- 1Economic and Technology Institute, State Grid Fujian Electric Power Co., Ltd., Fuzhou, China
- 2Distribution Network Planning and Operation Control Technology in Multiple Disaster Superimposed Areas State Grid Corporation Laboratory, Fuzhou, China
- 3School of Electronic Information and Electrical Engineering, Shanghai Jiao Tong University, Shanghai, China
In the process of decarbonization, the configuration of renewable energy and energy storage plays a crucial role. In current research, there is often a singular focus on the isolated optimization of either renewable energy configurations or energy storage configurations, resulting in limitations within the optimized outcomes. Therefore, we propose a collaborative configuration approach for renewable energy and energy storage under fixed investment, considering the impact of uncertainty on optimization results. By employing the W/S (wind-to-solar ratio) and E/P (energy-to-power ratio) and constructing a model with an hourly granularity, we can obtain the configurations of renewable energy and energy storage at crucial time points. Using the UK as a case study, we calculate the configurations for renewable energy and energy storage from 2020 to 2050, offering effective recommendations for the decarbonization efforts in the UK.
1 Introduction
With the advancement of modernizing the power grid, numerous scholars have delved into the exploration of novel power systems from various perspectives. For instance, at the operational and planning levels, Yang pioneered the introduction of an SCUC expert system based on E-Seq2Seq (Yang et al., 2022a) and delved into various methodologies addressing the modeling and solving of SCUC-related issues (Yang et al., 2018; Yang et al., 2022b; Yang e tal. 2022d). Li et al. (2021) focused on the dual-layer operation methodology of multi-energy microgrids. In the realm of intelligent grid operations, Zhang et al. (2023a); Zhang et al. (2023b) proposed an economically optimal strategy considering FDI and DoS attacks. Pertaining to the reliability of renewable energy operations, literature has explored hydroelectric system modeling and reliability (Xu et al., 2023), rolling bearing fault diagnosis (Fu et al., 2023), post-disaster recovery (Li, 2023a; Li, 2023b), and risk-averse restoration (Yang and Li, 2023). In the domain of innovative power system equipment, research has been conducted on transformers and high voltage conversion gain flyback converters (Zhu et al., 2023). Market-related investigations include studies on game theory in multi-energy microgrid energy trading (Li et al., 2023e). In the realm of electrified transportation, literature has investigated multi-objective coordinated energy dispatch and voyage planning for multi-energy ship microgrids (Li et al., 2020). In the face of unprecedented global climate challenges, the imperative to decarbonize our energy systems has never been more pressing (Jafari M et al., 2020; Yang et al., 2022c; Kabeyi and Olanrewaju, 2022). As the world grapples with the consequences of rising greenhouse gas emissions, nations across the globe are committing to ambitious climate targets and setting their sights on a future where carbon neutrality is the norm. The journey towards decarbonization is a multifaceted endeavor that requires a delicate balance of numerous factors, chief among them the integration of renewable energy sources and energy storage solutions (Huber and Weissbart, 2015).
A comprehensive survey of existing literature indicates a primary focus on optimizing configurations in a specific context. Specifically, the research concentrates on optimizing energy storage configurations given known configurations of renewable energy or optimizing renewable energy configurations under the assumption of known energy storage configurations. These studies often examine how the choice of energy storage configurations can be optimized to accommodate a given mix of renewable sources (Arbabzadeh et al., 2019a), how the selection of energy storage configurations can be optimized given a particular mix of renewable sources (Gao et al., 2020), long term energy storage in highly renewable systems (Hargreaves and Jones. 2020)and energy storage assessments under specific conditions (Li et al., 2023c; Li et al., 2023d), or planning of distributed renewable energy systems under uncertainty (Fu et al., 2022). While these approaches have undeniably contributed valuable insights into each respective component of the energy landscape, it has often led to a partial understanding of the broader decarbonization challenge.
