- 1Department of Electrical Engineering, King Faisal University, Al Hofuf, Saudi Arabia
- 2Department of Electrical Engineering, Al-Baha University, Alaqiq, Saudi Arabia
- 3School of Electrical Engineering, Southeast University, Nanjing, China
- 4Quaid-e-Awam University of Engineering Science and Technology Nawabshah, Nawabshah, Sindh, Pakistan
- 5Department of Electrical Engineering, Northern Border University, Arar, Saudi Arabia
- 6Department of Electrical Engineering, University of Business and Technology, Jeddah, Saudi Arabia
- 7Engineering Mathematics Department, Faculty of Engineering, Alexandria University, Alexandria, Egypt
- 8School of Electrical Engineering, Beijing Jiaotong University, Beijing, China
- 9Department of Electrical Engineering, College of Engineering, Jouf University, Sakaka, Saudi Arabia
The growing popularity of electric vehicles presents a significant challenge to current electric grids since the rising number of these vehicles places additional strain on power systems inside distribution networks. A proposed paradigm is presented for electric vehicles (EVs), which is subsequently partitioned into three distinct dispatching areas to assess its practicality. This study is structured around two primary objectives. The first objective focuses on EV owners aiming to minimize their electricity consumption costs while also receiving compensation for providing services. The second objective involves using an aggregator to establish distinct tariffs for each dispatching area. Additionally, the aggregator aims to shift the charging load demand from peak to off-peak hours and distribute the charging demand to each agent. The authors of this study propose the utilization of a charging and discharging coordination method, specifically the Multi-Agents Charging and Discharging (MACD) algorithm, as a means to successfully tackle the issue of charging demand during peak hours. The objective of the proposed algorithm is to effectively handle the increased charging requirements during peak periods by using vehicle-to-grid (V2G) technologies within the context of Smart Grid systems and electric vehicle (EV) batteries. Importantly, this reduction is achieved without compromising the performance of electric vehicles (EVs) or the convenience experienced by EV owners. The algorithm under consideration demonstrates reduced power charging costs for various sectors. Specifically, it achieves a decrease of 15% for households, 14.6% for corporate buildings, and 14.5% for Industrial Park EV aggregators.
1 Introduction
Electric Vehicles (EVs), designed and manufactured to be integrated into the future transportation system, have garnered increased attention due to various factors including their environmentally friendly nature, high power efficiency, safety features, and potential for energy management (Habib et al., 2022). The electric vehicle (EV) is considered a sustainable system with the potential to contribute to reducing CO2 emissions (Ma et al., 2017). Electric vehicles (EVs) can be categorized into two distinct types: Battery EV (BEV) and Plug-in Hybrid EV (PHEV) (Adapa et al., 2018). The BEV (Battery Electric Vehicle) is devoid of an Internal Combustion Engine (ICE), hence relying solely on battery packs to supply power to its electric motor (Chen et al., 2013). The issue of increasing fossil fuel use has been a topic of discussion within the research and development (R&D) community since the late 1900s. The consumption of fossil fuels has experienced an upward trend, leading to the emergence of environmental hazards, including energy independence (5). In order to effectively tackle these global challenges, governments must formulate and implement appropriate policy measures. The transportation sector is responsible for a significant proportion of the carbon dioxide (CO2) present in the Earth’s atmosphere. In recent times, there has been a global focus on the electrification of the transportation sector as a viable and promising solution to address the aforementioned challenges. Several European countries have made the decision to implement electrification of their surface transportation systems in the near future (Zhang et al., 2015). Electric vehicles (EVs) are widely regarded as essential elements in the realization of a smart grid. Electric vehicles (EVs) possess several characteristics that render them appealing to both the smart grid and EV owners alike. Electric vehicles (EVs) are required to undergo charging and obtain electricity from the electrical grid once the State of Charge (SOC) of their batteries reaches a depleted state (Wang et al., 2013). The electric vehicle has been widely recognized as a viable and sustainable solution to mitigate our reliance on conventional fuel sources while decreasing our carbon emissions. During the early phase, the progress of electric vehicles (EVs) is hindered by the significant production expenses and the limited charging infrastructure availability (Zeng and Wu, 2017). Electric vehicles (EVs) have garnered growing attention from various businesses and nations due to their environmentally friendly nature and energy efficiency. Additionally, they serve as an auxiliary energy resource, helping reduce the power grid strain. Therefore, the functionalities of an electrical vehicle can be categorized into two elements. Firstly, the battery of the EV can be seen as a variable load (Ahmed et al., 2023). Individuals who own these vehicles can enhance their financial gains by implementing optimal or smart charging strategies for electric vehicle (EV) batteries. This can be achieved by procuring energy from the Grid to Vehicle (G2V) system at the most economical electricity rates. The EV battery can also function as an energy storage unit, allowing for the potential provision of energy back to the grid through the Vehicle to Grid (V2G) system. Integrating a significant number of electric vehicles into the power grid, together with the advancement of vehicle-to-grid technology, has led to several ancillary services to the grid. These services include reserve power supply, peak shaving, and voltage and frequency regulation (Li et al., 2018).
