- 1State Grid Hebei Electric Power Co., Ltd., Shijiazhuang, Hebei, China
- 2State Grid Hebei Economics Research Institute, Shijiazhuang, Hebei, China
- 3North China Electric Power University National Institute of Energy Development Strategy, Beijing, China
The worsening of climate conditions is closely related to the large amount of carbon dioxide produced by human use of fossil fuels. Under the guidance of the goal of “carbon peaking and carbon neutrality goals”, with the deepening of the structural reform of the energy supply side, the hybrid energy system coupled with renewable energy has become an important means to solve the energy problem. This paper focuses on the comprehensive evaluation of hybrid energy systems. A complete decision support system is constructed in this study. The system primarily consists of four components: 1) Twelve evaluation criteria from economic, environmental, technological, and socio-political perspectives; 2) A decision information collecting and processing method in uncertain environment combining triangular fuzzy numbers and hesitation fuzzy language term sets; 3) A comprehensive weighting method based on Lagrange optimization theory; 4) Solution ranking based on the fuzzy VIKOR method that considers the risk preferences of decision-makers. Through a case study, it was found that the four most important criteria are investment cost, comprehensive energy efficiency, dynamic payback period and energy supply reliability with weights of 7.21%, 7.17%, 7.17%, and 7.15% respectively. A1 is the scheme with the best comprehensive benefit. The selection of solutions may vary depending on the decision-maker’s risk preference. Through the aforementioned research, the decision framework enables the evaluation of the overall performance of the system and provides decision-making references for decision-makers in selecting solutions.
1 Introduction
1.1 Background and motivation
Energy is the cornerstone of human survival and development. Faced with multiple challenges such as resource shortage, environmental damage and climate change, traditional energy production and supply modes cannot meet the needs of social development (Zhang et al., 2023). As the world’s largest carbon emitter, China’s main source of carbon dioxide emissions is the burning of fossil fuels, accounting for 88% (Zeng et al., 2023). Therefore, it is urgent to carry out clean and efficient reform of China’s energy supply system and consumption structure.
New energy sources such as wind and solar power, due to their abundant resources and zero emissions, will play a supporting role in the entire transition process (Niu et al., 2022). However, the mismatch between the output characteristics and the load of renewable energy, resulting in low actual utilization, still hinders its large-scale distribution (Liu et al., 2019; Liu et al., 2022a; Yong et al., 2022). With the continuous development of energy management, energy monitoring, energy storage (ES), and distributed generation technologies, the hybrid energy system (HES) that incorporates renewable energy generation is regarded as a crucial solution to address future energy challenges (Ke et al., 2022). HES achieves an organic coordination and optimization of energy production, transmission, distribution, conversion, storage, and consumption across multiple time scales, enabling an integrated supply of energy production and consumption (Liu et al., 2022b), as shown in Figure 1.
However, the layout and promotion of HES are still in the early stage, with limited demonstration projects. As an energy project, the lack of a comprehensive evaluation system during the investment decision-making stage is a significant obstacle to the development of HES. Decision-makers (DMs) need a comprehensive understanding of the project to be motivated to invest in its construction. Therefore, in order to address this issue and promote the sustainable development of HES, this study establishes a comprehensive evaluation framework for HES.
1.2 Literature review
HES, consisting of renewable and fossil energy sources, is an important approach to addressing energy supply issues (Li et al., 2018). As a result, researchers have conducted extensive studies on HES. Devrim and Bilir, (2016) investigated a system that integrates wind turbines, photovoltaic panels, and fuel cells to meet the electricity demand of residential buildings. Zhou et al. (2019) studied the performance of the entire system after incorporating wind and solar power generation into the integrated energy system. Sezer et al. (2019) proposed a multi-output system that stores and converts concentrated solar, wind, and hydrogen energy. Ruiming (2019) optimized a hydrogen-integrated energy system, including wind turbines, photovoltaics, electrolyzers, and fuel cells. Eriksson and Gray, (2017) provided a detailed review of energy systems that couple renewable energy generation, hydrogen storage, and fuel cells, conducting a comprehensive comparative analysis and outlook while maintaining a positive outlook on the industry’s development. Building on this foundation, Zhang et al. (2022a) proposed that the capacity configuration optimization of a HES is the basis for system development, with the goal of increasing system economics. Liu et al. (2022a) studied the optimal size of HES considering economic, environmental, and thermal comfort benefits and solved the model using NSGA-II. The aforementioned studies primarily focus on the structural characteristics and capacity configuration optimization of HES, revealing that HES with integrated renewable energy sources has a solid theoretical and practical foundation and provides significant environmental and social benefits.
Conducting a comprehensive evaluation of HES is important both for assessing the overall performance of the system and providing decision-making guidance for selecting appropriate solutions. Current research on the comprehensive evaluation of HES mainly includes the establishment of evaluation indicator systems, determination of indicator weights, and ranking of alternative solutions. Zhou et al. (2020) constructed performance analysis indicators from five aspects: energy utilization, economy, environment, technology, and society, to optimize decision-making for integrated energy systems coupling renewable energy generation. Yang et al. (2018) considered economic, technical, social, and environmental analysis indicators to comprehensively evaluate planning schemes for distributed energy systems. Ke et al. (2022) conducted a comprehensive evaluation of HES using nine indicators in four aspects: economic, energy utilization, environmental impact, and social acceptance. Building on this, Zhang et al. (2021) considered the comprehensive grid loss rate to analyze the overall benefits of HES driven by wind and solar energy, and conducted case studies. It is evident that the comprehensive evaluation of HES needs to consider multiple aspects, constituting a multi-criteria decision-making (MCDM) problem. The determination of indicator weights is an important step in solving MCDM and can be approached through subjective weight methods, objective weight methods, and integrated weight methods (Wu et al., 2016; Wu et al., 2018; Qian et al., 2021; Zhang et al., 2021; Yong et al., 2022). Subjective weight methods reflect the subjective preferences of decision-makers (Wu et al., 2023a), while objective weight methods focus on the intrinsic relationships among data. Integrated weight methods combine the two through certain mathematical methods to achieve a balance between subjectivity and objectivity (Zhang et al., 2022b). Yong et al. (2022) employed a combination of Step-wise Weight Assessment Ratio Analysis (SWARA) and entropy method using Lagrange optimization, achieving effective weight optimization solutions that might provide insights for this paper. As for the ranking of alternative solutions, commonly used methods include Analytic Hierarchy Process (AHP), Analytic Network Process (ANP), Technique for Order Preference by Similarity to an Ideal Solution (TOPSIS), among others. However, many of these methods do not adequately account for DMs` bounded rationality. The VIse Kriterijumski Optimizacioni Racun (VIKOR) method is capable of effectively addressing the aforementioned issues. Kamali Saraji et al. (2023) utilized the VIKOR method to rank eight challenges related to the adoption of renewable energy in rural areas. Abdul et al. (2022) employed the VIKOR method to prioritize the selection of solar energy, wind energy, hydropower, and biomass energy in developing countries. These studies demonstrate the mature application of the VIKOR method in the energy sector. Moreover, due to its ability to reflect decision-makers’ subjective preferences, this paper intends to use the VIKOR method to rank alternative scenarios for HES. However, the traditional VIKOR method may not fully meet the practical decision-making requirements, prompting further improvements in this study.
