- School of Business Administration, Liaoning Technical University, Huludao, China
Based on the data of listed companies in China’s iron and steel industry from 2007 to 2020, the article investigates the impact mechanism and the path of action of China’s carbon emissions trading pilot on the green total factor productivity of iron and steel enterprises by constructing a multi-period difference-in-difference model difference-in-differences. The study finds that: 1) China’s iron and steel enterprises significantly improve their green total factor productivity driven by the carbon trading pilot, and the findings pass the corresponding robustness tests. 2) the mechanism analysis indicates that the carbon trading pilot promotes the green total factor productivity of iron and steel enterprises by forcing the technological progress of enterprises. 3) The heterogeneity analysis shows that the positive effect is more significant for large iron and steel enterprises with high social responsibility rating and high local government competition intensity, but not for small enterprises with low social responsibility rating and low local government competition intensity. 4) the dynamic effect shows that there is a certain lag in the promotion effect of the carbon emission trading pilot on the green total factor productivity of iron and steel enterprises, but its long-term effect is more obvious. This paper puts forward corresponding suggestions for accelerating the construction of a national unified green and low-carbon market system and actively promoting the deepening of the “dual-carbon” goal.
1 Introduction
As global warming and traditional energy consumption continue to intensify (De and Sue, 2019; Shankar et al., 2016), China is actively exploring new economic development models, gradually realizing the goal of “double carbon” (Hua et al., 2022; Tan et al., 2023), contributing to high-quality economic development (Li and Liu, 2023; Wei and Zhang, 2023), making green the base color of high-quality economic development, and improving the green total factor productivity of enterprises (Zhang et al., 2022; Liu et al., 2023a). In this context, China has introduced “dual carbon” policies and institutional arrangements one after another (Cao et al., 2020; Qi and Han, 2023; Zhu and Ma, 2023). Among them, in order to build a nationwide unified green low-carbon market system, China has launched a market-oriented policy tool of carbon emission trading pilot (Liu et al., 2022a; Cui et al., 2022). Shenzhen, Shanghai, Beijing, Guangdong and Tianjin launched carbon trading pilot projects in 2013. In 2014, Chongqing and Hubei also launched the trading and performance of the carbon emission trading market. In December 2016, Fujian Province launched its carbon emissions trading market, becoming the eighth carbon emissions trading pilot in China (Liu et al., 2022b; Huang, 2023; Rao et al., 2023). With the gradual maturity of China’s carbon emission institutional mechanism and trading system, the national carbon emission trading market for the power generation industry was officially launched on 16 July 2021 (Gai et al., 2018; Ma and Duan, 2023), and on 22 February 2021, the Department of Climate Change of the Ministry of Ecology and Environment clearly pointed out that the iron and steel industry is the third most important industry to be included in the national carbon market after the power and building materials industries (Tian et al., 2022; Li et al., 2023). As the world’s largest iron and steel producer, many domestic iron and steel companies have made active attempts in carbon trading. So, can the “double carbon” policy improve the green total factor productivity of iron and steel enterprises? What is the mechanism of the “dual carbon” policy to improve the total factor productivity of enterprises?
The relationship between energy, the environment, and economic growth has received widespread attention in scientific research in recent years (Naeem et al., 2022; Duan et al., 2023a; Naeem et al., 2023; Ren et al., 2023), yet environmental sustainability remains a global issue. The development of renewable energy (Zhang and Wang, 2019; Adebayo et al., 2022), green technology innovation (Du et al., 2019; Habiba et al., 2022), and regulatory policy mechanisms (Abbas et al., 2021; Annamalaisamy and Vepur Jayaraman, 2023) contribute to reducing greenhouse gas emissions and supporting environmental sustainable development. Xu et al. (2023) found that green technology innovation and environmental regulation are the most effective ways to achieve zero emissions. Ali et al. (2021) argued that in the long run, if decision-makers do not take adequate and timely measures, emergencies could negatively impact the environment. Rauf et al. (2023) suggest that governments should adopt environmentally sustainable economic policies to reduce carbon emissions while attracting environmental protection technologies and encouraging investment in renewable energy projects to enhance regional environmental quality. Ali et al. (2022) found that green innovation aids BRICS countries in achieving their goal of environmental sustainability. Abbas et al. (2022) believe that BRICS should design market-based environmental regulatory policies, emphasizing the implementation of environmental taxes, and expanding renewable energy development and investments in environmental innovation are key to limiting carbon dioxide emissions and achieving environmental sustainable development. Wen et al. (2021) found that green innovation significantly improved South Asia’s environmental quality. Sheng Yin and Hussain, 2021 empirically found that political stability, rule of law, regulatory quality, and corruption control are statistically significant factors affecting renewable energy investment in BRI countries. Hu et al. (2021) suggest policymakers should encourage ASEAN countries to invest in renewable energy to promote green trade openness.
Carbon emission trading is a policy introduced by nations for a green transformation of industrial structures (Zang et al., 2020), hoping to internalize the externalities of carbon emissions (Du et al., 2021), thereby achieving low-carbon targets (Liu et al., 2023b). Many scholars have discussed carbon emission trading extensively and in-depth. Research on the impact of carbon emission trading on green development has mostly focused on its relationship with green technology innovation (Liu et al., 2022a; Cui et al., 2022). The EU’s carbon emission trading system (EU ETS) has been the subject of foreign research, where the low-carbon technology patenting has increased by 10% since 2005 without growing out effect, considered to be the largest cap-and-trade program (Calel and Dechezleprêtre, 2016). EU ETS entered a new phase in 2013 with auction-based quota allocation, having stronger renewable technology innovation capabilities (Qi and Zhang, 2019). Researchers at home and abroad began to pay attention to the carbon price dynamics, especially the carbon price prediction. Duan et al. (2023b) found that the carbon market has a unidirectional risk spillover to both traditional and green asset markets, and the outbreak of COVID-19 pandemic has strengthened cross-market risk spillover effects. Wang et al. (2020) through the method of synthetic control, explored that carbon trading can promote low-carbon technological innovation, and the synergy between carbon trading market and regional industrial structure upgrading is also an important driving force for low-carbon technological innovation. Huang et al. (2023) found that after COVID-19 pandemic, Bitcoin’s investment shelter role for distinctive assets was enhanced and expanded, while green assets have consistently acted as an effective hedge for Bitcoin. From a micro perspective, quota allocation and price signaling perspectives differ. From the perspective of quota allocation, the quota allocation method can be divided into free distribution and paid distribution. In the free distribution method, Song et al. (2021) discussed the influence of benchmark method and historical method on corporate green technology innovation, finding the benchmark method is more prominent in driving green technology innovation, the historical method has “whipping fast cow” effect. Zhu et al. (2019) discovered different quota allocation structures impact corporate green technology innovation, with innovation capacity clearly stronger in companies with large-scale allocations compared to ratio-based ones. From the perspective of price signals, Wang (2016) divided carbon emission right trading into pure market trading, non-market trading and mixed trading by constructing measurement models. At the same time, it studied the mechanism of clean technology progress bias from different perspectives. The results show that the price increase of limited resources can promote the occurrence of clean technology bias effect. Jiakui et al. (2023) found that establishing green finance legislation accelerates green finance development, thus enhancing corporate green total factor productivity. Meng and Han (2017) used different theories and research methods, analyzed the effect of low-carbon innovation subsidies, carbon taxes, and carbon trading on low-carbon innovation, finding that optimal results require reasonable application of all three policy tools, and corporate low-carbon technology innovation evolves with carbon trading price fluctuations. Tong et al. (2022) empirical study found that increasing the intensity of environmental regulation will significantly improve China’s green productivity.
