- 1School of Economics and Management, University of Mining and Technology, Xuzhou, China
- 2School of Economics and Management, Suqian University, Suqian, China
- 3Rural Revitalization Institute, Fujian Agriculture and Forestry University, Fuzhou, China
In this paper, 22 indexes are selected at three levels, including the informatization development level, the Internet development level, and the digital transaction development level, based on China’s provincial panel data from 2011 to 2020, so as to build a digital economy development index system. Moreover, 28 basic indexes are selected from three aspects, including energy construction, energy production and energy consumption, so as to develop an energy economy development evaluation index system. The development index of China’s digital economy and energy economy are measured by using the entropy weight method. The effect of the digital economy on the energy economy and its mechanism are tested by the static panel, the dynamic panel, and the mediating effect and regulating effect models. The results indicate that the digital economy has pronouncedly promoted the development of China’s energy economy, and the development of the digital economy can have an effect on the rationalization of the industrial structure and then affect the development of the energy economy, and there is an intermediary effect. Moreover, the upgrading of the industrial structure is conducive to regulating the digital economy and facilitates the development of the energy economy. The development of the energy economy can be better promoted by focusing on the coordinated regional layout of the digital economy development, building a reliable energy commodity trading platform, and expediting the optimization and upgrading of the industrial structure.
1 Introduction
The digital economy represented by several novel digital technologies (e.g., mobile Internet, artificial intelligence, cloud computing, big data, and 5G) has emerged in the post-industrial era. With the advance of digital technology, the boundaries of conventional factors of production are constantly expanding, and data turns out to be a novel factor of production that has been covered in the economic growth function. The digital economy, i.e., a new economic form, has spawned a considerable number of new formats and new business models and deeply integrated the application of digital technology with conventional industries. On that basis, the digital economy has progressively acted as the key driving force to boost global economic growth. The added value of the digital economy in China will reach 39.2 trillion yuan in 2020, accounting for 38.6% of GDP, as indicated by the data released by the China Academy of Information and Communications Technology. The above result suggests that the digital economy turns out to be indispensable to China’s economy. The Fifth Plenary Session of the 19th CPC Central Committee also highlighted that a digital China should be built, the development of digitalization should be expedited, and it is imperative to make the digital economy act as an accelerator of economic development. The 14th Five-Year Plan and the 2035 Vision Target outline stressed that it is imperative to accelerate the development of digitalization, build a digital China, and deepen the integration of the digital economy and real economy, and the overall digital transformation should be conducted to drive the transformation of production methods.
The Overall Layout Plan for the construction of Digital China proposes to “accelerate the innovation and application of digital technologies in key areas (e.g., agriculture, industry, finance, education, medical care, transportation and energy) and deepen the integration of digital technologies and the real economy.” The vigorous development of the energy economy and empowering the digital transformation of the energy economy with digital technology has been confirmed as the only way for the energy industry to seek high-quality development. Furthermore, this vigorous development is recognized as an urgent requirement and a vital way to facilitate the green and low-carbon transformation of energy and more effectively fulfill the “dual carbon” goal.
In the face of complex and changeable environment, frequent extreme weather and other realistic factors, the development of energy economy fluctuates frequently, and the situation of national energy security is grim. Doing a good job in energy economy, keeping up with the new trend of energy technology revolution, extending the industrial chain, improving the comprehensive utilization efficiency of energy resources, and accelerating the construction of a modern energy system are the internal requirements for ensuring national energy security, striving to achieve carbon peak and carbon neutrality on schedule, and are also important supports for promoting high-quality economic and social development. Technological innovation in the energy industry accelerates industrial upgrading, and it is also urgent to promote high-quality transformation of the industry. In order to cope with the rapid evolution of the development pattern of the energy economy and the energy industry under the fluctuations of the domestic and foreign macro environment, it is necessary to establish a comprehensive and scientific index system to monitor the performance of the energy industry, so as to accurately depict the development and evolution process of the energy economy and the current development heat of each energy industry. Identify the internal links between energy economy, social livelihood and macro economy, and predict the future change trend of energy economy development, determine the future investment hot industries, and discover the potential risks of the industry.
Accordingly, based on the current situation of the development of the digital economy and the energy economy, the paper primarily aims to address three problems, including what is the development status of the digital economy and energy economy in different regions of China, whether the digital economy has an effect on the development of the energy economy, and whether the effect is significant, as well as whether digital economy has mediating and regulating effects on the development of the energy economy.
In this paper, the entropy weight method will be first used to measure the development level of the digital economy and energy economy in each province, and static panel and dynamic panel models are built to investigate the effect of the digital economy on the energy economy. Moreover, the model of the mediating and regulating effects is built to analyze the influence mechanism of the digital economy on the energy economy through the rationalization and upgrading of the industrial structure, and the robustness of the influence mechanism is tested.
Compared with the existing research, the possible marginal contributions of this paper are presented as follows. First, for research indexes, we strive to overcome the lack of comprehensive measurement indexes of the digital economy in existing studies, fully consider the timeliness and accessibility of indexes, and set up 6 secondary indexes and 22 tertiary indexes from three dimensions, including informatization development, Internet development and digital transaction development, to build a more comprehensive index system for measuring the development level of the digital economy. There are relatively few studies on the construction of the energy economic development index system. This paper selects 28 basic indexes from four aspects of energy investment, energy production, energy generation and energy consumption to build an energy economic development evaluation index system and enrich the research on the construction of the energy economic development index system. Second, from the perspective of research, existing studies focus more on the effect of various subjects on energy efficiency, and few calculate the energy economic development index. This paper calculates the development index of the energy economy, and takes the digital economy as the research object, examines the effect of the digital economy on the energy economy, and explores a new path to better promote the development of the energy economy through the development of the digital economy, which can further enrich the research results of the digital economy development. Third, for the research content, the influence mechanism of the digital economy on the energy economy is systematically examined by using mediating effect and regulating effect models, and the research on the digital economy and energy economy is expanded. Fourth, in a practical sense, it not only provides empirical evidence to further boost the development of the digital economy and the energy economy, but also provides empirical support for the digital economy to enhance the energy economy. It takes on great practical significance in promoting the development of the energy economy and the sustainable road of low carbon. Providing the energy industry with the opportunity to improve efficiency, optimize resource use, integrate renewable energy and improve operational performance, ultimately leading to a more sustainable and resilient energy economy.