The Electric vehicle (EV) is a burgeoning load category that offers potential for enhanced flexibility in power consumption while charging EV batteries. This particular load type is considered a demand response asset due to its ability to modify the charging of individual EV batteries, as long as their energy requirements are met within the specified energy deadline (Li and Shahidehpour, 2016). Nevertheless, it is essential to note that electric vehicles (EVs) do not have zero carbon dioxide (CO2) emissions in practice, as they rely on energy derived from the power system, which includes non-renewable generation units. The extent of CO2 emissions produced by EVs depends on the composition of electricity sources (Barbour et al., 2017). Hence, the extent of the decrease in carbon dioxide (CO2) emissions exhibits variation across different geographical areas. However, it should be noted that electric cars (EVs) continue to exhibit reduced global warming emissions compared to conventional internal combustion engine vehicles, as supported by a countrywide study (Liu and Wang, 2016).
Over the recent years, there has been a substantial increase in the sales volume of electric vehicles (EVs). Figure 1 illustrates the varying volume of electric vehicle (EV) sales on a monthly basis from 2017 to 2019. The data reveals a growth in EV sales over the first 6 months of 2019, reaching a level nearly equivalent to that of 2018 in July, followed by a subsequent decline in December. Table 1-1 presents a comprehensive overview of the ten highest-selling plug-in electric vehicles (PEVs) in the global market throughout the year 2018, as indicated by reference (Zhang et al., 2017a). According to a survey, there is a projected increase in the adoption of electric vehicles (EVs) in 13 regions of the United States, with an estimated penetration rate of 25% by the year 2020 (Zhang et al., 2017b). Table 1 presents a comprehensive overview of the 10 highest-selling brands within the electric car market.
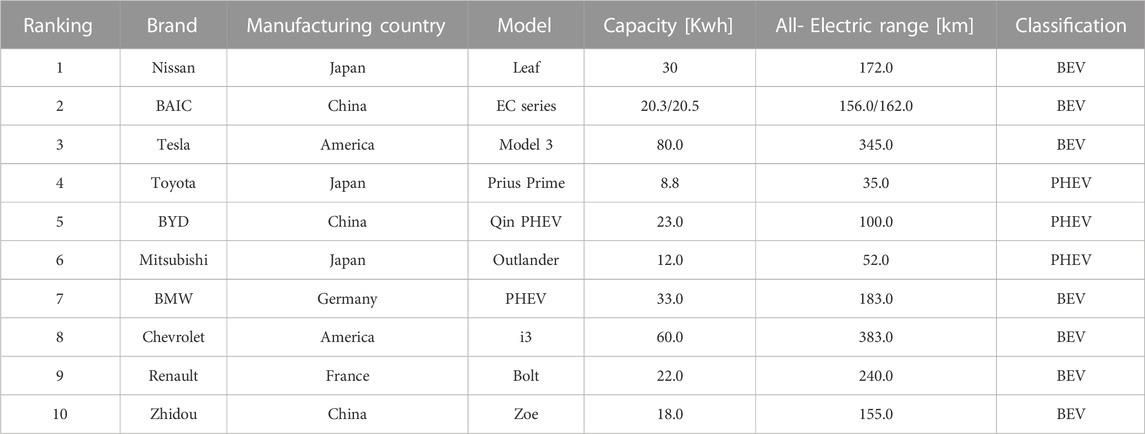
TABLE 1. Top 10 best-selling PEV products in international market 2018 (Adapa et al., 2018).
With the global proliferation of the electric vehicle (EV) market, there has been a corresponding surge in the development of technologies associated with electric vehicles. Consequently, several studies have been conducted in this field. One of the technologies under question is referred to as vehicle-to-grid (V2G) (Farag and Cheng, 2016). The conventional charging stations were limited to facilitating unidirectional energy transfer just from the power grid to electric vehicles (EVs). However, advancements in technology and recent research have introduced the concept of bi-directional power flow between electric vehicles (EVs) and the power grid, resulting in several advantages. In an alternative interpretation, it can be posited that electric vehicles (EVs) function in two capacities: as a conventional load and as a mobile generation unit or variable load (Gao and Wei, 2017).