Empirical research on carbon emission trading’s impact on green total factor productivity mainly uses the difference-in-differences model (Tang et al., 2021; Hua et al., 2022; Cheng and Meng, 2023). However, different scholars have used different research dimensions, with most using provincial-level data. Zhang and Qiao (2021) took the carbon emission right trading as the experimental object, on the basis of measuring the green total factor productivity of the Chinese manufacturing industry in 30 provinces from 2009 to 2017, studied the effect of carbon emission trading on the manufacturing industry’s green total factor productivity using the propensity score matching difference-in-differences method (PSM-DID), concluding that the green total factor productivity of manufacturing industry is gradually improved by the influence of environmental regulation. Chen and Chen (2022) based on 2000–2018 panel data from 30 provinces and cities, using DID and PSM-DID models, discussed the changes in green total factor productivity in china’s provinces, although not specific to cities, they are divided into different industries, concluding that carbon emission trading policies positively affected China’s industrial green total factor productivity, thus promoting the improvement of China’s industrial green total factor productivity. Qi et al. (2021) used the DID model for empirical analysis on 30 provinces and cities, showing that the policy significantly reduced carbon emissions in pilot areas. A few studies have focused on enterprises or industry-specific research. Zhang and Zhang (2021) also based on carbon emissions trading, but they chose China’s manufacturing listed companies from 2009 to 2020 as samples, using the difference-in-differences method for empirical analysis, which showed that carbon emission trading pilots improved the total factor productivity of manufacturing listed companies. Hu and Ding (2020) comprehensively analyzed China’s listed companies from 2006 to 2017 using the difference-in-differences model, including robustness tests with parallel trends, lagging two periods, instrumental variables, and placebo, and verified different company nature effects using the triple difference method. Finally, the mediation and moderation effect method is used to test the mediation effect of carbon trading and total factor productivity, and to investigate the mediation effect of regulation under the influence of green innovation, market and government subsidy.
In this paper, we use a multi-period DID approach to empirically test the above issues by considering the carbon emissions trading pilot as a quasi-natural experiment of the “dual carbon” policy. The research on the above issues is of great theoretical and practical significance to scientifically evaluate the implementation effect of the carbon trading pilot, and clarify the mechanism of carbon trading pilot affecting the green total factor productivity of enterprises.
2 Theoretical analysis and research hypothesis
In the pilot market of carbon emission trading, carbon emission rights are given commodity attributes, i.e., the rational allocation of resources among enterprises is achieved through free circulation. As a market-incentivized environmental regulation tool in China, the carbon emission trading pilot uses the market price mechanism to internalize the negative externality costs of environmental pollution and achieve equal marginal abatement costs among economic agents in order to reduce carbon emissions of enterprises in the pilot region (Germeshausen, 2020; Hu et al., 2020). Unlike traditional command-based environmental regulatory instruments, firms participating in the pilot carbon trading market have the flexibility to decide whether to continue production until the marginal cost of emission reduction is in line with the current trading price (Dai, 2019; Niizawa et al., 2020), without fear of shutting down production or paying a penalty higher than the marginal cost of emission reduction. However, with the increasingly stringent allocation of carbon allowances in various pilot carbon trading markets, although enterprises can respond by actively reducing production or purchasing corresponding carbon credits in the carbon trading market, in the long run, these measures largely run counter to their goal of profit maximization (Hu et al., 2020; Tanaka et al., 2020). At the same time, as an important market incentive and environmental regulation tool to achieve the “double carbon” target in China, the carbon emission trading pilot will play its role in the long run. Under this circumstance, iron and steel enterprises, which are the major consumers of energy and resources, will take the optimization of their own resources as the starting point to readjust and optimize the allocation of relevant factors in their production and operation activities, so as to withdraw more factors and resources from energy-consuming projects and shift to cleaner production, in order to reduce their total carbon emissions and promote their green total factor productivity by improving their own resource allocation efficiency. Thus, this paper proposes hypothesis 1:
H1. Pilot carbon trading can improve green total factor productivity of iron and steel enterprises.
Further, from the design rules of the pilot carbon emission trading market, for those enterprises with carbon emission reduction cost advantage in the pilot carbon emission trading region, they can gain additional revenue by selling their excess carbon emission allowances. At the same time, dominated by the cost advantage of carbon emission reduction, enterprises with the cost disadvantage of carbon emission reduction will also actively engage in low-carbon technology innovation and make efforts to reduce carbon emissions. Therefore, based on the optimal incentive mechanism inherent in the trading market, the implementation of the pilot carbon emission trading can not only promote carbon emission reduction by enterprises with the cost advantage of carbon emission reduction but also stimulate enterprises facing higher costs to continuously improve their low-carbon level. This, in turn, encourages firms to increase their R&D investments and promote improvements in their production technologies and product quality (Qi et al., 2018; Saether et al., 2021).
According to Figure 1, under the stricter environmental regulation constraints caused by carbon trading, once the production and operation of iron and steel enterprises cause carbon emissions to exceed their quotas, they need to compulsorily purchase the excess carbon dioxide emission rights, which increases their production costs and thus poses a serious challenge to the market competitiveness of high carbon emitting enterprises. In order to alleviate the pressure of increased production costs caused by the purchase of carbon emission rights, high carbon emitting enterprises need to increase their investment in process innovation and improve their motivation to carry out process innovation. The more dominant they are in clean technology research and development, the more high-carbon emitters will save on the cost of purchasing carbon credits in the carbon trading market. Therefore, process innovation has become one of the important ways for high-carbon-emitting enterprises to save production costs and improve their competitiveness in the market.
Low-carbon emission enterprises can reduce carbon dioxide emissions through advanced production technologies and clean production processes, and sell the saved carbon emission rights to high-carbon emission enterprises, making additional profits in the pilot carbon emission rights trading. When the benefits of the transfer of carbon emission rights exceed the cost of technological innovation, low-carbon enterprises will pay more attention to clean technology research and development, as the Porter hypothesis (Porter and Linde, 1995) knows, this measure will encourage enterprises to spend more resources on low-carbon technology research and development and innovation funds. By forcing enterprises to improve low-carbon capacity, and then reduce carbon emissions per unit product, and finally drive the overall green total factor productivity of enterprises. Therefore, no matter the surplus or lack of carbon emissions, iron and steel enterprises will increase their investment in technological innovation to promote their green total factor productivity. Based on this, this paper proposes Hypothesis 2.
H2. The carbon trading pilot will push the enterprises to improve their technology and promote the green total factor productivity of iron and steel enterprises.
