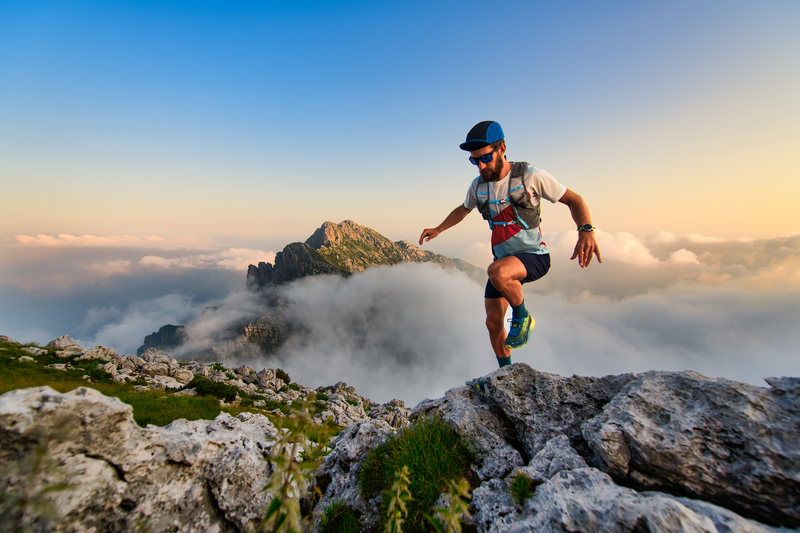
94% of researchers rate our articles as excellent or good
Learn more about the work of our research integrity team to safeguard the quality of each article we publish.
Find out more
HYPOTHESIS AND THEORY article
Front. Energy Res. , 29 November 2023
Sec. Energy Efficiency
Volume 11 - 2023 | https://doi.org/10.3389/fenrg.2023.1300158
With the proposal of “Carbon Peak and Carbon Neutrality” goals, China is facing a more serious carbon emissions reduction situation, and how the booming digital economy effectively helps China’s carbon emissions reduction is one of the most urgent things that should be solved. To study the impact of the digital economy on carbon emission intensity, this paper is based on the panel data of 30 provinces in China (excluding Tibet, Hong Kong, Macao, and Taiwan) from 2011 to 2021, and applies the double-fixed effect model and the threshold effect model to study the impact of the digital economy on carbon emission intensity and the mechanism of its action, as well as to analyze the mechanism of the digital economy’s action on carbon emission intensity from the perspective of technological innovation. The results of the study show that: i) The digital economy can reduce the intensity of regional carbon emissions; ii) The carbon emission reduction effect of the digital economy is non-linear, and its carbon emission reduction effect gradually increases with the level of development of the digital economy; iii) In addition to the direct impact of the digital economy on carbon emission intensity, it also has an indirect impact on carbon emissions through technological innovation; iv) There is regional heterogeneity in the carbon emission reduction effect of the digital economy, and the carbon emission reduction effect is more significant in the central and western parts of the country and regions with a high level of human capital development. Based on the conclusions obtained, this paper suggests: i) The rational integration of the digital economy and regional development should be strengthened; ii) Strong provinces in the digital economy should be encouraged to help weaker provinces, to narrow the “digital divide” between provinces; iii) Differentiated development strategies should be formulated in accordance with local conditions, to give full play to the optimal effect of the digital economy in carbon emission reduction.
In recent years, the digital economy has become a new driving force for China’s economic development and an important hand in constructing new national competitive advantages. With the rapid development of the digital economy, the environmental effects of the digital economy have gradually attracted the attention of scholars (Xue et al., 2022; Yu et al., 2022). While the digital economy is driving the economy to multiply, the operation of data centers and other infrastructures also has a multiplier effect on energy consumption, bringing about a large amount of carbon emissions. Therefore, in the context of the rapid development of the digital economy, many countries and regions are facing the problem of how to balance the growth of the digital economy and carbon emissions. China’s 14th Five-Year Development Master Plan, released in 2022, explicitly states that “the digital economy should be made one of the key pillars of China’s efforts to achieve the goal of carbon neutrality”. The development of the digital economy has been closely integrated with China’s carbon emission reduction process. Scientific assessment of the carbon emission reduction effect of the digital economy is not only an objective need to comprehensively accelerate the development of the digital economy but also a necessary way to sustainably promote China’s carbon emission reduction process.
The digital economy can be seen as a product of technological development in the new era, and the development of the digital economy cannot be separated from the support and innovation of technology. In turn, technological innovation plays a role in China’s carbon emission reduction process, so technological innovation is a key path to harmonize the development of the digital economy and carbon emissions. On the one hand, the technological changes brought about by the digital economy have eased the information asymmetry in the development of the energy system and played a positive role in reducing energy consumption and promoting carbon emission reduction (Ghisetti and Quatraro, 2017). On the other hand, the digital economy affects carbon emission intensity by weakening the constraints on the flow of factors of production and reducing the resources required per unit of output value through the construction of a digital governance system pathway (Cao et al., 2023). Therefore, the study of the role of technological innovation in the process of the digital economy affecting regional carbon emissions is crucial for China to realize the goal of “Carbon Peak and Carbon Neutrality”. Against this background, the motivation of this paper is to analyze the impact of the digital economy on regional carbon intensity and the role of technological innovation in the process of the digital economy’s impact on regional carbon emissions, and ultimately to propose targeted policy recommendations based on the results obtained.
This paper empirically examines the impact of the digital economy on regional carbon emissions using the provincial panel data of China from 2011 to 2021 as a sample. The double-fixed effect model and threshold effect model are used to test the mechanism by which the digital economy affects the intensity of regional carbon emissions. The mediating effect model is used to test the role of technological innovation in the process of carbon emission reduction by the digital economy. This paper has four marginal contributions: Firstly, the article examines the impact of the digital economy on carbon intensity from a holistic perspective. Existing studies have focused on examining the impact of the digital economy on carbon emission intensity in terms of one aspect of the digital economy, such as digital finance (Wang et al., 2022) or digital economic infrastructure (Song et al., 2021). Secondly, compared with the direct regression of the digital economy and carbon emissions in previous studies (Yu et al., 2022), this paper takes into account the possible heterogeneity of the role of the level of development of the digital economy on carbon emissions at different stages. The threshold effect model is used to test the long-term role of the digital economy on carbon emissions. Thirdly, considering that there may be a causal relationship between the digital economy and technological innovation, to avoid endogeneity problems (Wang and Hu, 2022), this paper adopts a combination of theoretical analysis and empirical tests to examine whether the influence mechanism of “digital economy - technological innovation - carbon emission intensity” is established. Fourthly, considering the heterogeneity of the carbon emission reduction effect of the digital economy (Yang and Jiang, 2021), the article analyzes the heterogeneity of the carbon emission reduction effect along with the digital economy from the perspectives of human capital and geographic location. The research in this paper provides a powerful decision-making reference for China to better deal with the relationship between the digital economy and carbon emissions, and to help the formulation and implementation of carbon emission reduction policies.
The remaining sections of this paper deal with the following: Section 2 is the literature review, Section 3 is the research hypothesis, Section 4 is the research design, Section 5 is the empirical analysis, and Section 6 is the conclusion.