Through the summary and analysis of related literature, the critical findings are as follows:
(1) Existing comprehensive evaluation studies mostly focus on HES that provide combined heat, power, and cooling, and there is a lack of research that incorporate renewable energy for hydrogen production.
(2) The existing comprehensive evaluation indicators for HES commonly suffer from deficiencies such as the lack of rational selection of indicators and difficulties in quantifying them.
(3) Current comprehensive evaluation research on HES lacks considerations for collecting complete decision-making information and addressing information loss during processing.
1.3 Objectives and contributions
The above literatures provide significant inspiration for this study, but it also highlights certain deficiencies in current research. Therefore, the main objectives of this paper are to address the existing gaps in research and construct a rational and comprehensive framework for the comprehensive evaluation of HES, providing DMs with solid theoretical and methodological support. The main contributions of this paper are as follows:
(1) This paper addresses the comprehensive evaluation of HES that incorporate renewable energy for hydrogen production, expanding the research in this field.
(2) This paper establishes a complete and operational decision support system for decision-makers. The HES comprehensive evaluation decision support system consists of three parts: evaluation indicators, indicator weight determination, and alternative solution ranking. DMs can directly apply this model to conduct comprehensive evaluations of various HES. Additionally, each part of the decision support system takes into account the subjective preferences of DMs.
(3) This paper thoroughly considers and resolves the issues of fuzziness and randomness in the decision-making environment. Extended fuzzy logic is employed for collecting and processing decision-making information in order to maximize information gathering and minimize losses.
The remainder of this paper is organized as follows: Section 2 establishes a comprehensive evaluation index system of HES; Section 3 utilizes a series of methods to construct the HES comprehensive evaluation model; Section 4 uses a park in Gansu Province to carry out empirical analysis; Section 5 analyzes and discusses the calculation results, including sensitivity analysis and comparative analysis; Section 6 gives the conclusion and outlook.
2 Evaluation criteria system for HES
The indicator system serves as an important foundation for conducting comprehensive evaluations of HES. A good indicator system should encompass comprehensiveness, rationality, and innovation. Therefore, this paper will first review the indicator systems used in relevant studies to explore the common indicators for HES comprehensive evaluation, as shown in Table 1. Secondly, since the experts are distributed in different regions, Delphi technology is used to collect the decision-making information of the experts. The information in Table 1 and the architecture of HES were sent to a number of experts. Experts analyze and select the comprehensive evaluation indicators. We aggregated the reports of each expert to form a preliminary indicator system, which is then distributed to the experts for analysis. So repeated, and eventually formed a generally recognized indicator system. Finally, based on this, innovative indicators applicable to HES systems including hydrogen production processes will be proposed.
Based on the above analysis, this paper constructs a comprehensive benefit evaluation indicator system for HES from four aspects: economy, environment, technology, and socio-policy.
2.1 Economic criteria
The economic indicators of evaluation index system are as follows:
Investment cost (C11): Investment cost refers to all expenses incurred in the initial stage of HES construction. It determines to a certain extent the difficulty of system construction and economic benefits. Since labor costs can be neglected compared to equipment procurement costs, the initial investment can be simplified as the cost of equipment procurement during the construction period. C11 is a cost criterion.
where
Dynamic payback period (C12): The dynamic payback period refers to the time required for a project’s net returns to offset the total investment, taking into account the time value of money. This metric examines the ability of the project to recover its investment and is related to investment risk (Li et al., 2022a). C12 is a cost criterion.
Operation and maintenance cost (C13): The operation and maintenance costs of HES consist of two parts. Firstly, there are the costs incurred from purchasing energy from external sources, which mainly include the gas consumption of the CCHP unit and the purchased electricity from the external grid when renewable energy generation is insufficient. The second part includes the management expenses and labor costs associated with operating the equipment (Ke et al., 2022). C13 is a cost criterion.
where
Hydrogen yield rate (C14): The ratio between the economic benefits obtained from the hydrogen production process and the input costs. This ratio can be used to evaluate the economic feasibility and profitability of the electrolytic hydrogen production equipment (Liang and Wang, 2023). C14 is a beneficial criterion.
where
2.2 Environmental criteria
The environmental indicators of evaluation index system are as follows:
Carbon dioxide emissions (C21): This indicator refers to the annual total carbon dioxide emissions from HES (Qin et al., 2021). C21 is a cost criterion.
where
Air pollutant emissions (C22): This indicator refers to the annual total emissions of SO2, NOx, and particulate matter generated by HES each year. C22 is a cost criterion.
where
Land occupation (C23): The construction of HES will require land, which will have a certain impact on natural scenery and urban planning (Wen et al., 2021). C23 is a cost criterion.
Noise (C24): Due to the presence of various energy supply equipment in HES, there will be some noise generated during operation. The noise can cause disruptions to the normal lives of workers and nearby residents and, in the long run, can have significant health impacts on the human body (Qian et al., 2021). C24 is a cost criterion.