3 Research design
3.1 Variable definition
The implementation of the fiscal decentralization system in China has led to the fact that each local government is the actual implementer of environmental regulation policies and has discretion over the actual intensity of its implementation of environmental regulation; therefore, this paper selects the intensity of local government competition as an indicator for heterogeneity analysis. Drawing on the definition of local government competition and the selection of indicators in the literature, this paper assesses the intensity of local government competition in three key dimensions: economic growth, attracting foreign investment, and financial self-sufficiency. 1) Competitiveness of economic growth: the growth rate of regional GDP (
3.2 Model setting
where
Drawing on the intermediary effect test proposed by Wen and Ye (2014), this paper aims to explore the mechanism of the impact of the carbon emissions trading pilot on the green total factor productivity of the iron and steel industry (Wen and Ye, 2014). Therefore, on the basis of Equation 1, the following test model is further constructed:
where
3.3 Data sources and statistical characteristics
This paper selects China’s ferrous metal smelting and rolling processing industry from 2007 to 2020 as the original research sample. The ST, *ST and PT categories and companies with incomplete data are excluded. After processing, the final sample covers 24 listed companies with a total of 336 observations. Emission data of relevant pollutants are obtained from China Industrial Statistical Yearbook and China Environmental Statistical Yearbook. The relevant data at the enterprise level are obtained from the CSMAR database. The relevant data at the enterprise location level are obtained from the provincial and municipal statistical yearbooks.
In this paper, the continuous variables were Winsorized by replacing their values less than the 1% quantile (or greater than the 99% quantile) with the 1% quantile (or 99% quantile) and descriptive statistics were performed for the main variables. As can be seen from Table 2, the green total factor productivity (GTFP) of iron and steel firms generally exhibited a low level in the overall sample with a mean of 1.056, and a standard deviation of 0.426, and a minimum value of 0.272, and a maximum value of 5.929.
4 Empirical tests
4.1 Average trend of green total factor productivity in iron and steel companies
Figure 2 presents the trend of the mean green total factor productivity of iron and steel enterprises, where: the horizontal axis represents the time line; the vertical axis corresponds to the mean green total factor productivity of iron and steel enterprises; the dotted line represents the experimental group, its pilot iron and steel enterprises; the solid line represents the control group, its non-pilot iron and steel enterprises; and 2013 represents the cut-off point of policy implementation. Before the operation of the pilot carbon trading market, the green total factor productivity of the experimental group shows a similar trend to that of the control group, and the mean value of green total factor productivity of the experimental group is higher than that of the control group; after the operation of the pilot carbon trading market, the mean value of green total factor productivity of the experimental group shows a certain volatility, which is more significant compared with that of the control group. Therefore, the premise of the hypothesis of parallel trend is met.
4.2 Impact of carbon trading pilot on green total factor productivity of iron and steel companies
In this paper, we use a multi-period difference-in-difference model to explore the impact of the carbon trading pilot on the green total factor productivity of iron and steel firms. According to the estimation results in column (1) of Table 3, the carbon trading pilot has a positive impact on the green total factor productivity of iron and steel firms when no control variables are included and no time and region fixed effects are controlled, which indicates that the implementation of this policy can improve the green total factor productivity of iron and steel firms. Column (2) considers both time and region-fixed effects on the basis of column (1), and the results show that the regression coefficient of the difference-in-difference term is positive and improves the goodness-of-fit of the model. Therefore, this paper concludes that the pilot carbon emissions trading policy has a positive contribution to the improvement of green total factor productivity of iron and steel enterprises. In addition, this paper further investigates the change in the regression effect of the multiple difference model after the introduction of the control variables. By including the control variables into the model in columns (3) and (4), it can be observed that the stability of the regression coefficients is significantly improved, and the regression coefficients of the difference-in-difference terms are significantly positive at the 10% and 5% levels, while the coefficient values also reach 0.130 and 0.235, respectively. This indicates that the inclusion of control variables can better explain the significant positive relationship between the pilot carbon trading policy and the green total factor productivity of iron and steel enterprises, further verifying the reliability of H1 in this paper.
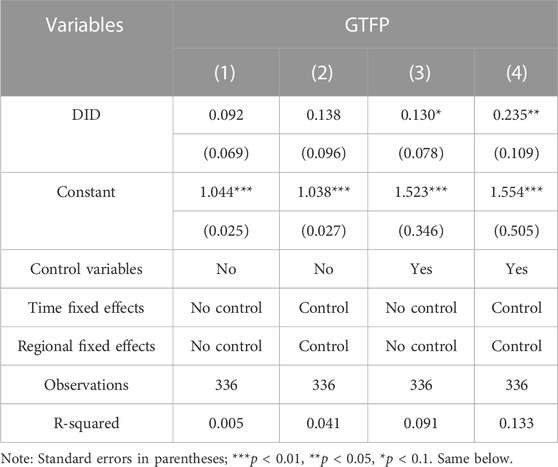
TABLE 3. Test results of the impact of carbon trading pilot on green total factor productivity of iron and steel companies.
4.3 Robustness tests
4.3.1 Parallel trend test
In order to enhance the persuasiveness of the parallel trend test, this paper adopts the method used by Ma et al. (2019) to obtain the changes and correspondence of each indicator under different periods, and use it as a data source for empirical analysis. Figure 3 shows the estimation results at 95% confidence interval, where: the horizontal axis generates dummy variables ± n based on the difference between the first batch of carbon emission trading pilot intervention years and the real years, specifying that all numbers less than or equal to −7 are replaced with −7, and all numbers greater than or equal to 7 are replaced with 7. Therefore, the pre-and post-policy value interval of the horizontal axis is [−7,7]; the vertical axis indicates the estimated value of green total factor productivity. In order to verify the feasibility of the method, 24 listed iron and steel companies were used as the research subjects for empirical analysis. As shown in Figure 3, before the release of the pilot carbon trading policy, there was no significant difference between the experimental group and the control group, and their estimated values did not reach a significant level; with the release of the pilot carbon trading policy, the green total factor productivity of iron and steel enterprises was significantly and positively affected, which indicates that the policy is consistent with the premise of the hypothesis of parallel trend.
4.3.2 Counterfactual test
Conventional counterfactual tests usually use the policy time advance to assess the significance of the policy effect. Considering the gradual operation characteristics of the carbon emission trading pilot, this paper advances its market operation in the pilot regions by 1 year. Specifically, this paper uses 2012 as the policy implementation time point in Shenzhen, Guangdong, Beijing, Tianjin and Shanghai, 2013 as the policy implementation time point in Hubei and Chongqing, and 2015 as the policy implementation time point in Fujian, as detailed in Table 4. By setting different parameter conditions, this paper analyzes the impact of the carbon emissions trading pilot on the green total factor productivity of iron and steel firms using a panel data model approach. It is found that although the test results of model (5) are significantly different from the quasi-regression, they are not significantly different from the estimated results of model (6) controlling for the multiple fixed effects model, which indicates that the carbon trading pilot has a significant effect on the growth of green total factor productivity of iron and steel enterprises, thus the reliability of the benchmark regression results can be verified.
