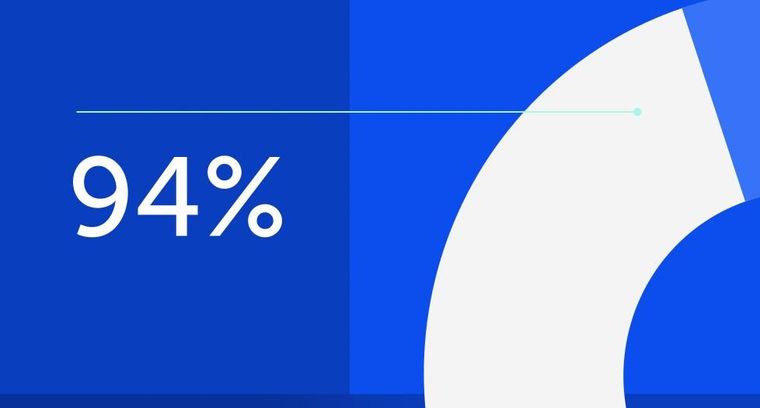
94% of researchers rate our articles as excellent or good
Learn more about the work of our research integrity team to safeguard the quality of each article we publish.
Find out more
ORIGINAL RESEARCH article
Front. Energy Res., 29 November 2023
Sec. Process and Energy Systems Engineering
Volume 11 - 2023 | https://doi.org/10.3389/fenrg.2023.1323077
This paper investigates the impact of the popularization and usage of the Internet on household electricity consumption in China, as well as the mediating role of sleep duration. By employing data from the China Family Panel Studies (CFPS) and employing the basic ordinary least squares (OLS) model, the mediation model, and the instrumental variable (IV) approach, we derive the following conclusions. The results from the basic OLS regression indicate a positive relationship between internet usage and household electricity expenditure, implying that households that use the Internet tend to have higher electricity bills. Subsequently, by introducing sleep duration as a mediating variable, we find that internet usage leads to shorter sleep duration, indirectly resulting in increased household electricity costs. To address potential endogeneity concerns, we employ the instrumental variable approach to correct for the impact of internet usage on household electricity consumption. In addition, through heterogeneity analysis, we found that internet usage impacts households with different characteristics.
The continuous growth of energy consumption on a global scale has brought about severe challenges in terms of energy security and environmental concerns. As one of the world’s largest energy consumers, China faces increasingly high energy demand and pressure to reduce carbon emissions. This situation urgently requires effective measures to promote energy efficiency and emissions reduction, aiming to achieve the goals of sustainable economic development and ecological civilization. In China’s energy consumption landscape, household electricity consumption plays a significant role within the overall energy consumption structure. Household energy consumption has always been a critical topic for achieving social sustainability.
In recent decades, the widespread availability of the Internet has achieved remarkable benefits on a global scale. As a rapidly developing country, China has made significant achievements as part of this trend. The ubiquity of the Internet has profoundly affected various aspects of society, becoming an indispensable part of people’s daily lives. On the one hand, the simplicity of Internet access has brought significant energy consumption challenges. In households, internet access involves various electronic devices such as computers, smartphones, and smart home appliances. The continuous operation and data transmission of these devices have increased the energy burden on households. Additionally, the prevalence of internet use has also impacted the sleep duration of households. With the popularity of the mobile Internet, an increasing number of people are using smartphones and other devices at night, leading to shortened sleep duration. The reduction in sleep duration may result in an extended total duration of use of various electrical appliances by family members, thus indirectly affecting household electricity consumption.
On the other hand, the widespread utilization of the Internet has enabled people to gain more access to knowledge and insights related to sustainable development and energy conservation. This newfound connectivity allows individuals to delve deeper into the intricacies of these concepts, fostering a heightened awareness of the need for responsible energy use. As people increasingly engage with online resources, they become better informed about innovative strategies, cutting-edge technologies, and practical tips for minimizing their ecological footprint. As they align their behaviors with sustainable principles and embrace energy-saving practices, households may reduce their energy consumption.
This study aims to comprehensively analyze the relationship between household internet use and electricity consumption through basic ordinary least squares (OLS) regression models, mediation effect models, and instrumental variable regression methods, providing valuable insights for energy-efficient internet utilization and environmental protection. By delving into how internet usage affects household electricity consumption and indirectly influences sleep duration, we can better understand the impact of the Internet’s proliferation on energy consumption. This can help in formulating effective energy-saving and emission reduction policies, optimizing household energy consumption patterns, and providing theoretical and practical support for achieving sustainable development goals.
Global energy consumption has long been a topic of much discussion. Over the past few centuries, the global energy supply has undergone significant changes, and we are currently in a continuous evolution of energy transformation (Ritchie et al., 2022). The new challenges presented in 2022 have raised concerns about rapidly rising energy prices and energy security (Raimi et al., 2022). With the ongoing development of the global economy and population growth, energy demand continues to rise, while environmental and climate change issues are becoming increasingly prominent (Hao, 2022). In recent years, the growth of global energy consumption has been primarily driven by major energy-consuming countries such as China, the United States, and India (Bp, 2023).
There are significant differences in global energy consumption among different regions and countries (Wu et al., 2018). Developed countries, such as the United States, European countries, and Japan (Ahmad and Zhang, 2020), play a crucial role in global energy consumption. However, as developing countries experience rapid economic growth, their energy demand also continuously increases (Keho, 2016). Emerging economies such as China and India have become the fastest-growing regions in global energy consumption, with their demand within the global energy market continuously increasing (Wang and Li, 2016).
Additionally, it is crucial to consider external factors that impact energy markets, such as oil price shocks. Recent research has shown that oil price shocks, particularly demand shocks, significantly affect clean energy markets, highlighting the interconnectedness of energy sectors with external events (Ren et al., 2023a).
To address their growing energy demands, countries need to formulate reasonable energy policies, promote efficient energy use, and encourage sustainable development (Sinha et al., 2018). Additionally, the development of clean energy and increased energy efficiency are essential for achieving an energy transition and reducing carbon emissions (Gielen et al., 2019). Therefore, addressing global energy consumption requires global cooperation and collective efforts to achieve the vision of a sustainable energy future (Valdes, 2021).
