- 1Powerchina Huadong Engineering Corporation Limited, Hangzhou, China
- 2College of New Energy, China University of Petroleum (East China), Qingdao, China
Green hydrogen generation driven by solar-wind hybrid power is a key strategy for obtaining the low-carbon energy, while by considering the fluctuation natures of solar-wind energy resource, the system capacity configuration of power generation, hydrogen production and essential storage devices need to be comprehensively optimized. In this work, a solar-wind hybrid green hydrogen production system is developed by combining the hydrogen storage equipment with the power grid, the coordinated operation strategy of solar-wind hybrid hydrogen production is proposed, furthermore, the NSGA-III algorithm is used to optimize the system capacity configuration with the comprehensive performance criteria of economy, environment and energy efficiency. Through the implemented case study with the hydrogen production capacity of 20,000 tons/year, the abandoned energy power rate will be reduced to 3.32% with the electrolytic cell average load factor of 64.77%, and the system achieves the remarkable carbon emission reduction. In addition, with the advantage of connect to the power grid, the generated surplus solar/wind power can be readily transmitted with addition income, when the sale price of produced hydrogen is suggested to 27.80 CNY/kgH2, the internal rate of return of the system reaches to 8% which present the reasonable economic potential. The research provides technical and methodological suggestions and guidance for the development of solar-wind hybrid hydrogen production schemes with favorable comprehensive performance.
1 Introduction
With the increasing energy demand and fossil fuel consumption, serve issues of energy shortage and environmental pollution are in urgent need to be addressed (Hussain et al., 2023). The development and utilization of renewable energy can effectively promote the transformation of clean and efficient energy structures and enhance the overall efficiency (Jaber et al., 2008; Kumar and Majid, 2020).
Wind and solar power have been extensively adopted in various sectors, including industrial production (Roesch et al., 2019), residential areas (Graça Gomes et al., 2023) and agriculture (Acosta-Silva et al., 2019), owing to their environmental-friendly attributes and associated advantages. However, the intermittent and unstable natures of wind speed and solar irradiation result in significant randomness and fluctuations of power output, which posing a substantial challenge to the reliable and stable functioning of the power grid (Wahbah et al., 2022; Teferra et al., 2023). In order to mitigate these fluctuations and enhance the grid stable operation, several approaches have been proposed, including wind and solar forecast (Kamani and Ardehali, 2023), energy storage (Wu and Zhang, 2021; Deymi-Dashtebayaz et al., 2022), and electrochemical conversion (Han et al., 2023; Liu et al., 2023).
The energy storage methods consist of battery energy storage, hydrogen energy storage, and flywheel energy storage. These three methods enable the flexible utilization of wind and solar resources (Amry et al., 2023; Fosso Tajouo et al., 2023; Liu et al., 2023). Nevertheless, as wind and solar resources continue to progress, greater demands emerge for enhancing the economic viability of batteries and technological advancements (Hutchinson and Gladwin, 2023). Meanwhile, hydrogen storage presents a more straightforward scaling approach, rendering it applicable in numerous scenarios, including the chemical industry and energy sectors, thereby resulting in heightened economic advantages (Tang et al., 2022; Kakavand et al., 2023).
Water electrolysis hydrogen production stands out as an electrochemical conversion method that strikes a balance between safety and economic feasibility, utilizing the wind and solar resources effectively and reducing the wind and solar energy curtailment by converting low-grade and fluctuating electrical energy into high-grade hydrogen energy (Shiva Kumar and Lim, 2022; Shin et al., 2023).