4.3.3 Placebo test
This paper draws on Wang and Ge (2022) A randomized experimental and control group was adopted for the placebo test, and 10 enterprises from 24 iron and steel enterprises were randomly selected as the experimental group for the implementation of the carbon trading pilot, while the remaining 14 enterprises were the control group, and the newly generated experimental and control groups were estimated based on the baseline regression model Eq. 1. In this paper, the above steps are repeated 500 times and the distributions of the 500 estimated coefficients and their associated p-values are further plotted, as shown in Figure 4. From the placebo test results, it is clear that the estimated coefficients of the newly generated samples are concentrated around zero, and most of the estimated values have p-values greater than 0.1 and are significantly different from the true values [from column (4) of Table 1], i.e., there is no other policy or random variable affecting the green total factor productivity enhancement of iron and steel enterprises, which also implies that the enhancement of green total factor productivity of iron and steel enterprises by carbon emissions trading policy is not a contingency.
4.3.4 Dynamic effects test
In view of the possible lags and timeliness of the impact of the carbon emissions trading pilot on the green total factor productivity of iron and steel firms, this paper argues that its impact should exhibit nonlinear characteristics, and therefore dynamic effects tests are needed to ensure its validity. Based on this, this paper constructs a sample group for each 7-year period before and after the promulgation of the carbon emissions trading pilot work policy using panel data regression method to empirically analyze the promotion effect of the carbon emissions trading market on the green total factor productivity of iron and steel enterprises. According to Table 5, the estimated values of the interaction term coefficients in model (7) for the first 3 years after the promulgation of the carbon emissions trading pilot policy are not significant, while, on the whole, the introduction of the policy does not lead to positive economic effects for all sample firms. This suggests that there is a lag in the effect of the carbon trading pilot on the growth of green total factor productivity of iron and steel firms. This may be due to the fact that it takes some time for the policy to be implemented on the ground, and the green transformation of different enterprises differs in their specific circumstances, leading to differences in the transformation paths and time points. However, over time, 3 years after the release of the carbon emissions trading pilot policy, the green total factor productivity of iron and steel enterprises is significantly positive in the estimated values of the interaction term coefficients, which indicates that the carbon emissions trading pilot may stimulate the own dynamic adjustment of iron and steel enterprises, which in turn has a long-term impact on the green transformation development of iron and steel enterprises. Therefore, the implementation of the carbon emission trading pilot will be beneficial to enhance the green competitiveness and sustainable development of iron and steel enterprises. In addition, model (8) controls for both time and regional solid effects based on model (7), and the regression results do not differ significantly from model (7), thus verifying the robustness of the dynamic effects.
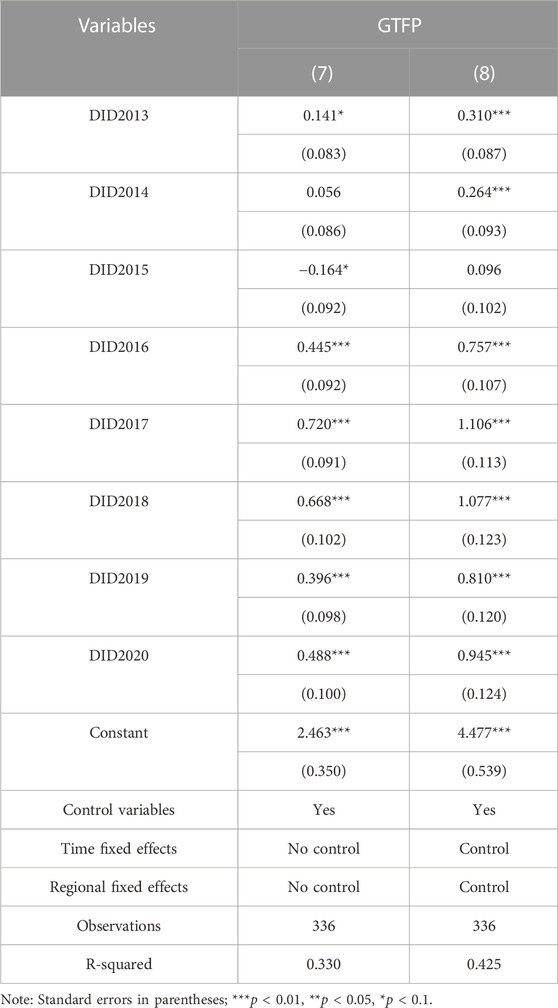
TABLE 5. Dynamic Effect of Carbon Emission Trading Pilot on Green Total Factor Productivity of Iron and steel Enterprises.
5 Further analysis
5.1 Mechanisms of the impact of carbon emissions trading pilot on green total factor productivity of iron and steel enterprises
Based on the previous research hypotheses, this paper investigates the mediating role of technological progress between the carbon trading pilot and green total factor productivity of iron and steel firms, as a way to explore the mechanism of the impact of the carbon trading pilot policy on green total factor productivity of iron and steel firms. According to Table 6, in model (9), the regression coefficients of DID and firms’ technological progress are positive but insignificant, while in model (10), the regression coefficients of Tech and GTFP are significantly positive at the 1% level, requiring a Sobel test, and the results pass the Sobel test, thus verifying H2. Therefore, this paper concludes that the implementation of the carbon emission trading pilot can push back enterprises to invest in low-carbon technologies and significantly improve the level of technological progress of iron and steel enterprises, which in turn leads to green and low-carbon transformation of iron and steel enterprises and ultimately promotes green total factor productivity of iron and steel enterprises.
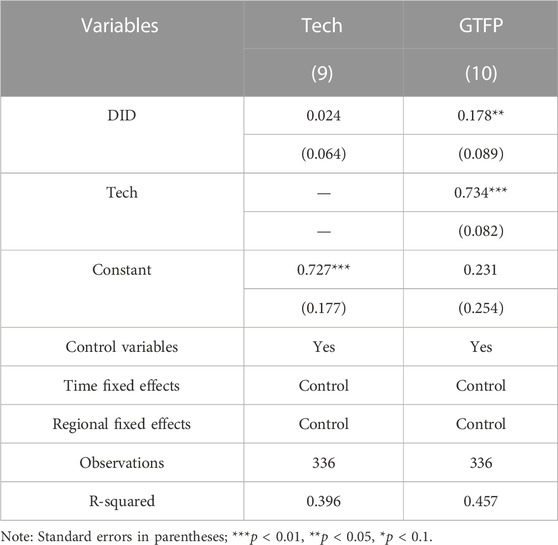
TABLE 6. Mechanisms of impact of carbon trading pilot on green total factor productivity of iron and steel companies.
5.2 Heterogeneity analysis
5.2.1 Enterprise size
To investigate the differences in the effects of the carbon trading pilot on the green total factor productivity of iron and steel firms of different sizes, this paper uses the median total asset value of firms as the grouping criterion for the sample firms. The regression results are shown in Table 7. According to model (11) in Table 7, the DID coefficients of large iron and steel enterprises are positive at the 5% level, which indicates that the implementation of the carbon trading pilot can greatly improve the green total factor productivity of large iron and steel enterprises; in model (12), the DID coefficients of small iron and steel enterprises are positive but insignificant, which indicates that the carbon trading pilot has a positive effect on their green total factor productivity. In model (12), the DID coefficients of small iron and steel companies are positive but not significant, indicating that the pilot carbon trading pilot does not have a significant effect on their green total factor productivity. Further analysis reveals that large enterprises are more affected by environmental costs in the production process, and have more advantages than small enterprises in terms of technology level and capital scale. Therefore, in the process of implementing the carbon emissions trading pilot, large enterprises can invest technology and capital more effectively in order to promote their development toward green transformation.