As one of the world’s largest energy consumers, China has attracted considerable attention due to its consumption patterns (Kang et al., 2015). With sustained rapid economic growth and the advancement of urbanization, China’s energy demand continues to rise, leading to environmental and climate challenges (Zhou et al., 2012). Fossil fuels, including coal, oil, and natural gas, dominate China’s energy consumption, especially coal, which still holds a significant share in the energy consumption mix (Abbasi et al., 2022). The extensive use of fossil fuels has exacerbated environmental pollution, resulting in a certain degree of social costs and welfare losses (Xu et al., 2019).
Distinct regional disparities exist in energy consumption across different areas of China, characterized by noticeable geographical agglomeration and spatial spillover effects (Yu et al., 2023). The eastern coastal regions represent the most concentrated area for energy consumption, primarily centered in large cities and economically developed regions (Liu et al., 2019). In contrast, the western regions, despite being rich in energy resources, exhibit relatively lower energy consumption levels (Liu et al., 2013a). Furthermore, China’s dependence on energy imports is gradually increasing, particularly with the rising demand for oil and natural gas imports (Wu, 2014).
It is essential to recognize that global energy markets can impact regional energy sectors. A study on the spillover effects of international crude oil markets on Chinese energy futures markets revealed significant risk spillovers, underlining the potential vulnerabilities in China’s energy sectors due to external events (Ren et al., 2023b). The study on geopolitical risk and firm idiosyncratic volatility among Chinese A-share listed companies found that geopolitical risks impact firms differently based on industry and ownership characteristics. This emphasizes the need to understand how external risks can affect corporate actions, including energy consumption (Ren et al., 2023c). Moreover, it is essential to consider digital transformation’s role in environmental performance. Recent research has explored how the peer effect of digital transformation influences corporate environmental performance among Chinese-listed companies, highlighting the influence of technological advancements on energy efficiency (Li et al., 2023).
To tackle the challenges posed by energy consumption growth and environmental pressures, the Chinese government is actively promoting energy structure adjustments and a green, low-carbon transformation (Liu et al., 2013b). In recent years, China has made significant progress in the field of energy transition, particularly in the expanding utilization of renewable energy sources such as hydro, wind, and solar energy (Li and Taeihagh, 2020). Additionally, enhancing energy efficiency has become a pivotal goal in China’s energy policy (Zhou et al., 2010). Through intensified energy technology innovation and the promotion of energy-saving measures, China has successfully lowered its energy consumption per unit of gross domestic product (GDP) (Li and Lin, 2018).
With the rapid development of the global economy and the acceleration of urbanization, household energy demand continues to grow, having become a crucial component of global energy consumption and exerting a significant impact on global climate change (Pablo-Romero et al., 2017). The primary sources of household energy consumption include electricity, natural gas, and other forms of fuel (Damette et al., 2018). Among these, electricity plays a vital role in household energy consumption, with increasing demand among households for electricity (Matsumoto et al., 2022). Within China, the primary sources of household energy consumption are electricity and natural gas (Zheng et al., 2014). Additionally, some households in developing countries still rely on traditional biomass fuels, such as firewood and biomass pellets, which are particularly common (Pachauri and Spreng, 2004).
The growth in household electricity consumption has also brought forth a series of environmental and energy challenges. The increased electricity consumption by households translates to greater energy resource demands, which could lead to the increased consumption of fossil fuels and carbon emissions, thereby exacerbating climate change issues (Hertwich and Peters, 2009). In this context, governments and energy agencies worldwide are continuously strengthening the monitoring and research of household energy consumption (Palm and Thollander, 2010). They are promoting the adoption of clean and efficient energy technologies, encouraging energy-saving and emissions-reduction behaviors in households, and fostering sustainable lifestyles to achieve the sustainable development of household energy consumption (Abrahamse and Steg, 2013).
Existing studies have analyzed various aspects of the factors influencing household electricity consumption. Katsoulakos et al. utilized data from Greece and found that factors such as household income, household size, housing area, housing age, number of rooms, number of bedrooms, air conditioning usage, and the installation of solar water heaters all have a significant positive impact on household electricity consumption (Kotsila and Polychronidou, 2021a). Paul et al., using U.S. data, discovered that policy measures such as energy efficiency standards and renewable energy regulations have a significant effect in reducing household electricity consumption (Paul et al., 2009). Das et al. employed data from the Bhutan Living Standards Survey (BLSS) and identified that household electricity consumption is influenced by factors including the head of household’s income level, age, education, and gender as well as the household’s electricity supply and location (Das et al., 2014).
Understanding how information flows and influences energy markets is increasingly important. A recent study on the information transmission between Chinese and international crude oil markets used a quantile-based framework to reveal the asymmetric and nonlinear transmission of information between these markets. These findings underline the significance of internal market dynamics in governing the relationship between Chinese and international oil markets, which holds crucial implications for energy market participants (Duan et al., 2023).
At the household level, Pothitou et al. found through their study that the extensive use of information and communication technology (ICT) entertainment devices contributes to increased household electricity consumption (Pothitou et al., 2017). Salahuddin et al., using Australian data, discovered that the proliferation and utilization of the Internet have led to increased electricity consumption, but at the same time, electricity consumption drives economic growth (Salahuddin and Alam, 2015). The research conducted by Morley et al. revealed that the usage of internet traffic contributes to an overall increase in electricity consumption, with a strong overlap observed between periods of high internet traffic and peak electricity usage (Morley et al., 2018).
Research conducted by Mei et al. revealed a strong and consistent association between the excessive use of technological devices and sleep-related issues, including shortened sleep duration, prolonged time to fall asleep, and decreased sleep quality (Mei et al., 2018). Lund et al. analyzed four sleep-related domains: time to fall asleep and difficulty falling asleep, sleep quality, sleep duration, and daytime fatigue. The study found that a higher overall electronic media usage was associated with shorter sleep duration among children and adolescents, while its impact on other sleep domains was less consistent (Lund et al., 2021). The study by Alimoradi et al. found that internet usage may affect sleep through various pathways, including delaying bedtime, increasing exposure to light, stimulating brain activity, disrupting the circadian rhythm, and inducing anxiety and depression (Alimoradi et al., 2019).