Currently, hydrogen production technology mainly includes Alkaline Electrolysis (AE), Proton Exchange Membrane Electrolysis (PEME), Anion Exchange Membrane Electrolysis (AEME), Solid Oxide Electrolysis Cell (SOEC). Among them, AE and PEME technologies have been commercialized, while AEMWE and SOEC have demonstrated improvements in hydrogen production efficiency and stability, but they are still under the experimental stage due to challenges of durability and cost considerations (Lim and Kim, 2022; Wappler et al., 2022). Thus, the solar-wind hybrid hydrogen production system is constructed by the integration of wind turbines, photovoltaic panels and water electrolysis cells, which enhances the competitiveness of solar-wind power in the energy market and advances the goal of carbon neutrality (Shen et al., 2021; Wang et al., 2021). developed a multi-energy system composed of alkaline electrolyzer, wind turbine, which can achieve stable power output and energy storage capabilities, and effectively address the power supply problem in remote areas (Temiz and Dincer, 2022; Zhang F. et al., 2023). proposed the solar-wind-hydrogen multi-energy system to meet residents energy demands (Song et al., 2022). concluded that the optimal cost-effective solution for carbon neutrality in the context of solar-wind energy-based power supply is the integration of complementary solar-wind hybrid hydrogen production system, which could further reduce the carbon emissions in industrial and transportation parts.
However, with the enlarged scale of solar-wind power plant and the trend towards large-scale hydrogen production, the issue of investment and maintenance costs for the hydrogen production and storage system needs to be considered, and thus the optimization of system capacity configuration becomes crucial (Prestat, 2023). To address these challenges (Kiehbadroudinezhad et al., 2022), developed a capacity configuration optimization model for the solar-wind combined seawater hydrogen production system, and proved the environmental benefits of the optimized system. It has contributed to alleviating the environmental limitations of wind and solar power generation hydrogen production applications (Al-Buraiki and Al-Sharafi, 2022). optimized the capacity configuration of a solar-wind hybrid hydrogen production system in a certain area, achieving a hydrogen production cost of up to 36.32 $/kg under reasonable conditions of loss of hydrogen supply probability (LHSP), it provides a framework for achieving a more stable and economical production of green hydrogen. Yang et al. (Zhang P. et al., 2023) optimized the capacity configuration of the solar-wind hybrid hydrogen production system based on government subsidies and environmental benefits, resulting in a 38.9% increase in annual profit for the optimized hybrid system, optimizing the system based on local policies to attain economic benefits demonstrates that policy support is a crucial factor influencing the cost of hydrogen production (Izadi et al., 2022). optimized the solar-wind hybrid hydrogen production system in buildings based on installation cost, CO2 production and loss of power supply probability, showing that the optimized hybrid system can meet 70%–80% of urban building electricity, it provides an important scheme for the high proportion utilization of renewable energy in the future (Nasrabadi and Korpeh, 2023). optimized the capacity configuration of the hydrogen production system based on minimizing the system cost, leading to an increased exergy efficiency of 20.7% and hydrogen production rate of 1% with the total cost rate value reduction of 2%. Additionally (Al-Ghussain et al., 2023), took the supply-demand relationship and energy cost as the capacity configuration optimization objectives of the solar-wind hybrid hydrogen production system. The optimized system required a higher storage capacity by 75.77%, but the hydrogen production cost is more competitive (Lv et al., 2023). optimized the capacity configuration of solar-wind hybrid hydrogen production system based on the fluctuation of green electricity transaction price and hydrogen demand. The optimized system hydrogen demand increased by 40%, which effectively improved the ability to resist the uncertainty of hydrogen demand, considering the demand of power grid and the price of power transaction to adjust the system and formulate the scheduling strategy can improve the flexibility of wind and solar resource scheduling and contribute to improving the market competitiveness of green hydrogen Lu et al. (2023). optimized the solar-wind complementary hydrogen production system in green buildings with the goal of minimizing system cost and maximizing reliability, the optimized improvement of the system's energy supply stability helps to promote the high proportion of renewable energy in life. The optimal configuration of the system occurs when the reliability of the system is 12% and 15%. Based on Levelized Cost of Hydrogen (Superchi et al., 2023), optimized the capacity configuration of solar-wind hybrid hydrogen production system. The results show that the optimized system can still achieve competitive hydrogen production cost under the current technical conditions (Behzadi and Sadrizadeh, 2023). optimized the solar-wind hybrid hydrogen production system with the optimal operating state, the optimized system carbon emissions and the cost are reduced by 8% and 38%, respectively, taking the economy of hydrogen production and carbon emissions as the measurement indicators, the further optimization research on green electricity hydrogen production can enhance the market competitiveness of green hydrogen, promote low-carbon environmental protection, and further promote the clean transformation of energy structure.
