- 1School of Electrical Engineering, Shanghai University of Electric Power, Shanghai, China
- 2Power Dispatching Control Center of State Grid Shaanxi Electric Power Co., Ltd., Xi’an, Shanxi, China
In recent years, the integration of wind power into the grid has steadily increased, but the volatility and uncertainty of wind power pose significant challenges to grid planning, scheduling and operation. Ultra-short term wind power forecasting technology as the basis of daily scheduling decision can accurately predict the future hourly wind power output, and has important research significance for ensuring the safe and stable operation of power grid. Although research on ultra-short-term wind power forecasting technology has reached maturity, practical engineering applications still face several challenges. These challenges include the limited potential for improving the accuracy of numerical weather forecasts, the issue of missing historical data from new wind farms, and the need to achieve accurate power prediction under extreme weather scenarios. Therefore, this paper aims to critically review the current proposed ultra-short-term wind power forecasting methods. On this basis, analyze the combined power forecasting method under extreme weather scenarios, and illustrate its effectiveness through wind farm case studies. Finally, according to the development trend and demand of future power systems, future research directions are proposed.
1 Introduction
The transition towards a new power system centered around renewable energy sources has necessitated the expansion of clean energy, particularly wind power, and the establishment of a low-carbon, secure, and efficient energy system to achieve China’s “dual carbon” target (Ren et al., 2022). Nowadays, the wind power industry has witnessed rapid growth, and the wind power market continues to thrive (Hui et al., 2021). The intermittency and variability of wind energy, combined with the fluctuating nature of wind turbines, lead to fluctuations in wind power output (Li et al., 2023). Ultra-short-term wind power forecasting involves predicting power levels for the next 15 min to 4 h, aiding power dispatching departments in promptly adjusting their plans to accommodate changes in wind power output (WEN, 2007). Furthermore, these prediction results serve as the basis for optimizing peak regulation, frequency modulation, economic load dispatching, and spinning reserve regulation within the power system (Yang et al., 2022a; Xu et al., 2023). Consequently, researchers have devoted considerable attention to accurately predicting ultra-short-term wind power to enhance wind power integration capacity and improve the operational efficiency of the power system.
Early methods for ultra-short-term wind power prediction primarily relied on physical models and traditional time series analysis. However, physical models necessitate extensive initial information and involve complex calculations (Ma et al., 2020). Traditional time series methods are limited in their ability to consider various factors affecting wind power and exhibit poor performance when analyzing high-dimensional data (Hanifi et al., 2020). In recent years, with the advancement of big data and artificial intelligence technologies, data-driven approaches, particularly deep learning, have gained prominence in ultra-short-term wind power prediction due to their superior nonlinear fitting capabilities and high-dimensional data processing abilities. Nan Yang et al. conducted pioneering research on SCUC problem and proposed a data-driven SCUC expert system based on extended sequence-to-sequence (E-Seq2Seq). The system can accommodate dynamic multi-sequence mapping samples and comprehensively consider various input factors that affect SCUC decision-making. Compared with traditional methods, the system has stronger generality, higher solution accuracy and efficiency (Yang et al., 2022b). Nonetheless, statistical methods like deep learning exhibit a ‘black box’ property, lacking interpretability and physical meaning to support them (Hu et al., 2016). While some progress has been made in ultra-short-term wind power prediction, several challenges need to be addressed in light of the current status of wind power generation in China. Frequent occurrence of extreme weather events leading to abrupt and unpredictable changes in power output, negatively impacting prediction accuracy (Wang et al., 2019).
The research team involved in this study has previously conducted research on new energy power prediction and achieved significant results (Yu et al., 2021; Zhou et al., 2021; Yu et al., 2022a; Yu et al., 2022b; Yu et al., 2023). The paper is structured as follows. Section 2 emphasizes the difference between ultra-short term wind power forecast and short term wind power forecast, compares and analyzes the current forecasting methods, and analyzes the advantages and disadvantages of each method. Section 3 takes wind farms as an example to show the research results of ultra-short term wind power forecasting methods under extreme weather conditions. These cases show that accurate modeling during periods of extreme weather can significantly improve overall forecast accuracy; Section 4 presents some challenges in ultra-short term wind power forecasting, such as the accuracy of Numerical Weather prediction (NWP), small sample learning, time resolution of meteorological forecasting, and extreme weather scenario forecasting. It also provides insight into the prospects for future development.
2 Overview of ultra-short-term wind power forecasting technology
The wind power prediction technology system consists of numerical weather prediction, wind power prediction model, error correction, and application of prediction results (QIAO et al., 2017; Fu et al., 2023a), as illustrated in Figure 1. Initially, historical data from wind farms are collected using the Supervisory Control And Data Acquisition (SCADA) system, meteorological measurement stations, and NWP system for feature analysis. Subsequently, a prediction model is constructed based on the nonlinear relationship between wind power characteristics and wind power. The wind power prediction results are then evaluated, and an error correction model is introduced to optimize the prediction model by rectifying prediction errors. Ultimately, the wind power prediction results are applied to power grid dispatching, maintenance plan adjustments, auxiliary power market trading, and other relevant applications.