A study by Sukarno et al. identified that the operating duration of appliances is a crucial factor influencing household electricity consumption (Sukarno et al., 2017). Lőrincz et al., using data from the United Kingdom, found that household electricity consumption is notably lower during periods of sleep and rest activities (Lőrincz et al., 2021).
This paper contributes to the existing literature by shedding light on the complex relationship between the proliferation and utilization of the internet and household electricity consumption in China. The study deepens our understanding of the digital era’s influence on energy use by delving into this relatively unexplored territory. It introduces a novel mediating factor, sleep duration, which mediates the link between internet usage and electricity expenditure. By unveiling the indirect influence of internet usage on electricity costs through altered sleep patterns, the study highlights an additional pathway through which technology affects daily life and energy consumption.
With the widespread adoption and utilization of the Internet, the growing number of household Internet-enabled devices and the increasing frequency of use may result in higher electricity consumption. Household members typically simultaneously utilize multiple internet-connected devices such as smartphones, computers, and smart home appliances, which directly impact household electricity usage through their continuous operation and data transmission. In particular, the prevalence of smart home devices, including smart televisions, smart air conditioners, and smart lighting, further intensifies the demand for electricity in households due to their automated controls and remote operations.
In addition to the frequency of device usage, the Internet brings many entertainment and learning opportunities to household members, such as online shopping, streaming videos, e-learning, and remote work activities. These activities contribute to increased electricity consumption in households in terms of both frequency and duration. For instance, household members may use smartphones to watch movies or videos during the evening, and smart TVs might become the primary source of shared entertainment, both of which directly contribute to the rise in household electricity consumption. Moreover, the Internet’s ubiquity allows for a wider range of entertainment activities that can be carried out within the home, leading to an overall escalation in household electricity usage.
Notably, the extent to which internet use affects household electricity consumption may vary depending on specific household circumstances. Factors such as household size, age distribution, and occupation of household members may influence the impact of internet adoption on electricity consumption differently. Therefore, investigating how the widespread use of the Internet contributes to the increase in household electricity consumption necessitates considering various factors to obtain more comprehensive and accurate research conclusions (Kotsila and Polychronidou, 2021b).
In contrast, there are several channels suggesting that the integration of the Internet into daily routines might lead to a reduction in household electricity consumption. First, the Internet is a powerful medium for information dissemination and awareness building. As individuals gain access to a wealth of knowledge about sustainable living practices and energy-saving techniques, they become more inclined to adopt environmentally friendly behaviors. The accessibility of online resources, ranging from energy-efficient appliances to eco-conscious lifestyle choices, empowers individuals to make informed decisions that align with the broader goal of minimizing energy consumption.
Moreover, the Internet fosters a culture of interconnectedness and community engagement. Online platforms provide spaces for individuals to share experiences, tips, and success stories related to energy conservation. Through social media, blogs, and forums, people exchange ideas about optimizing energy use, collectively contributing to a more conscious consumption pattern. This virtual network of environmentally conscious individuals creates a ripple effect, influencing others to follow suit and adopt energy-saving practices in their homes.
Additionally, the Internet has enabled the development and widespread use of smart technologies and home automation systems. These innovations empower users to monitor and regulate their energy consumption with unprecedented precision. From remotely controlling thermostat settings to receiving real-time updates on energy usage, individuals can make immediate adjustments to optimize energy efficiency. This level of control not only reduces wastage but also encourages mindful energy usage as people actively engage with their consumption patterns.
The widespread adoption and usage of the Internet directly impact household electricity consumption and may influence household energy consumption indirectly through changes in household members’ sleep duration. In modern society, the prevalence of the Internet enables household members to have easy access to vast amounts of information and entertainment content, especially during the evening hours when individuals are often tempted to use smart devices such as smartphones and computers while in bed, leading to a reduction in sleep duration to some extent. With the widespread availability of smartphones and other internet-connected devices, household members are more prone to being engrossed in a cycle of using these devices at night. The continuous updates and allure of social media, short videos, electronic games, and other content make it difficult for household members to disengage from screens in a timely manner, affecting the quality and duration of sleep. The decrease in sleep duration not only affects the health and work efficiency of household members but also has the potential to impact the operating duration of household electrical devices, indirectly leading to variations in household electricity consumption.
Therefore, sleep duration is a potential mediating variable that may play a crucial mediating role between internet usage and household electricity consumption. Internet usage can directly influence sleep duration, and the reduction in sleep duration may further increase household electricity consumption. When investigating the impact of internet usage on household electricity consumption, it is essential to consider the mediating role of sleep duration to gain a more in-depth understanding of this relationship.
In conclusion, the theoretical framework of this study focuses on the proliferation and usage of the Internet, exploring the impact of Internet usage on household electricity consumption from two perspectives: the direct increase in household electricity consumption and the indirect influence through changes in household members’ sleep duration. This comprehensive framework provides an important perspective for further research on the effects of internet usage on household electricity consumption while also offering theoretical and empirical support for the development of effective energy-saving and energy consumption optimization strategies.
Based on the established theoretical framework, we can propose a number of research hypotheses. In this paper, we investigate the relationship between internet usage and household electricity consumption while considering the mediating role of sleep duration. Therefore, we propose the following research hypotheses.
Internet usage’s direct impact on household electricity consumption outweighs its positive impact on household sustainable living practices, and thus internet usage is positively correlated with household electricity consumption.
Sleep duration acts as a mediator between internet usage and household electricity consumption, where internet usage reduces household members’ sleep duration, consequently increasing household electricity consumption.
The household and individual data used in this study are derived from the China Family Panel Studies (CFPS). CFPS is a long-term tracking survey project initiated by the Institute of Sociology at the Chinese Academy of Social Sciences in 2008, aiming to comprehensively understand the social and economic status and habits of Chinese families. This survey covers households and individuals from various regions across the country, including both urban and rural areas, thus ensuring a high level of representativeness and reliability. For this research, data from the 2018 CFPS survey are selected as the primary source for our study sample. The choice of using data from 2018 is based on the following considerations: First, the data from 2018 are relatively complete and stable, and unaffected by major events such as the beginning of the COVID-19 pandemic in 2020. Therefore, it provides favorable conditions for accurately grasping the relationship between internet usage and household electricity consumption. Second, selecting more recent data increases the timeliness and practical significance of the research findings, thereby better guiding energy conservation and energy consumption management and planning in modern society. Consequently, the data from 2018 possess relatively good data quality and timeliness, providing a reliable research foundation for our study.