The new round of scientific and technological revolution and industrial change is accelerating the evolution, and the flourishing rise of the digital economy has received continuous attention from the academic community. The earliest studies on the digital economy were mainly focused on the connotation of the digital economy, which is defined as a mode of economic development in which information is exchanged in a digitized form (Tapscott, 1966). As human society has gradually entered a new stage marked mainly by digitization, the connotation of the digital economy has been enriched. According to Modern Energy System Plan for the 14th Five-Year Plan, the digital economy is defined as an emerging economic form in which “data resources are the key elements, modern information networks are the main carriers, and information and communication technology is the main driving force”. With the continuous development of the digital economy, research on the digital economy has begun to gradually expand to study the economic effects of the digital economy, including the positive contribution of the digital economy to the upgrading of industrial structure (Chen and Yang, 2021) and the improvement of production efficiency (Yang and Jiang, 2021), and further focus on the environmental effects of the digital economy.
There is an academic consensus that the growth of the digital economy will cause changes in carbon emissions (Feng et al., 2023; Sun and Zhou, 2022; Xie, 2022). Table 1 summarizes relevant studies on the relationship between the digital economy and carbon emissions. However, there is still disagreement in the academic community about the specific effects of the development of the digital economy on carbon emissions. Most scholars believe that the development of the digital economy can reduce carbon emissions (Jiang and Jing, 2023; Lee et al., 2022; Xu et al., 2022; Yang and Shi, 2023). This part of the scholars believe that, on the one hand, the development of the digital economy can not only significantly reduce the emission of various types of pollutants (Deng and Zhang, 2022), but also through the extrusion effect of compression of the development of high-energy-consuming and high-carbon-emission industries space, forcing the green transformation of industrial structure (Han et al., 2022). On the other hand, the development of the digital economy mainly relies on the development of a large amount of data resources and powerful digital technology. Compared with the traditional industrial economy, the development of the digital economy can effectively reduce the consumption of fossil energy, and can optimize production methods and processes, and reduce carbon emissions by improving energy efficiency (Zhang et al., 2022). However, some scholars have pointed out that although the application of information technology can improve the efficiency of energy utilization, it will also further expand the energy demand, triggering a rapid growth in electricity consumption (Haseeb et al., 2019; Salahuddin and Alam, 2015). Based on the above two views, another viewpoint has been derived from the research on the impact relationship between the digital economy and carbon emissions: The relationship between the digital economy and carbon emissions is non-linear. The digital economy does not always have an inhibitory effect on carbon emissions, but only when the digital economy develops to a certain level will its carbon emission reduction effect come to the fore. Before that, the development of the digital economy will lead to an increase in carbon emissions, and when the development of the digital economy continues to improve, its impact on carbon emissions may become a positive contribution (Li et al., 2021; Liao et al., 2022; Xiangyan and Jinye, 2022; Wang et al., 2022). This part of scholars believes that the digital economy itself has high carbon-negative externalities, and the construction of digital technology facilities necessary for the development of the digital economy is precisely the source of carbon emissions from the digital economy (Wang and Wang, 2022). However, it is not yet known whether the resource-saving effects of the digital economy will outweigh its high-carbon negative externalities, making the long-term impact of digital technologies on carbon emissions unpredictable. Because of the controversial aspects of existing studies, this paper examines the effects of digital economic development on regional carbon emissions in the long run by using double-fixed effects model and panel threshold model based on data from 30 provinces in China from 2011 to 2021.
TABLE 1. Summary literature review of the relationship between the digital economy and carbon emissions.
The digital economy is a product of technological innovation, the digital economy is an economic model and mode of operation based on numbers and the Internet, and the continuous updating and application of digital technology has promoted the development of the digital economy. Table 2 summarizes studies related to the digital economy and technological innovation. Emerging technologies such as Big Data and Artificial Intelligence provide technical support for the digital economy and new opportunities and platforms for innovation. There are relatively few studies on the relationship between the digital economy and technological innovation. Most of the existing studies start from one aspect of the digital economy, such as ICT and digital finance, and study its impact on technological innovation. Wang and Qin (2022) used the system GMM model to examine the relationship between ICT and technological innovation in the cities of Chengdu-Chongqing region of China, and the study found that the relationship between ICT and technological innovation in Chengdu-Chongqing region of China is mutually reinforcing. Using a panel vector autoregressive model, Wang and Hu (2022) investigated the role of the relationship between digital financial development and technological innovation in Chinese provinces and found that the relationship between digital finance and technological innovation in Chinese provinces is mutually reinforcing. Chen et al. (2022) used the spatial durbin model and the threshold effect model to study the relationship between ICT and scientific and technological innovation in Chinese cities and found that ICT is conducive to enhancing the level of scientific and technological innovation in cities, and the innovation enhancement effect has the nonlinear characteristic of marginal increment. Liu (2023) used the panel effect model to study the relationship between the impact of digital finance on the innovation level of China’s manufacturing industry chain and found that digital finance can significantly promote the innovation level of the industry chain. Because of the above research status, this paper intends to construct the measurement system of the digital economy development level from three dimensions of digital economy technical facilities, digital industry development, and digital technology application, to get the digital economy development index, and to test the relationship between digital economy and technological innovation.
TABLE 2. Summary literature review of the relationship between the digital economy and technological innovation.
Currently, academic research on technological innovation and carbon emissions is relatively mature, and most scholars believe that technological innovation can significantly enhance carbon emission reduction and effectively curb carbon emissions (Gao et al., 2022; Khattak et al., 2022). Table 3 summarizes studies related to the impact of technological innovation on carbon emissions. Huo and Sun (2017) found that the enhancement of low-carbon technological capabilities can effectively curb carbon emission intensity. Tian, Sun, and Li (2018) found that technological innovation can effectively reduce and curb carbon emissions from resource-consuming industries by improving energy yield. Liu (2019) split technological capability into two dimensions, R&D intensity, and energy intensity, and found that both R&D intensity and energy intensity in Jiangsu Province can significantly inhibit CO2 emissions in the region. Wang and Xia (2020) used energy intensity as a proxy indicator for measuring local technological innovation capacity and found that energy intensity is an important factor influencing carbon emission intensity in Anhui and Henan Provinces. Yin et al. (2020) found that low-carbon technological advances can achieve carbon emission reductions by both carbon and energy intensity pathways. Hao et al. (2020) examined the impact of technological innovation on environmental pollution using panel data from 30 provinces in China from 1998–2016, and found that an increase in the level of technological innovation effectively reduces carbon dioxide equivalent. Using an autoregressive distributed lag model, Zhang X. et al. (2021) found that technological innovation significantly reduced CO2 emissions. By summarizing the relevant studies, it can be seen that there are more academic studies on the relationship between technological innovation and carbon emissions, and a consensus has been reached that technological innovation can significantly reduce regional carbon emissions.
TABLE 3. Summary literature review of the relationship between technological innovation and carbon emissions.