For instance, studies focused on optimizing energy storage configurations may not fully account for the variability of renewable energy generation, thus potentially leading to suboptimal solutions. On the other hand, research emphasizing renewable energy deployment strategies without considering energy storage may underestimate the potential benefits of effective storage integration, particularly in stabilizing energy supply and mitigating intermittency issues. Moreover, the majority of these studies tend to operate within deterministic frameworks, which, although informative, may not capture the full spectrum of uncertainties inherent in future energy landscapes. The transition to a low-carbon energy system is fraught with unpredictabilities, including fluctuations in energy demand, the uncertain pace of renewable technology development, and evolving policy and market dynamics. These uncertainties can profoundly influence the effectiveness of decarbonization strategies, and a failure to address them adequately could result in suboptimal outcomes.
In light of these existing research gaps, our study endeavors to transcend these limitations. We adopt a holistic approach that amalgamates both renewable energy and energy storage considerations into a unified framework. Furthermore, we recognize the indispensable need to accommodate uncertainties in our analysis, thus offering a more comprehensive view of the dynamic and multifaceted nature of energy decarbonization. Therefore, this paper will analyze Analysis of coordinated optimization method in decarbonization path from two aspects: (1) The long-term planning of renewable energy and energy storage configurations. (2) The impact of predictive uncertainty and technological uncertainty on coordinated source-load consideration methods. Through our research, we aim to demonstrate the value of an integrated approach and underscore its potential to facilitate the rapid and effective decarbonization of energy systems.
The remaining sections of the article are structured as follows: Section 2 introduces the long-term planning methods for renewable energy and energy storage, Section 3 discusses the constraints that the system needs to satisfy, Section 4 analyzes the impact of various factors on long-term planning, and Section 5 summarizes the work.
2 The long-term planning of renewable energy and energy storage configurations
We employ W/S (wind-to-solar ratio) and E/P (energy-to-power ratio) to characterize the configurations of renewable energy and energy storage. Drawing on data from the Department for Business, Energy and Industrial Strategy (BEIS) in the UK (MacDonald, 2018; BEIS, 2020), all other variables—such as load growth, system expansion, and the projected investment scales for energy storage and renewable energy from 2020 to 2050—remain constant. Capital expenditures and operational costs for both renewable energy and energy storage are factored in, aligning with anticipated future trends.
In contrast to the UK National Grid’s proposed Future Energy Scheme (FES), which independently assesses the ratios of E/P and W/S, our approach concurrently considers both, dynamically adjusting investments in energy storage and renewable energy.
We have developed a linear capacity-planning and electricity dispatch optimization model with hourly temporal granularity, aiming to minimize both operational expenses and carbon emissions for a macro-scale electrical system, as outlined in (1).
where PGeni,t, PPVi,t, PWindi,t represent the power output of the ith conventional generators, solar PV stations and wind farms at hour t; Additionally, Pchs,t and Pdischs,t denote the active power for charging and discharging of the sth energy storage system (ESS) at hour t; the power exchange efficiencies of the sth ESS are denoted as ηch,s and ηdisch,s; operational cost and carbon emission cost for the ith conventional generator are represented by lGeni and lemissioini; lPV and lWind are the operational cost of solar PV stations and wind farms; lESSs is the operational cost of the sth ESS; T is the total number of hours per year, i.e., 8,760.
Concerning capacity planning, the future capacity of wind and solar is dictated by the investment in renewables and the W/S ratio. Similarly, the energy and power capacity of ESS are determined by the ESS investment and the E/P ratio. Furthermore, these capacity variables impose constraints on the hourly operation of renewables and ESSs. Consequently, the optimization of the objective function involves dynamic adjustments to the E/P and W/S ratios.
2.1 Definition of W/S and E/P
The W/S ratio is expressed as
where RatioW/S,j represents the W/S ratio in the yrth year; PWindj and PPVj denote the installed power capacity of wind and solar in the jth year; PWind2020 and PPV2020 signify the installed power capacity of wind and solar in 2020; InvRESj stands for the investment in both wind and solar in the jth year; L represents the lifetime of wind and solar; CWindj and CPVj represent the capital expenditure (CAPEX) cost of wind and solar. The CAPEX cost encompasses all relevant expenses associated with constructing a generation or energy storage system, including pre-development costs, construction costs, and infrastructure costs.