This technological advancement enables electric vehicles (EVs) to not only draw energy from the power grid for battery charging purposes, but also to contribute energy or auxiliary services back to the power grid (Alizadeh-Mousavi and Rabiee, 2015). The provision of energy and associated services by electric vehicles (EVs) using vehicle-to-grid (V2G) technology offers advantages to both EV owners and power grid operators. Specifically, it generates financial gains for vehicle owners while simultaneously enhancing the financial viability, stability, and dependability of the power grid. A model for calculating optimal regulation power is developed in order to maximize the profitability of an aggregator and effectively monitor the operation of the frequency load control signal provided by the grid operator (Nazaripouya and Oo, 2017).
Hence, capitalizing on the underutilized automobiles during this significant time period seems to be appealing for both vehicle owners and grid operators. Therefore, it has garnered significant interest from scholars throughout the course of the last 10 years. In contemporary times, electric vehicles (EVs) that are connected to the power grid serve not only as charging loads, but also possess the capability to function as power generation and energy storage units inside the power grid (Ehsan and Yousefi, 2016). Participation in grid ancillary services is vital for maintaining stability and security within the electricity grid. These services include frequency regulation, voltage regulation, load peak shifting, and spinning reserve.
2 The proposed model
The scheduling technique utilized for the combined electric vehicle (EV) aggregator in this research is founded on the implementation of load control over the entire region, also known as area load control. The optimization of area load control involves the scheduling of charging and discharging activities for electric vehicle (EV) batteries within a specified geographical area, as opposed to a collective load management approach that encompasses all areas in a regional load control plan. One illustration of this concept is the engagement of a household EV aggregator in the management of the charging and discharging activities of electric vehicles (EVs) inside a residential area. This same principle applies to commercial buildings as well. The EV aggregator and industrial park EV aggregator technique is employed to effectively manage the charging and discharging activities of electric vehicles (EVs) within commercial and industrial areas, as seen in Figure 2. The aggregate daily power load consumption in the context of area load control is determined by adding up the quantity of electric vehicles (EVs) that are connected, as well as the other utility loads present within each specific area. The system is specifically engineered for electric vehicles (EVs), which is why the combined aggregator does not consider non-EV load as a relevant factor. The differentiation between EV load and other traditional loads utilized in area load control can be achieved through a simple process of differentiation. The aggregate power for the area load control is derived from the cumulative power consumption of residential, commercial, and industrial sectors within the designated area.
2.1 Aggregator mathematical model
The proposed model comprises of a two-layer optimization framework. In the upper layer, a combined aggregator is employed to oversee a cluster of three distinct EV aggregators/agents. This is achieved through the use of a centralized controller. The primary objective of the upper layer is to minimize the charging cost for each EV aggregator while adhering to the constraints imposed by the charging demand. In the lower layer, each EV aggregator/agent is connected to a number of EVs through a centralized controller. The main goal of the lower layer is to minimize the overall charging cost of each EV, taking into account the time of use (TOU) electricity price scheme associated with the power constraints of the batteries, charging demand, and state of charge. The primary goals of the integrated electric vehicle (EV) aggregator are to effectively manage the timing of charging and discharging activities for the EV agents it oversees, as well as to allocate the necessary energy demand to each individual EV agent under its purview. The aggregated entity will submit a bid directly to the utility on behalf of each individual agent, resulting in the acquisition of distinct tariff plans for each electric vehicle (EV) aggregator inside the collective. Figure 3 provides a clear depiction of the operational principles underlying the two-layer optimization model.
2.2 Upper layer optimization model
2.2.1 Objective function
The optimization model for the combined EV aggregator is shown in Eq. 1 and Eq. 2.
2.2.2 Constraints
The power demand constraint is:
The upper and lower bound constraints of the charging power exchanged between the combined EV aggregator and the associated EV aggregator (households, business building and industrial park) are:
2.3 Lower layer optimization model
2.3.1 Objective function
Objective function in this optimal scheduling problem is now shown in Eq. 5 and Eq. 6. The variables used in this problem is charging power control and aggregator energy level to understand the problem at hand clearly.