The ultra-short-term wind power prediction encompasses a rolling multi-step forecast of wind power output from wind farms over the next 15 min to 4 h. This model adopts a 15-min rolling prediction framework, comprising four steps within an hour. Using historical data at each time point, the model predicts the power value for the subsequent four steps, serving as the input for forecasting the power value in the following moment. This enables continuous rolling predictions.
In contrast to short-term wind power prediction, which only requires providing NWP data for the next 3 days, ultra-short-term prediction necessitates real-time updates of NWP data. Additionally, the physical method employed in ultra-short-term prediction involves modeling based on NWP information and geographical data such as landform, surface roughness, and turbulence intensity of the wind farm. It emphasizes the optimization of physical solution rules and the quality of NWP data. However, due to the complexity of the numerical simulation process and the extensive computational requirements, the physical method often results in lower prediction accuracy for ultra-short-term wind power forecasting (Chen et al., 2022). Consequently, physical methods are generally unsuitable for ultra-short-term prediction. In summary, this section provides a review of the application of data-driven methods (Yang et al., 2022c) in ultra-short-term wind power prediction, considering the current research focus in this field.
2.1 Point prediction method
The point prediction outcome refers to the specific value projected for a given future prediction time. Over the last decade, a significant proportion of domestic and international studies have focused on the point prediction research of ultra-short-term wind power, leading to a wealth of research outcomes. Currently, research on ultra-short-term wind power point prediction methods has achieved significant maturity (See Appendix Table A1 for details). However, point predictions only provide deterministic values and cannot quantitatively represent the uncertainty of wind power. Based on the point prediction model, data-driven techniques used in ultra-short-term wind power prediction can be categorized into statistical methods, artificial intelligence methods, and combined prediction methods.
On the other hand, the fluctuation process-based modeling method is a newly developed ultra-short-term prediction technology that has emerged with the advent of big data in recent years (Wang et al., 2016; Han and Alexander, 2022). This approach takes advantage of the inherent persistence patterns within wind power sequences at an ultra-short-term scale. By analyzing and extracting the evolutionary patterns of historically similar fluctuation states, it divides and combines the sequences to judiciously determine the future processing state (Ding et al., 2019; Sun et al., 2022a; Zhu et al., 2023). The methodology entails several technical steps, such as extracting the principal fluctuation component of the power sequence, identifying local extreme points, generating composite fluctuation sequences, mining historical class fluctuation processes, and fusing future fluctuation trends (Zhang et al., 2019a). The technique is intricate, computationally demanding, and relies upon an ample availability of historical power data.
Through an examination of wind power fluctuation characteristics (Lin et al., 2012; Yang and Qi, 2015; Yang and Dong, 2016; Zhou et al., 2017), it has been substantiated that wind wave characteristics significantly impact prediction accuracy. Additionally, our research group has conducted extensive investigations into the division and fluctuation characteristics of wind power fluctuation processes, affirming that incorporating these inherent characteristics is an effective means of enhancing wind power prediction accuracy.
2.2 Probabilistic prediction
The volatility of wind resources and the increasing proportion of wind power lead to a significant rise in the uncertainty of wind power prediction. Point prediction merely predicts the expected value and cannot accurately depict the uncertainty of wind power output. Moreover, wind power exhibits violent fluctuations when weather patterns change abruptly. Thus, relying solely on point prediction cannot effectively assess potential risks or provide a safe power fluctuation range for power grid planning, operation, security, and stability analysis as a dispatching reference (Yu et al., 2023).
To address this issue, ultra-short-term probabilistic prediction emerges as a new prediction form, providing more comprehensive information and quantitatively reflecting the uncertainty of wind power. This has the added benefit of reducing spinning reserve capacity and cutting power grid operation costs (Yang et al., 2022d). Probabilistic forecasting can be classified based on the form of the prediction results, which includes interval prediction, quantile prediction, and probability density prediction (YanTangDai et al., 2021). Additionally, it can be divided into parametric and non-parametric methods depending on whether the probability distribution function needs to be assumed in advance (See Appendix Table A2 for details).
The parametric method involves transforming probabilistic prediction into a parameter estimation problem. It assumes that wind power or its prediction error follows a known distribution in advance, such as the Gaussian distribution function, exponential distribution function (Zhu et al., 2019), beta distribution function (Yuan et al., 2019), or a combination of these probabilistic distributions. The Maximum Likelihood Estimation (MLE) and Delta method are then employed to estimate the parameters of the distribution function. However, due to the subjective inclusion of prior knowledge in parametric methods, it is often challenging to fit the model to the real distribution (Ding et al., 2013). Consequently, the assumption concerning the shape of the distribution is a key focus of current research, with the Gaussian and Beta distributions being two commonly used choices.