5.2.2 Corporate social responsibility
In this paper, the sample firms are classified according to the median of their social responsibility scores by referring to the social responsibility report of Hexun.com listed companies, and the high socially responsible firms have higher scores than the median, while the low socially responsible firms have lower scores than the median. According to model (13) in Table 7, the DID coefficients of highly socially responsible firms are significantly positive at the 10% level, which indicates that the carbon trading pilot has a significant contribution to promoting the improvement of green total factor productivity of high socially responsible iron and steel firms; while in model (14), the DID coefficients of low socially responsible firms are negative and insignificant, which indicates that the carbon trading In model (14), the DID coefficients of low socially responsible enterprises are negative and insignificant, which indicates that the carbon trading pilot does not significantly improve the green TFP of low socially responsible iron and steel enterprises, and even has a suppressive effect. The reason for this may be that the higher the CSR, the easier it is for firms to establish a good corporate image in the market. Highly socially responsible firms also perform better in terms of financial performance compared to low socially responsible firms. Therefore, with the impetus of the carbon trading pilot, highly socially responsible firms are able to inject technology and capital more effectively to facilitate their transition to green and thus enhance their green total factor productivity.
5.2.3 Local government competition
The decentralization and concessions accompanying the reform of China’s fiscal decentralization system have led to the fact that each local government is the actual implementer of environmental regulation policies, and local governments have discretion in implementing environmental regulations based on the actual situation in terms of their intensity, based on which environmental regulations have become a powerful tool for local governments to compete (Chen, 2009; Yang et al., 2020). Therefore, strategic interactions between different regions in the implementation of environmental policies may lead to implementation biases. When a region sets its environmental regulation intensity, its competing regional governments will adopt strategies to increase or decrease the intensity of environmental regulation depending on their level of environmental regulation, based on the principle of maximizing their own interests, thus creating a competitive strategy interaction of local governments’ environmental regulation (Li, et al., 2021; Yin and Chen, 2022).
To investigate the heterogeneity of the effect of carbon trading pilot on the green total factor productivity of iron and steel firms in different regions, this paper uses the median of local government competition intensity to group the regions where the sample firms are located, and the regions with higher competition intensity are larger than the median, while the regions with lower competition intensity are smaller than the median. According to model (15) in Table 7, the value of DID coefficient is significantly positive in the regions with higher competitive intensity, reaching the level of 10%, which indicates that the carbon trading pilot can significantly improve the green total factor productivity of iron and steel enterprises in the regions with higher competitive intensity; while in model (16), the value of DID coefficient is positive but not significant in the regions with lower competitive intensity, which indicates that the carbon trading pilot has a significant impact on the green total factor productivity of iron and steel enterprises in the regions with lower competitive intensity. In model (16), the DID coefficient is positive but not significant, indicating that the pilot carbon trading scheme does not significantly improve the green total factor productivity of iron and steel enterprises in less competitive regions. According to the relevant data, regions with higher competitive intensity are more flexible than regions with lower competitive intensity in terms of both fiscal expenditure structure and FDI scale. Therefore, regions with higher competitive intensity of local governments are more helpful for iron and steel enterprises to invest in technology and capital to promote their development toward green transformation and thus improve the green total factor productivity of iron and steel enterprises, driven by the carbon emission trading pilot.
6 Conclusion
For iron and steel enterprises, investigating the impact of the pilot carbon emission trading market on their green total factor productivity is a crucial part to accurately grasp the effect of the implementation of the pilot carbon emission market in China and to achieve the low-carbon transformation of the manufacturing industry and the goal of “double carbon.” Based on this, this paper uses a panel data regression method to construct a sample group for each of the 7 years before and after the promulgation of the pilot carbon emissions trading policy, and empirically analyzes the contribution of the carbon emissions trading market to the green total factor productivity of iron and steel enterprises. This paper selects 24 iron and steel enterprises listed in the period 2007–2020 as the research sample and conducts an in-depth study on the impact of the carbon emissions trading pilot on the green total factor productivity of iron and steel-producing enterprises and its intrinsic mechanism of action by using a multi-period difference-in-difference model, while discussing the heterogeneous impact of the pilot policy on the green total factor productivity of iron and steel enterprises, and draws the following research conclusions:
First, the benchmark regression shows that the green total factor productivity of iron and steel firms is significantly improved under the influence of the carbon emissions trading pilot, and this finding can be tested by parallel trends; the dynamic effects test shows that there is a certain lag in the promotion effect of the carbon emissions trading pilot policy, i.e., the policy effect starts to appear after 3 years, and the subsequent policy effect will persist for a long time; the counterfactual test and placebo test on the accuracy of the baseline regression results are fully verified. Second, the mechanism analysis shows that the carbon trading pilot will force the technological progress of enterprises, thus promoting the improvement of green total factor productivity of iron and steel enterprises. Finally, the heterogeneity analysis shows that the carbon emissions trading pilot has a heterogeneous impact on the improvement of green total factor productivity of iron and steel enterprises, mainly in three aspects: enterprise size, corporate social responsibility rating and local government competition intensity. Among them, the carbon trading pilot can significantly increase the green total factor productivity of large or high social responsibility rating iron and steel enterprises, but the promotion effect on small or low social responsibility rating iron and steel enterprises is not significant. Meanwhile, when the intensity of local government competition is high, the carbon trading pilot can significantly improve the green total factor productivity of local iron and steel firms; when the intensity of local government competition is low, the green total factor productivity of local iron and steel firms is not significantly boosted by the carbon trading pilot.
7 Policy recommendations and limitations
In order to accelerate the improvement of China’s carbon emission trading market mechanism and thus promote the development of China’s iron and steel enterprises towards green transformation, this paper makes the following suggestions:
First, when expanding the scale of the carbon emission trading market in the iron and steel industry, priority should be given to large or high social responsibility-rated enterprises with positive performance, and then gradually extended to enterprises with lower social responsibility ratings or smaller scale. In addition, the government should provide financial support or low-interest loans to iron and steel enterprises that encounter difficulties in their operations in order to motivate them to make a low-carbon transition. At the same time, it should also increase the investment in R&D of low-carbon technologies for iron and steel enterprises to improve their technology level and establish an effective incentive mechanism to promote the transformation of iron and steel enterprises to low-carbon direction. Secondly, during the construction of the carbon emission trading market in the iron and steel industry, we should take into full consideration the reality that the initial carbon emission quota is relatively lenient, and tighten the carbon quota to give better play to the policy effect of the market incentive-based environmental regulation tool, so as to release the effect of the “double carbon” policy on the green transformation of the iron and steel industry to a greater extent. The effect of the “double carbon” policy on the green transformation of the iron and steel industry can be released to a greater extent. Finally, we should establish a multi-objective promotion and assessment mechanism, regulate the competition among local governments, guide the benign competition among local governments, eliminate the market segmentation and local protectionism brought about by administrative planning, strengthen inter-regional cooperation, and play the role of convergence effect and leading demonstration, so as to achieve complementary advantages and common development. At the same time, it should also improve the relevant laws and regulations system, further increase the punishment of local governments and strengthen the supervision and restraint of local governments. We should advocate green development, improve the environmental governance capacity of local governments, pay more attention to the quality of economic development and the level of ecological environment, and guide local governments to change from “GDP as the basis of competition” to “green development as the competition goal".