2.3.2 Constraints
Where
Minimum power name is just a sign conversion as the charging power is positive and the discharging power is negative. Where
2.4 The proposed multi agent charging and discharging (MACD) algorithm
This study examines the scheduling solution for electric vehicles (EVs) to engage in vehicle-to-grid (V2G) technology, aiming to minimize the charging cost for EV owners inside each aggregator that is connected to a consolidated EV aggregator. In order to validate the efficacy of the outcomes attained by linear programming optimization models, the subsequent section of this work presents the creation of those models.
The algorithm under consideration establishes a correlation between charging and discharging operations during periods of high demand, with the aim of addressing the power demand issue during peak hours. It is commonly assumed by authors that the control of algorithms resides with the aggregator. The integrated aggregator initiates contact with each electric vehicle (EV) aggregator and gathers data on charging demand from EV agents using smart meters and surveys. Subsequently, the aggregator would undertake the task of arranging the charging and discharging procedures pertaining to the automobiles belonging to their customers. The communication between the aggregator and the agents is outside the scope of this thesis. However, it is additionally presumed that a continuous communication channel exists between the aggregator and the agents. This channel will transmit the state of charge (SOC) of the vehicle, as well as the instructions for charging and discharging. The aggregator employs the algorithm proposed in order to determine the selection of electric vehicles (EVs) that will engage in charging activities and those that will engage in discharging activities, based on the time of use pricing associated with each EV agent. The primary determinants influencing the algorithm are the state of charge (SOC) of each electric vehicle (EV). The objective of the algorithm is to minimize electricity costs and mitigate peak power needs. In order to enhance the dependability of our algorithm, the electric vehicles (EVs) are categorized according to three criteria: battery capacity, charging rate, and charging efficiency.
The Multi agent Charging and Discharging (MACD) algorithm aims to mitigate power use during peak periods by redistributing the load demand for charging. The charging process may occur at peak or off-peak times, depending on the state of charge (SOC) of the battery and the power requirements of each electric vehicle (EV) agent. Furthermore, in the event of an emergency situation where the vehicle departs from the station with an insufficient State of Charge (SOC) to fulfill the power requirements, owners have the option to recharge their vehicle at a designated charging station. Nevertheless, the discharge processes will occur during periods of high demand. The term “peak time” originated from the practice of charging higher prices for utility services during periods of high demand. Hence, the power consumption curve will have an upward trend over this period. Furthermore, there was a notable escalation in power prices throughout this period. The utilization of the MACD algorithm is not obligatory for clients. The algorithm encouraged owners of electric vehicles in households to charge their vehicles during nighttime hours when demand is low and prices are reduced. The method will compute the state of charge (SOC) and charging demand for electric vehicle (EV) agents located in commercial buildings and industrial parks. In this study, the authors employ Level 2 chargers for all electric vehicle (EV) agents. Furthermore, electric vehicles (EVs) have the capability to either depart from or remain at a charging station depending on the charging requirements of the owner. It is uncertain whether all electric vehicles (EVs) would be eligible to participate in the MACD algorithm. If individuals were to engage in the activity, they would be able to provide assistance to the power grid or the household during periods of high demand.
According to the data presented in Figure 4, the MACD algorithm is utilized to compute the charging demand for each electric vehicle (EV) agent, while also verifying the adequacy of the state of charge (SOC) in the battery. In the event that the state of charge (SOC) is deemed inadequate, the algorithm initiates electric vehicle (EV) charging until the power level reaches a satisfactory level or until the scheduled departure time is reached. If the electric vehicle (EV) driver does not require electricity, the MACD algorithm will initiate the discharge of the EV battery until the state of charge (SOC) reaches a certain threshold value, as previously mentioned. The depletion is stated to be at a rate of 20% according to reference (Zhang et al., 2017a). In essence, the battery’s efficiency and overall condition will be compromised if the battery is discharged below this specified threshold. The MACD algorithm performs updates on the state of charge (SOC) for all electric vehicles (EVs) participating in the system at the commencement of each time slot.
For instance, in the scenario where a car requires charging at 9:00 AM, having already achieved a full battery charge by 7:00 AM, and necessitating a power input of 10 kW. The MACD algorithm is utilized to compute the necessary power for a trip, which is then removed from the overall state of charge (SOC) in the battery. This power is subsequently discharged when the vehicle remains stationary or until the desired SOC level is achieved. An further illustration has a vehicle that requires a power output of 20 kW at 5:00 PM, with its State of Charge (SOC) recorded at 40% as of 2:00 PM. The algorithm computes the requisite power for completing the journey, and in cases where the state of charge (SOC) falls short, the vehicle initiates the charging process until the SOC reaches an adequate level for the trip or until the trip time approaches.
