trans. Power Syst. 36 (3), 2197–2210. doi:10.1109/tpwrs.2020.3040310
Malik, M. M., Kazmi, S. A. A., Asim, H. W., bin Ahmed, A., and Shin, D. R. (2020). An intelligent multi-stage optimization approach for community based micro-grid within multi-microgrid paradigm. IEEE Access 8, 177228–177244. doi:10.1109/ACCESS.2020.3022411
Mirjalili, S., Saremi, S., Mirjalili, S. M., and Coelho, L. dos S. (2016). Multi-objective grey wolf optimizer: a novel algorithm for multi-criterion optimization. Expert Syst. Appl. 47, 106–119. doi:10.1016/j.eswa.2015.10.039
Naderi, M., Bahramara, S., Khayat, Y., and Bevrani, H. (2017). Optimal planning in a developing industrial microgrid with sensitive loads. Energy Rep. 3, 124–134. doi:10.1016/j.egyr.2017.08.004
Oueid, R. K. (2019). Microgrid finance, revenue, and regulation considerations. Electr. J. 32 (5), 2–9. doi:10.1016/j.tej.2019.05.006
Purage, L., Isuru, M., Paudel, A., Nguyen, H. D., and Foo, E. Y. S. (2022). Peer-to-Peer energy trading enabled optimal decentralized operation of smart distribution grids. IEEE Trans. Smart Grid 13 (1), 654–666. doi:10.1109/TSG.2021.3110889
Rajaei, A., Fattaheian-dehkordi, S., Fotuhi-firuzabad, M., and Moeini-Aghtaie, M. (2020). Decentralized transactive energy management of multi-microgrid distribution systems based on ADMM,” Int. J. Electr. Power Energy Syst. 132, 107126. doi:10.1016/j.ijepes.2021.107126
Ramadan, M., Mohamad, B., Logenthiran, T., Naayagi, R. T., and Woo, W. L. (2019). A nano-biased energy management using reinforced learning multi-agent on layered coalition model: consumer sovereignty. IEEE Access 7, 52542–52564. doi:10.1109/ACCESS.2019.2911543
Rezaei, N., Pezhmani, Y., and Khazali, A. (2022). Economic-environmental risk-averse optimal heat and power energy management of a grid-connected multi microgrid system considering demand response and bidding strategy. Energy 240, 122844. doi:10.1016/j.energy.2021.122844
Shi, S., Wang, Y., and Jin, J. (2023). Multi-agent-based control strategy for centerless energy management in microgrid clusters. Front. Energy Res. 11, 2023. doi:10.3389/fenrg.2023.1119461
Systems, M., Bazmohammadi, N., Anvari-moghaddam, A., Tahsiri, A., Vasquez, J., and Guerrero, J. (2020). Stochastic predictive energy management of multi-microgrid systems. Appl. Sci. Stoch. Predict. Energy Manag.10, 4833. doi:10.3390/app10144833
Tan, B., and Chen, H. (2020). Multi-objective energy management of multiple microgrids under random electric vehicle charging. Energy 208, 118360. doi:10.1016/j.energy.2020.118360
Thirugnanam, K., Moursi, M. S. E., Khadkikar, V., Zeineldin, H. H., and Al Hosani, M. (2021). Energy management of grid interconnected multi-microgrids based on P2P energy exchange: a data driven approach. A Data Driven Approach 36 (2), 1546–1562. doi:10.1109/TPWRS.2020.3025113
Ton, D. T., and Smith, M. A. (2012). The US department of energy’s microgrid initiative. Electr. J. 25 (8), 84–94. doi:10.1016/j.tej.2012.09.013
Vu, B. H., Husein, M., Kang, H.-K., and Chung, I.-Y. (2019). Optimal design for a campus microgrid considering ESS discharging incentive and financial feasibility. J. Electr. Eng. Technol. 14 (3), 1095–1107. doi:10.1007/s42835-019-00142-9
Wang, S., Zhang, X., Wu, L., and Sun, S. (2018). New metrics for assessing the performance of multi-microgrid systems in stand-alone mode. Int. J. Electr. Power and Energy Syst. 98, 382–388. doi:10.1016/j.ijepes.2017.12.002
Xu, D., Zhong, F., and Bai, Z. (2023). A two-layer multi-energy management system for microgrids with solar, wind, and geothermal renewable energy. Front. Energy Res. 10 (Jan). doi:10.3389/fenrg.2022.1030662
Xu, Z., Yang, P., Zheng, C., Zhang, Y., Peng, J., and Zeng, Z. (2018). Analysis on the organization and Development of multi-microgrids. Renew. Sustain. energy Rev. 81, 2204–2216. doi:10.1016/j.rser.2017.06.032
Yang, X., He, H., Zhang, Y., Chen, Y., and Weng, G. (2019). Interactive energy management for enhancing power balances in multi-microgrids. IEEE Trans. Smart Grid 10 (6), 6055–6069. doi:10.1109/TSG.2019.2896182
Yin, L., and Li, S. (2021). Hybrid metaheuristic multi-layer reinforcement learning approach for two-level energy management strategy framework of multi-microgrid systems. Eng. Appl. Artif. Intell. 104, 104326. doi:10.1016/j.engappai.2021.104326
Zhong, X., Zhong, W., Liu, Y., Yang, C., and Xie, S. (2022). Optimal energy management for multi-energy multi-microgrid networks considering carbon emission limitations. Energy 246, 123428. doi:10.1016/j.energy.2022.123428
Zhuo, Z., Yang, K., Yang, J., Mao, R., and Xue, N. (2019). A multiagent-based hierarchical energy management strategy for maximization of renewable energy consumption in interconnected multi-microgrids. IEEE Access 7, 169931–169945. doi:10.1109/ACCESS.2019.2955552
Zlatkovic, M., Zlatkovic, S., Sullivan, T., Bjornstad, J., and Shahandashti, S. K. F. (2019). Assessment of effects of street connectivity on traffic performance and sustainability within communities and neighborhoods through traffic simulation. Sustain Cities Soc. 46, 101409. doi:10.1016/j.scs.2018.12.037
Keywords: multi-agent systems, multi-microgrid, energy management, day-ahead, real time, intelligent agent multi-agent systems, intelligent agents
Citation: Mujahid K, Altamimi A, Kazmi SAA, Khan ZA, Alharbi B, Alafnan H, Alshehry H and Mir AA (2024) An intelligent multi-layer, multi-agent MMG framework with amelioration of energy efficiency and future investment outlook, under the deregulated day-ahead and real-time market regime. Front. Energy Res. 12:1348458. doi: 10.3389/fenrg.2024.1348458
Received: 02 December 2023; Accepted: 04 January 2024;
Published: 01 February 2024.
Edited by:
Muhammad Farooq, University of Engineering and Technology, PakistanReviewed by:
Haroon Farooq, University of Engineering and Technology, PakistanIshak Ertugrul, Mus Alparslan University, Türkiye
Rami Reddy, Chonnam National University, Republic of Korea
Copyright © 2024 Mujahid, Altamimi, Kazmi, Khan, Alharbi, Alafnan, Alshehry and Mir. This is an open-access article distributed under the terms of the Creative Commons Attribution License (CC BY). The use, distribution or reproduction in other forums is permitted, provided the original author(s) and the copyright owner(s) are credited and that the original publication in this journal is cited, in accordance with accepted academic practice. No use, distribution or reproduction is permitted which does not comply with these terms.
*Correspondence: Abdullah Altamimi, a.altmimi@mu.edu.sa; Zafar A. Khan, zafarakhan@ieee.org