As there are many links and uncontrollable factors in the process of implementing the carbon emissions trading system in China, the enterprises are also affected by the trading price, trading quota, etc., which can not be taken into account in all of these factors, and it is more difficult to obtain the trading data in this regard, which to a certain extent restricts the more in-depth study of this paper. Future research can be expanded in the following aspects: 1) Adopt multiple data acquisition methods, such as the technology of big data mining, to broaden the panel data at the enterprise level. 2) Improve the accuracy of indicator measurement to more accurately assess the emission reduction effect of iron and steel enterprises. 3) Combine the carbon emissions trading system with other environmental regulatory policies to further analyze its impact on the green transformation of iron and steel enterprises.
Data availability statement
The original contributions presented in the study are included in the article/supplementary material, further inquiries can be directed to the corresponding author.
Author contributions
WX: Conceptualization, Data curation, Methodology, Writing–original draft, Writing–review and editing. CJ: Conceptualization, Data curation, Methodology, Writing–original draft, Writing–review and editing. KJ: Conceptualization, Methodology, Writing–original draft, Writing–review and editing. XY: Writing–original draft, Writing–review and editing.
Funding
The authors declare that no financial support was received for the research, authorship, and/or publication of this article. The 2023 projects of the Liaoning Science Public Welfare Research Foundation (Soft Science Research Program) (Class B) (2023JH410600041); The key research projects of the Liaoning 2023 decision-making consultation and the new-type think tank (23-A008); The Liaoning Technical University “Double Top” program (10242552305900183); Innovation Centre for Digital Business and Capital Development of Beijing Technology and Business University (SZSK202205); and Chinese-style bank governance modernization and systemic risk prevention (23-A017).
Conflict of interest
The authors declare that the research was conducted in the absence of any commercial or financial relationships that could be construed as a potential conflict of interest.
Publisher’s note
All claims expressed in this article are solely those of the authors and do not necessarily represent those of their affiliated organizations, or those of the publisher, the editors and the reviewers. Any product that may be evaluated in this article, or claim that may be made by its manufacturer, is not guaranteed or endorsed by the publisher.
References
Abbas, H. S. M., Xu, X., and Sun, C. (2021). Role of foreign direct investment interaction to energy consumption and institutional governance in sustainable GHG emission reduction. Environ. Sci. Pollut. Res. 28 (40), 56808–56821. doi:10.1007/s11356-021-14650-7
Abbas, S., Gui, P., Chen, A., and Ali, N. (2022). The effect of renewable energy development, market regulation, and environmental innovation on CO2 emissions in BRICS countries. Environ. Sci. Pollut. Res. 29 (39), 59483–59501. doi:10.1007/s11356-022-20013-7
Adebayo, T. S., Ağa, M., Agyekum, E. B., Kamel, S., and El-Naggar, M. F. (2022). Do renewable energy consumption and financial development contribute to environmental quality in MINT nations? Implications for sustainable development. Front. Environ. Sci. 10, 1068379. doi:10.3389/fenvs.2022.1068379
Ali, N., Hu, X., Hussain, J., and Nilofar, M. (2021). Assessing the environmental impacts of COVID-19; A review. Polish Journal of Environmental Studies. Pol. J. Environ. Stud. 30 (5), 4401–4403. doi:10.15244/pjoes/130337
Ali, N., Phoungthong, K., Techato, K., Ali, W., Abbas, S., Dhanraj, J. A., et al. (2022). FDI, Green innovation and environmental quality nexus: new insights from BRICS economies. Sustainability 14 (4), 2181. doi:10.3390/su14042181
Annamalaisamy, B., and Vepur Jayaraman, S. (2023). Renewable energy for sustainable development in Asia-Pacific region: do foreign direct investment and regulatory quality matter? Sustain. Dev. 31 (1), 108–124. doi:10.1002/sd.2377
Calel, R., and Dechezleprêtre, A. (2016). Environmental policy and directed technological change: evidence from the European carbon market. Rev. Econ. statistics 98 (1), 173–191. doi:10.1162/rest_a_00470
Cao, K., He, P., and Liu, Z. (2020). Production and pricing decisions in a dual-channel supply chain under remanufacturing subsidy policy and carbon tax policy. J. Operational Res. Soc. 71 (8), 1199–1215. doi:10.1080/01605682.2019.1605471
Chen, G. (2009). The competition for FDI, environmental, regulation and pollution haven. World Econ. Stud. (06), 3–7+43+87. doi:10.13516/j.cnki.wes.2009.06.006
Chen, H. X., and Chen, Y. X. (2022). Research on the impact of environmental regulation on the green development of China’s industry-A quasi-natural experiment based on carbon emissions trading policy. Hainan Finance (03), 26–41. doi:10.3969/j.issn.1003-9031.2022.03.003
Cheng, Z., and Meng, X. (2023). Can carbon emissions trading improve corporate total factor productivity? Technol. Forecast. Soc. Change 195, 122791. doi:10.1016/j.techfore.2023.122791
Cui, B., Shui, Z., Yang, S., and Lei, T. (2022). Evolutionary game analysis of green technology innovation under the carbon emission trading mechanism. Front. Environ. Sci. 10. doi:10.3389/fenvs.2022.997724
Dai, X. (2019). Actively expand imports: the promotion mechanism and realization path of high quality development. J. Macro-quality Res. 7 (01), 60–71. doi:10.13948/j.cnki.hgzlyj.2019.01.006
Du, G., Yu, M., Sun, C., and Han, Z. (2021). Green innovation effect of emission trading policy on pilot areas and neighboring areas: an analysis based on the spatial econometric model. Energy Policy 156, 112431. doi:10.1016/j.enpol.2021.112431
Du, K., Li, P., and Yan, Z. (2019). Do green technology innovations contribute to carbon dioxide emission reduction? Empirical evidence from patent data. Technol. Forecast. Soc. Change 146, 297–303. doi:10.1016/j.techfore.2019.06.010
Duan, K., Liu, Y., Yan, C., and Huang, Y. (2023a). Differences in carbon risk spillovers with green versus traditional assets: evidence from a full distributional analysis. Energy Econ. 127, 107049. doi:10.1016/j.eneco.2023.107049
Duan, K., Zhao, Y., Wang, Z., and Chang, Y. (2023b). Asymmetric spillover from Bitcoin to green and traditional assets: a comparison with gold. Int. Rev. Econ. Finance 88, 1397–1417. doi:10.1016/j.iref.2023.06.036
Gai, Z. J., Zhao, J. G., and Zhang, G. (2018). Typical calculation and analysis of carbon emissions in thermal power plants. IOP Conf. Ser. Earth Environ. Sci. 128 (1), 012176. doi:10.1088/1755-1315/128/1/012176
Germeshausen, R. (2020). The European Union emissions trading scheme and fuel efficiency of fossil fuel power plants in Germany. J. Assoc. Environ. Resour. Econ. 7 (4), 751–777. doi:10.1086/708894
Habiba, U. M. M. E., Xinbang, C., and Anwar, A. (2022). Do green technology innovations, financial development, and renewable energy use help to curb carbon emissions? Renew. energy 193, 1082–1093. doi:10.1016/j.renene.2022.05.084
Hu, J., Huang, N., and Shen, H. T. (2020). Can market-incentive environmental regulation promote corporate innovation? a natural experiment based on China’s carbon emissions trading mechanism. J. Financial Res. (01), 171–189.