The digital economy is centered on digital technology, which is deeply integrated with energy, electricity, transportation, and other key areas of carbon emissions, deriving new business forms and accelerating the low-carbon transformation of society. The digital economy drives the transformation of residents’ lifestyles through the use of digital technologies. The application of digital technologies such as e-commerce platforms and mobile payments based on Big Data and Artificial Intelligence can effectively reduce unnecessary human activities and bring great convenience to people’s daily lives (Yu et al., 2017). Compared with the traditional mode of living, residents in the context of the digital economy live more efficiently, greatly reducing energy consumption in the course of human activities and reducing carbon emissions from the source. Another notable effect of the digital economy on carbon emissions is the realization of efficient resource allocation (He and Xu, 2020). On the one hand, the connectivity function of the digital economy itself can effectively alleviate information asymmetry and break down information exchange barriers. It turns cumbersome data into useful resource information, improves the output of information boundaries, and reduces the gap between factor supply and demand. Then it can allocate the means of production more accurately in different parts of production and improve the allocation efficiency of resources (Bingtao et al., 2023). The efficient flow and sharing of data among different subjects through the network infrastructure can effectively reduce the cost and time of factor searching, trading, copying, and matching. It provides great convenience for government enterprises and the public to adjust factor holdings in real-time, which helps enterprises formulate effective and reasonable production plans and avoid wasting resources (Goldfarb and Tucker, 2019). On the other hand, supportive policies and dividend subsidies in the process of digital economy development can produce a siphon effect. It helps to attract more highly sophisticated and innovative talents and realize the reconfiguration of talent, capital, and other resource elements (Song et al., 2021). It promotes the effective integration of traditional enterprises and new technologies, gives rise to new industrial development models, eliminates high-energy-consuming and high-polluting enterprises through the substitution effect, and effectively improves regional carbon emission pollution. Based on the above analysis, the following hypothesis is proposed.
Hypothesis 1. The digital economy can reduce carbon emission intensity.
According to the environmental Kuznets curve theory, environmental pollution will show a trend of increasing and then decreasing with economic growth (Yilanci et al., 2022). The digital economy, as an emerging economic form, may also have non-linear characteristics in terms of its impact on carbon emissions at different stages of development. The development of the digital economy is highly dependent on the operation of data infrastructure. The power consumption of digital infrastructure represented by data centers, 5G base stations, etc. during operation is huge. In the early stages of the digital economy, the rapid increase in the rate of energy consumption of digital economy infrastructure cannot be quickly offset in the short term due to slow growth in energy efficiency (Fang and Wang, 2022). In addition, due to the reform of the electricity system, the promotion of the bulkhead power sales model has been slow and the operation of data centers relies on non-renewable energy sources for power supply due to the cost pressure on companies to use renewable energy sources, at a time when the dampening effect of the digital economy on the intensity of carbon emissions may not be obvious (Junbo et al., 2022). With the development of the digital economy, the infrastructure required for its development has been perfected, and the development of the digital economy has gradually entered a “mature phase”. The digital economy has formally released the “dividend effect” of carbon emission reduction, reducing the intensity of carbon emissions by efficiently integrating factor resources and improving the efficiency of resource utilization. Based on the above analysis, the following hypothesis is proposed.
Hypothesis 2. There is a threshold effect of the digital economy on carbon emission intensity, and the carbon reduction effect of the digital economy shows a marginal incremental effect.
The digital economy impacts technological innovation by way of the penetration of digital technology applications. The impact of the digital economy on technological innovation is reflected in the following areas: First, the information and communication technology (ICT) industry in the digital economy is itself a highly knowledge-intensive industry with abundant innovation resources, and it is easier to produce innovations due to the frequent exchange of internal innovation information (Zhang et al., 2020). When these innovations are involved in the production and R&D process of real enterprises as intermediates, new supporting products will be derived, and innovations will be produced in the process. For example, when artificial intelligence, high-end sensors, and other digital technologies are put into the production of enterprises, it can realize the re-innovation of the production process of enterprises, and then transform some high-energy-consuming, low-output equipment, improve the utilization rate of resources to achieve energy saving and emission reduction (Zhang T. et al., 2021). Second, the application of Big Data, Cloud Computing, and other digital technologies can search, integrate, analyze, and make decisions on technological innovation information, help enterprises make judgments on the direction of innovation, innovation paths, etc., and prompt enterprises to carry out innovative activities so that they can be transformed from empirical judgments to data judgments, and realize effective technological innovation decisions (Yi et al., 2020). Technological innovation is the key channel to influence regional carbon emissions (Xinxin and Ning, 2021). The relationship between the roles of the digital economy, technological innovation and carbon emissions is shown in Figure 1. Based on the above analysis, the following hypothesis is proposed.
Hypothesis 3. The digital economy reduces regional carbon emissions by promoting regional technological innovation.
Based on prior research, this paper develops a double-fixed effects model as a baseline regression model to experimentally examine the influence of the digital economy in influencing carbon emission intensity. The baseline model is set up as follows:
Where
In previous studies, some studies chose to use the mediating effects model for mechanism analysis, a process that requires the simultaneous identification of three equations and is likely to generate multiple endogenous variables, so the use of the mediating effects model for mechanism analysis remains to be considered. To avoid the above problems, this paper draws on the practice of Ting Jiang (Jiang, 2022) to use regression models to test the role of explanatory variables on the mediating variables, while the effect of the mediating variables on the explanatory variables is mainly explained through theoretical statements. In this paper, model (2) is developed to test the relationship of the explanatory variables to the mediating variables.
where
According to the previous theoretical analysis, it can be seen that the impact of the level of digital economy development at different stages on the intensity of carbon emissions may have non-linear characteristics. This paper draws on Hansen (Hansen, 1999) to construct a panel threshold model as shown in Eq. 3:
where
Currently, the main methods used in academia to measure CO2 emissions are the emission factor method, the actual measurement method, and the material balance method. Tsinghua University’s MEIC database measures carbon emissions based on sub-sectoral energy consumption using the emission factor method. This paper uses this data as the basis for the carbon emission intensity calculated from the ratio of carbon emissions to GDP as an explanatory variable.
The digital economy is an emerging economic form, and although the academic community has not yet fully agreed on the measurement of its level of development, there are still some common indicators. Existing studies have mostly measured the level of development of the digital economy from the aspects of digital economic infrastructure, Internet development, digital industrialization, and industrial digitization. Referring to the research of Liu and Xu (Liu and Xu, 2023), this paper constructs the evaluation index system of the development level of the digital economy from the three aspects of digital economy infrastructure, digital industry development, and digital technology application, and the specific indexes are shown in Table 4. Digital economy infrastructure is the foundation and guarantee for the development of the digital economy, providing a solid underlying architecture for all kinds of digitalization scenarios. The development of the digital industry is the root and driving force of the digital economy, especially the most representative information and communication industry, whose development level will directly determine the research and development and application of key technologies. The application of digital technology is an important part of the digital economy and can accurately reflect the degree of integration between the digital economy and the real economy. To ensure the scientific objectivity of the evaluation results, this paper adopts the entropy value method to measure the digital economy development index.
Existing studies mostly use the number of patents as a measure of technological innovation. The State Intellectual Property Office of the patent classification has three kinds of patents: invention, utility model, and design patents, invention patents have the highest innovative content, and are more rigorous in the application, this paper invention patent applications to measure the level of technological innovation.
