- 1Dispatching and Control Center, Central China Branch of State Grid Corporation of China, Wuhan, China
- 2School of Electrical Engineering and Automation, Wuhan University, Wuhan, China
The existing research on orderly participation of air conditioning load in frequency regulation needs a relatively complete communication architecture, but it cannot be achieved on a large scale in practice. This paper proposes a decentralized control method for the air-conditioning load control to participate in the power system primary frequency regulation, so that the air-conditioning loads can respond to system frequency deviation in an orderly manner without communication. Firstly, the triggering frequency is set to prevent the air-conditioning load from being over- or under-adjusted based on the frequency response interval, which is determined according to the outdoor temperature. Secondly, the response priority is arranged according to the holding time to ensure an orderly response of the air-conditioning load without communication. Finally, a simulation example of a thousand air-conditioning loads under different control strategy proves that the proposed method can achieve better frequency regulation effect.
1 Introduction
Maintaining frequency stability is a prerequisite for ensuring the stable operation of the power system and electricity supply for users. The real-time power balance between the generator side and the user side plays a decisive role in the stability of the system frequency. The traditional frequency regulation means of power system mainly relies on the generator side to track load fluctuation and stabilize the accident disturbance (Kundur et al., 1994). However, as a large number of renewable energy sources (RESs) are connected to the system, the randomness of RESs power output increases the probability and amplitude of system frequency fluctuations, and greatly increases the frequency regulation capacity requirements of the power system (Joos et al., 2000; Obaid et al., 2019). On the other hand, with the increasing of RESs proportion and the decreasing of conventional units proportion, the primary and secondary frequency regulation capacity of the traditional units and the system inertia will decline correspondingly, which brings further challenges to the frequency stability of the power system (Magdy et al., 2021).
Demand response (DR) participating in power system frequency regulation can greatly improve the frequency regulation capability and dynamic characteristics of the power system, which has become a research hotspot in the field of frequency regulation. The air-conditioning load is huge, accounting for about one-third of the total summer electricity load of Spain (Science Daily, 2012). In China, Jiangsu’s air-conditioning load power consumption accounts for about 45% of the total summer load power consumption (State Grid and State Grid Jiangsu Electric Power Co Ltd, 2019).
The basic idea of the air-conditioning load participating in the frequency regulation in the existing study is to make the air-conditioning load respond to the system frequency deviation automatically according to the local measurement of system frequency. In terms of the overall effect, the droop response of the load power of the entire air-conditioning cluster to the system frequency can be realized. However, the quantity of the air conditioning loads is huge. How to coordinate multiple air-conditioning loads within the cluster and orderly exert the regulating ability of different air-conditioning loads is the main problem to be solved (Li et al., 2020).
Previous studies mainly focus on the coordinated control of multiple air-conditioning loads based on full communication conditions. That is, the master station collects the status of all air-conditioning loads and then according to a certain sorting rule makes centralized decision to issue control commands to different air-conditioning loads when the frequency deviation occurs in the system. Centralized control uses the unified analysis and calculation of the control center to determine which air-conditioner loads participate in frequency response under different grid states, and then directly issues switch commands to each air-conditioning load. In (Lu and Zhang, 2013), a centralized load controller and its control algorithm are designed to generate a priority list and issue scheduling commands, which can optimize the response sequence of air-conditioning loads to obtain better continuous adjustment reserves. Some scholars found that using “transition time” instead of indoor temperature as the basis for priority ranking can improve the regulation effect of system frequency (Zhou et al., 2015). It needs to be pointed out that the centralized control method has high requirements for the completeness and timeliness of communication. However, it is actually difficult to establish complete and timely communication between the control center and each air-conditioning load under the existing conditions. Therefore, this type of control mode has great limitations in practical applications.
Another air-conditioning load control mode is “centralized-distributed control,” which adopts the basic mode of “upward aggregation-centralized analysis-unified setting-decentralized response.” The response mechanism is set for each air-conditioning load in advance, so that they can respond immediately when deviation occurs. In Biegel et al. (2013), Zhang et al. (2013), Song et al. (2019), the control center sends the same control signal α to each air-conditioning load so that it will turn on/off according to the probability α, so as to achieve different adjustments as a whole. In Bao et al. (2015), Wu et al. (2018), different triggering frequencies are set for different air-conditioning loads through priority sorting, so that air-conditioning loads with higher priority have lower trigger frequencies, so as to achieve orderly control of air-conditioning loads. The centralized-distributed control presents good control characteristics. Because the judgment mechanism of whether the air-conditioning load participates in the response is set in the user’s local area, the control mode can meet the frequency modulation time scale requirements. However, the centralized-distributed control still requires complete communication conditions and requires the control center to establish communication with each air-conditioning load to obtain its operating status. Such complete communication has not actually been established in the actual power grid, and its cost is extremely high and difficult to achieve. Under the condition of no communication, if each air-conditioning load can make an autonomous decision on its own control strategy based on its own state to realize global coordination at the cluster level, it is of great practical significance for the air-conditioning loads to participate in the system frequency modulation.