Based on W/S ratio in (2), the hourly power output PPVi,t and PWindi,t should be constrained as shown in Eqs 3, 4:
where AWindt and APVt are the normalised data of wind and solar generation.
To model the hourly operation of future decarbonized electricity systems, it is essential to forecast electricity demand and the variability of renewable sources. However, over such an extended time horizon, accurate predictions become challenging. Therefore, we employ the methodology proposed in (Arbabzadeh et al., 2019b), which utilizes the historical variation patterns of wind, solar, and load in a past year as a representation of future behavior. This involves normalizing the historical data and scaling it by the projected installation capacity or maximum electricity load. The calculation for the amount of wind/solar/load in hour-t is as shown in Eqs 5–7:
where Lmj is the maximum electricity demand in year-j; Bw2019,t, Bs2019,t, and Bl2019,t are the installed capacity of wind and solar and the electricity demand in hour-t in 2019, respectively; Pw 2019, Pw 2019, and Lm2019 refer to the installed capacity of wind and solar and the maximum electricity demand in 2019, respectively.
The E/P ratio is expressed as shown in Eqs 8, 9:
where RatioE/P,j denotes the E/P ratio in the jth year; QESSs,j and PESSs,j represent the energy capacity and power capacity of the sth ESS in the jth year; QESSs, 2020 and PESSs, 2020 signify the energy capacity and power capacity of the sth ESS in 2020; QinvESSs,j and PinvESSs,j indicate the energy capacity and power capacity of the sth ESS in the jth year; Cenergyj and Cpowerj are the energy-related and power-related CAPEX costs of the sth ESS in the jth year.
In our study, we categorize long-duration storage (e.g., pumped hydro energy storage, compressed air energy storage, hydrogen storage) and short-duration storage (e.g., electrochemical energy storage) as ESS. The charging/discharging power and the state of charge (SoC) of ESS must adhere to the following constraints as shown in Eqs 10–13:
where pchs,t and pdischs,t are the real-time active power for charging and discharging of the sth ESS at hour t; the uchs,t and udischs,t are binary variables to indicate the charging and discharging status, which should satisfy (12). The ηch,s and ηdisch,s are the power exchange efficiencies; the SoCs,t is the SoC value of sth ESS at hour t; the SoCmin,s and SoCmax,s are the min/max SoC boundaries of the sth ESS.
2.2 Renewble and storage investment
As the FES does not disclose the annual investment amount, we utilize the estimated capital expenditure cost to approximate the corresponding investment. The Department for Business, Energy, and Industrial Strategy (BEIS) periodically updates cost estimates and technical specifications for various generation technologies in the Electricity Generation Costs. This data breaks down costs into detailed expenditures per MW capacity or MWh generation for the full lifetime of a plant, encompassing planning costs, construction costs, operating costs, and carbon costs (BEIS, 2020).
Both the CAPEX and operational expenditure (OPEX) costs are computed as the Levelized Cost of Electricity (LCOE), defined as the cost per unit of electricity generated (Ioannou et al., 2018; Harvey, 2020). LCOE represents the ratio of the total costs of a generic plant to the total amount of electricity expected to be generated over the plant’s lifetime and can be calculated by Eq. 14:
where the Cf refers to the capacity factor of the specified generator.
We incorporate the operational expenditure (OPEX) cost as the cost coefficient in the objective function. Gas-fired generators stand out as the most expensive energy resources due to the inclusion of carbon emission costs in the OPEX cost.
Following the definition of LCOE, we calculate the investment amount in renewables (i.e., wind and solar) using Eq. 15:
where L is the lifetime of wind and solar which is assumed as 30 years; Cwj and Csj denote the CAPEX cost of wind and solar, respectively. Pwj and Psj are the installation capacity projected in the year of j. Table 1 gives our estimated investment amount.
The investment amount in energy storages is also not revealed in the FES. To optimise the E/P ratio under the same investment, we derived the investment amount by projected cost data. The Storage Cost and Technical Assumptions for BEIS gives the projection of CAPEX and OPEX costs in (Mott, 2018).