The non-parametric method does not make assumptions about the distribution of wind power or prediction error in advance. Instead, it fits the distribution based on the characteristics and properties of the data itself. Common data analysis methods include the empirical cumulative distribution method (Pinson and Kariniotakis, 2010), Quantile Regression (QR) method (He et al., 2021), Kernel Density Estimation (KDE) (Xu et al., 2021), and Lower Upper Bound Estimation (LUBE) (Li et al., 2020).
3 Research on extreme value prediction considering extreme weather scenarios
Despite the progress made in ultra-short-term wind power prediction, current single-value prediction methods lack extreme weather prediction models, resulting in poor prediction accuracy and stability. Extreme weather events that significantly impact wind turbines can be classified into two main categories. Firstly, severe convective weather conditions like typhoons lead to significant and rapid changes in wind speed, causing extreme power fluctuations within a short period. Offshore wind power generation, in particular, is highly susceptible to typhoons. As offshore wind power continues to expand, the research on power prediction for offshore wind generation becomes a crucial future development focus. Secondly, extremely cold weather, including cold waves and freezes, has seen three times faster growth in total installed wind power capacity in cold climates across North America, Europe, and Asia compared to the global average annual growth rate of offshore wind capacity. Extremely low ambient temperatures trigger the shutdown of wind turbines due to low-temperature protection mechanisms, resulting in a sudden and substantial power deficit. Furthermore, despite the overall accuracy level of existing research on ultra-short-term wind power prediction meeting the required standards, there are significant local errors, increasing the risks of wind abandonment and peak load loss. Therefore, studying extreme value prediction under extreme weather scenarios holds great significance.
The research team involved in this work has a solid research foundation regarding ultra-short-term wind power forecasting technology in extreme scenarios. They propose an ultra-short-term segmented prediction method for offshore wind power based on the adaptive division of extreme weather periods, the technical flow chart is shown in Figure 2. To validate the algorithm’s effectiveness and superiority under extreme weather scenarios, wind farm data from Texas, United States was taken as the sample for verification. The selected validation dataset covers 4 days (from September 12 to 15, 2019), including extreme weather events. Specific improvement methods include a hybrid model based on the Convolutional Neural Network (CNN) and the Long and Short Time Memory (LSTM) network in the stationary period, combined with an Improved Attention Mechanism (IAM) for point prediction. Meanwhile, during periods of power timing mutation, the probabilistic prediction method based on variable bandwidth kernel density estimation is adopted.
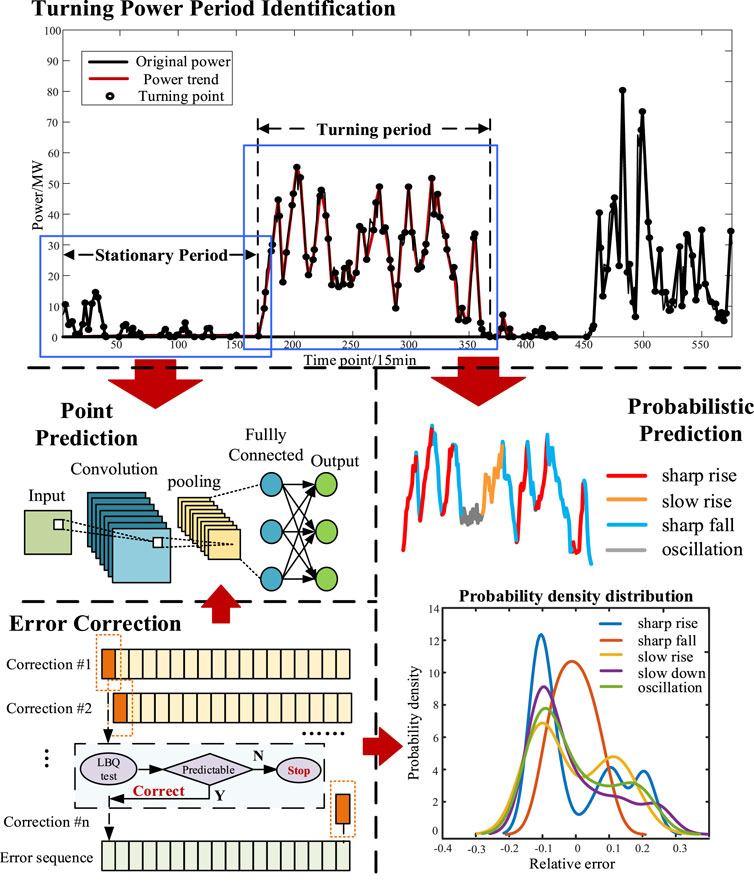
FIGURE 2. Framework of sub-forecast method for ultra-short-term wind power under transition weather environment.