2.3 Technical criteria
The technical indicators of evaluation index system are as follows:
Comprehensive energy efficiency (C31): The comprehensive energy utilization rate reflects the degree of coupling and complementary utilization of multiple energy flows at different time scales, and can be used to measure the level of comprehensive energy utilization in a system (Zheng and Wang, 2020). C31 is a beneficial criterion.
where
Energy supply reliability (C32): The ES in the park and their connection to the external power grid significantly reduce the impact of the intermittency of renewable energy and power equipment failures on the system’s reliability. Therefore, this study considers reflecting the system’s reliability by evaluating the energy supply-demand imbalance within the park when the system is operating in island mode (Ke et al., 2022). C32 is a beneficial criterion.
where
Device utilization rate (C33): The equipment utilization rate represents the ratio of the actual output power of the energy generation devices installed in the park to their rated power, reflecting the efficiency of the equipment’s production. C33 is a beneficial criterion.
where
ES equivalent utilization coefficient (C34):The ES equivalent utilization coefficient represents the utilization rate of the ES in HES, reflecting the significance of ES and the rationality of capacity allocation. C34 is a beneficial criterion.
where
2.4 Social-political criteria
The social-political criteria indicators of evaluation index system are as follows:
Level of advancement (C41): The level of advancement refers to the level of advancement of the HES compared to similar projects domestically and internationally. It influences the extent of policy and financial support that the project can receive after construction and implementation. This indicator is related to the technological advancement, innovative mode, and scalability of the project. C41 is a beneficial criterion.
Public satisfaction (C42): Public satisfaction is mainly related to two aspects: firstly, the public’s acceptance of the project’s construction, which is related to the engineering implementation plan and operational mechanisms; secondly, the users' intuitive experience with the HES. This indicator has a significant impact on the promotion and later operation of the project. C42 is a beneficial criterion.
Job creation (C43): The construction and operation of HES will stimulate local employment and the development of the service industry. The research and development, as well as the manufacturing of related equipment, will promote the employment of engineering and technical personnel (Qian et al., 2021). C43 is a beneficial criterion.
Compatibility with policies (C44): As a new type of energy utilization model, most HES are still in the planning and initial construction phase. Therefore, adopting system solutions that are more compatible with national policies is more conducive to obtaining financial support from the government. This aspect plays a significant role in determining whether the project can obtain feasibility approvals. C44 is a beneficial criterion.
3 Materials and methods
3.1 Methods of collecting decision-making information
The collection and processing of decision information are among the most crucial issues in the field of MCDM. In the investment decision-making process for the HES, there will be a significant amount of uncertainty due to its novelty and cutting-edge nature. Uncertainty can introduce ambiguity and randomness into the decision environment, making it challenging for DMs to assess the HES. Therefore, it is essential to address the significant issue of how to gather decision information that reflects the most authentic thoughts of DMs. Additionally, the process of handling decision information should minimize information loss as much as possible to ensure the rationality of evaluation results.
3.1.1 Hesitant fuzzy linguistic term set
In a fuzzy environment, the decision-making process often brings significant hesitation to DMs. Especially when evaluating qualitative indicators, DMs does not necessarily have an in-depth study of all aspects of HES. They may hesitate between adjacent measurement levels, unable to provide precise and singular decision information. Hesitant fuzzy linguistic term set (HFLTS) can obtain expert subjective evaluation information more flexibly, thereby maximizing the integrity of decision information (Yong et al., 2023).
The definitions related to HFLTS are as follows:
Definition 1:. A linguistic term set
Definition 2:. HFLTS allows DMs to evaluate the HES by selecting one or multiple linguistic variables
Definition 3:. The conversion relationship between the evaluation information provided by DMs based on the linguistic term set and HFLTS is as follows:
(1)
(2)
(3)
(4)
Based on the above definition, experts can give evaluation terms that look like the following expression:
3.1.2 Triangular fuzzy number
The expert evaluation information can be collected more comprehensively using HFLTS. However, this information is currently in qualitative form and cannot be directly analyzed and computed quantitatively. Triangular fuzzy numbers (TFNs) are widely used to transform qualitative information into quantitative information due to their ability to preserve fuzzy information and their advantages of simplicity and ease of operation. The main definitions and formulas involved in TFNs are as follows:
Definition 4:. When an information set
By performing the defuzzification operation on TFNs, their crisp values can be obtained:
The specific representation of TFNs in this paper is the quantitative characterization of linguistic terms in set
Based on the theoretical analysis above, further processing can be performed on the decision information represented by
Definition 5:. In the optimization of the HES, it involves comparing different evaluation values. Therefore, the distance formula between two TFNs
3.2 Methods of calculating criteria weights
The evaluation results of the HES are determined by a combination of multiple indicators. However, the contributions of different indicators may vary, which is reflected in the weights assigned to the indicators. Therefore, this section will discuss the methods for determining the indicator weights. Additionally, to account for both the subjectivity of the DM and the objectivity of the indicator values, this paper adopts a comprehensive weighting method that combines subjective and objective aspects to calculate the relative importance of each indicator.
3.2.1 SWARA method–Subjective weights
HES is a novel mode of energy production and utilization; Thus, the proper subjectivity of DMs is important to ensure the rationality of the evaluation results. The SWARA method, which effectively reflects the DMs’ viewpoints and balances operability and scientific rigor, has been widely used for determining the subjective weights of indicators (Ghenai et al., 2020).
The main steps of SWARA are shown as below:
Step 1:. According to the DM’s preferences, the indicators are ranked in descending order of importance. Additionally, the relative importance between the top-ranked indicator and the remaining indicators is evaluated. The evaluation language and the corresponding quantitative values are presented in Table 2.