However, the BEIS calculates the LCOE based on the power capacity of storage systems. Notably, the costs related to power and energy capacities for various storage technologies exhibit substantial variation. To address this, we conducted an extensive literature review, synthesizing technical and economic characteristics from sources such as (Grünewald et al., 2012; Hill et al., 2012). The California Energy Commission designates long-duration storages with an E/P ratio exceeding 10:1, including Pumped Hydro Storage (PHS), Compressed Air Energy Storage (CAES), and hydrogen storage (California Energy Commission, 2020). Batteries are typically classified as short-duration storages, featuring an E/P ratio lower than 5:1.
Long-duration storages often have a relatively lower energy-related cost (CAPEX cost per kWh of energy capacity) compared to short-duration storages (E/P ratio lower than 10:1). Conversely, the CAPEX cost per kW of power capacity for short-duration storages is generally lower than that for long-duration storages. Additionally, long-duration storages (typically PHS, CAES, and hydrogen storage) can have independently sized power and energy capacities, while separating these capacities can be challenging for batteries.
To project estimated cost data in the future, we adopt the cost reduction projections outlined in the Storage Cost and Technical Assumptions for BEIS. Batteries, due to the rapid advancements in the material industry, show the greatest potential for cost reduction.
Based on this literature review and analysis, we decouple energy-related and power-related costs with their respective reduction trends, leading to our formulated cost assumptions.
The capital investment in energy storage is calculated by Eq. 16:
where Cpi,j and Cei,j refer to power-related and energy-related CAPEX cost of the ith storage technology in year-j; Pesi,j and Qesi,j are the power capacity and energy capacity of the ith storage technology in year-j. Table 2 gives our estimated investment amount in storages. A total of 17.44 billion £ will be invested by 2050, and the investment sharply increases in 2030 because new large-scale storage projects are expected to come online.
Where Cpi,j and Cei,j represent the power-related and energy-related CAPEX costs of the ith storage technology in year-j; Pesi,j and Qesi,j denote the power capacity and energy capacity of the ith storage technology in year-j. Table 2 presents our estimated investment amounts in storages. A cumulative investment of 17.44 billion £ is projected by 2050, with a significant surge in 2030 due to the anticipated launch of new large-scale storage projects.
3 Constraints of system
3.1 Constraints of unit commitment of conventional generators
In this study, we consider the commitment and cycling (start-up, shut-down) of conventional thermal generators. The constraints applicable to thermal units, such as maximum and minimum generation limits, ramping constraints, and minimum on/off time constraints, are also extended to other conventional units.
Maximum and minimum generation limits: Power output for the ith conventional generator includes the ramping constraint as shown in Eq. 17:
where
Ramping constraints: Ramping constraint for the ith conventional generator is defined as follows:
where Rui and Rdi are the ramp-up and ramp-down limits for ith conventional generator.
Minimum on/off time constraints: The minimal on/off time constraints for conventional generator are formulated as follows:
where DTi and UTi represent the minimal on/off time of the ith conventional generator, Gi is the time interval to allow for change in on-off status with respect to the initial conditions.
3.2 System energy balance and reserves constraints
The analysis of power balance and reserve requirements is conducted as follows.
Power balance: The system load is equivalent to the sum of the power output from all generator units, encompassing conventional generators, wind, solar, hydro, and ESS units, as expressed by:
where
Reserve requirements: The uncertainties related to potential errors in forecasting energy demand and wind power generation are considered in determining reserve requirements, outlined as follows:
where
4 Analysis of the impact of uncertainty on system operation costs and energy prices
In this section, we consider the impact of forecasts on coordinated methods, primarily encompassing: (1) The difference between incorporating forecasted results and directly using official forecast data in the model. (2) The influence of forecast uncertainty on coordination pathways. (3) The influence of economic uncertainty on coordination pathways.
4.1 The difference between incorporating forecasted results and directly using official forecast data in the model
We utilized officially published data from 2015 to 2019 to conduct load forecasting, integrating the forecasted results into our model for deriving the decarbonization pathway for the UK. Following this, we replaced the official forecast data and conducted a comparative analysis. As illustrated in Figure 1, we observed a nearly identical pattern of change in optimization results between the two scenarios. Additionally, we compared the optimization results of our model with the CO2 emissions from the UK’s FES pathway, considering the lower carbon emissions achieved by the source-load model in 2050. The results are as shown in Figure 2.