To ensure the reliability and accuracy of the research results, this paper draws on previous research literature and puts other factors that may have an impact on carbon emissions into the regression model, mainly including level of income, government expenditure, density of population, level of foreign trade and industrial structure (Cutcu et al., 2022; Dong et al., 2022; Xie, 2022; Yi et al., 2022; Cheng et al., 2023). The level of income (Incom) is expressed in terms of disposable income per inhabitant. Regional affluence represents the level of regional economic development, which has an impact on the level of regional energy consumption as well as carbon dioxide emissions (Chen et al., 2018). Government expenditure (Gov) is expressed using the ratio of fiscal expenditures to Gross Domestic Product (GDP). The main driver of the third energy transition is government behavior, and the role of government behavior in reducing carbon emissions cannot be ignored. The density of population (Density) is expressed as the ratio of the sum of the urban population and the temporary population of the urban area to the area of the urban area. An increase in population leads to an increase in demand for transportation, housing, etc., and consequently to an increase in carbon dioxide emissions. The level of foreign trade (Trade) is expressed as the ratio of total exports and imports to GDP. Foreign trade affects the division of labor among regions in the international competitive landscape, influences the industrial structure of each region, and has a significant impact on carbon dioxide emissions. Industrial structure (IS) is expressed as the ratio of value added in the tertiary sector to value added in the secondary sector. All of the above variables are summarized in Table 5.
In this paper, 30 provinces in China (excluding Tibet, Hong Kong, Macao, and Taiwan) are selected as research samples from 2011 to 2021. The data on carbon emissions comes from Tsinghua University’s MEIC database; the digital financial inclusion index comes from the Peking University Digital Financial Inclusion Index published by Peking University’s Digital Finance Research Center; and the rest of the data comes from provincial statistical yearbooks and the National Bureau of Statistics. To avoid the effect of heteroskedasticity in the regression, absolute data such as carbon emission intensity, level of income, and population density were logarithmized. Missing values were added to the data using linear interpolation or neighbor interpolation. Descriptive statistical analysis of the main variables is shown in Table 6.
To control for the macroeconomic environment and inter-individual differences that do not vary over time, regressions are conducted using a double-fixed effects model with province and year. This paper utilizes stata17.0 to test the impact of digital economic development on carbon emission intensity, and the regression results are shown in Table 7.
Column 1) of Table 7 is the result of a regression controlling only for year-fixed and province-fixed effects. Column 2) is the result of regression with control variables, controlling for year and province effects. The regression results show that the coefficients of the digital economy development index are −2.317 and −1.457, both of which are significantly negative at the 1% level, indicating that the digital economy can significantly reduce the intensity of regional carbon emissions. Hypothesis 1 is verified.
On the one hand, the development of the digital economy has promoted techno-economic paradigm change, altered China’s traditional concept of energy consumption, and had an important impact on people’s production and lifestyle. The application of digital technology not only provides great convenience for people’s daily life but also effectively reduces the pollution generated by residents outside (Luo and Li, 2015). Shared bicycles based on digital technology have improved the travel experience of residents and significantly reduced the pollution emissions and energy consumption brought about by the previous “one car per capita” mode of travel. At the same time, thanks to the development of digital technology, intelligent mapping systems and other navigation software are becoming increasingly sophisticated. It not only reports road anomalies in real-time but also plans optimal routes for users, thus improving public efficiency. It effectively relieves road congestion and reduces tailpipe emissions, thus effectively reducing carbon intensity. On the other hand, the application of digital technology effectively alleviates the problem of information asymmetry in production life and improves the efficiency of resource allocation. The digital economy, with the modern information network as an important carrier, breaks down the barriers of time and space so that enterprises in different regions can learn from each other’s experiences and effectively allocate resources according to their needs. The reorganization and reallocation of factors of production will greatly enhance the efficiency of energy use and reduce the intensity of regional carbon emissions.
To further confirm the reliability of the findings and to avoid omitted variables and endogeneity issues from affecting the results, this paper conducts a robustness check on the baseline regression results. The results are shown in Table 8.
In this study, two approaches are used to verify the robustness of the benchmark regression results. The first way is to replace the explanatory variables. Carbon emission per capita is used as a proxy variable for carbon emission intensity. To reduce the impact of heteroskedasticity on the regression, per capita carbon emissions are logarithmized. The regression results are shown in column 1) of Table 8. The regression results show that after replacing the explanatory variables, the coefficient of the digital economy development index is still significantly negative, indicating that the baseline regression results are highly credible. The second way that tests the stability of the baseline regression results is to use the instrumental variable method. Considering that it takes time to build the infrastructure necessary for the development of the digital economy, at the same time, the digital economy to help the development of traditional industries also needs to have a process of integration. Therefore, the current development level of the digital economy also depends to some extent on the level of digital economy development in the previous period. Because of this, this study chooses the digital economy development index with a lag of one period as an instrumental variable to test the robustness of the regression results from the perspective of endogeneity. The regression results are shown in column 2) and column 3) of Table 8. The result of the first-stage regression shows that the instrumental variables are significantly correlated with the explanatory variables at the 1% level, suggesting that the first-order lagged term of the digital economy development index is a suitable instrumental variable. The second-stage regression result shows that the coefficient of the first-order lag term of the digital economy development index is significantly negative at the 1% level, which is consistent with the baseline regression results, again indicating the robustness of the baseline regression results.
To verify whether there is a threshold effect of the digital economy on regional carbon emission intensity, this paper uses a panel threshold model to test the panel effect. The model regression results are shown in Table 9.
When the digital economy is the threshold variable, the F-statistic of the single threshold test is 27.17, which is significant at the 1% level, indicating that there is a nonlinear relationship between the digital economy and carbon emission intensity. The threshold value is 0.3809. The marginal carbon reduction effect of the digital economy is 0.681 when the digital economy development index is less than 0.3809, and 0.957 when the digital economy development index is greater than 0.3809. As the level of development of the digital economy increases, the carbon emission reduction effect of the digital economy is gradually increasing.
In the context of the actual situation, when the level of development of the digital economy is low, part of the infrastructure necessary for the development of the digital economy is still under construction, and the digital economy is not yet well integrated with traditional industries. In this process, the power consumption of digital economy infrastructure, mainly 5G base stations and data centers, grows faster, so the carbon emission reduction effect of the digital economy is relatively weak at this time. When the development of the digital economy into a “maturity” period, the carbon emission reduction effect of the digital economy is gradually highlighted. The use of digital technologies accelerates the improvement of energy efficiency in traditional industries, leading to a more pronounced carbon reduction effect.
Technological innovation is a key initiative to reduce carbon emissions and plays a positive role in promoting regional carbon reduction (Kahouli, 2018; Mensah et al., 2018; Wen et al., 2021; Gao et al., 2022; Khattak et al., 2022). There are two main reasons why technological innovation can reduce carbon emission intensity. On the one hand, technological innovation reduces carbon emission intensity by improving production efficiency, enhancing energy use efficiency, and developing cleaner energy sources to reduce dependence on traditional patterns of economic development and energy use. On the other hand, it can also change the employment structure of the workforce, the demand structure, and the structure of imports and exports, optimize the allocation of production factor resources, promote the development of the economy from a crude to an intensive mode, and thus promote the reduction of regional carbon emission intensity. Therefore, this paper analyzes the influence mechanism between the digital economy and carbon emission intensity with technological innovation as a mediating variable. The results of the regression of the digital economy on technological innovation are shown in Table 10.