Step 2:. (Akhanova et al., 2020): Starting with the second attribute, calculate the relative importance between the criterion (marked
Step 3:. The coefficient value
Step 4:. Calculate the correction weight value
Step 5:. Compute the subjective weights
3.2.2 Entropy weights method–Objective weights
The evaluation indicator system for the HES includes a large number of quantitative indicators. When determining the weights of these indicators, ignoring the influence of numerical values can result in a lack of objectivity in the decision-making process. Therefore, this paper adopts the entropy method to determine the objective weights of the indicators. The main steps of the entropy method are as follows (Wu et al., 2023a):
Definition 6:. To eliminate the influence of different properties of the indicators on the data dimensions and scale, it is necessary to perform a standardization operation on the TFNs. The standardization formula for
where
Step 1:. Construct the initial decision matrix as shown below:
where
Step 2:. Standardize the initial decision matrix through Definition 6. And calculate the mean value of criterion
Step 3:. The entropy measure
Step 4:. Calculate the objective weight of the
3.2.3 Lagrange optimization–Comprehensive weights
According to the principle of minimum discriminant information, the comprehensive weight should reflect the subjective and objective characteristic information as much as possible. Thus, Lagrange optimization is used to obtain the comprehensive weights (Huang et al., 2021).
where
(1)
(2)
Then
3.3 Method of sorting the alternatives
After obtaining the weight information of the indicators, integrating it effectively with expert evaluation language becomes a crucial step in the selection of the optimal solution for the HES. The VIKOR method is a compromise-based MCDM method that ranks alternative solutions by comparing their proximity to the positive and negative ideal solutions (Meniz and Ozkan, 2023). The VIKOR method can fully consider the DMs' subjective preferences for the HES and balance the trade-offs between the benefits and harms of each solution. However, the effectiveness of the VIKOR method can be greatly influenced by uncertain environments. Therefore, this paper improves the VIKOR method using TFNs to enhance its applicability in such environments. The main steps of the fuzzy VIKOR method are as follows.
Step 1:. Based on the normalized initial evaluation matrix obtained from the fuzzy entropy method, determine the best
Step 2:. Compute the social utility value
Step 3:. Compute the collective benefit coefficient
where
Step 4:. Sort the solutions in ascending order based on their values of
Step 5:. To determine the compromise solution, the alternative solution
Condition 1:.
Condition 2:. Acceptable Stability: If, based on the ranking according to
If either of the two conditions mentioned above is not satisfied, a set of compromise solutions is obtained:
(1) If only Condition 2 is not satisfied, both
(2) If Condition 1 is not satisfied, the maximum value of
3.4 Decision-making framework
The decision framework of this paper is shown in Figure 2.
4 Case study
4.1 Case background
Gansu Province is an important base for new energy in China, ranking among the top in wind power generation and photovoltaic power generation. Therefore, this article selects an industrial park in Lanzhou, Gansu Province as the service object of HES for a case study. The industrial park is located in the northwest of Lanzhou City and has abundant wind and solar resources, making it suitable for the development of renewable energy generation. The area of the park available for solar energy is 17,500 square meters, with a solar irradiation intensity of 1,300 (kW·h)/m2. The average wind speed is 5.5 m/s. The electricity load in the park is 3.75 MW, with separate loads for heating (2.1 MW) and cooling (2.8 MW), and a gas load of 3.8 MW. The wind and solar resource data are obtained from the NASA, and the load data is provided by the local power company.
4.2 Comprehensive evaluation of the alternatives for HES
4.2.1 Data and decision information collection
Based on the network architecture of the HES shown in Figure 1, this paper has formulated six different schemes in Table 3 to meet the energy demands of the industrial park. Among them, A1, A2, and A3 compare the advantages and disadvantages of investing in photovoltaic and wind power in the park. A4 and A5 compare the advantages and disadvantages of electric boilers and gas boilers. A6 primarily utilizes CCHP units as the main heat source, coupled with small-scale gas boilers.
To maximize daily profits using the aforementioned six schemes, a four-season typical daily scheduling is conducted. Based on the scheduling results and the calculation methods of the three-level indicators in this paper, the quantitative data for the six alternative schemes in the comprehensive evaluation index system of HES are shown in Table 4.
The qualitative data for the six alternatives is sourced from an expert committee. The committee is composed of four experts who have long been engaged in research on integrated energy systems. The experts used HFLTS to evaluate the qualitative indicators of the alternative schemes. The evaluation results for the six alternative schemes are presented in Table 4.
4.2.2 Criteria weights calculation
4.2.2.1 Subjective weight calculation
In this paper, the TFNs-SWARA method is used to calculate the subjective weights of the indicators. The four experts evaluate the priority order of the various indicators based on their own expertise. The initial evaluation matrix by the experts is shown in Table 4.
Based on Table 5, the defuzzification operation is performed using Eq. 14. Afterwards, the subjective weights of the HES composite evaluation indicators can be obtained through Eqs 17–19.
4.2.2.2 Objective weights calculation
In this paper, the entropy method based on TFNs is used to calculate the objective weights of the indicators. Firstly, the information from Table 4 is integrated to form an initial decision matrix. Then, the qualitative decision information in the initial decision matrix is quantified using Eq. 15. Finally, the objective weights of the indicators can be obtained using Eqs 20–25, as shown in Table 6.
4.2.2.3 Comprehensive weights calculation
In order to incorporate both the subjective judgments of the experts and the inherent patterns of objective data, this paper integrates the results of two types of weights. In this process, it is important to minimize the loss of information. Therefore, the Lagrange optimization method is chosen in this paper. The integrated weights can be seen in Table 6.
It can be seen from the calculation result that economic index and technical index are the two most important first-level indexes. In the secondary index, C11 (Investment cost), C12 (Dynamic payback period), C31 (Comprehensive energy efficiency) and C32 (Energy supply reliability) are the most important criteria, which the DMs need to prioritize when making decisions.