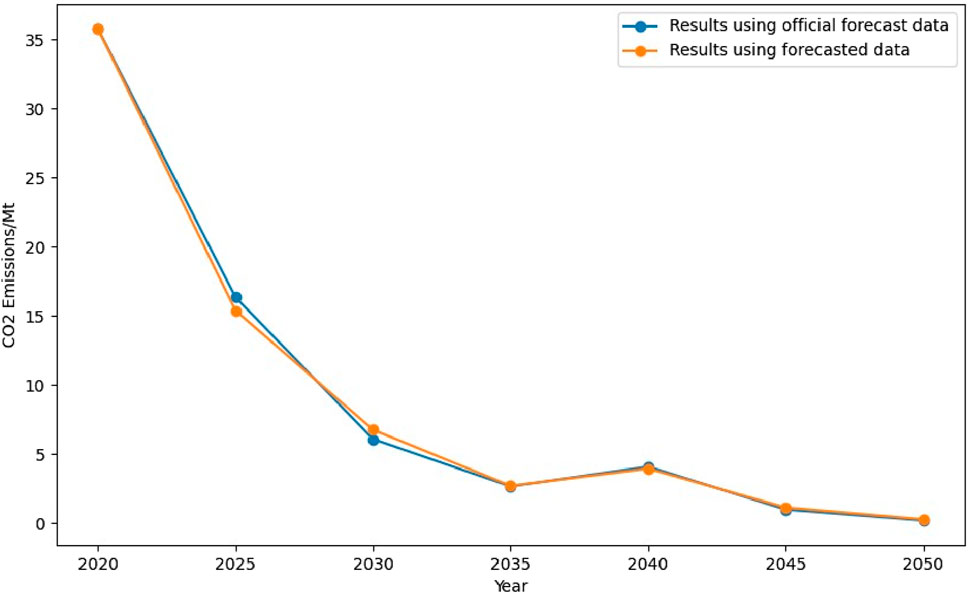
FIGURE 1. The difference between incorporating forecasted results and directly using official forecast data in the model.
4.2 The influence of forecast uncertainty on coordination pathways
We examined the impact of load forecasting errors on optimization results and compared the effects of a 5% and 10% increase in load forecasting. The results are as shown in Table 3. The results indicate that as the load increases, carbon emissions in 2050 also rise. Increasing the proportion of wind and solar power proves to be more cost-effective, while augmenting investments in long-term energy storage is more beneficial in mitigating the effects of load forecasting errors.
4.3 The influence of economic uncertainty on coordination pathways
We investigated the impact of energy storage cost fluctuations on optimization results, comparing the long-term and short-term storage investments between the FES pathway and our model. Additionally, we contrasted the scenario with a 5% increase in energy storage costs, as depicted in Figure 3. The results indicate that, in comparison to the FES pathway, the coordinated pathway tends to favor investments in long-term energy storage. This tendency becomes more pronounced when energy storage costs increase; with a 5% cost increase, investments in short-term energy storage are reduced by approximately 8.3%.
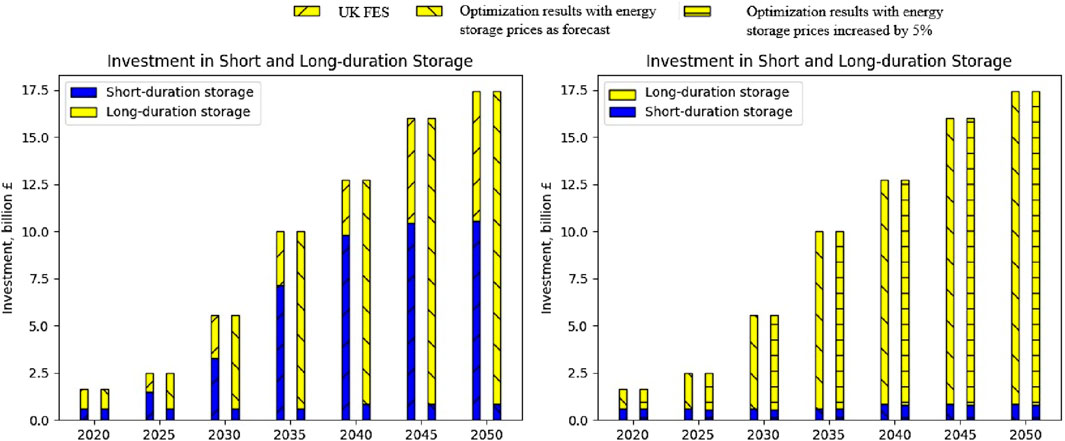
FIGURE 3. Comparison of long-term and short-term energy storage investments under different scenarios.