The chapters of this paper are organized as follows. The first chapter of this paper is the introduction, in which the research background and significance of this paper, the key problems solved, and the possible contribution of this paper are primarily introduced. The second chapter is literature review. In the third chapter, the theoretical basis is laid. In the fourth chapter, variable selection and modeling are introduced. The fifth chapter is empirical research. In the sixth chapter, the summary and policy suggestions are presented.
2 Literature review
2.1 Digital economy
The concept of the digital economy originates from the book The Age of the digital economy by Tapscott, (1996) in 1996. He reported that human beings achieve wealth creation and social development by combining intelligence, knowledge and creativity with Internet technology, and digital economy is considered a new type of economic relationship. He also highlighted that the digital economy is characterized by digitization, knowledge driven and virtualization, whereas the book does not make a clear definition of the digital economy. Since then, the digital economy has aroused wide attention and discussion in the academic community. Lane, (1999) suggested that the digital economy as a fusion economy formed by the integration of computer and communication technology in the Internet, capable of stimulating the transformation of e-commerce. Moulton, (1999) reported that the digital economy comprises information technology and e-commerce. Kling and Lamb, (1999) indicated that the digital economy covers goods and services performed with information technology. Mesenbourg, (2001) directly equated the digital economy with e-commerce. Quah, (2003) expanded the scope of the digital economy and considered it all economic activities that exploit the Internet to trade goods and services. Dahlman et al. (2016) reported the digital economy as a series of economic and social activities conducted after the integration of the Internet and related general technologies. Pei et al. (2018) argued that the data information that determines production efficiency in digital economy production and the technical means of its transmission. Liu, (2019) believes that digital economy is a new technological economic paradigm, which is manifested by the infiltration of digital infrastructure and digital technology into the traditional production mode and lifestyle, and thus the essential changes of macro and micro economic operation mode. The national digital competitiveness evaluation system constructed by Wu, (2019) contains ten relevant elements of digital economy and makes an international comparison. Xu and Zhang, (2020) believe that digital economy is a series of economic activities based on digital technologies and platforms and as the main medium, which digitally empowers infrastructure.
In the study on the measurement of the development level of digital economy, Porat, (1977) first proposed the measurement method of the added value of knowledge economy and information economy. Since then, some international organizations and national government agencies have carried out more in-depth studies, among which a representative and mature research result is the United States Bureau of Economic Analysis, Barefoot et al. (2018), which defined the digital economy as three categories: digital enabling infrastructure, e-commerce and digital media. Li and Han, (2022) and Nie and Zhang, (2022) selected indicators from the aspects of digital infrastructure, digital industrialization and industrial digitalization; (Liu et al., 2022) also considered the aspect of digital integration; Sheng and Liu, (2022) Construct a digital economy index system from the aspects of digital innovation, digital governance, and digital development environment. Liu et al. (2020), Wang et al. (2021), Wang et al. (2022) and others subdivided the second-level indicators on the basis of the first-level indicators, and established the development indicator system of digital economy from the dimensions of input, output, scale and influence.
Digital economy index measurement method research. The Australian Bureau of Statistics (ABS) also uses the BEA methodology. Xiang and Wu, (2019) used the digital economy conceptual framework released by the Organization for Economic Co-operation and Development (OECD) to estimate the added value of China’s digital industry and e-commerce industry. Xu and Zhang, (2020) used the BEA method to measure the added value and total output of China’s digital economy at the national level, and compared it with that of the United States and Australia. On the whole, the digital economy research framework and measurement methods of BEA and OECD have great influence in the world. Li and Wu, (2023) calculated the weights of various indicators and years by using the entropy method and the “thickening the present and thinning the past” method to measure and analyze the development level of digital economy in various provinces in China. There are big differences in the level of digital economy development among different provinces, with Beijing and Shanghai taking the lead in the comprehensive index of digital economy development level, four times more than Gansu, Heilongjiang and Xinjiang.
2.2 Energy economy
For the research on the evaluation system of energy economic development, foreign scholars construct the index system mainly from two aspects. First, the evaluation system is established based on the total factor analysis framework to measure energy economic efficiency or total factor energy efficiency. In the early days, energy economic measurement indexes mainly include labor, capital, energy and regional GDP, among which labor, capital and energy are input indexes, and GDP is the only output index. In 2006, Hu and Wang, (2006) established an input-output index system including labor, capital stock, energy consumption and real GDP, and used DEA method to measure China’s total factor energy efficiency for the first time. Genhua and Qin, (2012) measured the total factor energy efficiency of BRICS countries by incorporating “technology” into the input-output index system. Honma and Hu, (2014) established an index system (e.g., labor, capital stock, energy, non-energy intermediate input, and added value). Since climate change and environmental problems have been increasingly deteriorated, scholars began to pay attention to the effect of environmental pollution on the economy and society, and included environmental pollutants in the conventional input-output index system, defining them as non-expected output and GDP as expected output. Camioto et al., (2016), Zhao et al. (2018), Ohene-Asare et al. (2020) covered carbon dioxide emissions of environmental pollutants in the evaluation index system as non-expected output while measuring the energy efficiency of BRICS countries, Belt and Road countries and African countries respectively. Second, from the perspective of coordinated development of the energy economy and environment, the evaluation system is mainly established from three system levels: energy, economy and environment. Guan et al. (2011) evaluated the dynamic evolution and sustainable development of the system as well as the planning and development strategy using the established urban economy-resource-environment model. Carvalho et al. (2015) evaluated the comprehensive development of energy, economy and environment in Brazil based on the input-output mixed multi-objective model. Similar research involved Luis Cruz et al. (2019) and Wang et al. (2019).
There are numerous factors for the development of the energy economy. Scholars worldwide have discussed the factors for the development of the energy economy through qualitative analysis or empirical research. Indexes (e.g., technological progress, scale economy, and total energy) serve as the major factors considered by foreign scholars. Cui et al. (2014) analyzed the crucial factors for energy efficiency in nine countries using the panel regression model. As indicated by the research results, technical indexes and management indexes serve as the main factors. Liao and Yong, (2018) investigated the effect of technological progress, firm size and energy consumption structure on energy efficiency using the Super-SBM model, the panel regression model, as well as cluster analysis. Miskinis et al. (2020) conducted a comparative analysis of the energy intensity trends of Baltic countries and found that energy conversion efficiency, the share of energy-intensive industries and energy consumption are the factors limiting the development of the energy economy.