The results show that the regression result of the digital economy on technological innovation is significantly positive at the 1% level, indicating that the development of the digital economy can significantly promote the level of regional technological innovation.
On the one hand, the digital economy provides new platforms and opportunities for technological innovation. Through the use of digital technology, innovators can more easily access and share information, making it easier to identify new business models and opportunities. For example, the development of technologies such as cloud computing and big data analytics has provided businesses with more efficient and accurate decision support, driving the generation of innovation. On the other hand, the digital economy provides a broader market for technological innovation. The spread of digital technology has made it easier for people to communicate and transact across geographies and borders. This provides a larger market and potential user base for new product and service innovations, which in turn contributes to the accelerated development of technological innovations. The improvement of technological innovation can change the lifestyle of residents, and the production mode of enterprises and improve the efficiency of resource allocation to reduce regional carbon emissions.
To more accurately explain the regional differences in the impact of the digital economy on carbon emission intensity, this paper conducts a heterogeneity test in terms of both regional location and human capital level.
To further explore the heterogeneity of digital economy development on regional carbon emissions, the sample is divided into two regional sub-samples: the eastern region and central and western regions. Then the regressions are performed on the two subsamples. The eastern region refers to regions with a higher level of economic development, while the central and western regions represent regions with a lower level of economic development. The regression results are shown in Table 11.
The results show that the coefficient of the effect of the digital economy on carbon emission intensity in the central and western regions is −3.164, which is significantly negative at the 1% level. In contrast, the coefficient of the digital economy’s impact on carbon emissions in the eastern region is not significant. It indicates that the digital economy has a significant inhibiting effect on the carbon emission intensity only in the central and western regions, while the inhibiting effect on the carbon emission intensity in the eastern region is not obvious.
The operation of data centers, 5G base stations, and other digital economy infrastructure require the consumption of large amounts of energy consumption, and currently, more than 60% of the cost of building data centers in China is power consumption (Ding et al., 2022). Due to the early development of the digital economy in the eastern region, Big Data, Artificial Intelligence, and other digital technologies have been fully utilized in the field of production and life (Yang and Xiaodong, 2021), so that the carbon emission reduction “dividend” effect of the digital economy is released in advance. Eastern China, which already needs to carry the electricity consumption of a large-scale population, can hardly bear the huge energy demand of data centers, 5G base stations, etc., and even directly define data centers, 5G base stations, etc., as high-energy-consuming industries and restrict their development. The unique energy resource endowment of the central and western regions offers the possibility of shifting the energy consumption pattern, with greater potential for carbon reduction. The central and western regions are rich in renewable energy and have a low electricity demand. At the same time, China’s “East Counts, West Counts” project guides the arithmetic demand of the eastern region to the western region in an orderly manner, greatly optimizing the construction layout of China’s data centers and making the arithmetic resources in the western region more adequately support the data computing in the eastern region. At the same time, the western region, due to the construction of data centers, has improved the utilization of its green energy sources such as photovoltaic power, wind power, and hydropower, increasing the supply of green power and significantly reducing the intensity of regional carbon emissions.
In this paper, the ratio of the number of students enrolled in general colleges and universities to the year-end resident population of the region is chosen to indicate the level of regional human capital, and the average value is used as the division threshold to divide the sample into two sub-samples, high and low. The regression results are shown in Table 12.
The regression results show that the coefficients of the digital economy development index in the regression results of the two groups of samples are negative, but the absolute value of the coefficients of the samples of regions with high human capital is larger and more significant. This indicates that the regional human capital level and the carbon emission reduction effect of the digital economy are positively correlated. The higher the regional human capital level, the more obvious the effect of the digital economy in reducing carbon emission intensity.
The development of the digital economy has given rise to a series of new technology industries, which have higher knowledge and quality requirements for related employees, and the human capital structure has been optimized in the process of the rising number of employees in these industries. From another perspective, an increase in human capital implies an increase in the knowledge, skills, and experience of the labor force, which is manifested in the actual production of innovations in the form of a more rapid ability to learn and apply new knowledge and skills, as well as a higher level of proficiency in the operation of labor skills (Dai and Xiong, 2023). These new changes can accelerate the upgrading and transformation of enterprises’ internal technology, which is conducive to improving production efficiency and cleaner production, and also helps to learn advanced technology from outside, laying down technical support for saving production and reducing carbon emissions.
The wave of digital change has facilitated the convergence of digital technologies and traditional industries, bringing great changes and opportunities to China’s economic development. Against the backdrop of the “Carbon Peak and Carbon Neutral” goals, what is the impact of the digital economy on regional carbon emissions reduction? Based on the above considerations, this paper empirically analyzes the impact of the development of the digital economy on carbon emission intensity and the role of technological innovation in it by selecting the panel data of 30 provinces (excluding Tibet, Hong Kong, Macao, and Taiwan) in China from 2011 to 2021. The results of the study show that: Firstly, the digital economy can reduce the intensity of regional carbon emissions and has an obvious carbon reduction effect. This result still holds after robustness tests such as replacing the explanatory variables and instrumental variable method. Secondly, the carbon emission reduction effect of the digital economy is non-linear, and the carbon emission reduction effect of the digital economy gradually increases with the level of development of the digital economy. Thirdly, the digital economy has an obvious role in promoting technological innovation, and the digital economy can affect regional carbon emission intensity by influencing the level of regional technological innovation. Fourthly, the results of heterogeneity analysis show that the carbon emission reduction effect of the digital economy has obvious regional location heterogeneity and human capital heterogeneity. The carbon emission reduction effect of the digital economy is more significant in the central and western regions and regions with a high level of human capital development.
Based on the above findings, the paper makes the following policy recommendations:
(1) Accelerating the development of the digital economy, consolidating the construction of digital economic infrastructure, and providing new momentum for carbon emission reduction in the provinces. Firstly, it is necessary to accelerate the construction of 5G base stations, data centers, and other infrastructures that carry digital technologies, to improve the level of “new infrastructure” construction. This will provide the basis for digital industrialization and industrial digitization, and thus promote the deep integration of the digital economy and traditional industries in each province. Secondly, accelerate the application of big data, artificial intelligence, and other digital technologies. Promote the penetration of the digital economy and digital technologies into traditional industries, and strengthen the wide application of the digital economy and digital technologies in production, life, and other areas. With digitization and intelligence as the guiding force, improve the level of digital development in various fields, promote changes in energy efficiency, reduce energy consumption, and thus reduce carbon emission intensity. Thirdly, relying on digital technology to accelerate the development of enterprise digital transformation. It will link up all aspects of production, research and development, sales, and other business processes, to realize the role of improving quality and efficiency. Continuously improve the efficiency of resource utilization and optimize the efficiency of energy resource allocation, to continuously reduce the intensity of regional carbon emissions. Fourthly, accelerate the application of intelligent energy construction, promote the intelligent upgrading of the whole process of energy production and utilization, promote the efficient green transformation of the energy industry chain, and effectively reduce the intensity of regional carbon emissions at source.