Additionally, to acquire macrolevel data for various provinces, this research also adopts relevant data published by the National Bureau of Statistics of China. These macrolevel data include economic indicators and infrastructure development situations for each province, offering comprehensive background information and a reference basis for the study.
The data used in this study are derived from the China Family Panel Studies (CFPS), which consists of two parts: a household database and an individual database. Each household and each individual has a unique identifier, namely, the household ID and individual ID. With these two identifiers, we can accurately link individual data with their corresponding household data. The linked data are integrated to form a comprehensive dataset that includes both household information and information about the household’s head (i.e., the individual who serves as the household representative). Consequently, we can simultaneously determine various characteristics of the household (e.g., electricity consumption information) and personal features of the household head (e.g., internet usage).
By consolidating the data, we gain a more comprehensive and holistic perspective, enabling a more thorough examination of the impact of internet usage on household electricity consumption while controlling for the personal characteristics of the household head to mitigate the influence of other factors on the study’s outcomes. Through this data integration and analysis, we aim to derive more accurate and reliable conclusions, offering empirical support and an in-depth understanding of the relationship between internet usage and household electricity consumption.
Data collected for this study underwent rigorous data cleaning and organization. First, we scrutinized all variables for missing values and employed appropriate methods to handle them, such as removing samples with excessive missing values or using imputation techniques to fill in missing values, ensuring data completeness and usability. Second, we further examined all variables for outliers, and based on the actual data conditions, employed suitable methods, including the correction or exclusion of outliers, to ensure the accuracy and reliability of the analysis results. Through these data cleaning and organization steps, we ensured the quality of the data used in the study and prepared for the subsequent statistical analyses.
To examine Hypothesis 1, which aim to verify the direct impact relationship between internet usage and household electricity consumption, we constructed a baseline ordinary least squares (OLS) regression model. In this model, we took the logarithm of household electricity bills as the dependent variable and internet usage (determined by the responses to two questions: “mobile internet usage” and “computer internet usage”) as the core independent variable. The form of the baseline OLS regression model is as follows:
In the model,
To investigate whether the impact of internet usage on household electricity consumption is mediated through sleep duration (Hypothesis 2), we introduce sleep duration as a mediator variable and employ a stepwise approach to establish the mediation model. Our mediation model is set as follows.
Step 1. Regression analysis of the influence of internet usage on sleep duration.
Step 2. Regression analysis of the impact of sleep duration on household electricity expenses.
Step 3. Regression analysis of the impact of internet usage on household electricity expenses while controlling for the mediator variable, sleep duration.
Table 1 presents the regression results of the basic OLS model. From the results in the first column of the table, it can be observed that the variable “Internet Usage” is positive and statistically significant at the 1% level. This indicates that the widespread use of the Internet positively impacts household electricity expenses, leading to an increase in electricity consumption. This finding is in line with Hypothesis 1, which posited that the proliferation and use of the Internet would result in higher household electricity expenses.
TABLE 1. Results of the basic ordinary least squares (OLS) regression and mediation model regression.
The regression results of the stepwise mediation model are presented in Table 1, columns 2 to 4. In the first step of the regression, the “Internet Usage” coefficient is negative and significant at the 1% level. This suggests that internet usage has a negative impact on the sleep duration of household heads, indicating that using the Internet may lead to a reduction in their sleep duration.
In the second step of the regression, the “Sleep Duration” coefficient is negative and significant at the 1% level. This indicates that a shorter sleep duration is associated with higher household electricity expenses, meaning that households with shorter sleep durations tend to consume more electricity.
In the third step of the regression, both “Internet Usage” and “Sleep Duration” are significant at the 1% level. The “Internet Usage” coefficient is positive, while the “Sleep Duration” coefficient remains negative. These results suggest that both internet usage and sleep duration have significant effects on household electricity expenses. Specifically, internet usage has a direct positive effect on household electricity expenses, while sleep duration has a direct negative effect.
In summary, based on the results of the stepwise mediation model, we can preliminarily draw the following conclusions: internet usage has a direct positive impact on household electricity expenses, indicating that using the Internet leads to an increase in household electricity consumption. Additionally, internet usage also indirectly affects household electricity expenses by influencing the sleep duration of the household heads, as using the Internet is associated with a shorter sleep duration, which, in turn, leads to higher household electricity expenses.
While the basic ordinary least squares (OLS) regression reveals the overall association, a more comprehensive understanding of the impact of internet usage on household energy consumption requires a nuanced investigation into whether significant variations exist across different demographic groups. Although the fundamental OLS regression provides insights into the overall impact, it is imperative to recognize that diverse groups may exhibit distinct responses to the same influencing factor in real-life scenarios. Therefore, the primary objective of heterogeneity analysis is to explore potential divergences in the effect of internet usage on household energy consumption under varying contextual conditions. In this section, we employ heterogeneity analysis techniques to further deepen our comprehension of the complex relationship between internet usage and household energy consumption.
The significance of conducting heterogeneity analysis lies in its ability to accurately identify response patterns of distinct individuals and groups under specific contexts, thereby facilitating a finer-grained analysis of complex interactions among influencing factors. Furthermore, heterogeneity analysis also holds the potential to offer guidance for formulating targeted policies, enhancing the feasibility of policies, and better meeting the needs of specific demographic segments.
To conduct the heterogeneity analysis, we employ a stratified analysis approach to identify the impact of internet usage on household energy consumption under various contextual conditions. By contrasting the regression coefficients across different groups, potential heterogeneity effects can be unveiled, thus furnishing more robust evidence and enabling deeper comprehension of this complex relationship. The regression outcomes of this section are presented in Table 2.