Along with being a crucial component in large-scale hydrogen production, the size of wind and solar power generation, the capacity configuration of electrolytic cells, energy storage, and other equipment all have a significant impact on the system’s overall performance. Therefore, considerations like economy, environment, and energy consumption aspects will be taken into account and transformed into multi-objective optimization problems in order to better measure system performance from different viewpoints. To further improve the system, a redesigned system capacity configuration optimization method using the NSGA-III algorithm is proposed, in order to optimize the solar-wind hybrid hydrogen production system. In addition, the comprehensive performance and dynamic operation of the optimized system are thoroughly evaluated. The main contribution can be outlined as follows:
(1) The system capacity configuration optimization approach based on the NSGA-III algorithm is suggested for large-scale hydrogen production scenarios, with the aim of thoroughly optimizing the grid-connected solar-wind complementing hydrogen production system.
(2) A comprehensive performance evaluation of the optimized capacity design is thoroughly assessed in terms of economy, environment and utilization efficiency, and the monthly and daily conditions are further examined.
(3) Based on the proposed control strategy of solar-wind hydrogen production, the complementary characteristics of solar-wind power generation and the dynamic operation of the system under typical monthly cycles are analyzed.
The rest is organized as follows: the process of conceptual and mathematical modeling is descripted in Section 2. Section 3 introduces evaluation indicators and optimization objectives. The results and analysis are discussed in Section 4. In Section 5, the main conclusions are summarized.
2 Solar-wind hybrid hydrogen production system and performance evaluation method
The combination of water electrolysis hydrogen production technology and solar-wind power generation has multiple advantages, providing an effective approach to convert the renewable energy, and also provides an effective and feasible way for large-scale production of green hydrogen.
2.1 Solar-wind hybrid hydrogen production system
In this work, a green hydrogen generation system driven by solar-wind hybrid power with the water electrolysis technology is developed. The system will stabilize the output of hydrogen as the main goal, but also to meet the requirements of large-scale green hydrogen production throughout the year. It consists of wind turbines, photovoltaic arrays, alkaline electrolyzers, energy storage batteries and hydrogen tanks, as shown in Figure 1. The wind turbine and photovoltaic systems are employed as the primary power generation equipment to supply eco-friendly energy for electrolyzing hydrogen production. Concurrently, to mitigate the impact of fluctuations on hydrogen production, battery and hydrogen storage tanks are utilized as coordination equipment for power and hydrogen transmission, effectively enhancing system stability. Furthermore, the incorporation of the power grid enables the absorption of surplus wind and solar power, thereby optimizing the utilization of these renewable sources while also furnishing additional power to the electrolytic cell, further bolstering the system’s stability.
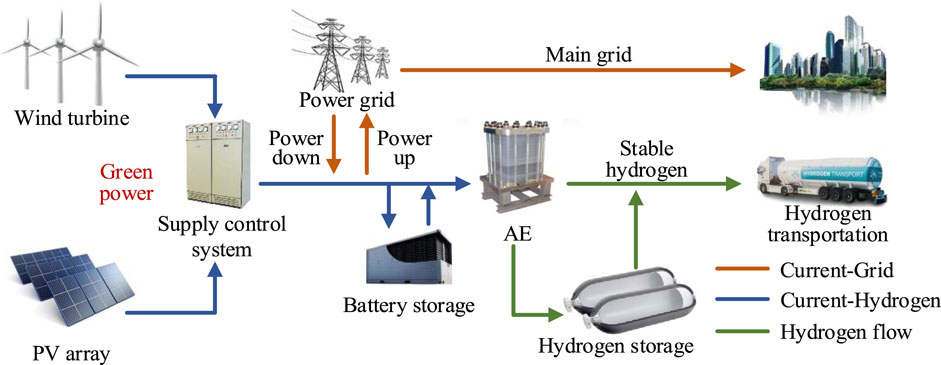
FIGURE 1. To further study the system capacity configuration optimization from green hydrogen generation system driven by solar-wind hybrid power, a brief and complete system is developed, which mainly consists of wind turbines, photovoltaic arrays, alkaline reactors (AE). Energy storage batteries and hydrogen tanks.