Among them, the evaluation indexes of point prediction performance include Root Mean Square Error (RMSE) and Mean Absolute Percentage Error (MAPE). The calculation formulas are as follows:
Where,
And the evaluation indexes of probability prediction performance include the prediction interval coverage index, reliability index, and comprehensive performance index to measure the overall performance of the forecast. The calculation formulas are as follows:
Where:
The Prediction Interval Coverage Percentage (PICP) reflects the probability of the actual value falling within the upper and lower bounds of the prediction interval. A PICP less than a renders the prediction invalid, while a higher PICP indicates a greater probability of the actual power falling within the prediction limits, thereby enhancing the prediction efficacy. The Reliability index Ra measures the deviation between the interval coverage rate and the preset confidence level. A positive Ra implies a favorable deviation with higher reliability than the given confidence level. The Prediction Interval Average Width (PINAW) evaluates the clarity of the prediction model. When the predicted results have the same PICP, a smaller PINAW corresponds to better prediction accuracy. Both the accuracy of point prediction and probability prediction results for full-time periods and subsection prediction were quantitatively analyzed and presented in Figure 3 and Table 1, respectively.
Figure 4 displays the combined prediction results wherein the actual power timing curve lies within the estimated confidence interval. The point prediction period exhibits a high degree of fitting between the predicted and actual power curves. During the probabilistic prediction period, the lower confidence interval surrounds the higher confidence interval, effectively avoiding quantile crossovers. This demonstrates the proposed method’s strong overall performance, especially its adaptability for predicting offshore wind power in extreme weather conditions. However, it is essential to focus on real-time detection and identification of turning weather periods.
4 Conclusion and future trends
Due to the large proportion of wind power in the power system, the inherent randomness, intermittency and uncertainty of wind power pose major challenges to grid connection, power system scheduling and consumption. In this paper, the literature of ultra short term wind power forecasting is reviewed, and the advantages and disadvantages and applicability of point forecasting and probability forecasting are compared and analyzed. In addition, taking a wind farm as an example, a case study of ultra-short term wind power accurate forecasting method under extreme scenarios is carried out to prove the feasibility of the proposed combined forecasting method. In the future, combining numerical weather forecasts with real-time meteorological data warnings should be considered to establish an advanced prediction model for such transitional periods. This will further enhance the accuracy and timeliness of ultra-short-term wind power prediction in extreme weather scenarios.
In addition, although China has made great progress in ultra-short term wind power forecasting, there are still challenges in dealing with the uncertainties of wind power, which are reflected in the following aspects: 1) The improvement of the accuracy of electric power forecasting is limited by the level of NWP, and the progress is slow (Liu H. et al., 2020; Niu and Ji, 2020); 2) It is an urgent task to solve the problem of missing sample data in data processing (Zhang et al., 2021; Fu et al., 2023b; Tong et al.; Wang et al., 2023); 3) Due to the limitations of the current time scale of numerical weather forecasting, it is necessary to study short-term and immediate forecasting with more practical applications (Kumar et al., 2022); 4) With the explosive growth of newly built new energy stations, it is particularly important to improve the online adaptive learning ability (Li et al., 2021) of the model due to the more complex operating conditions of the equipment in these scenarios and the more changeable meteorological conditions.
Data availability statement
The raw data supporting the conclusion of this article will be made available by the authors, without undue reservation.
Author contributions
GY: Writing–review and editing. LS: Writing–original draft. QD: Project administration, Writing–review and editing. GC: Project administration, Writing–review and editing. SW: Project administration, Writing–review and editing. DX: Software, Writing–review and editing. XC: Investigation, Writing–review and editing. WL: Formal Analysis, Writing–review and editing.
Funding
The author(s) declare financial support was received for the research, authorship, and/or publication of this article. The process of writing this paper was supported by the project of the National Natural Science Foundation of China, “Research on Accurate Prediction and Dynamic Frequency Response Method of New Energy Power Generation under Changing Meteorological Environment” (52207121).
Conflict of interest
Authors QD, GC, and SW were employed by Power Dispatching Control Center of State Grid Shaanxi Electric Power Co., Ltd.
The remaining authors declare that the research was conducted in the absence of any commercial or financial relationships that could be construed as a potential conflict of interest.
Publisher’s note
All claims expressed in this article are solely those of the authors and do not necessarily represent those of their affiliated organizations, or those of the publisher, the editors and the reviewers. Any product that may be evaluated in this article, or claim that may be made by its manufacturer, is not guaranteed or endorsed by the publisher.