4.2.3 Alternatives sorting
Once the weight calculation for the indicators is completed, this paper will conduct a comprehensive evaluation of the six alternative scenarios for the HES. Firstly, the normalized initial decision matrix obtained during the objective weight calculation process is used as the basis for the comprehensive evaluation. Secondly, the real values of the indicators are transformed by inversely utilizing the defuzzification formula to expand them into TFNs. For example, (0.75, 0.75, 0.75) = 0.75. Then, the best and worst indicator values are selected among all the standards, and the group utility value and individual regret value are calculated using Eqs 30, 31, as shown in Table 6. Finally, the group benefit coefficient is calculated using Eq. 32, as shown in Table 7. It is worth noting that the compromise coefficient
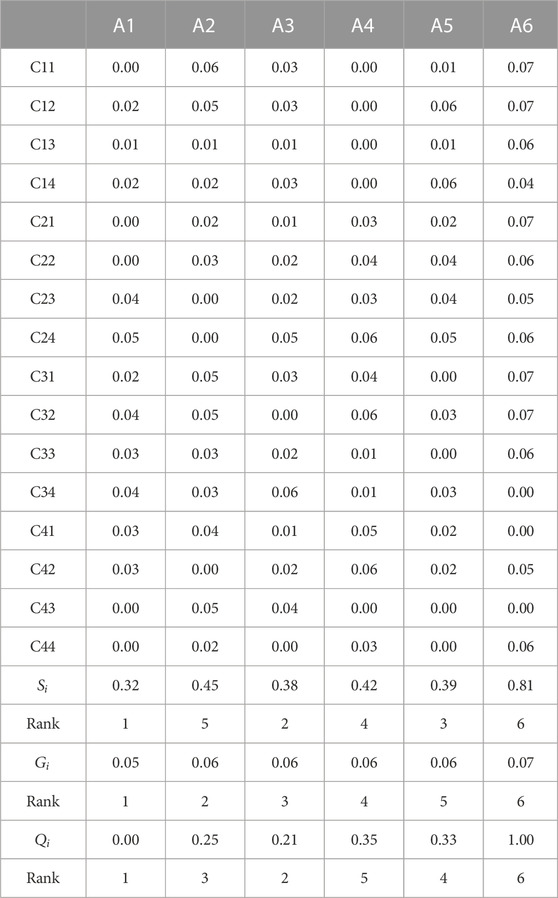
TABLE 7. Calculation results of group utility value, individual regret value, and collective benefit coefficient.
According to the compromise solution determination rules of the VIKOR, A1 is the optimal solution in
5 Discussion and analysis
In the previous chapter, this paper obtained the comprehensive evaluation results of HES, including index weight results and scheme ranking results. Therefore, the above results will be analyzed in this chapter. In addition, sensitivity analysis and comparative analysis will be employed to discuss the model. These two types of methods will respectively verify the robustness and rationality of the model.
5.1 Results analysis
5.1.1 Analysis of criteria weights results
The weight reweighting of HES comprehensive evaluation indicators is shown in Table 6. From the subjective weight of indicators, C11 (Investment cost), C31 (Comprehensive energy efficiency), C12 (Dynamic payback period) and C32 (Energy supply) reliability) has a high weight of 8.34%, 8.34%, 8.28%, and 7.95%, respectively. Among them, C11 and C12 are economic indicators, which mainly reflect the economic feasibility and investment risk of the program. C31 and C32 are technical indicators, which are the embodiment of system efficiency and supply assurance ability. From the objective weight of indicators, the weight of C24 (Noise) has a significant advantage over other indicators, which is 7.46%. The weights of the remaining indicators are between 6% and 6.5%. It indicates that there are some differences in technical scheme and comprehensive performance among the alternatives, but they are not very obvious. The obvious difference in the noise of each scheme is due to the greater noise of wind turbines. As a result, a scenario with more wind turbines would have a poorer C24 performance. From the comprehensive weight of indicators, the highest weights are C11, C31, C12 and C32, which are 7.21%, 7.17%, 7.17%, and 7.15% respectively. It is consistent with the trend of subjective weight, but the weights are reduced, which indicates that the comprehensive weight method can better reflect the subjective decision of experts and objective data information in the final weight.
5.1.2 Analysis of alternatives sorting
The fuzzy VIKOR method was used to rank HES alternatives, and the results are shown in Table 7. The final sorting result of the determined scheme is A1>A3>A2>A5>A4>A6. This result shows the relative advantages of each scheme considering group interests and individual regrets. However, when only the group benefits of each scheme are considered, the ranking results are A1>A3>A5>A4>A2>A6. This is because in the comprehensive evaluation, although the program may perform very poorly in one aspect, it will eventually be smoothed out by other aspects, resulting in large shortcomings in the implementation process of the project. VIKOR method takes this factor into account and reduces the impact of extreme results on comprehensive evaluation by introducing individual regret value. In all scenarios, A1 has the best performance in terms of group benefits and individual regrets. Therefore, A1 is the optimal solution.
5.2 Sensitivity analysis
5.2.1 Sensitivity analysis of criteria weights
The calculation of indicator weights is a crucial step in the comprehensive evaluation of HES, as it affects the final ranking results of alternatives. Therefore, this section will observe the trend of changes in the final evaluation results by altering the weights of indicators. Based on the calculated comprehensive weights obtained earlier, the weights of each indicator will be adjusted by ±10% and ±20% respectively. It should be noted that the sum of weights for all indicators remains unchanged.
The sensitivity analysis results of the four sub-indicators in the economy are shown in Figure 3. It can be observed that regardless of how the weights of the indicators change, A1 consistently remains the best-ranked option while A6 remains the worst. With an increase in the weight of C11, the ranking of A2 drops from third to fifth, indicating that A2 is sensitive to changes in the weight of C11. By examining the original indicator values for each scheme, it is evident that A2 has a significant disadvantage in C11 compared to other schemes. Conversely, DMs can prioritize A2 by lowering the weight of C11. Similarly, A5 is sensitive to changes in C12 and C14. Furthermore, the ranking results of the schemes do not undergo significant changes with variations in the weights of economic indicators.
The sensitivity analysis results of the environmental indicators are shown in Figure 4. A6 consistently remains the worst alternatives. A1 only drops to the second priority when the weight of C24 increases to 20%. When the weights of C12, C22, and C23 change, there is no change in the ranking results of all the schemes. By observing the sensitivity analysis results of C24 (Noise), it can be seen that as the weight of the indicator gradually increases, the ranking of A2 increases from third to first. This is due to the significant noise pollution generated by wind power compared to solar power, giving A2 a clear advantage over the other schemes in this indicator.