5 Conclusion
This paper proposes a coordinated configuration method for integrating new energy sources and energy storage. Optimization and comparisons are conducted based on the UK FES pathway, with an exploration of the impact of uncertainties on optimization results. The conclusions drawn are as follows:
1) Forecast accuracy affects optimization outcomes, with the overall trend in this model, compared to the UK’s proposed FES pathway, being an increase in investments in long-term energy storage and wind power.
2) Load forecasting errors directly impact optimization results, leading to higher carbon emissions in 2050 with an increase in load. This situation also escalates the proportions of wind/solar power and long-term/short-term energy storage.
3) A 5% increase in energy storage costs results in approximately an 8.3% reduction in investment in short-term energy storage in this model.
Data availability statement
The raw data supporting the conclusion of this article will be made available by the authors, without undue reservation.
Author contributions
JnL: Writing–original draft. GW: Writing–review and editing. JoL: Writing–review and editing.
Funding
The author(s) declare financial support was received for the research, authorship, and/or publication of this article. This paper is funded by project SGFJJY00GHJS2250008.
Conflict of interest
Authors JnL, GW, and LZ were employed by State Grid Fujian Electric Power Co., Ltd.
Publisher’s note
All claims expressed in this article are solely those of the authors and do not necessarily represent those of their affiliated organizations, or those of the publisher, the editors and the reviewers. Any product that may be evaluated in this article, or claim that may be made by its manufacturer, is not guaranteed or endorsed by the publisher.
References
Arbabzadeh, M., Sioshansi, R., Johnson, J. X., and Keoleian, G. A. (2019a). The role of energy storage in deep decarbonization of electricity production. Nat. Commun. 10, 3413–3511. doi:10.1038/s41467-019-11161-5
Arbabzadeh, M., Sioshansi, R., Johnson, J. X., and Keoleian, G. A. (2019b). The role of energy storage in deep decarbonization of electricity production. Nat. Commun. 10, 3413–3511. doi:10.1038/s41467-019-11161-5
BEIS (2020). Electricity generation costs 2020. https://www.gov.uk/government/publications/beis-electricity-generation-costs-2020.
California Energy Commission (2020). Assessing long-duration energy storage deployment scenarios to meet California’s energy goals, grant solicitation. https://www.energy.ca.gov/solicitations/2020-01/gfo-19-308-assessing-long-duration-energy-storage-deployment-scenarios-meet.