The decentralized control without communication has no upward aggregation and centralized analysis links. In most existing studies, the air-conditioning load adjusts the temperature setting or switch state by itself based on the monitored system frequency information. In Xu et al. (2011), the set temperature of the thermostatically controlled load is changed according to the pre-set fixed proportional coefficient and the system frequency deviation. However, because the proportional coefficient is fixed, the response amount will fluctuate greatly under different air-conditioning load totals, increasing the uncertainty of frequency modulation effect. In Molina-García et al. (2011), different triggering frequency and reconnecting frequency are set for different air-conditioning loads as the basis for participating in response and reconnect to system. Besides, the action delay is set to reduce measurement noise. However, because the triggering frequency and reconnecting frequency are both fixed and cannot be adjusted according to its own working status, the response time of the air-conditioning load is difficult to be guaranteed. The decentralized control does not rely on communication facilities, has low cost and high reliability, and is easy to implement. However, the uncertainty caused by the inability to unified dispatch and control makes it difficult for frequency regulation.
In this case that the triggering frequency is fixed, the response capacity has not been fully utilized and it is difficult to meet various environmental conditions. In response to the above problems, this paper proposes a decentralized air-conditioning demand response strategy by autonomous local decision-making based on local temperature information. The local temperature information and system frequency information measured by the air-conditioning load are calculated locally to set the appropriate triggering frequency. The main contributions are as follows:
1) Decentralized control strategy to optimize the frequency response interval of the air-conditioning cluster is proposed by monitoring the environmental temperature. According to the relationship between environmental temperature and total air-conditioning load, frequency response interval is adjusted. Thus, the over-adjustment or under-adjustment of the air-conditioning loads can be avoided in the incomplete communication condition.
2) The response priority of the air-conditioning load is sorted based on the “holding time,” which is defined according to the room temperature and the set temperature. Thus, the relatively orderly control of the air-conditioning loads can be realized to provide a longer frequency adjustment time without compromising the service life of the air-conditioning.
The rest is organized as follows: Section 2 introduces basement such as air-conditioning load droop control, frequency response action mechanism and equivalent model. Section 3 explains the air-conditioning triggering frequency setting mechanism. Section 4 introduces the working mechanism of the air-conditioning local controller. Section 5 is simulations.
2 Problem formulation for demand response
2.1 Architecture
Air-conditioning load participating in primary frequency regulation usually adopts proportional control based on the principle of analog generator primary frequency regulation. Air-conditioning active power adjustment is proportionally controlled by the system frequency deviation calculated in Eq. 1.
where
In the actual response process, the power of the fixed-frequency air-conditioning cannot be continuously adjusted. Thus, the continuous adjustment of the total power of the air-conditioning cluster can be achieved by switching large-scale air-conditioning loads.
For a single air-conditioning load, the triggering frequency is pre-set, and the system frequency is monitored at the same time. By comparing the triggering frequency and the system frequency deviation, it is determined whether and how the air-conditioning load will respond. The air-conditioning response mechanism is shown in Figure 1, and the ordinate represents the system frequency deviation. When the system frequency drops to
For air-conditioning clusters, different triggering frequencies are set for each air-conditioning load to achieve different responses under different frequency deviations and the correlation in Eq. 2.
2.2 Equivalent model of air-conditioning
This paper uses the classic ETP model as the air-conditioning model. All details about ETP model can be found in Wen et al. (2021).
Indoor temperature can be expressed by Eq. 4.
where
The indoor temperature fluctuates between the maximum allowed value
3 Design of decentralized control for air-conditioning loads based on local temperature
3.1 Frequency response interval setting based on environmental information
Compared with the centralized control mode with communication, one of the characteristics of the decentralized control is uncertainty (Li et al., 2021). For the same air-conditioning cluster, there must be load peaks and load valleys. If the triggering frequencies of all the air-conditioning loads are between the fixed interval
The main factor that affects residents’ switching on and off of air-conditioning is weather, which is prominently manifested in temperature (De Felice et al., 2013). For the analytical expression of the correlation between the total air-conditioning load and the outdoor temperature, many documents have been studied (Pourmousavi and Nehrir, 2014). Because it is not included in the research content of this paper, it will not be repeated.
For the same system frequency deviation, compared to the larger total power of the air-conditioning cluster, when the total power of the air-conditioning cluster is small, in order to keep the air-conditioning cluster a relatively stable response so that its participation in demand response effect is not affected, the air-conditioning must be triggered between a smaller frequency interval, that is, the frequency response span
The frequency response interval can be given:
In the formula,
Combining Figure 3, Eqs 8, 9 and the correlation between the total air-conditioning load and the outdoor temperature, the corresponding relationship between outdoor temperature and frequency response interval can be obtained.