Balashova et al. (2020) suggested that with the improvement of renewable energy production level and technological changes, energy efficiency will be increased through data analysis. They confirmed that the enhancement of total factor productivity will facilitate the increase of energy efficiency with the assistance of econometric tools.
2.3 Digital economy and energy economy
There is little research on the digital economy and the energy economy. Xiong, (2022) specifically analyzed the effect of the digital economy on the efficiency of industrial green development from the perspectives of energy and innovation, selected the digital economy development level index as the explanatory variable, and the industrial green development efficiency used the green production efficiency index as the explained variable, and conducted benchmark regression verification through fixed effects. He also used three intermediary variables, energy structure, human capital and innovation output, to test the intermediary effect, and empirically analyzed the effect of the digital economy on the efficiency of industrial green development. Li, (2022) made a qualitative analysis on the mechanism of the digital economy’s effect on industrial energy efficiency in China by combing the relevant literature on the digital economy and energy efficiency. The development level of the digital economy is examined based on the TOPSIS entropy weight method, and the industrial energy efficiency of China is measured by using the SBM model (e.g., unexpected output). The correlation between digital economy development and industrial energy efficiency of China is empirically analyzed by using the two-way panel fixed effect model based on the data of 30 provinces (e.g., cities and districts) in China from 2012 to 2020. Junior et al. (2018) and Rehman et al. (2021) suggest that the general penetration and popularization of the digital economy in the field of energy consumption and environmental protection can be conducive to addressing the problems of environmental carrying capacity decline and scarcity. Alam and Murad, (2020) suggest that the digital economy has great potential in improving the ecological environment. Xia, (2022) analyzed the effect of the digital economy on China’s green energy efficiency, and found that the effect of the digital economy on the green energy efficiency is characterized by a positive “U” shape of first inhibition and then promotion. From the perspective of impact mechanism, digital economy can boost the improvement of green energy efficiency through three channels, including technological innovation, rationalization of the industrial structure and upgrading. Lange et al. (2020) analyzed the effect of the digital economy on energy consumption, and the results indicated that ICT can reduce energy demand. Sun et al. (2023) analyzed the impact of digital finance on carbon productivity using panel data from 201 cities from 2011 to 2020. The research results indicate that digital finance can significantly improve carbon productivity through two transmission channels: human capital and marketization effects. Digital finance has a spatial spillover effect on carbon productivity, and the improvement of local digital finance level will increase the productivity of neighboring regions with carbon emissions. Liu et al. (2023) conducted on the operation, management, and investment of carbon assets for enterprises in the carbon finance market, provided a quantitative decision-making plan for enterprises to purchase carbon emission rights. Created a new method to solve the optimal parking problem.
The literature review indicates that the following three aspects of relevant research are worthy of further promotion. First, few studies have focused on the effect of the digital economy on the energy economy. The essence of the digital economy is digital knowledge + information, and modern information network and information communication technology are its carrier and core driving force. How the digital economy affects the energy economy is worth analyzing. Second, it focuses on the measurement of the digital economy itself or the effect of the spatial agglomeration of the digital economy on the industrial energy efficiency, and rarely studies the effect of the development level of the digital economy on the development level of the energy economy. Third, there is a lack of research on the mechanism of the digital economy’s influence on the development level of the energy economy as the intermediary variable and the rationalization of the industrial structure. Accordingly, this paper will focus on the shortcomings of the above three aspects to promote research, so as to enrich and expand the study of the effect of the digital economy on the energy economy.
3 Theoretical analysis of the effect of the digital economy on the energy economy
3.1 Effect of the digital economy on the energy economy
The G20 Summit defines the digital economy as: The digital economy refers to the use of digital knowledge and information as a key element, modern information networks as an important carrier, and the effective use of information and communication technologies as a vital driving force for efficiency improvement and structural optimization. The digital economy exert two types effects on the development of the energy economy (i.e., positive and negative effects). First, the digital economy exerts a negative effect on the energy economy. Considerable infrastructure construction will consume more energy resources at the initial stage of the development of the digital economy, arising from the energy-intensive nature of the digital economy based on communication technology. Moreover, the energy efficiency increase driven by technological advances may be accompanied by direct or indirect rebound effects. The immediate rebound effect is manifested as follows. The use of efficient energy technologies allows consumers to receive the same amount of services at a lower cost, whereas the decline in economic pressure on consumers may consume more energy. The indirect rebound effect is revealed as follows. The savings in the cost of living of consumers may be adopted to increase other living consumption, such that other consumptions will be risen. As a result, the digital economy may increase energy consumption. Besides, the digital economy positively affects the energy economy. First, at the macro level, the government can use digital technology to gain more insights into the trend of energy market prices and control the total energy supply. Second, from the perspective of the industrial structure, the development of the digital economy can comprehensively boost the optimization and upgrading of the industrial structure, and transfer production factors from inefficient sectors to efficient sectors, thus driving the increase of energy efficiency. Lastly, from the perspective of energy conservation, the digital economy is capable of breaking the time and space constraints and accelerating the flow of production factors, such that energy consumption arising from time and space constraints can be saved, and the overall increase of energy efficiency can be boosted. This paper holds that the effect of the digital economy on the energy economy is mainly positive.
On that basis, hypothesis H1 is proposed, i.e., the digital economy positively affects the energy economy at the national level.
Based on hypothesis 1, this paper will construct digital economy development index system and energy economy development index system in the following analysis, and measure the development level of the digital economy and energy economy in various regions of China through entropy weight method, so as to provide explained variables and core explanatory variables for the subsequent empirical analysis of the effect of the digital economy on the energy economy development level.
3.2 Impact mechanism of the digital economy on the energy economy
Some research has revealed that the digital economy, with data as the key production factor, is capable of greatly reducing the excessive consumption of tangible resources and energy in the conventional production process, accelerating the adjustment of factor structure, and facilitating the improvement of factor utilization efficiency. With the continuous development of the digital economy, the flow barriers of resources in different provinces will be notably reduced, and green technology innovation in different regions will be encouraged to achieve high-quality development with low energy consumption. Furthermore, the digital economy can expedite the transformation of the industrial structure to a higher level and rationalization by stimulating the innovation impetus of a wide range of regions. For instance, Han et al. (2014) reported the progress of production technology as the fundamental driving force to boost the optimization of production sector structure. Digitalization has the attribute of technological progress. Its application in production and operation will facilitate the reconfiguration of production resources and elements and optimize the production system and organizational structure, such that the efficiency of resource allocation can be increased. Moreover, the dissemination and integration of digital technologies will comprehensively boost the optimization and upgrading of the industrial structure in a way that matches industrial development. When industrial structure optimization and upgrading are commensurate with the level of regional development, digital technology will change the economy’s path dependence on energy resources and use efficiency, improve the efficiency of resource allocation, and facilitate the development of the energy economy. Based on the above analysis, the following hypothesis is proposed.