The sensitivity analysis results of the technical indicators are shown in Figure 5. The ranking results of A1 and A6 do not change with the variation of technical indicator weights. By observing the sensitivity analysis results of C32 and C34, it can be concluded that A2, A4, and A5 are sensitive to C32, while A3 is sensitive to C34. This indicates that these schemes have noticeable advantages or disadvantages compared to other schemes in these two indicators. Furthermore, the ranking results of all alternatives do not undergo significant changes with variations in the weights of the indicators.
The sensitivity analysis results of the social-political indicators are shown in Figure 6. The best and worst schemes among the six alternatives remain A1 and A6, respectively. By observing the sensitivity analysis results of all the indicators, it can be seen that A4 is sensitive to C41 and C42. A2 is sensitive to C43. Additionally, only a few schemes experience minor changes in their priority ranking.
By employing the sensitivity analysis method on the variation of indicator weights, it can be observed that the priority ranking of A1 and A6 remains largely unchanged. Additionally, the ranking results of all alternatives do not undergo significant changes with variations in the weights of individual indicators.
5.2.2 Sensitivity analysis of decision support coefficient
The advantage of VIKOR method over other MCDM methods primarily lies in its ability to reflect the DMs’ subjectivity, allowing them to make more aggressive or conservative decisions. This advantage is manifested in the specific calculation method through the choice of the compromise coefficient. A higher compromise coefficient indicates a greater emphasis on maximizing the overall group utility and less consideration for the personal regrets of the dissenting individuals, which reflects a risk-seeking DM. Conversely, a lower compromise coefficient represents a decision mechanism that aims to minimize individual regrets and belongs to the risk-averse category.
From Figure 7, it can be observed that regardless of how the DMs' strategy changes, A1 and A6 consistently remain the best and worst options, respectively. As the compromise coefficient gradually increases, the priority of the A2 option decreases, indicating that A2 has a significant advantage in a certain criterion. Conversely, the priority of the A3 option increases, suggesting a more balanced performance across multiple indicators. Furthermore, the ranking of the alternative schemes does not undergo significant changes with variations in the compromise coefficient.
5.3 Comparatives analysis
To validate the rationality of comprehensive evaluation model, this paper compares it with several commonly used MCDM methods in the field, as shown in Figure 8. In addition to the VIKOR method, TOPSIS (Technique for Order Preference by Similarity to an Ideal Solution), TODIM, and FCE (Fuzzy Comprehensive Evaluation) have been widely used by many scholars in the field of comprehensive evaluation. By observing Figure 8, it can be noted that A1 and A3 are consistently ranked among the top two options across all methods. Furthermore, except in the case of FCE, A1 is the optimal solution in all methods, as FCE does not consider the specificity of the solutions and the DMs' preferences. Additionally, the ranking of the alternatives remains relatively stable across all methods. Therefore, the model constructed in this paper demonstrates rationality.
6 Conclusion and outlook
Sustainable development is a consensus and goal of the entire human society. With the continuous maturation of new energy generation technologies and storage technologies such as hydrogen energy, HES represents the inevitable trend towards integrating energy sources and loads in future energy systems. However, the lack of a comprehensive evaluation system hinders the development and layout of HES. Therefore, this paper constructs a comprehensive evaluation framework for HES from three aspects: system architecture, evaluation indicators, and evaluation models. Firstly, the energy flow of HES, including electricity, heating, and cooling, is clearly decomposed and presented. Secondly, 12 indicators related to the comprehensive evaluation of HES are identified from four dimensions: economic, environmental, technological, and social-policy. Specific quantitative methods are provided for the quantitative indicators. Then, a comprehensive evaluation model based on fuzzy theory and MCDM theory is constructed. Finally, the robustness and rationality of the proposed method are verified through sensitivity analysis and comparative analysis. The main conclusions derived from this study are as follows:
(1) C11(Investment cost), C31 (Comprehensive energy efficiency), C12 (Dynamic payback period) and C32 (Energy supply reliability) are the four most important criteria, with weights of 7.21%, 7.17%, 7.17% and 7.15% respectively. C11 and C12 reflect the economic characteristics of HES as an energy project. C31 and C32, on the other hand, represent the energy supply characteristics of HES.
(2) A1 is the optimal alternative for the layout of the HES in a certain industrial park in Gansu. However, A1 has shortcomings in land occupation, noise, and ES equivalent utilization coefficient. DMs can optimize this scheme in these three aspects to maximize the benefits of the HES.
(3) When collecting and processing expert information, the reasonable use of fuzzy theory can maximize the acquisition and retention of original decision information, and it can fully reflect the psychological factors of DMs.
(4) In the application process of the fuzzy VIKOR method, DMs can change the compromise coefficient to reflect the changes in decision psychology and influence the final determination of the scheme.
The comprehensive evaluation framework of the HES constructed in this article is universal and can serve as a reference for the layout of HES in places with abundant wind and solar resources. However, There are still some shortcomings in this paper: ① Since this decision support model has not been used in real HES, the true performance of the optimal scheme selected based on this model is still open to question. ② In the VIKOR method, the combination coefficient of group benefit value and individual loss value is 0.5, which is the value used in most literature. Therefore, how to improve the value of coefficient is also an important direction of optimization model; ③ Comprehensive evaluation index system of HES is established in the current development background. When the future socio-economic situation changes or disruptive technologies emerge, the indicators should also be adjusted accordingly. Therefore, we will continue to optimize the model and solve the problems in the above three aspects in the follow-up research work.
Data availability statement
The raw data supporting the conclusions of this article will be made available by the authors, without undue reservation.
Author contributions
XC: Conceptualization, Formal Analysis, Investigation, Methodology, Writing–original draft, Writing–review and editing. CC: Conceptualization, Methodology, Writing–review and editing. GT: Data curation, Investigation, Methodology, Project administration, Supervision, Writing–review and editing. YY: Investigation, Methodology, Resources, Supervision, Writing–review and editing. YZ: Conceptualization, Funding acquisition, Investigation, Project administration, Supervision, Validation, Visualization, Writing–review and editing.