Due to the instability of the wind speed and solar radiation throughout the year, in order to further realize the demand of generating stable hydrogen load, the basic operation strategy of grid-connected solar-wind hybrid hydrogen production system is developed. Firstly, the hydrogen output load is predetermined, and the system utilizes this load as a stable output, with wind and solar power generation serving as the primary power supply sources. Based on the hydrogen load and available wind power, two modes of operation can be identified:
(1) Wind-solar power exceeds the power required for hydrogen output load: In this scenario, the hydrogen production rate of the electrolytic cell surpasses the hydrogen output load, leading to an excess of hydrogen, which is then stored in the hydrogen storage tank. Any surplus power generated is directed towards charging the battery. If the battery capacity reaches its upper limit, the surplus power can be transmitted to the grid for external use;
(2) Wind-solar power is less than the power required for hydrogen output load: In such cases, the hydrogen production rate of the electrolytic cell is unable to meet the required hydrogen output load. Consequently, the hydrogen from the hydrogen storage tank is simultaneously utilized to meet the demand. If there’s not enough hydrogen in the tank, power from the battery will be utilized to enhance the hydrogen production rate of the electrolytic cell. If both reserves prove insufficient, the system resorts to purchasing electricity from the grid to augment the hydrogen production rate.
Compared with the off-grid type system, by considering the power grid connection scenario, the grid-connected hydrogen production system allows for the maintenance of a minimum operating interval for the electrolyzer, thus minimizing start-stop cycles, and also enhancing the hydrogen production capacity and the operational lifespan of the electrolyzer. This operational strategy ensures the fulfillment of stable hydrogen load requirements while guaranteeing the safe and stable operation of each equipment component.
2.2 Solar-wind hybrid hydrogen system modeling
2.2.1 Solar and wind power output modeling
The energy source of the whole system comes from the wind turbine and photovoltaic array, and the wind turbine output power PWT is mainly dependent on the wind speed v, so the wind power has the significant and irregular fluctuation characteristics. The output power by the wind turbine can be calculated as follows (Chaichan et al., 2022; Kiehbadroudinezhad et al., 2022):
where vin, vout are the cut-in and cut-out wind speed, respectively. vr is the rated wind turbine speed. NWT means the number of installations. PWT_r is the rated wind turbine power and can be calculated as follows:
where ρ means the air density. SWT means the swept area of the rotor, and τ represents the coefficient of performance of the wind turbines.
Photovoltaic array converts the solar radiation into electrical energy through photoelectric effect, and the photovoltaic output power PPV can be calculated as follows (Praveenkumar et al., 2022).
where Nb and Nc are the number of photovoltaic cells in parallel and photovoltaic cells in series, respectively. Iph and Irs mean photo-generated current and the reverse saturation current of the diode, respectively. UPV is the output voltage of photovoltaic cells. TPV is the output temperature of photovoltaic cells. α and β represent the diode quality factor and electron charge, respectively. K is the Boltzmann constant.
2.2.2 AE electrolyzer modeling
The AE is adopted as one of the critical equipment in this hydrogen production system. Its operating power is mainly affected by its own polarization characteristics. According to its polarization characteristics, the power PAE of the electrolyzer can be calculated as follows (Fang and Liang, 2019).
where IAE is the AE input current. TAE and AAE mean the cell temperature and the electrolytic cell effective area, respectively. w1, w2, w3, w4 and w5 are empirical coefficients. U0 is reversible voltage and s is the electrode overvoltage coefficient.