Hypothesis H2: Digital economy promotes the development of the energy economy through the upgrading and rationalization of the industrial structure.
Based on hypothesis 2, in the following analysis, this paper will analyze whether the digital economy has a mediating effect and a regulating effect on the development of the energy economy by constructing a mediating effect model and a regulating effect model.
The effect of the digital economy on the energy economy can be seen in Figure 1.
4 Variable selection and modeling
In order to better empirically test the effect of the development of the digital economy on the development of the energy economy, this paper uses the following methods for analysis. See Figure 2 for details.
4.1 Variable selection
According to the empirical arrangement, the variables involved in this paper are listed in Table 1.
4.2 Introduction of variables
4.2.1 Explained variable
The energy economy is the economic activity of the production, exchange, distribution and consumption of the materials that produce energy. Based on this, this paper mainly reflects the development of energy economy from three aspects: energy construction, energy production and energy consumption. The secondary index selects 28 basic indicators from four aspects, such as energy investment, energy production, energy generation and energy consumption, to build an evaluation index system for energy economic development, as shown in Table 2.
4.2.2 Core explanatory variables
The development index of digital economy is the core explanatory variable. Digital economy is an economic form in which human beings can identify, select, filter, store and use big data (digital knowledge and information) to guide and realize the rapid optimal allocation and regeneration of resources and achieve high-quality economic development. The digital economy includes the “four modernizations” of digital industrialization, industrial digitalization, digital governance, and data value. The digital industrialization includes technologies, products, and services such as 5G, software, AI, and big data. Industrial digitalization includes new industries, new models and new business forms such as industrial Internet, intelligent manufacturing, and Internet of vehicles. Digital governance includes digital government, smart city, etc. Data value includes data right confirmation, data security and so on. In combination with the connotation of digital economy and the construction of other scholars’ index system, as well as the availability of data, this paper selects 22 indicators to build a digital economy development index system from three aspects: informatization development, Internet development and digital transaction development, as shown in Table 3. Digital industrialization and industry digitization are reflected through the information development level, digital governance is reflected through the development of the Internet, and data value is reflected through the development of digital transactions.
4.2.3 Mediating variables and regulating variables
Mediating variable: Industrial structure rationalization (lnTS) serves as the mediating variable. In this paper, the structural deviation degree and Theil index are employed, which have been extensively used in academic circles. When the TS rationalization index is equal to 0, the industrial structure is in the equilibrium state; the larger the index value, the more the industrial structure will deviate from the equilibrium state.
Regulatory variable: This paper uses industrial structure upgrading (lnTC) as a regulatory variable. The upgrading of the industrial structure refers to the gradual transfer of the focus of the industrial structure from the primary industry to the second and third industries. In general, the ratio of output value of tertiary industry to that of secondary industry is adopted to examine the level of the industrial structure upgrading.
4.3 Model setting
As revealed by the above analysis, digital economy can affect the energy economy. Static panel regression analysis (multiple regression analysis, fixed effect model analysis and random effect model analysis) and dynamic panel regression analysis (system GMM model analysis) are established for the model to test whether hypothesis H1 is valid. To test whether the construction of H2 is valid, the mediating effect model and the regulating effect model are established.
4.3.1 Static panel regression model
First, a static panel regression model is built. In the linear regression model of panel data, if the intercept term of the model is different for different cross sections or different time series, whereas the slope coefficient of the model is identical, the model is termed a fixed-effect model. Besides fixed effects model, typical panel data analysis methods also cover random effects model. The fixed effects model (FEM) assumes that all covered studies share a common true effect size, whereas the real effects in the random effects model (REM) vary with extensive research. Based on the calculation of different models, the average value of the combined effect size is different. See form Eq. (1) for details.
In the fixed effect model,
4.3.2 Dynamic panel regression analysis
Given the time dependence of the dependent variable (i.e., adding the time lag term of the dependent variable), the dynamic spatial panel data model can be obtained based on the static panel data model. The development of 30 provinces and cities in China has a certain imbalance in all aspects, thus exhibiting significant spatial characteristics. To better analyze, this paper further adopts the spatial Durbin model to carry out research. The dynamic spatial Durbin model covers the spatial-time term of the dependent variable, the spatial-lag term of the dependent variable, as well as the spatial-lag term of the independent variable. The specific form Eq. (2) is illustrated below:
4.3.3 Intermediary effect model and regulatory effect model
In the previous analysis, this paper believes that the digital economy not only has a direct effect on the development of the energy economy, but also indirectly affects the development of the energy economy by influencing the rationalization of the industrial structure. Thus, the rationalization of industrial organization is an intermediary variable. To verify the existence of intermediary effect, this paper establishes the following intermediary effect model based on model Eqs. (3, 4):
This paper believes that the upgrading of the industrial structure can strengthen the role of the digital economy on the development of the energy economy, and it is necessary to verify the regulatory effect of the development of the digital economy on the development of the energy economy from an empirical perspective. The following regulatory effect model Eq. (5) is established in this paper:
5 Empirical analysis
5.1 Data selection and processing
In this paper, the panel data of 30 provinces, autonomous regions and municipalities in China from 2011 to 2020 are primarily selected as the research object (except for Tibet). In general, the data originate from China Statistical Yearbook, EPS Database, Energy Economic Statistical Yearbook, and so forth. Table 4 lists the descriptive statistical results of the data.
As depicted in Table 4, the standard deviations of the respective variable are relatively small, suggesting that a certain gap between variables in different regions. Nevertheless, the gap is not particularly prominent. The maximum and minimum values of the respective variable suggest a certain gap between variables in different years.