(2) Strengthen the scientific and technological research of new technologies, make full use of the advantages of data elements and digitization technology to support the development of technological innovation, and enhance the level of technological innovation in each province. Firstly, the government introduces policies and regulations related to technological innovation to create a favorable environment for technological innovation. Improve the laws and regulations system and intellectual property rights system, and create a fair competition environment and policy system in an all-round and multi-dimensional way, to stimulate enterprises to actively carry out activities related to technological innovation. Secondly, a sound data factor market should be constructed and institutional safeguards should be strengthened. Uniform and effective data standards should be established to enhance the smooth interconnection of data between regions, industries, and subjects, and to create a fair and open market. Enhance the market-oriented application of data, and integrate data resources into all segments of society to accelerate the marketization process. Thirdly, enterprises set up special R&D funds, which have greater flexibility and autonomy, enabling them to choose and promote potential technological innovation projects more freely, thus effectively enhancing the level of technological innovation of enterprises. Fourthly, digital platforms are being developed to facilitate the exchange of information on innovation. Task-oriented matching of talents in related fields from different regions using the advantages of algorithms is carried out rapidly. It realizes the efficient exchange of innovation information and improves the level of technological innovation in each region in the process of exchange.
(3) Based on regional differences, formulate strategies for the development of the digital economy according to local conditions and differentiation, to fully release the carbon reduction potential of the digital economy. Firstly, based on the differences in endowments of different regions and the differences in the impact of the digital economy on carbon emissions, adjust the pace of digital economy development in each region. Break down the industry barriers and geographical restrictions on new models and new business forms, and enhance the differences and synergies in the governance of the digital economy in each region. Secondly, the Government should focus on rationalizing the allocation of digital economy resources among different regions. While ensuring that the eastern region continues to maintain a positive trend in digital economy development, it should increase financial investment, infrastructure development, and policy support for the central and western regions. Thirdly, less-developed regions need to take the initiative to strengthen economic ties with developed regions and to catch up through competition, cooperation, and learning. By upgrading their level of digital economic development, they can promote coordinated regional development. Fourthly, developed regions should play the “radiation-driven effect”. Relying on their resources and technological advantages, they can drive the improvement of digital technological innovation in less developed regions and fully release the potential of data elements in less developed regions.
(4) Optimize the structure of human capital by taking the development of the digital economy as an opportunity. Firstly, digital technology should be utilized to cultivate cross-border and composite talents and to promote the transformation and upgrading of traditional industries. Through the systems of “learning by doing” and “industry-academia-research”, the digital literacy and skills of relevant workers will be upgraded, alleviating the current lack of digital talents in traditional employment positions. Secondly, accelerate the degree of integration between the existing education system and the development of the digital economy, enhance the interconnection between digital knowledge and skills and different disciplines and specialties, and lay a solid foundation of talents for the transformation of digital innovation research results and the green development of cities. Thirdly, continuously promote the transformation and upgrading of traditional industries empowered by the digital economy, to improve the level of human capital in traditional industries. The upgrading of traditional industries is forcing practitioners in traditional industries to quickly master new technologies and knowledge, thus promoting the upgrading of human capital levels in all aspects. Fourthly, establish a big data system at the national level. Network interconnection realizes cross-regional human capital sharing. The development of the platform will optimize the allocation of human capital, bridge the regional human capital density divide, and effectively promote the coordinated development of human capital across regions.
The original contributions presented in the study are included in the article/Supplementary Material, further inquiries can be directed to the corresponding author.
XY: Writing–review and editing. YZ: Writing–original draft.
The author(s) declare that no financial support was received for the research, authorship, and/or publication of this article.
The authors declare that the research was conducted in the absence of any commercial or financial relationships that could be construed as a potential conflict of interest.
All claims expressed in this article are solely those of the authors and do not necessarily represent those of their affiliated organizations, or those of the publisher, the editors and the reviewers. Any product that may be evaluated in this article, or claim that may be made by its manufacturer, is not guaranteed or endorsed by the publisher.
The Supplementary Material for this article can be found online at: https://www.frontiersin.org/articles/10.3389/fenrg.2023.1300158/full#supplementary-material
Bingtao, Q., Yu, Y., Ge, L., and Guo, Y. (2023). Smart carbon reduction: the effect and mechanism of digital economy development on urban carbon emissions. J. Guangdong Univ. Finance Econ. 38 (03), 4–23.
Cao, W., Zhao, W., and Si, Y. (2023). Impact of digital economy on low-carbon development: moderating effect and threshold effect of green technology innovation. Soft Sci. 37 (09), 47–54. doi:10.13956/j.ss.1001-8409.2023.09.07
Chen, C., Ye, A., and Chen, J. (2022). Effects of information and communication technology on urban innovation output. Econ. Geogr. 42 (10), 92–99+168. doi:10.15957/j.cnki.jjdl.2022.10.011
Chen, X., and Yang, X. (2021). The impact of digital economic development on the upgrading of industrial structure: based on the research of grey relational entropy. Reform (03), 26–39.
Chen, Z., Wu, S., Ma, W., Liu, X., Cai, B., Liu, J., et al. (2018). Driving forces of carbon dioxide emission for China’s cities: empirical analysis based on extended STIRPAT model. China Popul. Resour. Environ. 28 (10), 45–54. doi:10.12062/cpre.20180729
Cheng, Y., Zhang, Y., Wang, J., and Jiang, J. (2023). The impact of the urban digital economy on China's carbon intensity: spatial spillover and mediating effect. Resour. Conservation Recycl., 189. doi:10.1016/j.resconrec.2022.106762
Cutcu, I., Ozkok, Y., and Golpek, F. (2022). Environment, education, and economy nexus: evidence from selected EU countries. Environ. Sci. Pollut. Res. 30 (03), 7474–7497. doi:10.1007/s11356-022-22674-w
Dai, Y., and Xiong, Y. (2023). Digital economy, human capital and regional innovation efficiency. XIinjiang State Farms Economy (01), 27–38, 80.
Deng, R., and Zhang, A. (2022). Research on the impact of urban digital economy development on environmental pollution and its mechanism. South China J. Econ. (02), 18–37. doi:10.19592/j.cnki.scje.390724
Ding, Z., Cao, Y., Zhang, S., Wang, P., Liu, J., Cheng, M., et al. (2022). Coordinated operation for data center and power system in the context of energy Internet (I): energy demand management model of data center. Proc. CSEE 42 (09), 3161–3177. doi:10.13334/j.0258-8013.pcsee.210813
Dong, F., Hu, M., Gao, Y., Liu, Y., Zhu, J., and Pan, Y. (2022). How does digital economy affect carbon emissions? Evidence from global 60 countries. Sci. Total Environ. 852, 158401. doi:10.1016/j.scitotenv.2022.158401
Fang, Y., and Wang, W. (2022). Promoting the greening of new infrastructure to help achieve carbon neutrality. China Price.