3.2 Response priority ranking based on hold time
In order to ensure users’ comfort, when the indoor temperature rises to the upper limit
According to Eqs 4–7, the calculation formulas for holding time and maximum holding time can be obtained:
According to Figure 4 and Eq. 11, it can be seen that the farther the indoor temperature is from the upper limit set by the user at the time of demand response, the longer the hold time. For system frequency modulation, the longer the hold time, the longer the air conditioning participates in the frequency modulation, which has a positive effect on the frequency modulation effect. For the air-conditioning itself, the holding time is too short and frequent switching will affect its service life. Therefore, the air-conditioning with a long holding time should be turned off first, that is, the air-conditioning has a higher priority to participate in the response.
It needs to be pointed out that for the same air-conditioning, as the indoor temperature drops, the holding time gradually increases, but the relationship between the two is not linear. According to Figure 2, it can be seen that as the indoor temperature rises, its increasing speed gradually slows down. For different air-conditionings, it can be seen from Eq. 10 that the holding time is not only related to the indoor temperature, but also related to the heat capacity and thermal resistance. Therefore, for different air-conditioning loads, even if the indoor temperature is the same, the holding time is different, such as A and A’in Figure 4. However, for demand response, the grid pays more attention to the holding time of the air-conditionings participation in the response, so the holding time is used here instead of temperature.
3.3 Autonomous decision of triggering frequency based on response priority
The higher the priority of the air-conditioning, the sooner it should participate in system frequency regulation, that is, the lower the triggering frequency
Considering that the decentralized control does not have communication function, the triggering frequency is calculated locally according to the environmental information and air-conditioning parameters. The calculation method is as Eq. 12 and the complete flow chart for triggering frequency calculation is as Figure 5.
where
Combining Eqs 10–11, Eq. 12 can be transformed into Eq. 13:
In addition, in order to avoid frequent switching on and off causing damage to the service life of the air-conditionings, a lock time is set. When the hold time is less than the lock time, the air-conditioning is locked and the triggering frequency is not set, that is, it does not participate in demand response.
4 Local controller mechanism and method application
The air-conditioning load local controller has five modules: information acquisition module, calculation module, monitoring module, control module and locking unit, which are respectively responsible for multi-party information acquisition, triggering frequency and parameter calculation, system frequency monitoring, air-conditioning switch control and air-conditioning lock.
As shown in Figure 6, the working mode of the local controller for air-conditioning load is as follows:
The information acquisition module transmits real-time updated information to the calculation module. The computing module performs online calculations of the air conditioner’s holding time and trigger frequency, and sends this information respectively to the locking unit and monitoring module. By comparing system frequency offset and trigger frequency, the monitoring module makes decisions on air conditioner switching instructions, while the locking unit determines whether the air conditioner participates in the demand response by comparing the hold time and lock time. The control module receives instructions from both the monitoring module and locking unit in order to switch the operating state of the air conditioner.
4.1 Information acquisition module
1) Collection of environmental information: sampling the indoor temperature and outdoor temperature where the air-conditioning is located once every minute; 2) Collection of external input information: temperature set by the user, frequency response dead zone, frequency response interval, etc., can be updated annually/quarterly according to actual needs, with extremely low timeliness and communication requirements and various implementation methods; 3) Collection of setting information for air-conditioning manufacturers: temperature setting threshold, lock time, rated power, etc.
4.2 Calculation module
1) Fit the curve based on historical data and combine Eq. 5 to calculate the equivalent heat capacity and thermal resistance; 2) Accept the data information from the information acquisition module, and calculate the hold time online according to (12) (13); 3) Calculate the triggering frequency according to (14).
4.3 Monitoring module
1) Real-time monitoring of the system frequency; 2) Once the frequency deviation exceeds the triggering frequency, a switch command will be issued.
4.4 Control module
Accept commands issued by the calculation module to directly control the air-conditioning switch.
4.5 Locking unit
Accept the hold time transmitted by the calculation module, compare the hold time with the lock time, if the hold time is less than the lock time, lock the control module to prevent the air-conditioning from participating in demand response.
4.6 Method application
The proposed method theoretically enables a large number of decentralized air conditioners to participate in the primary frequency regulation of a real-world scenario, providing auxiliary frequency regulation services for the power system. Firstly, in simulating the participation of air conditioning in primary frequency regulation in real scenarios, a power system frequency response (SFR) model can be constructed to accurately reflect the system frequency response characteristics through model parameter identification method. Secondly, based on the method proposed in this paper, the local controller of the air conditioner will dynamically calculate the triggering frequency and holding time. Different triggering frequencies make different response sequences of the air conditioner. By monitoring the dynamic frequency deviation of the system, the air conditioner can realize local autonomous decision-making under the premise of ensuring the operation life and the comfort requirements of users, which means the air conditioner cluster changes response power according to the variation of the system frequency in an orderly manner, and realize the rapid recovery of the system frequency.