Hu, X., Ali, N., Malik, M., Hussain, J., Fengyi, J., and Nilofar, M. (2021). Impact of economic openness and innovations on the environment: a new look into ASEAN countries. Pol. J. Environ. Stud. 30 (4), 3601–3613. doi:10.15244/pjoes/130898
Hu, Y. F., and Ding, Y. Q. (2020). Can carbon trading mechanism balance corporate efficiency and green efficiency? Chin. J. Popul. Resour. Environ. 30 (03), 56–64. doi:10.12062/cpre.20191112
Hua, J., Zhu, D., and Jia, Y. (2022). Research on the policy effect and mechanism of carbon emission trading on the total factor productivity of agricultural enterprises. Int. J. Environ. Res. Public Health 19 (13), 7581. doi:10.3390/ijerph19137581
Huang, Y., Duan, K., and Urquhart, A. (2023). Time-varying dependence between Bitcoin and green financial assets: a comparison between pre-and post-COVID-19 periods. J. Int. Financial Mark. Institutions Money 82, 101687. doi:10.1016/j.intfin.2022.101687
Huang, Z. J. (2023). Difficulties and countermeasures for China's CCER reboot under the carbon neutrality goal. Southwest Finance (10).
Jiakui, C., Abbas, J., Najam, H., Liu, J., and Abbas, J. (2023). Green technological innovation, green finance, and financial development and their role in green total factor productivity: empirical insights from China. J. Clean. Prod. 382, 135131. doi:10.1016/j.jclepro.2022.135131
Li, C. G., Wang, S., and Guo, Y. H. (2023). Analysis of policy tools and pathways for low-carbon transformation development of iron and steel industry——a simulation study based on dynamic CGE model. Inq. into Econ. Issues.
Li, G., Guo, F., and Di, D. (2021). Regional competition, environmental decentralization, and target selection of local governments. Sci. Total Environ. 755, 142536. doi:10.1016/j.scitotenv.2020.142536
Li, Y., and Liu, W. (2023). Spatial effects of environmental regulation on high-quality economic development: from the perspective of industrial upgrading. Front. Public Health 11, 1099887. doi:10.3389/fpubh.2023.1099887
Liu, B., Chang, H., Li, Y., and Zhao, Y. (2023a). Analysis of influencing factors of industrial green and low-carbon transformation under the background of" double carbon": evidence from Sichuan province, China. Front. Environ. Sci. 11, 1257855. doi:10.3389/fenvs.2023.1257855
Liu, B., Ding, C. J., Hu, J., Su, Y., and Qin, C. (2023b). Carbon trading and regional carbon productivity. J. Clean. Prod. 420, 138395. doi:10.1016/j.jclepro.2023.138395
Liu, W., Qiu, Y., Jia, L., and Zhou, H. (2022a). Carbon emissions trading and green technology innovation—a quasi-natural experiment based on a carbon trading market pilot. Int. J. Environ. Res. Public Health 19 (24), 16700. doi:10.3390/ijerph192416700
Liu, Y., Tian, L., Sun, H., Yuan, L., and Zhang, X. (2022b). Marginal return-ability measurement of carbon emission right and its application to unification route analysis of carbon markets. J. Clean. Prod. 345, 130684. doi:10.1016/j.jclepro.2022.130684
Ma, G. S., and Duan, M. S. (2023). Potential risks of double-counting carbon emission reductions in environmental rights trading and countermeasures. Clim. Change Res.
Ma, X., Wang, C., Dong, B., Gu, G., Chen, R., Li, Y., et al. (2019). Carbon emissions from energy consumption in China: its measurement and driving factors. Sci. total Environ. 648, 1411–1420. doi:10.1016/j.scitotenv.2018.08.183
Meng, F. S., and Han, B. (2017). Research on impact of government environmental regulation on enterprises’ low carbon technology innovation behavior. Forecasting (01), 74–80. doi:10.11847/fj.36.1.74
Naeem, M. A., Karim, S., Rabbani, M. R., Bashar, A., and Kumar, S. (2023). Current state and future directions of green and sustainable finance: a bibliometric analysis. Qual. Res. Financial Mark. 15 (4), 608–629. doi:10.1108/qrfm-10-2021-0174
Naeem, M. A., Karim, S., Uddin, G. S., and Junttila, J. (2022). Small fish in big ponds: connections of green finance assets to commodity and sectoral stock markets. Int. Rev. Financial Analysis 83, 102283. doi:10.1016/j.irfa.2022.102283
Niizawa, H., Hayashi, D., and Liu, X. (2020). Features and prospect of China’s national GHG emissions trading scheme. Carbon Manag. 11 (2), 155–167. doi:10.1080/17583004.2020.1721975
Porter, M. E., and Linde, C. V. D. (1995). Toward a new conception of the environment-competitiveness relationship. J. Econ. Perspect. 9 (4), 97–118. doi:10.1257/jep.9.4.97
Qi, S., Cheng, S., and Cui, J. (2021). Environmental and economic effects of China’s carbon market pilots: empirical evidence based on a DID model. J. Clean. Prod. 279, 123720. doi:10.1016/j.jclepro.2020.123720
Qi, S. Z., Lin, D., and Cui, J. B. (2018). Do environmental rights trading schemes induce green innovation? evidence from listed firms in China. Econ. Res. J. 53 (12), 129–143.
Qi, S. Z., and Zhang, Z. Y. (2019). EU ETS, EUA allocation and renewable energy technological innovation. World Econ. Stud. 307 (09), 119–133+136.
Qi, X., and Han, Y. (2023). Research on the evolutionary strategy of carbon market under “dual carbon” goal: from the perspective of dynamic quota allocation. Energy 274, 127265. doi:10.1016/j.energy.2023.127265
Rao, X., Qiu, W. Y., Ai, J. Y., Lin, H. L., and Yang, N. (2023). Risk measurement and influence mechanism analysis of China’s carbon market under the background of dual carbon strategy. Ecol. Econ. 39 (11).