Feng, L., Yang, W., Wang, Z., and Fan, X. (2023). Digital economy and urban carbon emission intensity in China: spatio-temporal evolution and mechanisms. China Popul. Resour. Environ. 33 (01), 150–160. doi:10.12062/cpre.20220610
Gao, P., Wang, Y., Zou, Y., Su, X., Che, X., and Yang, X. (2022). Green technology innovation and carbon emissions nexus in China: does industrial structure upgrading matter? Front. Psychol. 13, 951172. doi:10.3389/fpsyg.2022.951172
Ghisetti, C., and Quatraro, F. (2017). Green technologies and environmental productivity: a cross-sectoral analysis of direct and indirect effects in Italian regions. Ecol. Econ. 132, 1–13. doi:10.1016/j.ecolecon.2016.10.003
Goldfarb, A., and Tucker, C. (2019). Digital economics. J. Econ. Literature 57 (1), 3–43. doi:10.1257/jel.20171452
Gao, P., Wang, Y., Zou, Y., Su, X., Che, X., and Yang, X. (2022). Green technology innovation and carbon emissions nexus in China: does industrial structure upgrading matter?. Front. Psychol. 13, 951172. doi:10.3389/fpsyg.2022.951172
Guo, Y., Zhang, B., Chen, B., Yang, Q., and Li, J. (2019). Disparities in socio-economic drivers behind China's provincial energy-related mercury emission changes. J. Environ. Manag. 251, 109613. doi:10.1016/j.jenvman.2019.109613
Han, J., Chen, X., and Feng, X. (2022). The real challenge and path of enabling green development of digital economy. Reform (09), 11–23.
Hansen, B. E. (1999). Threshold effects in non-dynamic panels: estimation, testing, and inference. J. Econ. 93 (02), 345–368. doi:10.1016/s0304-4076(99)00025-1
Hao, Y., Wu, Y., Wu, H., and Ren, S. (2020). How do FDI and technical innovation affect environmental quality? Evidence from China. Environ. Sci. Pollut. Res. 27 (08), 7835–7850. doi:10.1007/s11356-019-07411-0
Haseeb, A., Xia, E., Saud, S., Ahmad, A., and Khurshid, H. (2019). Does information and communication technologies improve environmental quality in the era of globalization? An empirical analysis. Environ. Sci. Pollut. Res. 26 (09), 8594–8608. doi:10.1007/s11356-019-04296-x
He, D., and Xu, Y. (2020). Digital economy operation and supply-side structure remodeling. Study Dev. Strategy (04), 57–67.
Huo, Y., and Sun, P. (2017). A research on the effects of financial development and technological progress on carbon emission reduction: based on dynamic GMM method of provincial panel data. Ecol. Econ. 33 (07), 25–30+97.
Jiang, R., and Jing, X. (2023). Study on the impact of digital economy development on carbon emissions in beijing-tianjin-hebei region: empirical evidence from the city level in 2011-2019. Social Science of Beijing (04), 40–50. doi:10.13262/j.bjsshkxy.bjshkx.230404
Jiang, T. (2022). Mediating effects and moderating effects in causal inference China industrial economics, (05), 100–120. doi:10.3969/j.issn.1006-480X.2022.05.007
Junbo, L., Xiangyang, X., and Siwen, L. (2022). Lifecycle carbon footprint analysis of China’s power industry. China Population, Resources and Environment 32 (01), 31–41. doi:10.12062/cpre.20210624
Kahouli, B. (2018). The causality link between energy electricity consumption, CO2 emissions, R&D stocks and economic growth in mediterranean countries (MCs). Energy 145, 388–399. doi:10.1016/j.energy.2017.12.136
Khattak, S. I., Ahmad, M., Haq, Z. u., Gao, S., and Jiang, H. (2022). On the goals of sustainable production and the conditions of environmental sustainability: does cyclical innovation in green and sustainable technologies determine carbon dioxide emissions in G-7 economies. Sustain. Prod. Consum. 29, 406–420. doi:10.1016/j.spc.2021.10.022
Lee, C., Yuan, Y., and Wen, H. (2022). Can digital economy alleviate CO2 emissions in the transport sector? Evidence from provincial panel data in China. Nat. Resour. Forum 46 (3), 289–310. doi:10.1111/1477-8947.12258
Li, X., Liu, J., and Ni, P. (2021). The impact of the digital economy on CO2 emissions: a theoretical and empirical analysis. Sustainability 13 (13), 7267. doi:10.3390/su13137267
Liao, L., Chen, J., Fan, T., and Fan, Y. (2022). The impact of digital economy development on carbon emissions - panel data analysis based on 278 prefecture-level cities. South China Finance. doi:10.3969/j.issn.1007-9041.2022.02.004
Liu, J. (2023). Can digital finance promote innovation in the industry chain? Morden Manag. Sci. (04), 46–56.
Liu, J., Xu, X., and Li, S. (2022). Lifecycle carbon footprint analysis of China's power industry. China Popul. Resour. Environ. 32 (01), 31–41. doi:10.12062/cpre.20210624
Liu, T., and Xu, Z. (2023). Digital economy, heterogeneous technological innovation and carbon dioxide emissions. Sci. &Technology Prog. Policy 40 (13), 1–10. doi:10.6049/kjjbydc.Q202207206
Liu, X. (2019). Study on influencing factors of carbon emissions from industrial energy consumption based on STIRPAT model. Ecol. Econ. 35 (03), 27–31.
Liu, X., and Hui, N. (2021). Internet, technological innovation and high-quality manufacturing development ———heterogeneous effect analysis based on innovation model. Inq. into Econ. Issues (09), 143–155.
Liu, Y., and Chen, X. (2021). Eff. Digital Econ. Industrial Struct. Upgrade China Res. Econ. Manag. 42 (08), 15–29. doi:10.13502/j.cnki.issn1000-7636.2021.08.002
Liu, Y., Xie, K., and Deng, H. (2020). Data-driven R&D transformation of new product: a case study from the perspective of adaptive change in organizational practices. Popul. Res. 36 (03), 164–183. doi:10.3969/j.issn.1002-5502.2020.03.011
Luo, M., and Li, L. (2015). The innovation of bussiness model in Internet era: from value creation persepctive. China Ind. Econ. 01, 95–107.
Mensah, C. N., Long, X., Boamah, K. B., Bediako, I. A., Dauda, L., and Salman, M. (2018). The effect of innovation on CO2 emissions of OCED countries from 1990 to 2014. Environ. Sci. Pollut. Res. 25 (29), 29678–29698. doi:10.1007/s11356-018-2968-0
Salahuddin, M., and Alam, K. (2015). Internet usage, electricity consumption and economic growth in Australia: a time series evidence. Telematics Inf. 32 (04), 862–878. doi:10.1016/j.tele.2015.04.011
Song, D., Li, C., and Li, X. (2021). Does the construction of new infrastructure promote the “quantity” and “quality” of green technological innovation. China Popul. Resour. Environ. 31 (11), 155–164.