5 Case study
The simulation assumes that 1,000 air conditioners equipped with local controllers are in the on state at the current outdoor temperature, and the temperature settings of each air-conditioning are the same. To simulate the differentiated features of actual scenarios, the indoor temperature where the air-conditioning is located is regarded as uniformly distributed within the allowable room temperature range, and the rated power of the air-conditioning load, the equivalent heat capacity of the room, and the equivalent thermal resistance obey the normal distribution (Xu et al., 2011). The outdoor temperature is regarded as the same and constant due to the short frequency modulation time scale and the parameter settings for simulation are shown in Table 1.
In a certain air-conditioning cluster, the smaller the triggering frequency value, the more priority the air-conditioning participates in the demand response. In other words, the triggering frequency represents the order in which the air-conditioning participates in the demand response. If the triggering frequency value is randomly and uniformly assigned to the air-conditioning loads without priority calculation and sorting, the room temperature of the air-conditioning has nothing to do with the triggering frequency value, and it is in a disordered state most of the time, such as Figure 7B. In some special cases, there may also be extreme situations in which the room temperature decreases as the triggering frequency increases, such as Figure 7A. If Figures 7A, B is assigned to the triggering frequency through the priority arrangement of this paper, then Figure 7C can be obtained. The three scenes of Figures 7A–C are called scene 1, scene 2 and scene 3 respectively, and simulations are performed in the three cases respectively.
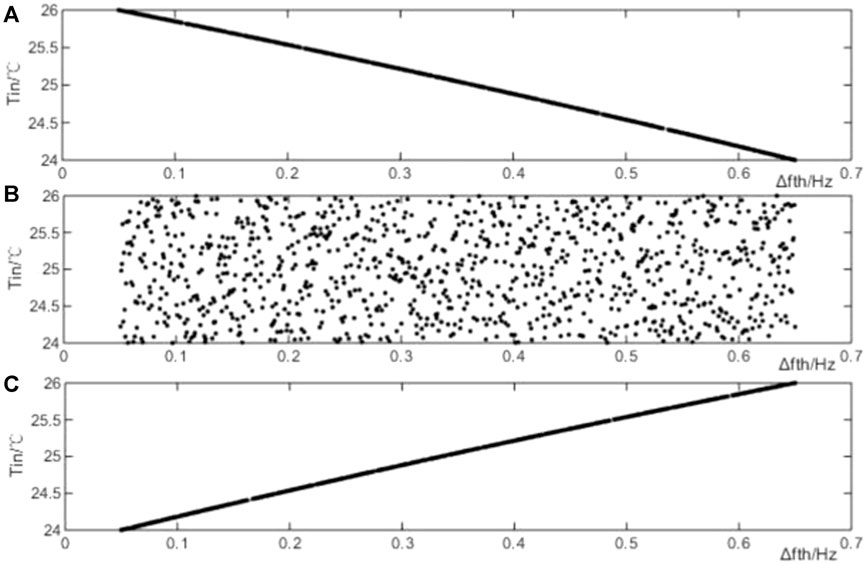
FIGURE 7. Room temperature and triggering frequency distribution (A) The triggering frequency increases as room temperature decreases (B) A disordered state (C) The triggering frequency increases as room temperature increases (strategy in this paper).
CASE 1. Step disturbance
Assuming that the system suffers a step disturbance of 0.1 p.u. (account for
Compared with scene l and scene 2, scene 3 has been prioritized, and the lowest system frequency has been significantly improved, which is about
Each point in Figure 9 represents one of the air-conditioning loads that participated in the demand response. The horizontal and vertical coordinates are respectively the moment of its participation in the demand response and its holding time (p.u.). Due to the difference of the holding time of the air conditioners, the hold time is equivalent to the identification code of the air conditioner. That is, from left to right, it represents the air-conditioning load’s participation in demand response sequence. Figure 10 represents the change of the probability distribution of the indoor temperature of the air-conditioning over time. This calculation can be obtained by the kernel density estimation of the ksdensity function in MATLAB according to the sample data. Analyzing Figure 9 and Figure 10, we can draw conclusions:
In scene 1, air-conditioning loads basically follow the principle that the shorter the hold time is, the faster the response is, which can also be observed in Figure 10. The probability of air-conditioning at indoor temperature close to
In scene 2, the air-conditioning loads participating in the demand response have a relatively uniform distribution of indoor temperature, and the probability distribution of the indoor temperature varies less. However, because there is still air-conditioning loads with short holding time participating in the response, the rapid load rebound is obvious, resulting in a poor frequency modulation effect.
In scene 3, due to the differences in parameters such as heat capacity and thermal resistance, the air-conditioning response sequence does not strictly follow the principle of “air conditioning with a long holding time responds first,” but the air-conditioning load that participates in demand response as a whole has a longer holding time, which obtains a longer frequency adjustment time and a better frequency modulation effect.