Funding
The author(s) declare that no financial support was received for the research, authorship, and/or publication of this article.
Conflict of interest
Authors XC, CC, and GT were employed by State Grid Hebei Electric Power Co., Ltd.
The remaining authors declare that the research was conducted in the absence of any commercial or financial relationships that could be construed as a potential conflict of interest.
Publisher’s note
All claims expressed in this article are solely those of the authors and do not necessarily represent those of their affiliated organizations, or those of the publisher, the editors and the reviewers. Any product that may be evaluated in this article, or claim that may be made by its manufacturer, is not guaranteed or endorsed by the publisher.
References
Abdul, D., Wenqi, J., and Tanveer, A. (2022). Prioritization of renewable energy source for electricity generation through AHP-VIKOR integrated methodology. Renew. Energy 184, 1018–1032. doi:10.1016/j.renene.2021.10.082
Akhanova, G., Nadeem, A., Kim, J. R., and Azhar, S. (2020). A multi-criteria decision-making framework for building sustainability assessment in Kazakhstan. Sustain. Cities Soc. 52, 101842. doi:10.1016/j.scs.2019.101842
Devrim, Y., and Bilir, L. (2016). Performance investigation of a wind turbine–solar photovoltaic panels–fuel cell hybrid system installed at İncek region – ankara, Turkey. Energy Convers. Manag. 126, 759–766. doi:10.1016/j.enconman.2016.08.062
Eriksson, E. L. V., and Gray, E. M. (2017). Optimization and integration of hybrid renewable energy hydrogen fuel cell energy systems – a critical review. Appl. Energy 202, 348–364. doi:10.1016/j.apenergy.2017.03.132
Ghenai, C., Albawab, M., and Bettayeb, M. (2020). Sustainability indicators for renewable energy systems using multi-criteria decision-making model and extended SWARA/ARAS hybrid method. Renew. Energy 146, 580–597. doi:10.1016/j.renene.2019.06.157
Huang, R., Han, L., Zhang, F., and Peng, H. (2021). Application of the fuzzy interval multi-attribute assessment in the analysis of water quality. Yellow River 43 (05), 104–109.
Kamali Saraji, M., Aliasgari, E., and Streimikiene, D. (2023). Assessment of the challenges to renewable energy technologies adoption in rural areas: a Fermatean CRITIC-VIKOR approach. Technol. Forecast. Soc. Change 189, 122399. doi:10.1016/j.techfore.2023.122399
Ke, Y., Liu, J., Meng, J., Fang, S., and Zhuang, S. (2022). Comprehensive evaluation for plan selection of urban integrated energy systems: a novel multi-criteria decision-making framework. Sustain. Cities Soc. 81, 103837. doi:10.1016/j.scs.2022.103837
Li, H., Wang, X., Gao, Y., and Liang, H. (2018). Evaluation research of the energy supply system in multi-energy complementary park based on the improved universal generating function method. Energy Convers. Manag. 174, 955–970. doi:10.1016/j.enconman.2018.08.068
Li, J., Qu, C., Li, C., Liu, X., and Novakovic, V. (2022a). Technical and economic performance analysis of large flat plate solar collector coupled air source heat pump heating system. Energy Build. 277, 112564. doi:10.1016/j.enbuild.2022.112564
Li, Z., Wang, J., Zhou, H., Zong, X., Sun, Y., and Xiong, J. (2022b). Evaluation method for park-level integrated energy system planning considering the interaction of multiple indices. Electr. Power Constr. 43 (10), 98–110.
Liang, S., and Wang, Y. (2023). Evaluation of an integrated energy system planning scheme for an industrial park based on an improved cloud matter-element model. Power Syst. Prot. Control 51 (09), 165–176. doi:10.19783/j.cnki.pspc.221097
Liu, C., Wang, H., Wang, Z., Liu, Z., Tang, Y., and Yang, S. (2022b). Research on life cycle low carbon optimization method of multi-energy complementary distributed energy system: a review. J. Clean. Prod. 336, 130380. doi:10.1016/j.jclepro.2022.130380
Liu, D., Gao, X., An, H., Qi, Y., Sun, X., Wang, Z., et al. (2019). Supply and demand response trends of lithium resources driven by the demand of emerging renewable energy technologies in China. Resour. Conservation Recycl. 145, 311–321. doi:10.1016/j.resconrec.2019.02.043
Liu, Z., Cui, Y., Wang, J., Yue, C., Agbodjan, Y. S., and Yang, Y. (2022a). Multi-objective optimization of multi-energy complementary integrated energy systems considering load prediction and renewable energy production uncertainties. Energy 254, 124399. doi:10.1016/j.energy.2022.124399
Meniz, B., and Ozkan, E. M. (2023). Vaccine selection for COVID-19 by AHP and novel VIKOR hybrid approach with interval type-2 fuzzy sets. Eng. Appl. Artif. Intell. 119, 105812. doi:10.1016/j.engappai.2022.105812
Niu, X., Zhan, Z., Li, B., and Chen, Z. (2022). Environmental governance and cleaner energy transition: evaluating the role of environment friendly technologies. Sustain. Energy Technol. Assessments 53, 102669. doi:10.1016/j.seta.2022.102669
Qian, J., Wu, J., Yao, L., Mahmut, S., and Zhang, Q. (2021). Comprehensive performance evaluation of Wind-Solar-CCHP system based on emergy analysis and multi-objective decision method. Energy 230, 120779. doi:10.1016/j.energy.2021.120779
Qin, G., Zhang, M., Yan, Q., Xu, C., and Kammen, D. M. (2021). Comprehensive evaluation of regional energy internet using a fuzzy analytic hierarchy process based on cloud model: a case in China. Energy 228, 120569. doi:10.1016/j.energy.2021.120569
Ruiming, F. (2019). Multi-objective optimized operation of integrated energy system with hydrogen storage. Int. J. Hydrogen Energy 44 (56), 29409–29417. doi:10.1016/j.ijhydene.2019.02.168