Household behaviors are often influenced by a multitude of factors, among which the household registration type is deemed a potential significant influencer. Serving as a representative social indicator, household registration type somewhat reflects the socioeconomic background, geographical location, and occupational and social status of a household’s members. In China, household registration types can be broadly categorized as agricultural and nonagricultural registrations, with these two categories exhibiting noticeable differences in lifestyle, social environment, and economic conditions. These disparities may impact the household’s way of life, consumption habits, and energy utilization patterns, thereby introducing heterogeneous effects on the relationship between internet usage and energy consumption.
The results of the heterogeneity analysis are presented in columns 1 and 2 of Table 2. It is evident that within the agricultural registration group, there exists a significant positive correlation between internet usage and household energy consumption, with a regression coefficient of 0.1023 at the 1% significance level. This indicates a pronounced positive link between the proliferation and utilization of the Internet and higher energy consumption within agricultural households. Conversely, among the nonagricultural registration group, the impact of internet usage on household energy consumption is not statistically significant, as evidenced by the regression coefficient not meeting the 10% significance threshold. This finding suggests that within nonagricultural households, the diffusion and utilization of the Internet do not appear to have a notable effect on household energy consumption.
The emergence of this heterogeneity phenomenon may be attributed to the combined influence of several factors. First, rural areas typically offer limited recreational activities, and thus, internet usage such as online entertainment and e-commerce might rapidly fill the leisure time of registered agricultural households, consequently leading to an increase in energy consumption associated with internet usage. In contrast, urban areas tend to provide a more diverse array of entertainment options, potentially limiting the impact generated by internet usage on energy consumption.
Furthermore, the process of urbanization has exerted distinct influences on energy consumption in rural and urban areas. As urbanization has advanced, urban regions have been at the forefront of introducing advanced energy utilization technologies and facilities, consequently achieving effective control over energy consumption. Conversely, in rural areas, the adoption of similar advancements has been comparatively lagging due to inherent conditions there. This aspect is also a plausible factor contributing to the observed disparities.
The diverse marital statuses within Chinese society can be generally grouped into households with partners and those without. These different marital statuses may lead to variations in household energy consumption. Marital relationships entail the amalgamation of household resources, adjustments in lifestyles, and coordination among family members. Households with partners are typically engaged in shared living and resource management, whereas those without partners might exhibit more independence and individualism. Additionally, marital status could mold the societal roles and resource allocation of family members, further influencing consumption patterns and energy utilization within the household. Considering the comprehensive impact of marital status on family life, it is reasonable to postulate that it has the potential to induce heterogeneity in the relationship between internet usage and household energy consumption.
The results of the heterogeneity analysis are presented in columns 3 and 4 of Table 2. In households with partners, the regression coefficient between internet usage and energy consumption is 0.0822, showing a positive correlation at the 1% significance level. Conversely, in households without partners, the regression coefficient is 0.1175, displaying a positive correlation at the 10% significance level. This suggests that the impact of internet usage on energy consumption is more pronounced in households without partners.
We contend that households with partners, due to the need for harmonizing lifestyles toward common goals, exhibit relatively stable consumption patterns, resulting in a minor influence of internet usage on habits and, consequently, energy consumption. In contrast, in households without partners, the greater flexibility in the head of the household’s lifestyle results in a more substantial impact of internet usage on energy consumption.
The presence of children in a household is a factor can significantly influence over household behaviors. The existence of children typically triggers variations in resource allocation, lifestyles, and consumption habits within the family. In this context, it is reasonable to hypothesize that the presence or absence of children in a household could lead to significant heterogeneity in the relationship between internet usage and energy consumption.
The results of the heterogeneity analysis are presented in columns 5 and 6 of Table 2. The results reveal that in households with children, the regression coefficient between internet usage and energy consumption is 0.1314, showing a significant relationship at the 1% significance level. However, in households without children, the regression coefficient for internet usage is 0.0794, displaying a significant relationship at the 1% significance level. This suggests that the impact of internet usage on energy consumption is more pronounced in households with children.
This could be attributed to the fact that the presence of children might entail more online educational and entertainment activities, such as the use of smartphones, computers, and other electronic devices to meet their learning and recreational needs. These activities require additional energy use. Moreover, households with children might exhibit greater engagement with internet-related activities, such as social media, online education, and entertainment, than offline activities, further amplifying the impact of internet usage on energy consumption. In contrast, households without children might place more emphasis on personal life and leisure activities. Consequently, while the influence of internet usage on energy consumption still exists, its impact might be comparatively smaller in households without children.
Retirement status typically implies that household members have more leisure time and greater autonomy in daily activities and time management. Based on this premise, we posit in the research hypothesis that the retirement status of the household head might introduce heterogeneity in the relationship between internet usage and electricity consumption.
The results of the heterogeneity analysis are presented in columns 7 and 8 of Table 2. Upon conducting heterogeneity analysis, we find that the impact of internet usage differs between two distinct groups: households with retired household heads and those with nonretired household heads. For the former, the regression coefficient between internet usage and household electricity consumption is 0.1008, showing a significant relationship at the 1% significance level. In contrast, for the latter, the regression coefficient for internet usage is 0.0898, which is also significant at the 1% level. This suggests that the impact of internet usage on energy consumption is more pronounced in households with retired heads.
One plausible explanation is that retired household heads possess more discretionary time and are more likely to use the Internet frequently at home, leading to higher electricity consumption in this subgroup.
Another interpretation can be drawn from the perspective of household activity patterns. Retired household heads might engage more frequently in certain activities at home, such as watching movies, engaging in social interactions, and shopping online. These activities often require more electricity than other activities, potentially intensifying the connection between internet usage and household electricity consumption within the group of retired household heads.
Endogeneity is a common challenge in regression analysis and refers to the correlation between explanatory variables and error terms, which can lead to biased estimation results. In this study, internet usage may be influenced by unobserved factors that also affect household electricity consumption, rendering the regression results unreliable. To address the endogeneity issue, we introduce instrumental variables. Instrumental variables are variables that are correlated with the explanatory variable but not with the error term. They can be used to replace the original explanatory variable and thus eliminate endogeneity. By incorporating instrumental variables, we can more accurately estimate the impact of internet usage on household electricity consumption and ensure the credibility of our research findings.