The MACD algorithm prioritizes three key factors while utilizing an EV battery to mitigate peak power demands: owner comfort, battery health, and EV performance. Hence, the MACD algorithm employs a collection of mathematical equations to ensure the attainment of these objectives. This section will proceed to present and afterwards discuss the aforementioned relations. Typically, the algorithm will compute the State of Charge (SOC) for every Electric Vehicle (EV). At each time slot, each EV can be charged and discharged by the amount of power referred to as charge step
Regarding the evolution of SOC over time, it is given by the following expression:
Where
The initial phase of this thesis is the selection of a power grid as an illustrative case study. The power grid is interconnected with an electric vehicle (EV) aggregator, which in turn is linked to three other EV aggregators located in various dispatch regions, namely agent’s houses, company buildings, and an industrial park. For the sake of simplicity, let us assume that there are 10, 20, and 30 electric vehicles (EVs) in the dispatch regions. It is also assumed that all of these EVs share the same specifications. The charging power of any electric vehicle (EV) is identical at its highest capacity. Throughout the simulation, many parameters have been employed to represent the electric vehicles (EVs). These characteristics are utilized to demonstrate the outcomes and establish the credibility of the proposed algorithms. The specification of simulation parameters and their corresponding values has significant importance for individuals seeking to review the thesis and make comparisons between their own work and our findings. Some parameters, such as
3 Results and discussions
This study undertakes two case studies. The initial case study examines the integrated scheduling of electric vehicle (EV) aggregators, wherein the charging costs for both coordinated charging and discharging, as determined by an algorithm, and uncoordinated charging and discharging, are computed. In the second scenario, an analysis is conducted to compare the charging demand and the corresponding reaction. In order to enhance the validity of the study, a selection of 10, 20, and 30 electric vehicles (EVs) were picked at the charging station.
3.1 First case study: combined EV aggregator profile—Simulation
In this instance, the authors utilize the profiles of the Electric Reliability Council of Texas (ER-COT) market and the Pacific Gas and Electric Company (PG&E) market price of Electricity Company for electric vehicle (EV) consumers in the residential, commercial, and industrial sectors. This profile aims to do a comparison analysis of electricity prices between uncoordinated charging at 40 A–240 V and coordinated charging, also known as “Smart charging”. Figure 5 illustrates a cost comparison between coordinated and uncoordinated charging for a combined aggregator profile. According to the suggested algorithm, the overall cost of power over a 24-h period is $152.71, representing a 14.7% reduction compared to the cost of uncoordinated charging, which amounts to $179.6347.
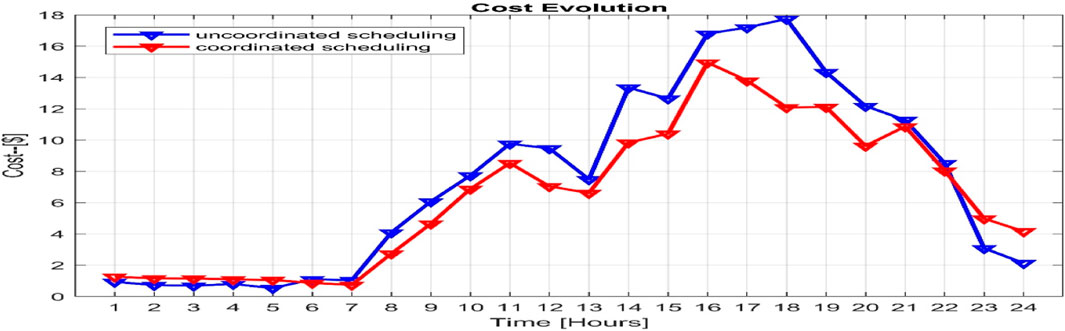
FIGURE 5. Cost Comparison between Coordinated & Uncoordinated charging (Combined EV Aggregator profile).
3.1.1 Uncoordinated charging
The charging process for the uncoordinated vehicles occurs instantaneously upon being plugged in, with the objective of fully recharging the batteries as quickly as possible, without taking into account the daily electricity price. Whenever a car arrives at a charging station, the battery initiates the charging process without taking into account the cost of electricity. Figure 6 illustrates the state of charge (SOC) of electric vehicles (EVs) within a combined EV aggregator profile. Consequently, there will be an increase in the overall expenditure on electricity, accompanied by a surge in demand for electric vehicle charging during periods of peak hours.