Sun, W., and Zhou, H. (2022). The effect and mechanism of digital economy on carbon emissions in Chinese cities. J. Environ. Econ. 7 (03), 25–42. doi:10.19511/j.cnki.jee.2022.03.002
Tian, Y., Sun, H., and Li, J. (2018). Study on the influencing factors and mechanism of low carbon transformation of resource-based industries: empirical analysis of dynamic panel data based on STIRPAT model. Ecol. Econ. 34 (08), 14–18+30.
Wang, D., and Qin, Z. (2022). The dynamic relationship between ICT, innovation ability and green high-quality development: based on the analysis of chengdu-chongqing area. Ecol. Econ. 38 (05), 75–81+106.
Wang, S., and Wang, H. (2022). Research on the strategies of healthy development of China’s digital economy under the background of carbon peaking and carbon neutrality goals. Contemp. Econ. Manag. 44 (08), 11–16. doi:10.13253/j.cnki.ddjjgl.2022.08.003
Wang, W., and Hu, C. (2022). Digital finance, scientific and technological innovation and carbon emission: empirical study based on provincial panel data. J. Tianjin Univ. Commer. 42 (03), 40–48.
Wang, X., and Li, J. (2022). Did digital economy effectively promote energy conservation and CO2 reduction? China Popul. Resour. Environ. 32 (11), 83–95. doi:10.12062/cpre.20221022
Wang, X., and Xia, D. (2020). Research on carbon emission and influencing factors of industrial coal production and consumption———comparison of Anhui and henan provinces based on STIRPAT-EKC. Soft Sci. 34 (08), 84–89. doi:10.13956/j.ss.1001-8409.2020.08.14
Wang, Y., Zhang, Y., and Li, J. (2022). Digital finance and carbon emissions: an empirical test based on micro data and machine learning model. China Popul. Resour. Environ. 32 (06), 1–11. doi:10.12062/cpre.20220422
Wen, J., Ali, W., Hussain, J., Khan, N. A., Hussain, H., Ali, N., et al. (2021). Dynamics between green innovation and environmental quality: new insights into south asian economies. Econ. Polit. 39 (02), 543–565. doi:10.1007/s40888-021-00248-2
Xiangyan, W., and Jinye, L. (2022). Did the digital economy effectively promote energy conservation and CO2 reduction? [J]. China Population, Resources and Environment 32 (11), 83–95. doi:10.12062/cpre.20221022
Xie, Y. (2022). The effect and mechanism of digital economy on regional carbon emission intensity. Contemp. Econ. Manag. 44 (02), 68–78. doi:10.13253/j.cnki.ddjjgl.2022.02.008
Xinxin, Y., and Ning, H. (2021). Internet, technological innovation and high-quality manufacturing development—heterogeneous effect analysis based on innovation model. Inquiry into Economic Issues 09, 143–155.
Xu, W., Zhou, J., and Liu, C. (2022). The impact of digital economy on urban carbon emissions: based on the analysis of spatial effects. Geogr. Res. 41 (01), 111–129. doi:10.11821/dlyj020210459
Xue, F., Zhou, M., and Liu, J. (2022). The effect of DIgital infrastructure on reducing carbon emissions: quasi natural experiment based on "broadband China" pilot. South China J. Econ. 10, 19–36. doi:10.19592/j.cnki.scje.391533
Yang, H., and Jiang, L. (2021). Digital economy, spatial effects and total factors productivity. Stat. Res. 38 (04), 3–15. doi:10.19343/j.cnki.11-1302/c.2021.04.001
Yang, J., and Shi, Y. (2023). Has the digital economy improved regional carbon emission levels? Wuhan. Finance Mon. (05), 51–58+20.
Yang, L., and Xiaodong, C. (2021). The Effect of digital economy on industrial structure upgrade in China. Research on Economics and Management 42 (08), 15–29. doi:10.13502/j.cnki.issn1000-7636.2021.08.002
Yi, L., Kang, X., and Honglin, D. (2020). Data-driven R&D transformation of new product: a case study from the perspective of adaptive change in organizational practices. Population Research 36 (03), 164–183. doi:10.19744/j.cnki.11-1235/f.2020.0040
Yi, M., Liu, Y., Sheng, M. S., and Wen, L. (2022). Effects of digital economy on carbon emission reduction: new evidence from China. Energy Policy 171, 113271. doi:10.1016/j.enpol.2022.113271
Yilanci, V., Cutcu, I., and Cayir, B. (2022). Is the environmental Kuznets curve related to the fishing footprint? Evidence from China. Fish. Res. 254, 106392. doi:10.1016/j.fishres.2022.106392
Yin, H., Wang, W., Wang, L., and Jiang, H. (2020). How do low-carbon technological advances curb carbon emissions? ---Empirical evidence from China. Mod. Manag. 40 (05), 90–94. doi:10.19634/j.cnki.11-1403/c.2020.05.021
Yu, B., Ma, Y., Xue, M., Tang, B., Wang, B., Yan, J., et al. (2017). Environmental benefits from ridesharing: a case of beijing. Appl. Energy 191, 141–152. doi:10.1016/j.apenergy.2017.01.052
Yu, S., Fan, X., and Jiang, H. (2022). Research on the impact of economy development on carbon productivity improvment. J. statistics Inf. 37 (07), 26–35. doi:10.3969/j.issn.1007-3116.2022.07.003
Zhang, J., Fu, K., and Liu, B. (2022). Can digital economy promote low-carbon transformation of cities from the perspective of dual objective constraint. Mod. Finance Economics-Journal Tianjin Univ. Finance Econ. 42 (08), 3–23.
Zhang, S., Wen, J., and Liu, H. (2020). Digital economy innovation: a comprehensive perspective. Econ. (02), 80–87.
Zhang, T., Jiang, F., and Wei, Z. (2021). Can digital economy become a new driving force for China's high-quality economic development? Inq. into Econ. Issues (01), 25–39.
Keywords: digital economy, carbon emission intensity, technological innovation, double-fixed effect, mediating effect, threshold effect
Citation: Yan X and Zhang Y (2023) Empirical study on the impact of digital economy on carbon emission intensity--based on the mediating role of technological innovation. Front. Energy Res. 11:1300158. doi: 10.3389/fenrg.2023.1300158
Received: 23 September 2023; Accepted: 13 November 2023;
Published: 29 November 2023.
Edited by:
Dan Wang, Tianjin University, ChinaReviewed by:
Ibrahim Cutcu, Hasan Kalyoncu University, TürkiyeCopyright © 2023 Yan and Zhang. This is an open-access article distributed under the terms of the Creative Commons Attribution License (CC BY). The use, distribution or reproduction in other forums is permitted, provided the original author(s) and the copyright owner(s) are credited and that the original publication in this journal is cited, in accordance with accepted academic practice. No use, distribution or reproduction is permitted which does not comply with these terms.
*Correspondence: Yan Zhang, enlfMTk5ODA5MTdAMTYzLmNvbQ==
Disclaimer: All claims expressed in this article are solely those of the authors and do not necessarily represent those of their affiliated organizations, or those of the publisher, the editors and the reviewers. Any product that may be evaluated in this article or claim that may be made by its manufacturer is not guaranteed or endorsed by the publisher.
Research integrity at Frontiers
Learn more about the work of our research integrity team to safeguard the quality of each article we publish.