References
Aslam, M., Kim, J. S., and Jung, J. (2023). Multi-step ahead wind power forecasting based on dual-attention mechanism. Energy Rep. 9, 239–251. doi:10.1016/j.egyr.2022.11.167
Banik, A., Behera, C., Sarathkumar, T. V., and Goswami, A. K. (2020). Uncertain wind power forecasting using LSTM-based prediction interval. IET Renew. Power Gener. 14 (14), 2657–2667. doi:10.1049/iet-rpg.2019.1238
Chen, Y., Hu, X., and Zhang, L. (2022). A review of ultra-short-term forecasting of wind power based on data decomposition-forecasting technology combination model. Energy Rep. 8, 14200–14219. doi:10.1016/j.egyr.2022.10.342
Ding, M., Zhang, C., Wang, B., Bi, R., Miao, L. Y., and Che, J. F. (2019). Short-term forecasting and error correction of wind power based on power fluctuation process. Automation Electr. Power Syst. 43 (3), 2–9. doi:10.7500/AEPS20180322011
Ding, T., Feng, D., Lin, X., Chen, J., and Chen, L. (2017). Ultra-short-term wind speed prediction based on modified ARIMA-GARCH model. Power Grid Technol. 41 (06), 1808–1814.
Ding, H., Song, Y., Hu, Z., Wu, J., and Fan, X. (2013). The probability density function of day-ahead wind power forecast errors based on power curves of wind farms. Proc. CSEE 33 (34), 136–144. doi:10.13334/j.0258-8013.pcsee.2013.34.019
Fu, W., Fu, Y., Li, B., Zhang, H., Zhang, X., and Liu, J. (2023). A compound framework incorporating improved outlier detection and correction, VMD, weight-based stacked generalization with enhanced DESMA for multi-step short-term wind speed forecasting. Appl. Energy 348, 121587. doi:10.1016/j.apenergy.2023.121587
Fu, W., Jiang, X., Li, B., Tan, C., Chen, B., and Chen, X. (2023b). Rolling bearing fault diagnosis based on 2D time-frequency images and data augmentation technique. Meas. Sci. Technol. 34 (4), 045005. doi:10.1088/1361-6501/acabdb
Han, C., and Alexander, V. (2022). Reducing forecasting error by optimally pooling wind energy generation sources through portfolio optimization. Energy 239, 122099. doi:10.1016/j.energy.2021.122099
Hanifi, S., Liu, X., Lin, Zi, and Lotfian, S. (2020). A critical review of wind power forecasting methods—past, present and future. Energies 13 (15), 3764. doi:10.3390/en13153764
He, Y., Li, H., Wang, S., and Yao, X. (2021). Uncertainty analysis of wind power probability density forecasting based on cubic spline interpolation and support vector quantile regression. Neurocomputing 430, 121–137. doi:10.1016/j.neucom.2020.10.093
He, Y., and Wang, Y. (2021). Short-term wind power prediction based on EEMD–LASSO–QRNN model. Appl. Soft Comput. 105, 107288. doi:10.1016/j.asoc.2021.107288
Hu, Q., Zhang, R., and Zhou, Y. (2016). Transfer learning for short-term wind speed prediction with deep neural networks. Renew. Energy 85, 83–95. doi:10.1016/j.renene.2015.06.034
Hui, Li, Liu, D., and Yao, D. (2021). Research and judgment on the development of China's power system facing the goal of carbon peaking and carbon neutrality. Proc. CSEE 41 (18), 6245–6259. doi:10.13334/j.0258-8013.pcsee.210050
Kumar, B., Abhishek, N., Chattopadhyay, R., George, S., Singh, B. B., Samanta, A., et al. (2022). Deep learning-based short-range forecasting of Indian summer monsoon rainfall using earth observation and ground station datasets. Geocarto Int. 37, 17994–18021. doi:10.1080/10106049.2022.2136262
Li, C., Tang, G., Xue, X., Chen, X., Wang, R., and Zhang, C. (2020). The short-term interval prediction of wind power using the deep learning model with gradient descend optimization. Renew. Energy 155, 197–211. doi:10.1016/j.renene.2020.03.098
Li, Z., Wu, L., and Xu, Y. (2021). Risk-averse coordinated operation of a multi-energy microgrid considering voltage/var Control and thermal flow: an adaptive stochastic approach IEEE transactions on smart grid. Early access, 12 3914-3927. doi:10.1109/TSG.2021.3080312
Li, Z., Wu, L., Xu, Y., Wang, L., and Yang, N. (2023). “Distributed tri-layer risk-averse stochastic game approach for energy trading among multi-energy microgrids”. Applied Energy. 331 120282 (accepted). doi:10.1016/j.apenergy.2022.120282
Lin, Ye, and Liu, P. (2011). Short-term wind power portfolio prediction model based on empirical modal decomposition and support vector machine. Proc. CSEE 31 (31), 102–108. doi:10.13334/j.0258-8013.pcsee.2011.31.014
Lin, W., Wen, J., and Ai, X. (2012). Study on the probability distribution of wind power fluctuation characteristics. Proc. CSEE 32 (01), 38–46+20. doi:10.13334/j.0258-8013.pcsee.2012.01.010
Liu, A., Xue, Y., and Hu, J. (2015). Ultra-short-term wind power forecasting based on SVM optimized by GA. Power Syst. Prot. Control 43 (2), 90–95.