And then the molar rate of hydrogen production nH2 is obtained as follows:
where NAE represent the number of electrolytic cells. F is the Faraday constant of 96487 C/mol.
2.2.3 Battery and hydrogen storage modeling
In order to further improve the utilization rate of wind and solar energy, the lithium iron phosphate battery is employed as an energy storage device, which enables the storage of the excess wind and solar energy power after the hydrogen production and to supplement when the power is insufficient. The capacity EBA(t) at time t can be expressed as follows:
where σ means the self-discharge rate of the battery. ηBA_in and ηBA_out represent charging efficiency and discharging efficiency respectively. EBA_max and PBA(t) are the max total capacity and power of the battery. In addition, when PBA(t) > 0 the battery will be charged, and when PBA(t) < 0, the battery will be discharged.
Moreover, considering the volatility inherent in solar-wind hydrogen production, the inclusion of hydrogen storage equipment is crucial to enhance the stability of hydrogen transportation. Within this solar-wind hybrid hydrogen production system, gaseous high-pressure hydrogen storage technology is primarily employed for short-term storage of hydrogen, ensuring efficient and reliable operation. According to the Clapeyron equation, the state of the tank can be obtained by Eq. 7.
where QHT is the volume of the hydrogen storage tank. JHT(t) and nHT(t) are the volume of the hydrogen storage tank and the hydrogen production rate at time t, respectively. THT is the thermodynamic temperature of hydrogen storage, and R represents the ideal gas constant.
3 Solar-wind hybrid hydrogen production system comprehensive evaluation method
3.1 System comprehensive evaluation method
The implementing the operation strategy of solar-wind hybrid hydrogen production system contributes to effectively achieving the goal of stable hydrogen production, meanwhile, in order to make full use of the renewable energy, a grid-connected solar-wind hybrid hydrogen production system is established. The operational dynamics and capacity configuration of this system significantly influence the comprehensive benefits throughout the life cycle, subsequently impacting the expansion and investment in solar-wind hydrogen production. As a result, it plays a vital role in the development of related investments and requires comprehensive performance evaluation methods for assessing its overall benefits, as depicted in Figure 2.
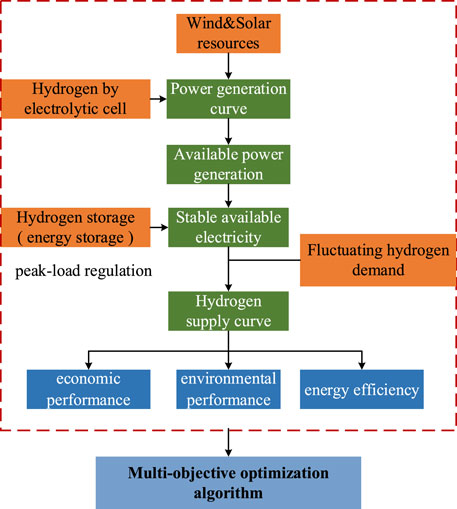
FIGURE 2. To comprehensively assess the overall benefits of the solar-wind hybrid hydrogen production system, including economic performance, environmental performance, and energy efficiency, a set of rigorous performance evaluation methods is employed. These methods facilitate a holistic analysis of the system’s effectiveness. The accompanying optimization flow chart visually outlines the process.
While the evaluation encompasses economic performance evaluation, quantifying the economic benefits throughout the system’s life cycle; environmental performance evaluation, assessing the environmental friendliness during the system’s life cycle; and energy efficiency evaluation, measuring the energy utilization during system operation.