Rauf, A., Ali, N., Sadiq, M. N., Abid, S., Kayani, S. A., and Hussain, A. (2023). Foreign direct investment, technological innovations, energy use, economic growth, and environmental sustainability nexus: new perspectives in BRICS economies. Sustainability 15 (18), 14013. doi:10.3390/su151814013
Ren, X., Li, W., Duan, K., and Zhang, X. (2023). Does climate policy uncertainty really affect corporate financialization? Environ. Dev. Sustain., 1–19. doi:10.1007/s10668-023-02905-x
Saether, E. A., Eide, A. E., and Bjørgum, Ø. (2021). Sustainability among Norwegian maritime firms: green strategy and innovation as mediators of long-term orientation and emission reduction. Bus. Strategy Environ. 30 (5), 2382–2395. doi:10.1002/bse.2752
Shankar, V. K. A., Umashankar, S., Paramasivam, S., and Hanigovszki, N. (2016). A comprehensive review on energy efficiency enhancement initiatives in centrifugal pumping system. Appl. Energy 181, 495–513. doi:10.1016/j.apenergy.2016.08.070
Sheng Yin, X., and Hussain, J. (2021). The implication of technological innovation and tourism development on FDI-growth-environment nexus in Association of Southeast Asian countries: a simultaneity modeling analysis. Energy Sources, Part B Econ. Plan. Policy 16 (9), 878–902. doi:10.1080/15567249.2021.1971801
Song, D. Y., Zhu, W. B., and Wang, B. B. (2021). Micro-empirical evidence based on China’s carbon trading companies: carbon emissions trading, quota allocation methods and corporate green innovation. China Popul. Resour. Environ. 31 (1), 37–47. doi:10.12062/cpre.20200608
Tan, L., Yang, Z., Irfan, M., Ding, C. J., Hu, M., and Hu, J. (2023). Toward low-carbon sustainable development: exploring the impact of digital economy development and industrial restructuring. Bus. Strategy Environ. doi:10.1002/bse.3584
Tanaka, K., Matsukawa, I., and Managi, S. (2020). An experimental investigation of bilateral oligopoly in emissions trading markets. China Econ. Rev. 59, 101349. doi:10.1016/j.chieco.2019.101349
Tang, K., Zhou, Y., Liang, X., and Zhou, D. (2021). The effectiveness and heterogeneity of carbon emissions trading scheme in China. Environ. Sci. Pollut. Res. 28, 17306–17318. doi:10.1007/s11356-020-12182-0
Tian, Y., Tian, Z. M., Wang, N. J., Li, Y. N., Lv, J. Q., Ning, S. L., et al. (2022). Analysis on control measures for carbon emission sources from iron and steel industry in China and study on control strategy. Angang Technol. (05). doi:10.3969/j.issn.1006-4613.2022.05.001
Tong, L., Jabbour, C. J. C., Najam, H., and Abbas, J. (2022). Role of environmental regulations, green finance, and investment in green technologies in green total factor productivity: empirical evidence from Asian region. J. Clean. Prod. 380, 134930. doi:10.1016/j.jclepro.2022.134930
Wang, F., and Ge, X. (2022). Can low-carbon transition impact employment——empirical evidence from low-carbon city pilot policy. China Ind. Econ. (05), 81–99. doi:10.19581/j.cnki.ciejournal.2022.05.004
Wang, J. (2016). Carbon emissions trading system and biased clean technology effects. Econ. Rev. (02), 29–47.
Wang, W. D., Wang, D., and Lu, N. (2020). Research on the impact mechanism of carbon emissions trading on low-carbon innovation in China. China Popul. Resour. Environ. 30 (2), 41–48. doi:10.12062/cpre.20191007
Wei, J., and Zhang, X. (2023). The role of big data in promoting green development: based on the quasi-natural experiment of the big data experimental zone. Int. J. Environ. Res. Public Health 20 (5), 4097. doi:10.3390/ijerph20054097
Wen, J., Ali, W., Hussain, J., Khan, N. A., Hussain, H., Ali, N., et al. (2021). Dynamics between green innovation and environmental quality: new insights into South Asian economies. Econ. Polit. 39, 543–565. doi:10.1007/s40888-021-00248-2
Wen, Z. L., and Ye, B. J. (2014). Analyses of mediating effects: the development of methods and models. Adv. Psychol. Sci. 22 (05), 731–745. doi:10.3724/SP.J.1042.2014.00731
Xu, D., Abbas, S., Rafique, K., and Ali, N. (2023). The race to net-zero emissions: can green technological innovation and environmental regulation be the potential pathway to net-zero emissions? Technol. Soc. 75, 102364. doi:10.1016/j.techsoc.2023.102364
Yang, T., Liao, H., and Wei, Y. M. (2020). Local government competition on setting emission reduction goals. Sci. Total Environ. 745, 141002. doi:10.1016/j.scitotenv.2020.141002
Yin, X. G., and Chen, Y. J. (2022). Environmental regulation, local government competition and transformation of old-to-new economic drivers. Statistics Decis. 38 (14), 163–169. doi:10.13546/j.cnki.tjyjc.2022.14.033
Zang, J., Wan, L., Li, Z., Wang, C., and Wang, S. (2020). Does emission trading scheme have spillover effect on industrial structure upgrading? Evidence from the EU based on a PSM-DID approach. Environ. Sci. Pollut. Res. 27, 12345–12357. doi:10.1007/s11356-020-07818-0
Zhang, B., Yu, L., and Sun, C. (2022). How does urban environmental legislation guide the green transition of enterprises? Based on the perspective of enterprises' green total factor productivity. Energy Econ. 110, 106032. doi:10.1016/j.eneco.2022.106032
Zhang, P. D., and Zhang, H. L. (2021). Research on the path of improving total factor productivity of enterprises through environmental regulation: a quasi natural experiment based on carbon emission trading pilot. Jiang-huai Trib. (04), 44–51. doi:10.16064/j.cnki.cn34-1003/g0.2021.04.006
Zhang, Y. J., and Wang, W. (2019). Do renewable energy consumption and service industry development contribute to CO 2 emissions reduction in BRICS countries? Environ. Sci. Pollut. Res. 26, 31632–31643. doi:10.1007/s11356-019-06330-4
Zhang, Y. Z., and Qiao, Y. H. (2021). Research on the impact of environmental regulation on green total factor productivity in manufacturing industry: PSM-DID test based on carbon emission trading policy. Ecol. Econ. 37 (4), 34–40.
Zhu, C., and Ma, J. (2023). Dynamic strategies of horizontal low-carbon supply chains under double carbon policies: a bounded rationality perspective. Comput. Industrial Eng. 181, 109309. doi:10.1016/j.cie.2023.109309
Keywords: “double carbon” policy, iron and steel enterprises, green total factor productivity, carbon emission trading pilot, DID
Citation: Xu W, Jiang C, Jia K and Yu X (2023) Did the “double carbon” policy improve the green total factor productivity of iron and steel enterprises? a quasi-natural experiment based on carbon emission trading pilot. Front. Energy Res. 11:1307347. doi: 10.3389/fenrg.2023.1307347
Received: 04 October 2023; Accepted: 21 November 2023;
Published: 30 November 2023.
Edited by:
Xiaofeng Xu, China University of Petroleum, ChinaReviewed by:
Kun Duan, Huazhong University of Science and Technology, ChinaNajabat Ali, Soochow University, China
Copyright © 2023 Xu, Jiang, Jia and Yu. This is an open-access article distributed under the terms of the Creative Commons Attribution License (CC BY). The use, distribution or reproduction in other forums is permitted, provided the original author(s) and the copyright owner(s) are credited and that the original publication in this journal is cited, in accordance with accepted academic practice. No use, distribution or reproduction is permitted which does not comply with these terms.
*Correspondence: Kaiwei Jia, MTk4MGprd0AxNjMuY29t