Liu, B., Zhao, S., Yu, X., Zhang, L., and Wang, Q. (2020). A novel deep learning approach for wind power forecasting based on WD-LSTM model. Energies 13 (18), 4964. doi:10.3390/en13184964
Liu, H., Tian, H.-Q., and Li, Y.-F. (2012). Comparison of two new ARIMA-ANN and ARIMA-Kalman hybrid methods for wind speed prediction. Appl. Energy 98, 415–424. doi:10.1016/j.apenergy.2012.04.001
Liu, H., Yang, R., and Duan, Z. (2020). Wind speed forecasting using a new multi-factor fusion and multi-resolution ensemble model with real-time decomposition and adaptive error correction. Energy Convers. Manag. 217, 112995. doi:10.1016/j.enconman.2020.112995
Liu, H., Yang, R., Wang, T., and Zhang, L. (2020). A hybrid neural network model for short-term wind speed forecasting based on decomposition, multi-learner ensemble, and adaptive multiple error corrections. Renew. Energy 11, 002. doi:10.1016/j.renene.2020.11.002
Lu, P., Ye, L., Pei, M., Zhao, Y., Dai, B., and Li, Z. (2022). Short-term wind power forecasting based on meteorological feature extraction and optimization strategy. Renew. Energy 184, 642–661. doi:10.1016/j.renene.2021.11.072
Ma, J., Yang, M., and You, L., (2020). Ultra-short-term probabilistic wind turbine power forecast based on empirical dynamic modeling. IEEE Trans. Sustain. Energy 11 (2), 906–915. doi:10.1109/tste.2019.2912270
Niu, D., and Ji, H. (2020). Quantitative analysis method for errors introduced by physical prediction model of wind power. Autom. Electr. Power Syst 44 (08), 57–65. doi:10.7500/AEPS20191224003
Niu, D., Sun, L., Yu, M., and Wang, K. (2022). Point and interval forecasting of ultra-short-term wind power based on a data-driven method and hybrid deep learning model. Energy 254, 124384. Part A. doi:10.1016/j.energy.2022.124384
Pinson, P., Kariniotakis, G., Nielsen, H. A., Nielsen, T. S., and Madsen, H. (2006). “Properties of quantile and interval forecasts of wind generation and their evaluation,” in Proceeding of the European Wind Energy Conference and Exhibition, Athens, Greece, February 27-March 2, 2006 .
Pinson, P., and Kariniotakis, G. (2010). Conditional prediction intervals of wind power generation. IEEE Trans. Power Syst. 25 (4), 1845–1856. doi:10.1109/tpwrs.2010.2045774
Qiao, Y., Lu, Z., and Yong, M. I. N. (2017). A method to improve the accuracy of wind power prediction. Power Grid Technol. 41 (10), 3261–3269. doi:10.13335/j.1000-3673.pst.2017.1581
Ren, D., Xiao, J., and Hou, J., (2022). Research on the construction and evolution of new electric power system under double carbon target. Power Grid Technol 46 (10), 3831–3839. doi:10.13335/j.1000-3673.pst.2022.0387
Singh, S. N., and Mohapatra, A. (2019). Repeated wavelet transform based ARIMA model for very short-term wind speed forecasting. Renew. Energy 136, 758–768. doi:10.1016/j.renene.2019.01.031
Su, X., Zhou, W., Li, C., Lin, X., Chen, Y., Hu, X., and Li, L. (2022). Explainable offshore wind power output prediction based on dual-attention LSTM neural network. Automation Electr. Power Syst 46 (07), 141–151. doi:10.1080/15567036.2023.2276901
Sun, Y., Huang, Y., and Yang, M. (2022). Ultra-short-term wind power interval prediction based on fluctuating process partitioning and quantile regression forest. Front. Energy Res. 10, 317. doi:10.3389/fenrg.2022.867719
Sun, Y., Wang, X., and Yang, J. (2022). Modified particle swarm optimization with attention-based LSTM for wind power prediction. Energies 15 (12), 4334. doi:10.3390/en15124334
Tong, J.-H., Wu, Z.-G., and Xie, Y. (2022) Ultra-short term wind power forecast in the absence of Meteorological Data. J. Power Syst. Automation 34 (03), 142–150. doi:10.19635/j.cnki.csu-epsa.000906
Wan, C., Lin, J., Wang, J., Song, Y., and Dong, Z. Y. (2016). Direct quantile regression for nonparametric probabilistic forecasting of wind power generation. IEEE Trans. Power Syst. 32 (4), 2767–2778. doi:10.1109/tpwrs.2016.2625101