Initially, the key indicators corresponding to each evaluation criterion are defined as the foundation for optimizing the capacity configuration. Specifically, a multi-objective optimization approach is employed to optimize the capacity configuration of wind turbines, photovoltaic arrays, alkaline electrolyzers, energy storage batteries, hydrogen storage tanks, and other components in the solar-wind hybrid hydrogen production system. The NSGA-III algorithm is applied to achieve an optimal configuration that maximizes the overall performance. Subsequently, the optimized scheme is thoroughly analyzed using comprehensive performance indicators, resulting in the development of a comprehensive evaluation methodology for solar-wind hybrid hydrogen production system.
3.2 Comprehensive performance evaluation modeling
The performance evaluation model comprises three categories of performance indicators, establishing a comprehensive framework for evaluating the solar-wind hybrid hydrogen production system. This model is utilized to simulate and assess the system’s performance by evaluating its economic performance, environmental performance, and energy efficiency.
3.2.1 Economic evaluation
The primary objective of the solar-wind hybrid hydrogen production system is to utilize water electrolysis for hydrogen generation, and the produced excess electricity will be transmitted and sold by the connected power grid. In this regard, the economic analysis of hydrogen production, specifically LCOH, serves as a vital metric for assessing the economic viability of the solar-wind hybrid hydrogen production system, as expressed by Eq. 8 (Almutairi et al., 2021):
where IIC and OM means the initial investment cost and operation and maintenance costs, respectively. RS is the residual value of fixed assets. y is the lifetime from the project. f is the inflation rate and i is the interest rate of 8%. L and MH2 are the lifetime and the mass of hydrogen production.
The internal rate of return (IRR) is used to measure the investment efficiency and also reflect the project profitability, which can be represented by Eq. 9 (Emrani et al., 2022).
where CI and CO are cash inflows and cash flow.
Payback Period (PP) is introduced to evaluate the project financial investment recovery ability, and the dynamic investment of pay-back period can be calculated by Eq. 10
where θ is the number of years in which the cumulative net cash flow of each year is positive or zero for the first time.
The total investment profit rate (ROI) indicates the profitability of the total investment of the project, which can be calculated by Eq. 11
where EPIT is annual earnings before interest and tax, and TI means the total investment of the project.
3.2.2 Environmental evaluation
The utilization of renewable energy sources, such as wind or hydrogen, in lieu of fossil fuels for electricity and hydrogen supply, leads to a reduction in fossil fuel consumption and the consequent pollutant emissions, particularly carbon dioxide. In this study, the emission coefficient is employed to evaluate CO2 emission reduction.
The solar-wind hybrid power generation systems are grid-connected, operating within the permissible limits set by the grid. The electricity generated through these systems contributes to a decrease in CO2 emissions. In order to investigate the carbon emission of this system, the carbon emission reduction CERWT_PV is defined as Eq. 12 (Pan et al., 2021; Wang et al., 2023).
where EFele is the carbon emission factor of grid. DWT_grid and DPV_grid means the on-grid electricity of wind and solar power generation during the operation period and Dgrid_AE is the down-grid electricity to AE.
The hydrogen produced by the solar-wind hybrid hydrogen production system is characterized by its absence of CO2 emissions upon combustion. The output of per cubic meter hydrogen needs to consume 4.5–5.5 kWh of electricity, and the carbon reduction benefit of hydrogen production will be quantified by comparing the equivalent electricity consumption of the power grid for the same amount of hydrogen. (Rezaei et al., 2018). Therefore, the carbon emission reduction of the hydrogen produced CERH2 can be calculated by Eq 13.
where WH2 is electricity consumed for the unit of hydrogen production, and ρH2 is the density of hydrogen.
Green hydrogen ratio (GHR) measures the proportion of green hydrogen in the system, indicating the amount of renewable energy in the system, which can be expressed as Eq. 14
3.2.3 Evaluation of energy efficiency
The utilization of renewable energy during the operation of the electrolytic cell can be measured by abandoned energy power rate (AEPR), with the expression of:
During the real-time operation of the electrolytic cell, the dynamic performance of the cell is assessed based on the electrolytic cell load rate. The electrolytic cell load rate is calculated as Eq. 16:
where ΔG is the Gibbs free energy of the electrochemical reaction.