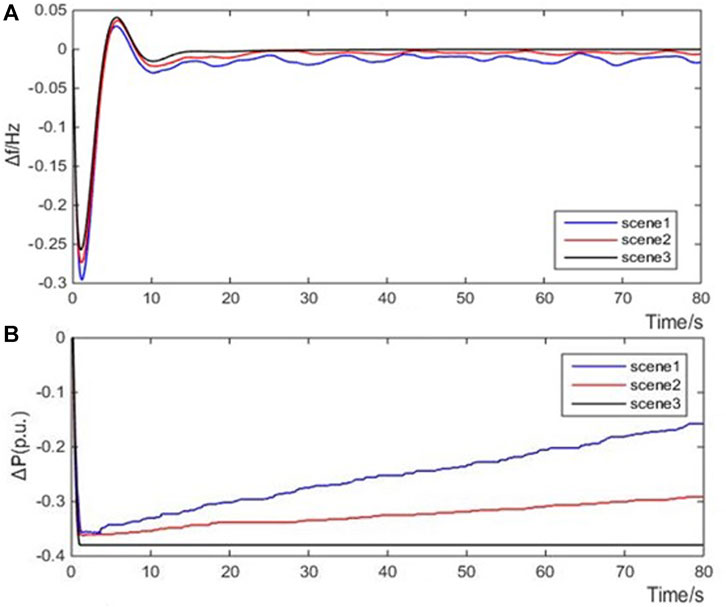
FIGURE 8. Simulation results subjected to step disturbance (A) System frequency fluctuation (B) Air-conditioning load power reduction.
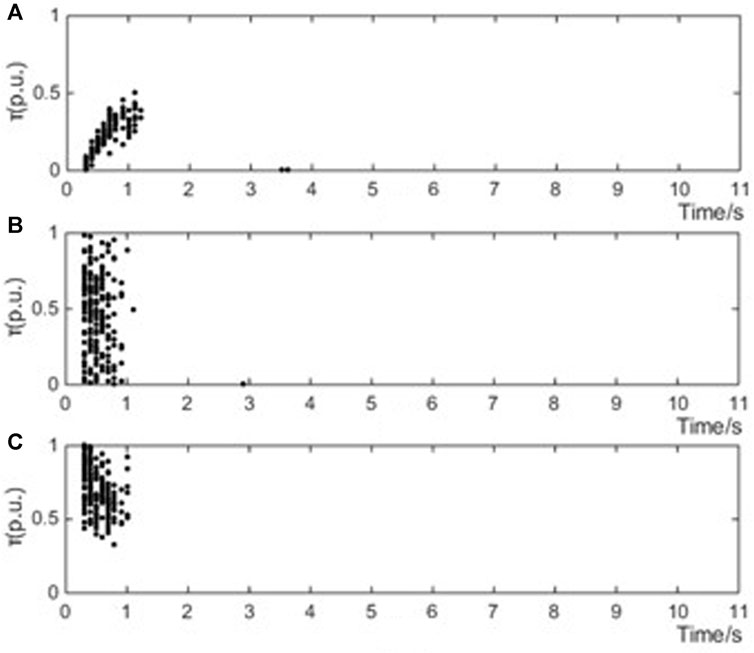
FIGURE 9. Response order and hold time subjected to step disturbance (A) The triggering frequency increases as room temperature decreases (B) A disordered state (C) The triggering frequency increases as room temperature increases (strategy in this paper).
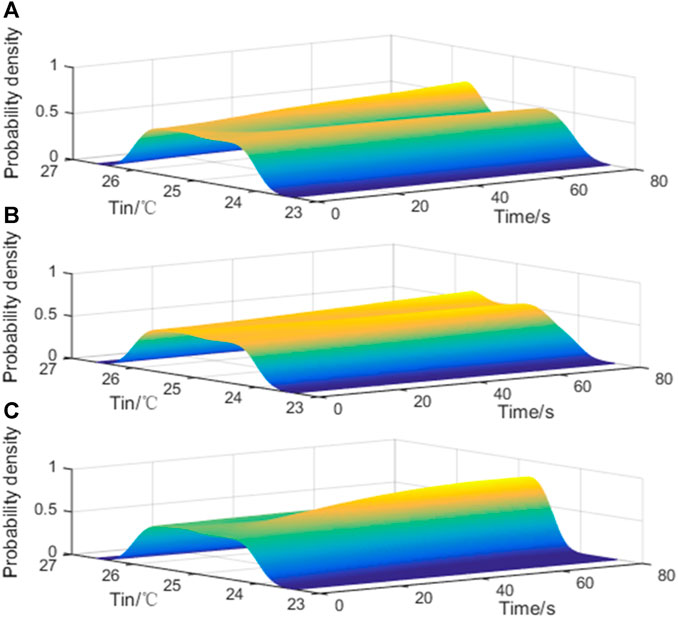
FIGURE 10. Indoor temperature distribution changes with time subjected to step disturbance (A) The triggering frequency increases as room temperature decreases (B) A disordered state (C) The triggering frequency increases as room temperature increases (strategy in this paper).