5.2 Measurement results of the digital economy development index
Table 5 present the digital economy development index of Chinese provinces and cities. There is a significant difference in the comprehensive index of the digital economy development level in China’s 30 provinces in 2020. Guangdong and Beijing rank the top two in the comprehensive index of the digital economy development level. Their digital economy development level plays a “bellwether” role in China, and their digital economy development far outpaces that of other provinces. Jiangsu, Zhejiang, and Shanghai are slightly inferior, second only to the first level, which is closely correlated with the better economic development level of these regions. Nevertheless, Qinghai, Ningxia, Hainan, Gansu, and Jilin ranked among the bottom five for the level of the digital economy development since these regions are slow in their economic digital transformation due to factors including remote location, weak infrastructure, insufficient innovation drive, and imperfect talent incentive mechanism. From 2011 to 2020, China’s provinces have shown a strong momentum of the digital economy development, whereas there is a spatial development pattern of high in the south and low in the north, hot in the east and cold in the west, and the development level of the digital economy varies pronouncedly among regions. The Beijing-Tianjin-Hebei region and the Yangtze River Delta region have a higher level of the digital economy development, followed by Shandong, Sichuan, Hubei, Hunan, Anhui, and other Yangtze River Economic belt regions. The above-mentioned areas are densely populated, with high levels of industrial and service development and economic prosperity. Moreover, these areas have produced numerous digital elements, and the digital elements of the areas should be urgently valued, which can provide impetus for the development of the digital economy. Compared with the southern region, the northern region is characterized by a small population, a relatively backward economic level, and the advanced industries and advanced technologies in the digital economy are subjected to slow development.
5.3 Measurement results of energy economic development index
The measurement results of the energy economic development index of China’s provinces and cities are presented in Table 6.
As depicted in Table 6, from 2011 to 2020, the energy economy of China’s provinces has been developing, whereas the overall is relatively stable. In 2020, there is a significant difference in the comprehensive index of energy economic development level of 30 provinces in China, with Shaanxi and Shandong ranking the top two in the comprehensive index of energy economic development level. According to the type of energy demand of the digital economy, China’s energy development presents a highly advanced “double peak” structure. The possible reasons for this structure are large terrain drop, abundant water energy, and high hydropower production. Xinjiang, Guangdong, and Inner Mongolia fare slightly worse, second only to the first tier. Inner Mongolia and Xinjiang are characterized by sufficient sunshine time, strong wind, and large solar and wind power generation, such that these provinces have a high level of energy economic development. In general, the development of China’s energy economy presents a distribution pattern of strong west and weak east. The energy economic development of Hainan, Beijing, Shanghai, Jilin, and Guizhou ranked among the bottom five, which is closely correlated with the relatively low energy production and energy consumption in these regions.
5.4 Regression results of the digital economy development on the energy economy development
Table 7 lists the regression analysis results of the effect of the development of the digital economy on the development of the energy economy.
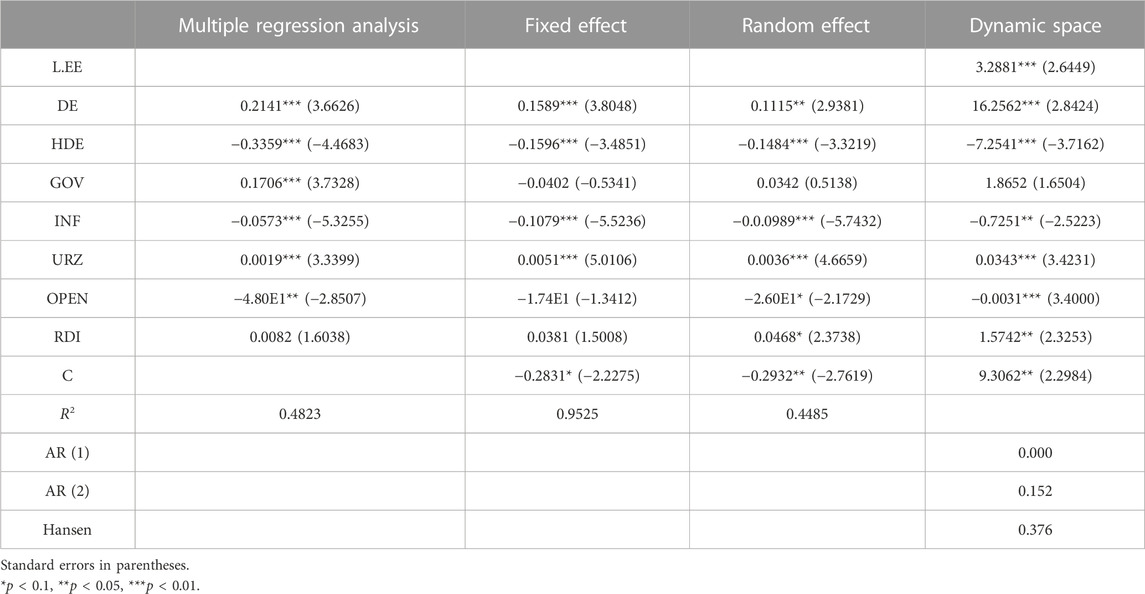
TABLE 7. Regression analysis results for the effect of the digital economy development on the energy economy development.
From the above regression analysis results, it can be seen that the impact coefficient of digital economy on energy economy is 0.2141 in the multiple regression model, 0.1589 in the fixed effect model, 0.1115 in the random effect model, and 16.2562 in the dynamic spatial model. Multiple regression analysis, fixed effects model analysis, random effects model analysis and dynamic spatial model have significant positive effects on the development of energy economy. The development of digital economy is conducive to the development of energy economy. It can be seen from the regression models that the impact of the high quality economic development index on the energy economy is negative, which is consistent with the theoretical analysis. In the multiple regression analysis, government fiscal expenditure has a positive impact on energy economy, while in other models, government fiscal expenditure has no significant impact on energy economy. In each model, the construction of transportation infrastructure and the degree of opening to the outside world have a significant negative impact on the development of energy economy. Both urbanization rate and R&D investment intensity have a significant positive impact on the development of energy economy. Improving urbanization rate and enhancing R&D investment intensity is conducive to promoting the development of digital economy. The above empirical analysis results are basically consistent with the previous theoretical analysis.