Fu, W., Jiang, X., Li, B., Tan, C., Chen, B., and Chen, X. (2023). Rolling bearing fault diagnosis based on 2D time-frequency images and data augmentation technique. Meas. Sci. Technol. 34 (4), 045005. doi:10.1088/1361-6501/acabdb
Fu, X., Wu, Wu X., Zhang, C., Fan, S., and Liu, N. (2022). Planning of distributed renewable energy systems under uncertainty based on statistical machine learning. Prot. Control Mod. Power Syst. 7 (4), 41–27. doi:10.1186/s41601-022-00262-x
Gao, N., Zhang, H., Wang, Z., Xu, J., Cheng, J., Gao, R., et al. (2020). Planning for site selection and capacity determination of distributed energy storage in regional power grid. High. Volt. Appar. 56 (08), 52–58. doi:10.13296/j.1001-1609.hva.2020.08.009
Grünewald, P. H., Cockerill, T. T., Contestabile, M., and Pearson, P. J. (2012). The socio-technical transition of distributed electricity storage into future networks-System value and stakeholder views. Energy Policy 50, 449–457. doi:10.1016/j.enpol.2012.07.041
Hargreaves, J. J., and Jones, R. A. (2020). Long term energy storage in highly renewable systems. Front. Energy Res. 8, 553251. doi:10.3389/fenrg.2020.00219
Harvey, L. D. (2020). Clarifications of and improvements to the equations used to calculate the levelized cost of electricity (LCOE), and comments on the weighted average cost of capital (WACC). Energy 207, 118340. doi:10.1016/j.energy.2020.118340
Hill, C. A., Such, M. C., Chen, D., Gonzalez, J., and Grady, W. M. (2012). Battery energy storage for enabling integration of distributed solar power generation. IEEE Trans. smart grid 3, 850–857. doi:10.1109/tsg.2012.2190113
Huber, M., and Weissbart, C. (2015). On the optimal mix of wind and solar generation in the future Chinese power system. Energy 90, 235–243. doi:10.1016/j.energy.2015.05.146
Ioannou, A., Angus, A., and Brennan, F. (2018). Parametric CAPEX, OPEX, and LCOE expressions for offshore wind farms based on global deployment parameters. Energy sources., 13, 281–290. doi:10.1080/15567249.2018.1461150
Jafari, M., Price, J., Korpås, M., and Botterud, A. (2020). Power system decarbonization: impacts of energy storage duration and interannual renewables variability. Renew. Energy 156, 1171–1185. doi:10.1016/j.renene.2020.04.144
Kabeyi, M. J., and Olanrewaju, O. A. (2022). Sustainable energy transition for renewable and low carbon grid electricity generation and supply. Front. Energy Res. 9, 743114. doi:10.3389/fenrg.2021.743114
Li, J., Mu, G., Zhang, J., Li, C., Yan, G., Zhang, H., et al. (2023a). Dynamic economic evaluation of hundred megawatt-scale electrochemical energy storage for auxiliary peak shaving. Prot. Control Mod. Power Syst. 8 (3), 50–18. doi:10.1186/s41601-023-00324-8
Li, Z., Xu, Y., Wang, P., and Xiao, G. (2023b). Research on the selection of shared energy storage evaluation based on economic evaluation methods. High voltage apparatus:1-10. http://kns.cnki.net/kcms/detail/61.1127.TM.20231221.1131.002.html.
Li, Z., Wu, L., Xu, Y., Wang, L., and Yang, N. (2023c). Distributed tri-layer risk-averse stochastic game approach for energy trading among multi-energy microgrids. Apply Energy 331, 120282. doi:10.1016/j.apenergy.2022.120282
Li, Z., Wu, L., Xu, Y., and Zheng, X. (2021). Stochastic-weighted robust optimization based bilayer operation of a multi-energy building microgrid considering practical thermal loads and battery degradation. IEEE Trans. Sustain. Energy 13 (2), 668–682. doi:10.1109/tste.2021.3126776
Li, Z., Xu, Y., Fang, S., Wang, Y., and Zheng, X. (2020). Multiobjective coordinated energy dispatch and voyage scheduling for a multienergy ship microgrid. IEEE Trans. Industry Appl. 56 (2), 989–999. doi:10.1109/tia.2019.2956720
Li, Z., Xu, Y., Wang, P., and Xiao, G. (2023d). Restoration of multi energy distribution systems with joint district network recon figuration by a distributed stochastic programming approach. IEEE Trans. Smart Grid, 1–13. doi:10.1109/TSG.2023.3317780
Li, Z., Xu, Y., Wang, P., and Xiao, G. (2023e). Coordinated preparation and recovery of a post-disaster multi-energy distribution system considering thermal inertia and diverse uncertainties. Apply Energy 336, 120736. doi:10.1016/j.apenergy.2023.120736
MacDonald, M. (2018). Storage cost and technical assumptions for BEIS. https://www.gov.uk/government/publications/storage-cost-and-technical-assumptions-for-electricity-storage-technologies.