CASE 2. Continuous disturbance
The system sometimes encounters continuous disturbances, such as the continuous sharp drop in wind power output, etc. Set it to Figure 11. The total load of the air-conditioning cluster is still set to 0.2p.u. The simulations were performed in the three scenes of Figure 7. Simulation results as shown in Figure 12.
Observing Figure 12, it can be found that the frequency deviation of scene 1 is the largest and the fluctuation is the most intense, scene 2 is the second, and the frequency deviation of scene 3 is the smallest and the fluctuation is small. In addition, the RMSE of the frequency stabilization stage in the three scenarios are 0.0173, 0.0074, and 0.0053, and the MSE are 0.0089, 0.0051, and 0.0043, which can also be used as evidence.
The meanings of Figure 13 and Figure 14 are similar to Case 1. The air-conditioning loads involved in demand response in Scene 1 has a shorter holding time, and there are still many air-conditioning loads responding around 10 s, which directly leads to the subsequent frequency fluctuations.
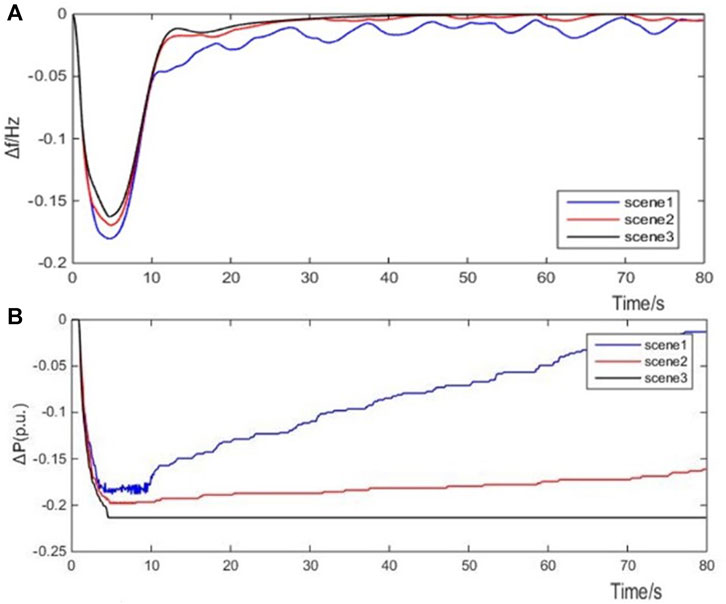
FIGURE 12. Simulation results subjected to continuous disturbance (A) System frequency fluctuation (B) Air-conditioning load power reduction.
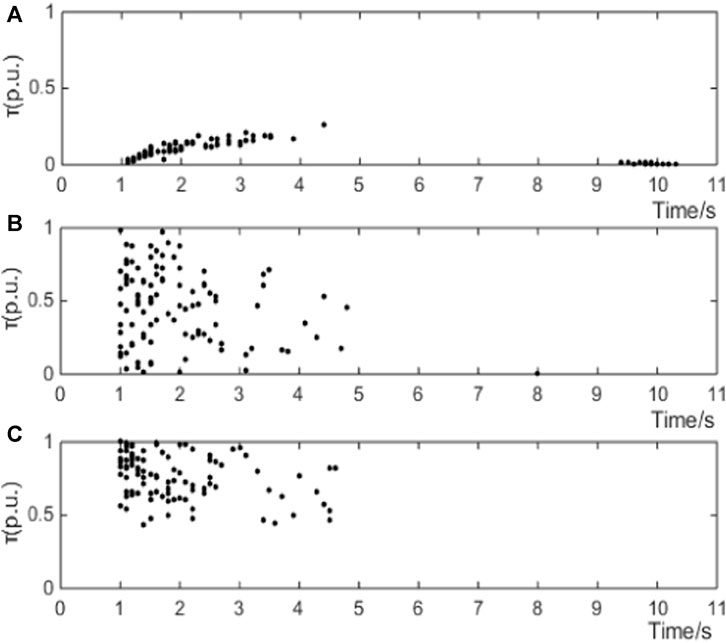
FIGURE 13. Response order and hold time subjected to continuous disturbance (A) The triggering frequency increases as room temperature decreases (B) A disordered state (C) The triggering frequency increases as room temperature increases (strategy in this paper).