5.5 Results of mediating effect and regulating effect
The regression results in Table 8 are analyzed in the following. First, the regression results of the industrial structure rationalization on the digital economy suggest that the regression coefficient of the digital economy on industrial structure rationalization is 761.2482, which is significant at 1% and indicates that the digital economy has a positive impact on industrial structure rationalization. Second, the results of the industrial structure rationalization on the development of the energy economy suggest that the regression coefficient of the industrial structure rationalization is 0.0252, which is significant at 1%. The development of the digital economy can comprehensively facilitate the optimization and upgrading of the industrial structure and make the production factors transfer from the inefficient sector to the efficient sector, which can contribute to the improvement of the development level of the energy economy. This contribution reveals that the rationalization of the industrial structure plays an intermediary role in facilitating the development of the energy economy by digital economy. Table 8 shows that the pre-coefficient
5.6 Robustness test
Robustness test examines the robustness of the interpretation ability of the evaluation methods and indexes, i.e., whether the evaluation methods and indexes still maintain a relatively consistent and stable interpretation of the evaluation results when certain parameters are changed. A specific parameter varies, and the experiment is repeated to verify whether the empirical results vary with the change of the parameter setting. If the results suggest that the symbol and significance vary with the change of the parameter setting, it is not robust, and the problem should be identified.
To more effectively test robustness, Carbon Finance Development Index (CD) is adopted, instead of Energy Economic Development Index for in-depth analysis. Table 8 lists the specific results. As depicted in Table 8, the core explanatory variable Digital Economy Development Index can positively affect the Energy Economic Development Index, and the symbol and significance remain nearly unchanged, suggesting that the model is robust.
6 Conclusion and countermeasures
There has been some research on the connotation and level measurement of the digital economy, which lays a certain basis for this paper. Moreover, the academic circle lays a certain research basis for the development of the energy economy. However, there are still some shortcomings, which are presented as follows. (1) At present, the construction of the evaluation index system for the development of the digital economy in the academic circle should be further improved. (2) There are abundant theoretical studies on energy economic development, whereas there have been few empirical studies on the calculation of energy economic development index. In this paper, the digital economy development index and the energy economy development index are measured by using the entropy weight method. The results show that there is a significant gap between the digital economy development index and energy economy development index in each region. The digital economy development index of Beijing, Shanghai, Jiangsu, Guangdong and other regions with relatively good economic development is higher. In addition, the energy economic development index of Shaanxi, Shandong, Inner Mongolia and Xinjiang is higher. Moreover, the multiple regression analysis model, the fixed effect model, the random effect model and System GMM are used in this paper to empirically test the effect of the digital economy development on China’s energy economy development, and mechanism analysis is conducted. The results suggest that the digital economy has pronouncedly boosted the development of China’s energy economy. The development of the digital economy can have a certain effect on the rationalization of the industrial structure and affect the development of the energy economy, and there is an intermediary effect. Furthermore, the upgrading of the industrial structure has a regulating effect on the digital economy to promote the development of the energy economy.
In this paper, the relevant research on the development of the digital economy and energy economy is enriched, and a new perspective is provided for scholars to calculate the development index of the digital economy and energy economy. At the same time, it provides a certain reference for each region to gain insight into the development level of regional digital economy and energy economy. Moreover, the empirical analysis of the effect of the digital economy on the energy economy is further enriched, and the mechanism study of the effect of the digital economy on the energy economy is expanded, which can better explain how mediating and regulating effects work. However, there is still room for improvement in the calculation of the digital economy development index and the energy economy development index. Since the relevant data for 2021 and 2022 have not been released, the data for 2000–2020 are only measured during the calculation, and the digital economy development index and the energy economy development index for 2021 and 2022 are not measured.
Based on the existing research conclusions, this paper puts forward the following countermeasures and suggestions.
6.1 Focus on the coordinated distribution of the digital economy development among regions
As revealed by the above empirical analysis, the development of the digital economy significantly contributes to the development of the energy economy. To more effectively facilitate the development of the digital economy, a focus should be placed on integrating regional resources, policy support should be offered to backward areas in the development of the digital economy, and “needy households” should be supported from capital, technology, data, talents and other elements. Moreover, it is imperative for local governments to introduce distinctive, regionalized, and highly compatible digital economy development policies, boost the construction of independent technology platforms, accelerate the integration of the real economy and the digital economy, facilitate the flow of factors between regions, and build a digital economy system featuring the coordinated development of financial capital, infrastructure, human resources, as well as research and development levels.
Moreover, a diversified, modern and highly coordinated governance system should be built to expedite the development of the digital economy. The government is required to be the core of supervision, guide judicial organs, industry associations and other organizations, thus that it is enabled to play a certain role in supervision, pay attention to coordination and consider the legitimate interests of enterprises, and jointly address data security, personal privacy, public network security, and other issues. Furthermore, the local Internet regulatory authorities should pay attention to the problem of digital industry monopoly, prevent Internet companies from using information technology loopholes, through algorithmic manipulation, big data and other methods to fulfill the objective of unfair competition, avoid monopolistic behavior to expand the “digital divide” in depth, and guide multiple parties to work jointly for shifting the energy development to the distributed direction.
6.2 Building a reliable energy commodity trading platform
Since the digital information energy platform exhibits integration, interactivity, and rapidness, it is capable of managing energy across regions and across time, ensuring that energy flows to the most needed direction in the form of maximum value, facilitating the development of energy from single to diversified, promoting all parties to actively participate in the efficient allocation of energy, and developing the “energy + green industry” in a coordinated manner. Thus, the Internet platform should serve as the medium, and digital technology should be employed as the tool to develop digital energy service institutions, broaden digital energy information channels and platforms, formulate digital energy trading rules, regulations and policies, deepen the linkage between energy trading markets and futures exchanges, break the conventional barriers to energy trading between regions, and stimulate the transformation of energy trading to digitalization and financialization. On that basis, the information flow between regions turns out to be more extensive and convenient, and the information transparency of energy trading market can be achieved.
6.3 Promoting the optimization and upgrading of the industrial structure
The previous empirical analysis indicates that there are intermediary effects and regulatory effects, and the rationalization of the industrial structure is an important transmission mechanism for the digital economy to enhance the development of the energy economy. To better develop the role of rationalization of the industrial structure and upgrading of the industrial structure, the optimization and upgrading of the industrial structure should be promoted in different development stages of the digital economy, and the long-term increase of energy efficiency should be achieved. The industrial structure is the intermediary variable of the spatial aggregation of the digital economy on energy efficiency. The combination of the digital economy and various industries will produce differences in growth rates to change the industrial structure, and the change of the industrial structure will have an impact on the efficiency of energy use, so that the optimization and upgrading of the industrial structure in the middle and later stages of the development of the digital economy will have a significant effect on energy efficiency.
Accordingly, in the early stage of the development of the digital economy, it is necessary to improve energy efficiency from other factors, and promote the upgrading of the industrial structure in the middle and later stages. However, it is noteworthy that we cannot simply pursue “two backward and three forward”. The specific situation of different regions should be considered, and it is imperative to formulate reasonable policies for optimizing and upgrading the industrial structure; otherwise, the shortage of supply of energy resources will be triggered, resulting in the stagnation of the development of the digital economy and the regression of energy efficiency.