3.1.2 Co-ordinated charging (applied algorithm)
The concept of coordinated charging aims to optimize the charging process in order to minimize associated costs. The attainment of an ideal control strategy will be realized by implementing bidirectional power flow between the electrical grid and the electric vehicle (EV) battery. There exists a prevailing notion that the charging of electric vehicles is typically carried out during periods of electricity price reduction. The coordinated charging cost is represented by the red line in Figure 5, while the uncoordinated charging cost is depicted by the blue curve. The algorithm applied results in a relatively lower coordinated charging cost.
The cost comparison between coordinated and uncoordinated charging of electric vehicles (EVs) for the combined aggregator, as depicted in Figure 5, reveals the analysis conducted on the cost implications. The study focuses on the aggregator’s connection to EV users from households, business establishments, and industrial sectors, with data obtained from 10, 20, and 30 EVs, respectively. The peak timeframe for each drug varies. The charging power exhibits a gradual increase starting at approximately 08:00 in the morning. The application of the proposed algorithm allows for a clear observation that, in the case of a coordinated charging profile during peak charging demand, the maximum charging cost amounts to $16. Conversely, in the scenario of an uncoordinated charging profile, the charging cost during peak charging demand is approximately $13.8, which is comparatively higher than that of the coordinated scheduling profile.
The uncontrolled charging and discharging of electric vehicles can be shown in Figure 6. The uncoordinated charging profile entails the charging and discharging of electric vehicles (EVs) anytime they are connected to a power source, regardless of the cost of electricity or the need for unplugging to facilitate travel. It is evident that the majority of charging activities take place during peak hours, leading to a significant increase in charging expenses. The demand for industrial electric vehicle (EV) agents experiences a significant surge between the hours of 08:00 and 19:00. As depicted in Figure 5, the uncoordinated charging profile fails to account for the disparity between peak and off-peak power consumption, potentially resulting in an insufficiency of electricity supply. The positive values on the curve represent the charging process of electric vehicles (EVs), while the negative values indicate the discharging of EVs. The flat curve demonstrates the absence of any charging or discharging processes.
The coordinated charging and discharging of electric vehicles can be observed in Figure 7. In the context of coordinated charging profiles, electric vehicles (EVs) undergo charging and discharging operations as required, taking into account the specific charging demands of each car. It is evident that the majority of charging activities take place during peak hours, leading to a substantial increase in charging expenses. Therefore, by implementing the proposed algorithm and coordinated charging approach, it is possible to mitigate the charging demand during peak hours. The peak demand of the system exhibits a surge from 8:00 to 22:00, encompassing all electric vehicle users within the region. The coordinated charging profile, as depicted in Figure 7, takes into account the disparity between peak and off-peak power demand. This consideration has the potential to result in reduced charging costs as compared to uncoordinated charging. The positive portion of the curve represents the charging process of electric vehicles (EVs), while the negative portion represents the discharging process. The flat portion of the curve indicates the absence of both charging and discharging activities.
3.2 First case study: EV aggregator
Figure 8 illustrates the charging demand, both prior to and subsequent to the implementation of demand response (DR), exhibiting a gradual rate of charging. The graph illustrates the practice of load shifting, wherein the charging load is strategically redistributed from peak to valley hours. This approach aims to limit the charging load during periods of high charging demand, hence reducing the overall power cost for the Combined EV aggregator. Figure 8 displays the projected charging demand for the area control encompassing residential, commercial, and industrial electric vehicle (EV) agents. The blue line represents this forecasted demand, while the red line represents the optimal EV charging strategy. This strategy involves shifting the load from peak to valley hours, thereby minimizing the charging load during periods of high demand and reducing the overall power cost for the entire region.
The charging demand for area load control comprises three distinct electric vehicle (EV) agents, including households, business buildings, and industrial parks. The total peak demand from all regions indicates that the area load control is projected to be between 84.5, 83, 78, and 80 over the time period of 09:00 to 13:00, as depicted in Figure 8 by the blue curve. In contrast, the optimal charging demand for this period, represented by the red curve, is relatively lower. Figure 8 displays the projected charging demand for the area load control region, represented by the blue curve. Conversely, the red curve illustrates the optimal electric vehicle (EV) charging strategy, wherein a portion of the EV load is shifted from peak hours (09:00 to 12:00) to off-peak hours. This strategy aims to minimize the charging load during peak charging demand, as well as reduce the overall power cost for all agents involved. A strategic adjustment has been made to redistribute a portion of the charging demand to off-peak hours, characterized by lower electricity prices.