We selected three instrumental variables: the proportion of the population in the same province using the Internet, the terrain ruggedness of the province where the sample is located, and the per capita length of optical fiber cables in the province. These three instrumental variables are related to the core explanatory variable of internet usage but not to the dependent variable of household electricity consumption, thus meeting the requirements of instrumental variable methodology. For instance, the proportion of the population using the Internet in the same province may reflect the extent of Internet penetration in that province, which is related to individual Internet usage. The terrain ruggedness in regions with greater variations may pose challenges in establishing network infrastructure, impacting internet penetration. However, these variables do not directly influence household electricity consumption, fulfilling the criteria for instrumental variables.
To address endogeneity, we adopted the two-stage least squares (2SLS) method, a commonly used instrumental variable regression approach. This method mitigates endogeneity through two stages of regression, ensuring the reliability of the research results. In the first stage, we estimated the impact of the three instrumental variables on internet usage. Using the first-stage regression results, we obtained the predicted values for whether household heads use the Internet and then incorporated them as additional explanatory variables in the second-stage regression model. In the second stage, we used the logarithm of household electricity expenses as the dependent variable and include whether internet usage and other control variables as explanatory variables. Through the second-stage regression, we obtained the impact of internet usage on household electricity consumption. The regression results of the instrumental variable method are presented in Table 3.
In the first stage of the instrumental variable (IV) regression, we used “Internet Usage” as the dependent variable. The results showed that the regression coefficient of the proportion of samples with internet usage in the same province to the total sample size was significant at the 1% level. In contrast, the regression coefficient of the topography variability of the province where the samples were located was not significant at the 10% level. Additionally, the regression coefficient of the per capita fiber optic cable length in the province was significant at the 1% level but negative. These results indicate that the proportion of samples with internet usage in the same province and the per capita fiber optic cable length in the province have significant effects on internet usage, while the topography variability of the province does not have a significant effect.
In the second stage of the instrumental variable (IV) regression, we used the logarithm of “Family Electricity Expenses” as the dependent variable and “Internet Usage” as the independent variable and controlled for other control variables. The regression results showed that the coefficient of “Internet Usage” was significant at the 1% level and positive. This indicates that households where the head uses the Internet are likely to have higher family electricity expenses than those whose heads do not. By conducting a two-stage regression using the instrumental variable (IV) approach, we obtained a more accurate estimation of the impact of internet usage on family electricity expenses and mitigated the influence of endogeneity issues. These findings further support the conclusion of a positive association between internet usage and family electricity expenses.
In addition to the previously discussed analysis using internet usage as a determining factor, we conducted an additional robustness check. In this check, we replaced internet usage with monthly per capita telecommunications costs (hereafter referred to as “telecommunications costs”) in the regression model. This step aimed to confirm the robustness of the relationship between internet usage and household electricity consumption.
We employed a similar regression model to the one used previously, substituting telecommunications costs for internet usage, and conducted an analysis of the mediating effects. The results demonstrated that even when using telecommunications costs as a substitute variable, we observed a similar impact pattern. The relationship between internet usage (or telecommunications costs as a substitute) and household electricity consumption remained statistically significant.
This result reinforces our previous observations regarding the connection between internet usage and electricity consumption, indicating that this linkage remains robust under different measures. The results are shown in Table 4.
This study explores the relationship between internet usage and household electricity consumption, further analyzing the mediating role of sleep duration in this context. Based on the regression results of the fundamental ordinary least squares (OLS) model, we find a positive association between internet usage and household electricity expenses, suggesting that households with internet users tend to have higher electricity expenditures than those without internet users.
Then, our heterogeneous analysis revealed distinct variations in the influence of internet usage on household electricity consumption among different family groups categorized by household registration status, marital status, family structure, and retirement status. These findings further refine the results of our basic analysis.
Subsequently, we introduced sleep duration as a mediating variable and observe its correlation with internet usage, indirectly resulting in increased household electricity costs. To address endogeneity concerns, we employed the instrumental variable method for correction and validate the impact of internet usage on household electricity expenses. Synthesizing the regression outcomes of various models, we drew the following conclusions: While the widespread adoption of the Internet has achieved significant progress in Chinese households, it is also accompanied by increased household electricity costs.
Building upon these findings, our study suggests several implications for policies aimed at fostering sustainable energy practices in households. First, there is a need to enhance energy education and awareness through targeted campaigns that empower individuals to make informed choices about energy consumption. Second, promoting energy-efficient habits should be prioritized, with the initiation of promotional activities to guide families in cultivating electricity-saving behaviors. Third, the establishment of efficiency standards for smart devices can incentivize the production and use of energy-efficient internet-enabled devices. Moreover, advocating for the widespread adoption of energy-saving smart home equipment holds the potential to significantly reduce household energy consumption. To manage the potential impact of excessive internet usage on sleep quality, encouraging the reasonable scheduling of online activities, particularly during evenings, is crucial. Government energy subsidies could be introduced to incentivize households to opt for energy-efficient appliances and home equipment. Finally, by increasing support for research and development in energy technology, policymakers can facilitate the application of innovative and efficient energy-saving solutions across households, ultimately contributing to a more sustainable energy future.
In conclusion, well-considered policies have the potential to effectively decrease household electricity consumption, enhance household energy efficiency, and advance sustainable development. These measures are crucial for addressing energy resource scarcity and environmental preservation. Nonetheless, it should be noted that this study solely examines certain factors influencing household electricity consumption. Future research can examine other variables to comprehensively understand the mechanisms underlying household energy consumption.
Publicly available datasets were analyzed in this study. This data can be found here: http://www.isss.pku.edu.cn/cfps/download.
BG: Conceptualization, Data curation, Formal Analysis, Funding acquisition, Project administration, Resources, Writing–original draft. XH: Conceptualization, Data curation, Formal Analysis, Investigation, Methodology, Software, Validation, Visualization, Writing–original draft, Writing–review and editing. FY: Data curation, Methodology, Software, Supervision, Validation, Visualization, Writing–review and editing. YL: Data curation, Investigation, Software, Supervision, Validation, Visualization, Writing–review and editing.