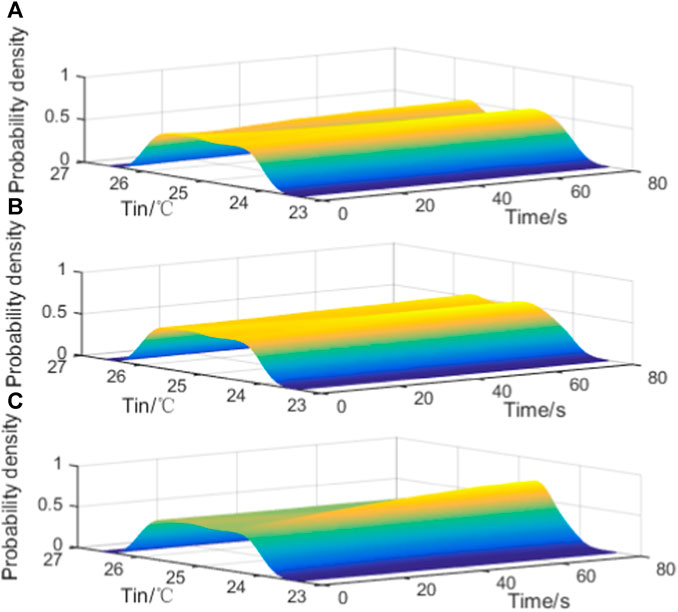
FIGURE 14. Indoor temperature distribution changes with time subjected to continuous disturbance (A) The triggering frequency increases as room temperature decreases (B) A disordered state (C) The triggering frequency increases as room temperature increases (strategy in this paper).
6 Conclusion
This paper proposes an adaptive control strategy for air-conditioning loads based on environmental perception. Decentralized control strategy for coordination of multiple air-conditioning loads is proposed based on the frequency response interval and the response priority in the condition that the communication is incomplete. On the one hand, by optimizing the setting of the frequency response interval, the over- or under-adjustment of the air-conditioning loads is avoided to obtain a better frequency modulation effect, and it will not cause excessive overshoot to bring new impact to the grid. On the other hand, the definition of holding time and prioritization realizes relatively orderly control of air-conditioning loads, which will not damage the service life of air-conditioning while gaining longer frequency adjustment time for the power grid. Based on the method proposed in this paper, the relative order control of air-conditioning cluster under incomplete communication can be realized. However, this paper mainly focuses on the orderly control strategy of decentralized air conditioning participating in primary frequency regulation, without considering the robustness of the control strategy under system uncertainties. We will conduct further research on this issue in the future.
Data availability statement
The original contributions presented in the study are included in the article/Supplementary Material, further inquiries can be directed to the corresponding author.
Author contributions
BJ: Funding acquisition, Project administration, Writing–review and editing. ZY: Data curation, Investigation, Writing–review and editing. WX: Conceptualization, Methodology, Writing–review and editing. LC: Formal Analysis, Methodology, Writing–original draft, Validation. PW: Methodology, Software, Visualization, Writing–review and editing. SL: Writing–review and editing.
Funding
The author(s) declare financial support was received for the research, authorship, and/or publication of this article. This work was supported by the technology project of Central China Branch of State Grid Corporation of China (SGHZ0000DKJS2310392). This paper is supported by the technology project: research on key technologies for inter provincial peak shaving and supply guarantee adapting to multi section constraints (Project No. 521400230001).
Conflict of interest
Authors BJ, ZY, and WX were employed by Central China Branch of State Grid Corporation of China.
The remaining authors declare that the research was conducted in the absence of any commercial or financial relationships that could be construed as a potential conflict of interest.
Publisher’s note
All claims expressed in this article are solely those of the authors and do not necessarily represent those of their affiliated organizations, or those of the publisher, the editors and the reviewers. Any product that may be evaluated in this article, or claim that may be made by its manufacturer, is not guaranteed or endorsed by the publisher.
References
Bao, Y., Li, Y., Hong, Y., and Wang, B. (2015). Design of a hybrid hierarchical demand response control scheme for the frequency control. Generation Transm. Distribution IET 9 (15), 2303–2310. doi:10.1049/iet-gtd.2015.0628
Biegel, B., Hansen, L. H., Andersen, P., and Stoustrup, J. (2013). Primary control by ON/OFF demand-side devices. IEEE Trans. Smart Grid 4 (4), 2061–2071. doi:10.1109/tsg.2013.2257892
De Felice, M., Alessandri, A., and Ruti, P. M. (2013). Electricity demand forecasting over Italy: potential benefits using numerical weather prediction models. Electr. Power Syst. Res. 104, 71–79. doi:10.1016/j.epsr.2013.06.004
Joos, G., Ooi, B. T., Mcgillis, D., Galiana, F. D., and Marceau, R. (2000). “The potential of distributed generation to provide ancillary services,” in Proceedings of the 2000 Power Engineering Society Summer Meeting (Cat. No.00CH37134), Seattle, WA, USA, July 2000, 1762–1767. doi:10.1109/PESS.2000.868792
Kundur, P., Balu, N. J., and Lauby, M. G. (1994). “Control of active power and reactive power,” in Power system stability and control (NY: McGraw-Hill), 159–161.