The focus of central and western provinces should be placed on the role of the industrial structure rationalization in increasing green energy efficiency. A significant imbalance of the industrial structure exists in the central and western provinces, inhibiting the increase of energy efficiency. Thus, the provinces in the central and western regions should facilitate the gradual transfer of skilled labor in the secondary industry to high value-added manufacturing and high-tech industries and expedite the transfer of skilled labor in the tertiary industry to high-end service industries, so as to form a reasonable flow mechanism of labor. Furthermore, since the upgrading of the industrial structure can expedite the increase of green energy efficiency, a wide range of regional provinces should formulate reasonable industrial structure optimization and upgrading policies based on the actual situation and level of economic and social development in different regions.
Data availability statement
The original contributions presented in the study are included in the article/Supplementary Material, further inquiries can be directed to the corresponding authors.
Author contributions
LJ: Methodology, Writing–original draft. HN: Supervision, Funding acquisition, Writing–review and editing. YR: Conceptualization, Data curation, Writing–original draft. AT: Formal Analysis, Investigation, Writing–original draft. YW: Writing–review and editing.
Funding
The author(s) declare financial support was received for the research, authorship, and/or publication of this article. This paper is a phased achievement of the General Project of Philosophy and Social Sciences in Jiangsu Province’s Universities - Research on the Coupling Development of High Quality Economic Development and Comprehensive Rural Revitalization from the Perspective of “Two Strives for One Front” (2022SJYB2405). This paper was supported by the Fundamental Research Funds for the Central Universities (2022ZDPYSK02), National Nature Science of China (71871120). This paper was supported by the Jiangsu Blue Project.
Conflict of interest
The authors declare that the research was conducted in the absence of any commercial or financial relationships that could be construed as a potential conflict of interest.
Publisher’s note
All claims expressed in this article are solely those of the authors and do not necessarily represent those of their affiliated organizations, or those of the publisher, the editors and the reviewers. Any product that may be evaluated in this article, or claim that may be made by its manufacturer, is not guaranteed or endorsed by the publisher.
References
Alam, M. M., and Murad, M. W. (2020). The impacts of economic growth, trade openness and technological progress on renewable energy use in organization for economic co-operation and development countries. Renew. Energy 145 (01), 382–390. doi:10.1016/j.renene.2019.06.054
Balashova, S. A., Gomonov, K. G., and Matyushok, V. M. (2020). The influence of energy production from renewable energy sources on energy efficiency and total factor productivity. IOP Conf. Ser. Mater. Sci. Eng. 937, 012003. doi:10.1088/1757-899x/937/1/012003
Barefoot, B., Curtis, D., Jolliff, W., Nicholsom, J. R., and Omohundro, R. (2018). Definin and measuring the digital ecomomy. BEA Working Paper.
Camioto, F. D. C., Moralles, H. F., Mariano, E. B., and Rebelatto, D. A. d. N. (2016). Energy efficiency analysis of G7 and BRICS considering total-factor structure. J. Clean. Prod. 122 (20), 67–77. doi:10.1016/j.jclepro.2016.02.061
Carvalho, A., Antunes, C. H., Freire, F., and Henriques, C. O. (2015). A hybrid input-output multi-objective model to assess economic-energy-environment trade-offs in Brazil. Energy 82, 769–785. doi:10.1016/j.energy.2015.01.089
Cruz, L., Imori, D., Ferreira, J.-P., Guilhoto, J. J. M., Barata, E., and Ramos, P. (2019). Energy-economy-environment interactions: a comparative analysis of Lisbon and sao paulo metropolitan areas. J. Environ. Assess. Policy Manag. 21 (1), 1950002. doi:10.1142/s1464333219500029
Cui, Q., Kuang, H. B., Wu, C. Y., and Li, Y. (2014). The changing trend and influencing factors of energy efficiency: the case of nine countries. Energy 64 (1), 1026–1034. doi:10.1016/j.energy.2013.11.060
Dahlman, C., Mealy, S., and Wermelinger, R. (2016). Harnessing the digital economy for developing countries. Oecd Development Centre Working Papers.
Genhua, H. U., and Qin, S. (2012). Comparative research of total-factor energy efficiency in BRICS based on DEA and tobit models. Resour. Sci. 34 (3), 533–540.
Guan, D., Gao, W., Su, W., Li, H., and Hokao, K. (2011). Modeling and dynamic assessment of urbaneconomy-resource-environment system with a coupled system dynamics-geographic information system model. Ecol. Indic. 11 (5), 1333–1344. doi:10.1016/j.ecolind.2011.02.007
Han, X., Ning, H., and Song, W. (2014). Can informatization improve the efficiency of technological innovation in China's industrial sector. China Ind. Econ. (12), 70–82. doi:10.19581/j.cnki.ciejournal.2014.12.006
Honma, S., and Hu, J. L. (2014). Industry-level total-factor energy efficiency in developed countries: a Japan-centered analysis. Appl. Energy 119 (APR.15), 67–78. doi:10.1016/j.apenergy.2013.12.049
Hu, J. L., and Wang, S. C. (2006). Total-factor energy efficiency of regions in China. Energy Policy 34 (17), 3206–3217. doi:10.1016/j.enpol.2005.06.015
Jiang, L. (2021). The impact of green finance development on China's energy structure optimization. Discrete Dyn. Nat. Soc. 12, 1–13. doi:10.1155/2020/8851684
Junior, J. A. G., Busso, C. M., Gobbo, S. C. O., and Carreão, H. (2018). Making the links among environmental protection, process safety, and industry 4.0. Process Saf. Environ. Prot. 117, 372–382. doi:10.1016/j.psep.2018.05.017
Kling, R., and Lamb, R. (1999). IT and organizational change in digital economies. ACM SIGCAS Comput. andSociety 29 (3), 17–25. doi:10.1145/572183.572189
Lane, N. (1999). Advancing the digital economy into the 21st century. Inf. Syst. Front. 1 (3), 317–320. doi:10.1023/a:1010010630396
Lange, S., Pohl, J., Santarius, T., and Santarius, T. (2020). Digitalization and energy consumption. Does ICT reduce energy demand? Ecol. Econ. 176, 106760. doi:10.1016/j.ecolecon.2020.106760
Li, C., and Wu, L. (2023). Comprehensive measurement and analysis of provincial digital economy development level in China. Statistics Decis. 14 (03), 17–21. doi:10.13546/j.cnki.tjyjc.2023.14.003
Li, S. (2022). Study on the impact of digital economy on industrial energy efficiency in China. Fuxin: Liaoning Technical University.