4 Conclusion
The MACD algorithm is proposed in this paper to optimally manage electric vehicle (EV) charging and discharging in a smart grid setting. The algorithm optimizes the combined aggregator profile by considering the charging demand of households, businesses, and an industrial park EV aggregator. The algorithm also analyzes grid electricity costs. The algorithm maintains the maximum state of charge (SOC) of electric cars (EVs) before they leave the charging station by following each vehicle owner’s constraints. The algorithm effectively redistributes the charging demand of individual electric vehicle (EV) agents from high to low demand and takes their charging requirements into consideration during charging process coordination. This method helps EV owners manage electricity costs and stabilizes the system during peak hours. The methodology under consideration reduces power charging prices by 15% for consumers, 14.6% for business buildings, and 14.5% for the industrial park EV aggregator.
The integration of electric vehicle (EV) loads from numerous agents and fair communication routes between EV agents and a unified power grid aggregator make this project feasible. Electric vehicles (EVs) may be inappropriate for future transportation because to uncoordinated discharge procedures that damage battery health. The simulation results show that coordinated charging is cheaper than uncoordinated charging. The MACD algorithm orchestrates charging and discharging processes to reduce peak charging demand by using surplus power in electric vehicle (EV) batteries to meet the collective EV aggregator’s charging needs.
Data availability statement
The original contributions presented in the study are included in the article/Supplementary Material, further inquiries can be directed to the corresponding author.
Author contributions
MB: Formal Analysis, Funding acquisition, Writing–review and editing. MH: Conceptualization, Investigation, Writing–original draft, Writing–review and editing. Sheharyar: Supervision, Writing–review and editing. AA: Formal Analysis, Writing–review and editing. GA: Conceptualization, Formal Analysis, Writing–original draft, Writing–review and editing. AK: Data curation, Software, Supervision, Writing–review and editing. ET: Validation, Writing–review and editing. AY: Project administration, Supervision, Validation, Visualization, Writing–original draft. SM: Software, Visualization, Writing–review and editing. AA: Validation, Writing–review and editing.
Acknowledgments
The author(s) disclosed receipt of the following financial support for the research, authorship, and/or publication of this article: This work was funded by the Deanship of Scientific Research, King Faisal University Project No. “GRANT4997”.
Conflict of interest
The authors declare that the research was conducted in the absence of any commercial or financial relationships that could be construed as a potential conflict of interest.
Publisher’s note
All claims expressed in this article are solely those of the authors and do not necessarily represent those of their affiliated organizations, or those of the publisher, the editors and the reviewers. Any product that may be evaluated in this article, or claim that may be made by its manufacturer, is not guaranteed or endorsed by the publisher.
References
Adapa, R. S., Khaitan, S. K., and Albadi, M. H. (2018). Optimal scheduling of electric vehicle aggregators in power distribution networks. IEEE Trans. Sustain. Energy 9 (2), 703–713.
Ahmed, M. A., Abbas, G., Jumani, T. A., Rashid, N., Bhutto, A. A., and Eldin, S. M. (2023). Techno-economic optimal planning of an industrial microgrid considering integrated energy resources. Front. Energy Res. 11, 1–12. doi:10.3389/fenrg.2023.1145888
Ali, A., Abbas, G., Keerio, M. U., Koondhar, M. A., Chandni, K., and Mirsaeidi, S. (2023a). Solution of constrained mixed-integer multi-objective optimal power flow problem considering the hybrid multi-objective evolutionary algorithm. IET Gener. Transm. Distrib. 17 (1), 66–90. doi:10.1049/gtd2.12664
Ali, A., Abbas, G., Keerio, M. U., Mirsaeidi, S., Alshahr, S., and Alshahir, A. (2023b). Pareto front-based multiobjective optimization of distributed generation considering the effect of voltage-dependent nonlinear load models. IEEE Access 11 (February), 12195–12217. doi:10.1109/ACCESS.2023.3242546
Alizadeh-Mousavi, O., and Rabiee, A. (2015). Optimal distributed scheduling of electric vehicle charging under high penetration of renewables. IEEE Trans. Power Syst. 30 (6), 3157–3167.
Barbour, E., Zhang, P., and Haben, S. (2017). Optimal scheduling for electric vehicle aggregators with distribution network constraints. IEEE Trans. Industrial Inf. 13 (2), 766–774.