Li, Z., Wu, L., Xu, Y., and Zheng, X. (2021). Stochastic-weighted robust optimization based bilayer operation of a multi-energy building microgrid considering practical thermal loads and battery degradation. IEEE Trans. Sustain. Energ. 13 (2), 668–682. doi:10.1109/TSTE.2021.3126776
Li, Z., Xu, Y., Fang, S., Zheng, X., and Feng, X. (2020). Robust coordination of a hybrid AC/DC multienergy ship microgrid with flexible voyage and thermal loads. IEEE Trans. Smart Grid 11 (4), 2782–2793. doi:10.1109/TSG.2020.2964831
Lu, N., and Zhang, Y. (2013). Design considerations of a centralized load controller using thermostatically controlled appliances for continuous regulation reserves. IEEE Trans. Smart Grid 4 (2), 914–921. doi:10.1109/tsg.2012.2222944
Magdy, G., Bakeer, A., and Alhasheem, M. (2021). Superconducting energy storage technology-based synthetic inertia system control to enhance frequency dynamic performance in microgrids with high renewable penetration. Prot. Control Mod. Power Syst. 6 (1), 36–13. doi:10.1186/s41601-021-00212-z
Molina-García, A., Bouffard, F., and Kirschen, D. S. (2011). Decentralized demand-side contribution to primary frequency control. IEEE Trans. Power Syst. 26 (1), 411–419. doi:10.1109/tpwrs.2010.2048223
Obaid, Z. A., Cipcigan, L. M., Abrahim, L., and Muhssin, M. T. (2019). Frequency control of future power systems: reviewing and evaluating challenges and new control methods. J. Mod. Power Syst. Clean. Energy 7, 9–25. doi:10.1007/s40565-018-0441-1
Pourmousavi, S. A., and Nehrir, M. H. (2014). Introducing dynamic demand response in the LFC model. IEEE Trans. Power Syst. 29 (4), 1562–1572. doi:10.1109/tpwrs.2013.2296696
Science Daily (2012). Air conditioning consumers one third of peak electric consumption in the summer. Available at: https://www.sciencedaily.com (Accessed October 22, 2012).
Song, M., Gao, C., Shahidehpour, M., Li, Z., Yang, J., and Yan, H. (2019). State space modeling and control of aggregated TCLs for regulation services in power grids. IEEE Trans. Smart Grid 10 (4), 4095–4106. doi:10.1109/tsg.2018.2849321
State Grid, State Grid Jiangsu Electric Power Co Ltd (2019). The highest dispatching power load of Jiangsu Power Grid reaches a new peak. Available at: http://www.js.sgcc.com.cn (Accessed July 26, 2019).
Wen, M., Pan, X., Zhang, Y., Guo, Q. H., and Liao, J. (2021). “A joint strategy of air-conditioning and electrolytic aluminum participating in primary frequency modulation,” in Proceedings of the 2021 IEEE 5th Conference on Energy Internet and Energy System Integration (EI2), Taiyuan, China, October 2021, 1368–1373. doi:10.1109/EI252483.2021.9713545
Wu, X., He, J., Xu, Y., Lu, J., Lu, N., and Wang, X. (2018). Hierarchical control of residential HVAC units for primary frequency regulation. IEEE Trans. Smart Grid 9 (4), 3844–3856. doi:10.1109/tsg.2017.2766880
Xu, Z., Ostergaard, J., and Togeby, M. (2011). Demand as frequency controlled reserve. IEEE Trans. Power Syst. 26 (3), 1062–1071. doi:10.1109/tpwrs.2010.2080293
Zhang, W., Lian, J., Chang, C. Y., and Kalsi, K. (2013). Aggregated modeling and control of air conditioning loads for demand response. IEEE Trans. Power Syst. 28 (4), 4655–4664. doi:10.1109/tpwrs.2013.2266121
Keywords: decentralized control, primary frequency regulation, demand response, thermostatically controlled loads, environmental information
Citation: Jiang B, Yang Z, Xiong W, Chen L, Wang P and Liao S (2024) Decentralized control strategy of air-conditioning loads for primary frequency regulation based on environment information. Front. Energy Res. 11:1347789. doi: 10.3389/fenrg.2023.1347789
Received: 01 December 2023; Accepted: 18 December 2023;
Published: 15 January 2024.
Edited by:
Zhengmao Li, Aalto University, FinlandReviewed by:
Xiao Hu, Northeast Electric Power University, ChinaYuchen Fang, Tsinghua University, China
Copyright © 2024 Jiang, Yang, Xiong, Chen, Wang and Liao. This is an open-access article distributed under the terms of the Creative Commons Attribution License (CC BY). The use, distribution or reproduction in other forums is permitted, provided the original author(s) and the copyright owner(s) are credited and that the original publication in this journal is cited, in accordance with accepted academic practice. No use, distribution or reproduction is permitted which does not comply with these terms.
*Correspondence: Pengyu Wang, 2023102070046@whu.edu.cn