Wang, H., Wang, G., Li, G., Peng, J., and Liu, Y. (2016). Deep belief network-based deterministic and probabilistic wind speed forecasting approach. Appl. Energy 182, 80–93. doi:10.1016/j.apenergy.2016.08.108
Wang, J., Yan, G., Ren, M., Xu, X., Ye, Z., and Zhu, Z. (2023). Short-term photovoltaic power prediction based on transfer learning and considering sequence uncertainty. J. Renew. Sustain. Energy 15 (1), 013501. doi:10.1063/5.0126788
Wang, Q., Yu, Z., Ye, R., Lin, Z., and Tang, Y. (2019). An ordered curtailment strategy for offshore wind power under extreme weather conditions considering the resilience of the grid. IEEE Access 7, 54824–54833. doi:10.1109/access.2019.2911702
Wang, Y., Hu, Q., Srinivasan, D., and Wang, Z. (2018). Wind power curve modeling and wind power forecasting with inconsistent data. IEEE Trans. Sustain. Energy 10 (1), 16–25. doi:10.1109/tste.2018.2820198
Wang, Z., Rui, P., and Feng, S. (2017). A short-term wind power portfolio forecasting method based on dynamic correction of weighting coefficients. Power Syst. Technol 41 (02), 500–507. doi:10.13335/j.1000-3673.pst.2016.0953
Wen, B. (2007). Research on optimization and correction of generation planning deviation under power market conditions. Proceedings of the CSEE (13), 111–116. doi:10.3321/j.issn:0258-8013.2007.13.020
Wu, Y., Su, P., Wu, T., Hong, J. S., and Hassan, M. Y. (2018). Probabilistic wind-power forecasting using weather ensemble models. IEEE Trans. Industry Appl. 54 (6), 5609–5620. doi:10.1109/tia.2018.2858183
Xu, H., Chang, Y. Q., Wang, F. L., Wang, S., and Yao, Y. (2021). Univariate and multivariable forecasting models for ultra-short-term wind power prediction based on the similar day and LSTM network. J. Renew. Sustain. Energy 13, 6. doi:10.1063/5.0027130
Xu, P., Fu, W., Lu, Q., Zhang, S., Wang, R., and Meng, J. (2023). Stability analysis of hydro-turbine governing system with sloping ceiling tailrace tunnel and upstream surge tank considering nonlinear hydro-turbine characteristics. Renew. Energy 210, 556–574. doi:10.1016/j.renene.2023.04.028
Yang, N., Dong, Z., Wu, L., Zhang, L., Shen, X., Chen, D., et al. (2022). A comprehensive review of security-constrained unit commitment. J. Mod. Power Syst. Clean Energy 10 (No.3), 562–576. doi:10.35833/mpce.2021.000255
Yang, N., Qin, T., Wu, L., Huang, Yu, Huang, Y., Xing, C., et al. (2022). A multi-agent game based joint planning approach for electricity-gas integrated energy systems considering wind power uncertainty. Electr. Power Syst. Res. 204, 107673. doi:10.1016/j.epsr.2021.107673
Yang, N., Yang, C., Wu, L., Shen, X., Jia, J., Li, Z., et al. (2022). Intelligent data-driven decision-making method for dynamic multisequence: an E-Seq2Seq-based SCUC expert system. IEEE Trans. Industrial Inf. 18 (No.5), 3126–3137. doi:10.1109/tii.2021.3107406
Yang, N., Yang, C., Xing, C., Ye, Di, Jia, J., Chen, D., et al. (2022). Deep learning-based SCUC decision-making: an intelligent data-driven approach with self-learning capabilities. Wiley-Blackwell) 16 (No.4), 629–640. doi:10.1049/gtd2.12315
Yang, M., and Dong, J. (2016). Research on wind power fluctuation characteristics based on a hybrid distribution model. Proc. CSEE 36 (S1), 69–78. doi:10.13334/j.0258-8013.pcsee.152482
Yang, M., and Qi, Y. (2015). Analysis of wind power fluctuation characteristics based on phase space reconstruction and its impact on prediction error. Proc. CSEE 35 (24), 6304–6314. doi:10.13334/j.0258-8013.pcsee.2015.24.005
YanTangDai, C. Y. J., Wang, C., and Wu, S. (2021). Uncertainty modeling of wind power frequency regulation potential considering distributed characteristics of forecast errors. Prot. Control Mod. Power Syst. 6 (1), 22–13. doi:10.1186/s41601-021-00200-3
Yesilbudak, M., Sagiroglu, S., and Colak, I. (2017). A novel implementation of kNN classifier based on multi-tupled meteorological input data for wind power prediction. Energy Conversion and Management, 135. 434-444. doi:10.1016/j.enconman.2016.12.094