Sezer, N., Bicer, Y., and Koç, M. (2019). Design and analysis of an integrated concentrated solar and wind energy system with storage. Int. J. Energy Res. 43, 3263–3283. doi:10.1002/er.4456
Shen, M., Zhang, G., and Zhang, K. (2022). Comprehensive evaluation method and application study of campus-level regional integrated energy system. J. Of Beijing Inst. Of Technol. Soc. Sci. Ed. 24 (04), 52–65. doi:10.15918/j.jbitss1009-3370.2022.1649
Song, X., Su, Y., Zhang, L., and Li, S. (2022). Benefit evaluation of rural comprehensive energy system based on improved matter-element extension model. Price theory Pract. (02), 170–174. doi:10.19851/j.cnki.CN11-1010/F.2022.02.082
Wen, Q., Liu, G., Wu, W., and Liao, S. (2021). Multicriteria comprehensive evaluation framework for industrial park-level distributed energy system considering weights uncertainties. J. Clean. Prod. 282, 124530. doi:10.1016/j.jclepro.2020.124530
Wu, Y., Yong, X., Tao, Y., Zhou, J., He, J., Chen, W., et al. (2023a). Investment monitoring key points identification model of big science research infrastructures -- Fuzzy BWM-entropy-PROMETHEE Ⅱ method. Socio-Economic Plan. Sci. 86, 101461. doi:10.1016/j.seps.2022.101461
Wu, Y., Zhang, J., Yuan, J., Geng, S., and Zhang, H. (2016). Study of decision framework of offshore wind power station site selection based on ELECTRE-III under intuitionistic fuzzy environment: a case of China. Energy Convers. Manag. 113, 66–81. doi:10.1016/j.enconman.2016.01.020
Wu, Y., Zhou, J., Hu, Y., Li, L., and Sun, X. (2018). A TODIM-based investment decision framework for commercial distributed PV projects under the energy performance contracting (epc) business model: a case in east-Central China. Energies 11, 1210. doi:10.3390/en11051210
Yang, K., Ding, Y., Zhu, N., Yang, F., and Wang, Q. (2018). Multi-criteria integrated evaluation of distributed energy system for community energy planning based on improved grey incidence approach: a case study in Tianjin. Appl. Energy 229, 352–363. doi:10.1016/j.apenergy.2018.08.016
Yong, X., Chen, W., Wu, Y., Tao, Y., Zhou, J., and He, J. (2022). A two-stage framework for site selection of underground pumped storage power stations using abandoned coal mines based on multi-criteria decision-making method: an empirical study in China. Energy Convers. Manag. 260, 115608. doi:10.1016/j.enconman.2022.115608
Yong, X., Wu, Y., Zhou, J., Tao, Y., and Chen, W. (2023). Prospects and barriers analysis framework for the development of energy storage sharing. Sustain. Cities Soc. 89, 104368. doi:10.1016/j.scs.2022.104368
Zeng, B., Zheng, T., Yang, Y., and Wang, J. (2023). A novel grey Verhulst model with four parameters and its application to forecast the carbon dioxide emissions in China. Sci. Total Environ. 899, 165648. doi:10.1016/j.scitotenv.2023.165648
Zhang, H., Jiang, J., Song, M., and Yong, X. (2022b). Comprehensive benefit evaluation of transmission and substation project for renewable energy connection: a case in China. Energy Rep. 8, 12653–12667. doi:10.1016/j.egyr.2022.09.085
Zhang, L., Chai, J., Xin, H., and Zhao, Z. (2021). Evaluating the comprehensive benefit of hybrid energy system for ecological civilization construction in China. J. Clean. Prod. 278, 123769. doi:10.1016/j.jclepro.2020.123769
Zhang, S., Shi, B., and Ji, H. (2023). How to decouple income growth from household carbon emissions: a perspective based on urban-rural differences in China. Energy Econ. 125, 106816. doi:10.1016/j.eneco.2023.106816
Zhang, Y., Sun, H., Tan, J., Li, Z., Hou, W., and Guo, Y. (2022a). Capacity configuration optimization of multi-energy system integrating wind turbine/photovoltaic/hydrogen/battery. Energy 252, 124046. doi:10.1016/j.energy.2022.124046
Zhao, E., Zhang, B., Li, H., Wu, Z., and Lei, X. (2022). Economic benefit evaluation of regional integrated energy system based on improved minimum cross entropy method. Electr. Power Eng. Technol. 41 (02), 215–223.
Zheng, G., and Wang, X. (2020). The comprehensive evaluation of renewable energy system schemes in tourist resorts based on VIKOR method. Energy 193, 116676. doi:10.1016/j.energy.2019.116676
Zhou, J., Wu, Y., Dong, H., Tao, Y., and Xu, C. (2020). Proposal and comprehensive analysis of gas-wind-photovoltaic-hydrogen integrated energy system considering multi-participant interest preference. J. Clean. Prod. 265, 121679. doi:10.1016/j.jclepro.2020.121679
Keywords: hybrid energy system, comprehensive evaluation, comprehensive weights method, VIKOR method, hesitant fuzzy linguistic term set
Citation: Chen X, Chen C, Tian G, Yang Y and Zhao Y (2023) Comprehensive evaluation research of hybrid energy systems driven by renewable energy based on fuzzy multi-criteria decision-making. Front. Energy Res. 11:1294391. doi: 10.3389/fenrg.2023.1294391
Received: 14 September 2023; Accepted: 28 November 2023;
Published: 29 December 2023.
Edited by:
Jianli Zhou, Xinjiang University, ChinaReviewed by:
Yalong Li, China University of Mining and Technology, ChinaShuai Geng, Shandong Jianzhu University, China
Quanying Lu, Beijing University of Technology, China
Copyright © 2023 Chen, Chen, Tian, Yang and Zhao. This is an open-access article distributed under the terms of the Creative Commons Attribution License (CC BY). The use, distribution or reproduction in other forums is permitted, provided the original author(s) and the copyright owner(s) are credited and that the original publication in this journal is cited, in accordance with accepted academic practice. No use, distribution or reproduction is permitted which does not comply with these terms.
*Correspondence: Yunhao Zhao, eXVuaGFvemhAMTI2LmNvbQ==