Mott, M. D. (2018). Storage cost and technical assumptions for BEIS. https://www.gov.uk/government/publications/storage-cost-and-technical-assumptions-for-electricity-storage-technologies.
Xu, P., Fu, W., Lu, Q., Zhang, S., Wang, R., and Meng, J. (2023). Stability analysis of hydro-turbine governing system with sloping ceiling tailrace tunnel and upstream surge tank considering nonlinear hydro-turbine characteristics. Renew. Energy 210, 556–574. doi:10.1016/j.renene.2023.04.028
Yang, N., Dong, Z., Wu, L., Zhang, L., Shen, X., Chen, D., et al. (2022b). A comprehensive review of security-constrained unit commitment. J. Mod. Power Syst. Clean Energy 10 (3), 562–576. doi:10.35833/mpce.2021.000255
Yang, N., Qin, T., Wu, L., Huang, Y., Huang, Y., Xing, C., et al. (2022d). A multi-agent game based joint planning approach for electricity-gas integrated energy systems considering wind power uncertainty. Electr. Power Syst. Res. 204, 107673. doi:10.1016/j.epsr.2021.107673
Yang, N., Yang, C., Wu, L., Shen, X., Jia, J., Li, Z., et al. (2022a). Intelligent data-driven decision-making method for dynamic multisequence: an e-seq2seq-based SCUC expert system. IEEE Trans. industrial Inf. 18 (5), 3126–3137. doi:10.1109/tii.2021.3107406
Yang, N., Yang, C., Xing, C., Ye, D., Jia, J., Chen, D., et al. (2022c). Deep learning-based SCUC decision-making: an intelligent data-driven approach with self-learning capabilities. IET Generation, Transm. Distribution 16 (4), 629–640. doi:10.1049/gtd2.12315
Yang, N., Ye, D., Zhou, Z., Jiazhan, C., Daojun, C., and Xiaoming, W. (2018). Research on modelling and solution of stochastic SCUC under ac power flow constraints. IET Generation Transm. Distribution 12 (15), 3618–3625. doi:10.1049/iet-gtd.2017.1845
Yang, Y., Li, Z., Mandapaka, P. V., and Lo, E. Y. (2023). Risk-averse restoration of coupled power and water systems with small pumped-hydro storage and stochastic rooftop renewables. Apply Energy 339, 120953. doi:10.1016/j.apenergy.2023.120953
Zhang, Y., Wei, L., Fu, W., Chen, X., and Hu, S. (2023b). Secondary frequency control strategy considering DoS attacks for MTDC system. Electr. Power Syst. Res. 214, 108888. doi:10.1016/j.epsr.2022.108888
Zhang, Y., Xie, X., Fu, W., Chen, X., Hu, S., Zhang, L., et al. (2023a). An optimal combining attack strategy against economic dispatch of integrated energy system. IEEE Trans. Circuits Syst. II Express Briefs 70 (1), 246–250. doi:10.1109/tcsii.2022.3196931
Keywords: decarbonization, renewable energy integration, energy storage systems, collaborative optimization, uncertainty
Citation: Liao J, Wu G and Li J (2024) Collaborative configuration of renewable energy and energy storage under fixed investment in the decarbonization process. Front. Energy Res. 12:1345780. doi: 10.3389/fenrg.2024.1345780
Received: 28 November 2023; Accepted: 30 January 2024;
Published: 14 February 2024.
Edited by:
Wenlong Fu, China Three Gorges University, ChinaReviewed by:
Xiao Xu, Sichuan University, ChinaTao Zhou, Nanjing University of Science and Technology, China
Copyright © 2024 Liao, Wu and Li. This is an open-access article distributed under the terms of the Creative Commons Attribution License (CC BY). The use, distribution or reproduction in other forums is permitted, provided the original author(s) and the copyright owner(s) are credited and that the original publication in this journal is cited, in accordance with accepted academic practice. No use, distribution or reproduction is permitted which does not comply with these terms.
*Correspondence: Jinlin Liao, bGlhb2ppbmxpbkBhbHVtbmkuc2p0dS5lZHUuY24=