Yu, G., Liu, C., Tang, Bo, Chen, R., Lu, L., Cui, C., et al. (2022). Short-term wind power prediction for regional wind farms based on spatial-temporal characteristic distribution. Renew. Energy 199, 599–612. doi:10.1016/j.renene.2022.08.142
Yu, G., Liu, Lu, and Tang, B., (2021). Improved hybrid neural network ultra-short-term PV power prediction method based on cloud map feature extraction. Proceeding CSEE 41 (20), 6989–7003. doi:10.13334/j.0258-8013.pcsee.201929
Yu, G., Liu, Lu, Tang, B., Wang, S., and Dong, Q. (2022). Research on ultra-short-term piecewise prediction method for offshore wind power considering transformational weather. Proc. CSEE 42 (13), 4859–4871. doi:10.1109/TPWRS.2022.3224557
Yu, G. Z., Lu, L., Tang, B., Wang, S. Y., and Chung, C. Y. (2023). Ultra-short-term wind power subsection forecasting method based on extreme weather. IEEE Trans. Power Syst. 38 (6), 5045–5056. doi:10.1109/TPWRS.2022.3224557
Yuan, X., Chen, C., Jiang, M., and Yuan, Y. (2019). Prediction interval of wind power using parameter optimized Beta distribution based LSTM model. Appl. Soft Comput. 82, 105550. doi:10.1016/j.asoc.2019.105550
Zhang, Y., Han, J., Pan, G., Xu, Y., and Wang, F. (2021). A multi-stage predicting methodology based on data decomposition and error correction for ultra-short-term wind energy prediction. J. Clean. Prod. 292, 125981. doi:10.1016/j.jclepro.2021.125981
Zhang, Y., Hao, S., and Qian, X. (2019). Interval prediction of wind power based on error composition and bootstrap method. Power Syst. Technol. 43, 1941–1947. doi:10.13335/j.1000-3673.pst.2018.3023
Zhang, Y., Sun, H., and Guo, Y. (2019). Wind power prediction based on PSO-SVR and grey combination model. IEEE Access 7, 136254–136267. doi:10.1109/access.2019.2942012
Zhou, Y., Yu, G., Liu, J., Song, Z., and Kong, P. (2021). Offshore wind power prediction based on improved long-term recurrent convolutional neural network. Power Syst. Autom. 45 (03), 183–191. doi:10.7500/AEPS20191212003
Zhou, T., Chen, L., and Jian, L. I. (2017). Research on wind power fluctuation characteristics based on finite mixture Laplace model. Power Syetem Technol. 41 (02), 543–550. doi:10.13335/j.1000-3673.pst.2016.0844
Zhu, B., Liu, Yu, Zhi, S., Wang, K., and Liu, J. (2023). A family of bipolar high step-up zeta–buck–boost converter based on “coat circuit”. IEEE Trans. Power Electron. 38 (3), 3328–3339. doi:10.1109/tpel.2022.3221781
Zhu, X., Chen, R., Ding, K., Gong, D., Xi, W., Yao, H., et al. (2019). “Error analysis of ultra short term wind power prediction model and effect on the power system frequency,” in Proceedings of the 2019 IEEE PES Asia-Pacific Power Energy Eng. Conf. (APPEEC), Macao, China, 01-04 December 2019, 1–6. doi:10.1109/APPEEC45492.2019.8994671
Appendix
Keywords: ultra-short-term power prediction, numerical weather prediction, point prediction, probabilistic prediction, transitory weather
Citation: Yu G, Shen L, Dong Q, Cui G, Wang S, Xin D, Chen X and Lu W (2024) Ultra-short-term wind power forecasting techniques: comparative analysis and future trends. Front. Energy Res. 11:1345004. doi: 10.3389/fenrg.2023.1345004
Received: 27 November 2023; Accepted: 28 December 2023;
Published: 12 January 2024.
Edited by:
Wenlong Fu, China Three Gorges University, ChinaReviewed by:
Ning Tong, Guangdong University of Technology, ChinaMenglin Zhang, China University of Geosciences Wuhan, China
Copyright © 2024 Yu, Shen, Dong, Cui, Wang, Xin, Chen and Lu. This is an open-access article distributed under the terms of the Creative Commons Attribution License (CC BY). The use, distribution or reproduction in other forums is permitted, provided the original author(s) and the copyright owner(s) are credited and that the original publication in this journal is cited, in accordance with accepted academic practice. No use, distribution or reproduction is permitted which does not comply with these terms.
*Correspondence: Lingxu Shen, c2x4NjE0MTg5MUAxNjMuY29t