Chen, M., Wu, L., and Shahidehpour, M. (2013). Stochastic unit commitment with vehicle-to-grid participation. IEEE Trans. Smart Grid 4 (1), 352–361.
Ehsan, M., and Yousefi, G. R. (2016). Optimal scheduling of electric vehicle charging in smart grids considering demand response. IEEE Trans. Power Syst. 31 (5), 3723–3732.
Farag, H., and Cheng, S. J. (2016). Optimal charging and discharging of electric vehicles in smart microgrids. IEEE Trans. Industrial Inf. 12 (5), 1802–1810.
Gao, B., and Wei, J. (2017). Optimal scheduling of electric vehicle charging and discharging considering renewable energy penetration. IEEE Trans. Industrial Inf. 13 (3), 1217–1226.
Habib, S., Abbas, G., Jumani, T. A., Bhutto, A. A., Mirsaeidi, S., and Ahmed, E. M. (2022). Improved whale optimization algorithm for transient response, robustness, and stability enhancement of an automatic voltage regulator system. Energies 15 (14), 5037. doi:10.3390/en15145037
Li, B., and Shahidehpour, M. (2016). Optimal scheduling of electric vehicle charging under uncertainty. IEEE Trans. Power Syst. 31 (6), 4753–4761.
Li, F., Wei, J., and Zhang, Y. (2018). Joint optimization of electric vehicle scheduling and renewable energy generation in distribution grids. IEEE Trans. Industrial Inf. 14 (4), 1532–1540.
Liu, C., and Wang, J. (2016). Stochastic optimal scheduling of electric vehicle charging and discharging considering renewable energy penetration. IEEE Trans. Sustain. Energy 7 (2), 552–562.
Ma, H., Shahidehpour, M., and Li, Z. (2017). Optimal dispatch of electric vehicles and vehicle-to-grid considerations. IEEE Trans. Power Syst. 32 (3), 2061–2072.
Nazaripouya, H., and Oo, A. M. T. (2017). Stochastic model predictive control for optimal PEV charging scheduling in a smart grid with renewable energy resources. IEEE Trans. Industrial Inf. 13 (5), 2415–2423.
Wang, Y., Zhang, Y., and Liu, X. (2013). A distributed optimization algorithm for electric vehicle charging management in smart grids. IEEE Trans. Industrial Electron. 60 (4), 1596–1605.
Zeng, P., and Wu, Q. (2017). Optimal scheduling of electric vehicle charging and discharging with vehicle-to-grid capability. IET Generation, Transm. Distribution 11 (13), 3259–3266.
Zhang, P., Barbour, E., and Dong, Z. Y. (2017b). Joint optimization of PEV charging and grid operations for energy and ancillary services. IEEE Trans. Smart Grid 8 (5), 2146–2157.
Zhang, P., Barbour, E., and Huang, S. (2015). Optimal charging and discharging scheduling of electric vehicles in vehicle-to-grid. IEEE Trans. Industrial Inf. 11 (2), 484–493.
Keywords: combined aggregator, demand response, electricity market, vehicle-to-grid (V2G), EVs (electric vehicles)
Citation: Bouzguenda M, Hatatah M, Sheharyar , Ali A, Abbas G, Khan A, Touti E, Yousef A, Mirsaeidi S and Alshahir A (2023) An optimal dispatch schedule of EVs considering demand response using improved MACD algorithm. Front. Energy Res. 11:1295476. doi: 10.3389/fenrg.2023.1295476
Received: 16 September 2023; Accepted: 02 November 2023;
Published: 20 November 2023.
Edited by:
Maria Grazia De Giorgi, University of Salento, ItalyReviewed by:
Kiran Chandni, Shaheed Benazir Bhutto University Shaheed Benazirabad, PakistanKhaled Kaaniche, Jouf University, Saudi Arabia
Copyright © 2023 Bouzguenda, Hatatah, Sheharyar, Ali, Abbas, Khan, Touti, Yousef, Mirsaeidi and Alshahir. This is an open-access article distributed under the terms of the Creative Commons Attribution License (CC BY). The use, distribution or reproduction in other forums is permitted, provided the original author(s) and the copyright owner(s) are credited and that the original publication in this journal is cited, in accordance with accepted academic practice. No use, distribution or reproduction is permitted which does not comply with these terms.
*Correspondence: Mounir Bouzguenda, bWJ1emdhbmRhQGtmdS5lZHUuc2E=