Li, Y., and Han, P. (2022). Comprehensive evaluation and forecast of China's digital economy development. Statistics Decis. (2), 90–94. doi:10.13546/j.cnki.tjyjc.2022.02
Liao, N., and Yong, H. (2018). Exploring the effects of influencing factors on energy efficiency in industrial sector using cluster analysis and panel regression model. Energy 158, 782–795. doi:10.1016/j.energy.2018.06.049
Liu, C., Jiang, Y., Zhang, Q., et al. (2022). Research on the statistical measurement and spatial-temporal evolution trend of the development level of digital economy. Ind. Tech. Econ. 41 (2), 129–136. doi:10.3969/j.issn.1004-910X.2022.02.016
Liu, J., Yang, Y., and Zhang, S. (2020). Research on Measurement and driving factors of China's digital economy. Shanghai Econ. Res. (6), 81–96. doi:10.19626/j.cnki.cn31-1163/f.2020.06.008
Liu, S. (2019). Targeted path and policy supply for high-quality development of China's digital economy. Economist (6), 52–61. doi:10.16158/j.cnki.51-1312/f.2019.06.006
Liu, Y., Sun, H., Meng, B., Jin, S., and Chen, B. (2023). How to purchase carbon emission right optimally for energy-consuming enterprises? Analysis based on optimal stopping model. Energy Econ. 124 (8), 106758–8. doi:10.1016/j.eneco.2023.106758
Moulton, B. R. (1999). Moulton. GDP and the digital economy:keeping up with the changes. Underst. Digital Econ. Data 4 (5), 34–48.
Miskinis, V., Galinis, A., Konstantinaviciute, I., Lekavicius, V., and Neniskis, E. (2020). Comparative analysis of energy efficiency trends and driving factors in the Baltic States. Energy Strategy Rev. 30, 100514. doi:10.1016/j.esr.2020.100514
Nie, C., and Zhang, F. (2022). Regional differences and driving factors of digital economy development in China: an empirical analysis based on spatial panel model. Tech. Econ. Manag. Res. (4).
Ohene-Asare, K., Nuertey Tetteh, E., and Lamisi Asuah, E. (2020). Total factor energy efficiencyand economic development in Africa. Energy Effic. 13 (6), 1177–1194. doi:10.1007/s12053-020-09877-1
Pei, C. H., Ni, J., and Yue, L. (2018). Political Economy analysis of digital economy. Finance Trade Econ. 39 (9), 5–22. (in Chinese).
Porat, M. (1977). The information economy:definition and measurement. Washington, D.C.: U.S. Department of Commerce.
Rehman, A., Ma, H., Ahmad, M., Ozturk, I., and Işık, C. (2021). Estimating the connection of information technology, foreign direct investment, trade, renewable energy and economic progress in Pakistan: evidence from ARDL approach and cointegrating regression analysis. Environ. Sci. Pollut. Res. 1, 13.
Sheng, B., and Liu, Y. (2022). Measurement and spatial differentiation of China's digital economy development Index. Nanjing Social Sciences.
Sun, H., Chen, T., and Wang, C. N. (2023). Spatial impact of digital finance on carbon productivity. Geosci. Front. 7, 1–15. doi:10.1016/j.gsf.2023.101674
Tapscott, D. (1996). The digital economy:promise and peril in the age of networked intelligence[M]. NewYork: Mc Graw-Hill.
Wang, F., Liu, L., Liu, Q., and Wu, L. (2022). Construction and demonstration of early warning model for high-quality development of digital economy. Statistics Decis. (13), 15–20. doi:10.13546/j.cnki.tjyjc.2022.13.003
Wang, J., Zhu, J., and Luo, Q. (2021). The development level and evolution measurement of China's digital economy. Res. Quantitative Tech. Econ. 38 (7), 26–42. doi:10.13653/j.cnki.jqte.2021.07.002
Wang, S., Song, J., Wang, X., and Yang, W. (2019). The spatial and temporal research on the coupling and coordinated relationship between social economy and energy environment in the belt and road initiatives. Sustainability 11 (2), 407. doi:10.3390/su11020407
Wu, Y. (2019). Study on national digital competitiveness index and international comparison. Stat. Res. 36 (11), 14–25. doi:10.19343/j.cnki.11-1302/c.2019.11.002
Xia, Z. (2022). The impact of the digital economy on China's green energy efficiency: an analysis based on intermediary and threshold effects. Technol. Econ. Manag. Res. (10), 3–9.
Xiang, S., and Wu, W. (2019). Research on the design of satellite account framework for China's digital economy. Stat. Res. 36 (10), 3–16. doi:10.19343/j.cnki.11-1302/c.2019.10.001
Xiong, W. (2022). Study on the impact and measurement of digital economy on the efficiency of industrial green development -- Based on the perspective of energy and innovation. Nanchang: Jiangxi University of Finance and Economics.
Xu, X., and Zhang, M. (2020). Research on the scale measurement of China's digital economy: from the perspective of international comparison. China Ind. Econ. (5), 23–41.
Keywords: digital economy, energy economy, entropy weight method, mediating effect, regulating effect
Citation: Jiang L, Niu H, Ru Y, Tong A and Wang Y (2023) Can the digital economy promote the development of the energy economy? Evidence from China. Front. Energy Res. 11:1257794. doi: 10.3389/fenrg.2023.1257794
Received: 13 July 2023; Accepted: 04 December 2023;
Published: 29 December 2023.
Edited by:
Michael Carbajales-Dale, Clemson University, United StatesCopyright © 2023 Jiang, Niu, Ru, Tong and Wang. This is an open-access article distributed under the terms of the Creative Commons Attribution License (CC BY). The use, distribution or reproduction in other forums is permitted, provided the original author(s) and the copyright owner(s) are credited and that the original publication in this journal is cited, in accordance with accepted academic practice. No use, distribution or reproduction is permitted which does not comply with these terms.
*Correspondence: Huawei Niu, niuhuawei@cumt.edu.cn; Yufan Ru, vam10081@163.com; Aihua Tong, Tongaihua12@163.com; Yifeng Wang, wyf870403@163.com