The author(s) declare financial support was received for the research, authorship, and/or publication of this article. XH acknowledges the support from the National Social Science Foundation of China (Grant # 20CJL030) and the National Natural Science Foundation of China (Grant # 72203039).
The authors declare that the research was conducted in the absence of any commercial or financial relationships that could be construed as a potential conflict of interest.
All claims expressed in this article are solely those of the authors and do not necessarily represent those of their affiliated organizations, or those of the publisher, the editors and the reviewers. Any product that may be evaluated in this article, or claim that may be made by its manufacturer, is not guaranteed or endorsed by the publisher.
Abbasi, K. R., Shahbaz, M., Zhang, J., Irfan, M., and Alvarado, R. (2022). Analyze the environmental sustainability factors of China: the role of fossil fuel energy and renewable energy. Renew. Energy 187, 390–402. doi:10.1016/j.renene.2022.01.066
Abrahamse, W., and Steg, L. (2013). Social influence approaches to encourage resource conservation: a meta-analysis. Glob. Environ. change 23 (6), 1773–1785. doi:10.1016/j.gloenvcha.2013.07.029
Ahmad, T., and Zhang, D. (2020). A critical review of comparative global historical energy consumption and future demand: the story told so far. Energy Rep. 6, 1973–1991. doi:10.1016/j.egyr.2020.07.020
Alimoradi, Z., Lin, C. Y., Broström, A., Bülow, P. H., Bajalan, Z., Griffiths, M. D., et al. (2019). Internet addiction and sleep problems: a systematic review and meta-analysis. Sleep. Med. Rev. 47, 51–61. doi:10.1016/j.smrv.2019.06.004
Damette, O., Delacote, P., and Del Lo, G. (2018). Households energy consumption and transition toward cleaner energy sources. Energy Policy 113, 751–764. doi:10.1016/j.enpol.2017.10.060
Das, S., De Groote, H., and Behera, B. (2014). Determinants of household energy use in Bhutan. Energy 69, 661–672. doi:10.1016/j.energy.2014.03.062
Duan, K., Ren, X., Wen, F., and Chen, J. (2023). Evolution of the information transmission between Chinese and international oil markets: a quantile-based framework. J. Commod. Mark. 29, 100304. doi:10.1016/j.jcomm.2022.100304
Gielen, D., Boshell, F., Saygin, D., Bazilian, M. D., Wagner, N., and Gorini, R. (2019). The role of renewable energy in the global energy transformation. Energy strategy Rev. 24, 38–50. doi:10.1016/j.esr.2019.01.006
Hao, Y. (2022). Effect of economic indicators, renewable energy consumption and human development on climate change: an empirical analysis based on panel data of selected countries. Front. Energy Res. 10, 841497. doi:10.3389/fenrg.2022.841497
Hertwich, E. G., and Peters, G. P. (2009). Carbon footprint of nations: a global, trade-linked analysis. Environ. Sci. Technol. 43 (16), 6414–6420. doi:10.1021/es803496a
Kang, S., Selosse, S., and Maïzi, N. (2015). Strategy of bioenergy development in the largest energy consumers of Asia (China, India, Japan and South Korea). Energy Strategy Rev. 8, 56–65. doi:10.1016/j.esr.2015.09.003
Keho, Y. (2016). What drives energy consumption in developing countries? The experience of selected African countries. Energy Policy 91, 233–246. doi:10.1016/j.enpol.2016.01.010
Kotsila, D., and Polychronidou, P. (2021a). Determinants of household electricity consumption in Greece: a statistical analysis. J. Innovation Entrepreneursh. 10 (1), 19–20. doi:10.1186/s13731-021-00161-9
Kotsila, D., and Polychronidou, P. (2021b). Determinants of household electricity consumption in Greece: a statistical analysis. J. Innovation Entrepreneursh. 10 (1), 19–20. doi:10.1186/s13731-021-00161-9
Li, K., and Lin, B. (2018). How to promote energy efficiency through technological progress in China? Energy 143, 812–821. doi:10.1016/j.energy.2017.11.047
Li, L., and Taeihagh, A. (2020). An in-depth analysis of the evolution of the policy mix for the sustainable energy transition in China from 1981 to 2020. Appl. Energy 263, 114611. doi:10.1016/j.apenergy.2020.114611
Li, Y., Yan, C., and Ren, X. (2023). Do uncertainties affect clean energy markets? Comparisons from a multi-frequency and multi-quantile framework. Energy Econ. 121, 106679. doi:10.1016/j.eneco.2023.106679
Liu, H., Wang, C., Tian, M., and Wen, F. (2019). Analysis of regional difference decomposition of changes in energy consumption in China during 1995–2015. Energy 171, 1139–1149. doi:10.1016/j.energy.2019.01.067
Liu, J., Niu, D., and Song, X. (2013a). The energy supply and demand pattern of China: a review of evolution and sustainable development. Renew. Sustain. Energy Rev. 25, 220–228. doi:10.1016/j.rser.2013.01.061
Liu, J., Niu, D., and Song, X. (2013b). The energy supply and demand pattern of China: a review of evolution and sustainable development. Renew. Sustain. Energy Rev. 25, 220–228. doi:10.1016/j.rser.2013.01.061
Lőrincz, M. J., Ramírez-Mendiola, J. L., and Torriti, J. (2021). Impact of time-use behaviour on residential energy consumption in the United Kingdom. Energies 14 (19), 6286. doi:10.3390/en14196286
Lund, L., Sølvhøj, I. N., Danielsen, D., and Andersen, S. (2021). Electronic media use and sleep in children and adolescents in western countries: a systematic review. BMC Public Health 21 (1), 1–14. doi:10.1186/s12889-021-11640-9
Matsumoto, S., Mizobuchi, K., and Managi, S. (2022). Household energy consumption. Environ. Econ. Policy Stud. 24, 1–5. doi:10.1007/s10018-021-00331-9
Mei, X., Zhou, Q., Li, X., Jing, P., Wang, X., and Hu, Z. (2018). Sleep problems in excessive technology use among adolescent: a systemic review and meta-analysis. Sleep Sci. Pract. 2 (1), 9–10. doi:10.1186/s41606-018-0028-9
Morley, J., Widdicks, K., and Hazas, M. (2018). Digitalisation, energy and data demand: the impact of Internet traffic on overall and peak electricity consumption. Energy Res. Soc. Sci. 38, 128–137. doi:10.1016/j.erss.2018.01.018
Pablo-Romero, M. D. P., Pozo-Barajas, R., and Yñiguez, R. (2017). Global changes in residential energy consumption. Energy Policy 101, 342–352. doi:10.1016/j.enpol.2016.10.032
Pachauri, S., and Spreng, D. (2004). Energy use and energy access in relation to poverty. Econ. Political Wkly., 271–278.
Palm, J., and Thollander, P. (2010). An interdisciplinary perspective on industrial energy efficiency. Appl. Energy 87 (10), 3255–3261. doi:10.1016/j.apenergy.2010.04.019
Paul, A. C., Myers, E. C., and Palmer, K. L. (2009). A partial adjustment model of US electricity demand by region, season, and sector.
Pothitou, M., Hanna, R. F., and Chalvatzis, K. J. (2017). ICT entertainment appliances’ impact on domestic electricity consumption. Renew. Sustain. Energy Rev. 69, 843–853. doi:10.1016/j.rser.2016.11.100
Raimi, D., Campbell, E., Newell, R. G., Prest, B., Villanueva, S., and Wingenroth, J. (2022). Global energy outlook 2022: turning points and tension in the energy transition. Washington, DC, USA: Resources for the Future.
Ren, X., Cao, Y., Liu, P. J., and Han, D. (2023a). Does geopolitical risk affect firms' idiosyncratic volatility? Evidence from China. Int. Rev. Financial Analysis 90, 102843. doi:10.1016/j.irfa.2023.102843
Ren, X., Li, Y., Sun, X., Bu, R., and Jawadi, F. (2023c). Modeling extreme risk spillovers between crude oil and Chinese energy futures markets. Energy Econ. 126, 107007. doi:10.1016/j.eneco.2023.107007
Ren, X., Zeng, G., and Sun, X. (2023b). The peer effect of digital transformation and corporate environmental performance: empirical evidence from listed companies in China. Econ. Model. 128, 106515. doi:10.1016/j.econmod.2023.106515
Ritchie, H., Roser, M., and Rosado, P. (2022). Energy production and consumption: our world in data.
Salahuddin, M., and Alam, K. (2015). Internet usage, electricity consumption and economic growth in Australia: a time series evidence. Telematics Inf. 32 (4), 862–878. doi:10.1016/j.tele.2015.04.011
Sinha, A., Shahbaz, M., and Sengupta, T. (2018). Renewable energy policies and contradictions in causality: a case of Next 11 countries. J. Clean. Prod. 197, 73–84. doi:10.1016/j.jclepro.2018.06.219
Sukarno, I., Matsumoto, H., and Susanti, L. (2017). Household lifestyle effect on residential electrical energy consumption in Indonesia: on-site measurement methods. Urban Clim. 20, 20–32. doi:10.1016/j.uclim.2017.02.008
Valdes, J. (2021). Participation, equity and access in global energy security provision: towards a comprehensive perspective. Energy Res. Soc. Sci. 78, 102090. doi:10.1016/j.erss.2021.102090
Wang, Q., and Li, R. (2016). Drivers for energy consumption: a comparative analysis of China and India. Renew. Sustain. Energy Rev. 62, 954–962. doi:10.1016/j.rser.2016.04.048
Wu, K. (2014). China׳ s energy security: oil and gas. Energy Policy 73, 4–11. doi:10.1016/j.enpol.2014.05.040
Wu, Y., Zhu, Q., and Zhu, B. (2018). Comparisons of decoupling trends of global economic growth and energy consumption between developed and developing countries. Energy Policy 116, 30–38. doi:10.1016/j.enpol.2018.01.047
Xu, B., Zhong, R., Hochman, G., and Dong, K. (2019). The environmental consequences of fossil fuels in China: National and regional perspectives. Sustain. Dev. 27 (5), 826–837. doi:10.1002/sd.1943
Yu, D. J., An, F., Ye, Y. F., and Zeng, H. H. (2023). Study on regional differences and influencing factors of energy consumption in China. Sci. Prog. 106 (1), 00368504221148003. doi:10.1177/00368504221148003
Zheng, X., Wei, C., Qin, P., Guo, J., Yu, Y., Song, F., et al. (2014). Characteristics of residential energy consumption in China: findings from a household survey. Energy Policy 75, 126–135. doi:10.1016/j.enpol.2014.07.016
Zhou, N., Levine, M. D., and Price, L. (2010). Overview of current energy-efficiency policies in China. Energy policy 38 (11), 6439–6452. doi:10.1016/j.enpol.2009.08.015
Keywords: internet development, electricity consumption, mediating effect model, family behavior, China
Citation: Gao B, Han X, Ye F and Li Y (2023) Internet usage and household electricity consumption. Front. Energy Res. 11:1323077. doi: 10.3389/fenrg.2023.1323077
Received: 17 October 2023; Accepted: 08 November 2023;
Published: 29 November 2023.
Edited by:
Xiaohang Ren, Central South University, ChinaReviewed by:
Shan Pan, Jinan University, ChinaCopyright © 2023 Gao, Han, Ye and Li. This is an open-access article distributed under the terms of the Creative Commons Attribution License (CC BY). The use, distribution or reproduction in other forums is permitted, provided the original author(s) and the copyright owner(s) are credited and that the original publication in this journal is cited, in accordance with accepted academic practice. No use, distribution or reproduction is permitted which does not comply with these terms.
*Correspondence: Xuze Han, MTcwMDAxNTg4MUBwa3UuZWR1LmNu; Fangbing Ye, eWVmYW5nYmluZ0Bwa3UuZWR1LmNu
Disclaimer: All claims expressed in this article are solely those of the authors and do not necessarily represent those of their affiliated organizations, or those of the publisher, the editors and the reviewers. Any product that may be evaluated in this article or claim that may be made by its manufacturer is not guaranteed or endorsed by the publisher.
Research integrity at Frontiers
Learn more about the work of our research integrity team to safeguard the quality of each article we publish.