4 Capacity optimization configuration model based on NSGA-III
To meet the hydrogen production requirements and ensure the efficient solar-wind hybrid hydrogen generation, an operation strategy that satisfies the load operation is essential. In addition, the NSGA-III multi-objective optimization algorithm is used to optimize the capacity configuration.
4.1 Optimization of objects
With the target annual hydrogen output capacity of 20,000 tons, the solar-wind hybrid hydrogen production system defines the optimization objective based on the comprehensive performance evaluation criteria. The following contents are taken as the optimization objectives: (1) minimizing the levelized cost of hydrogen (LCOH) to improve the economy of the system; (2) maximizing the carbon emission reduction (CER) to reduce environmental impact and increase hydrogen production; (3) minimizing the abandoned energy power rate (AEPR) of wind and solar energy to improve the utilization of renewable energy. These objectives are formulated as the objective functions for optimization, while various constraints are employed to ensure system stability. The optimization algorithm, specifically the NSGA-III algorithm, is employed to solve the comprehensive optimization of the system. Additionally, to comply with the requirements of the NSGA-III algorithm, the maximization of CER is transformed into the minimization of the reciprocal of CER. Therefore, the optimization goal is expressed by Eq. 17
4.2 Decision variables and constraints
According to the objective function, the rated capacity of wind turbines, photovoltaic arrays, electrolyzers, batteries, and hydrogen storage tanks are selected as decision optimization variables, and expressed by Eq. 18
where EWT, EPV and EAE are the construction scale capacity of wind turbine, photovoltaic array and alkaline electrolyzer cell, respectively.
In order to improve the system reliability, the following constraints should be satisfied. During operation, it is imperative for the system to uphold law of conservation of energy, ensuring that power input and output adhere to the following balance constraints:
where Paban is the abandoned power of solar-wind power generation.
Energy storage state constraints: ensuring that the pressure in the hydrogen storage tank remains within operational requirements. Battery charge and discharge constraints: maintaining the state of charge within the desired range. These constraints are expressed as Eq. 20
where JHT_min and JHT_max mean the upper and lower pressure constraint values of hydrogen storage tank. SOCess_min and SOCess_max are the upper and lower limits of the battery state of charge.
Power operation constraints: during the system operation, it is necessary to ensure the service life of the electrolyzer and the safe transmission of the power grid. Therefore, the power operation constraints of the electrolyzer and the transmission power constraints of the power grid line can be expressed as Eq. 21
where PAE_min and PAE_max are the upper and lower constraint values of the power of the electrolytic cell, respectively. Pgrid_min, Pgrid_max are the transmission power limits of power down and power up.
4.3 NSGA-III algorithm
The capacity configuration optimization of solar-wind hybrid hydrogen production system is a multi-objective and multi-constrained optimization problem. Therefore, the Non-dominated Sorting Genetic Algorithm (NSGA) III algorithm is adopted. Compared with the NSGA-II algorithm, NSGA-III uses widely distributed reference points to maintain the diversity of decision variables, which reduces the time complexity of the algorithm and improves the effect on high-dimensional problems (Sharma et al., 2023). The specific algorithm optimization process is shown in Figure 3.
(1) Initialize the scale of wind turbines, photovoltaic arrays, alkaline electrolyzers, batteries, hydrogen storage tanks and other equipment to form a population of N, form initial variables and select reference points on the hyperplane;
(2) According to the operation strategy of the grid-connected solar-wind hydrogen production system, and initialize the population;
(3) The optimal individual is selected by the tournament method, and the progeny individual is generated through crossover and variation, and different non-dominated layers are obtained by further non-dominated sorting;
(4) Normalize the population operation and the individuals in the critical layer are associated with the reference line according to the reference point;
(5) According to the number of individuals associated with the reference line, the population individuals are selected from the critical dominant layer to enter the new generation of parents, and the number of iterations is added by 1;
(6) Repeat operation (2)–(5) until the number of iterations reaches the maximum number of iterations.
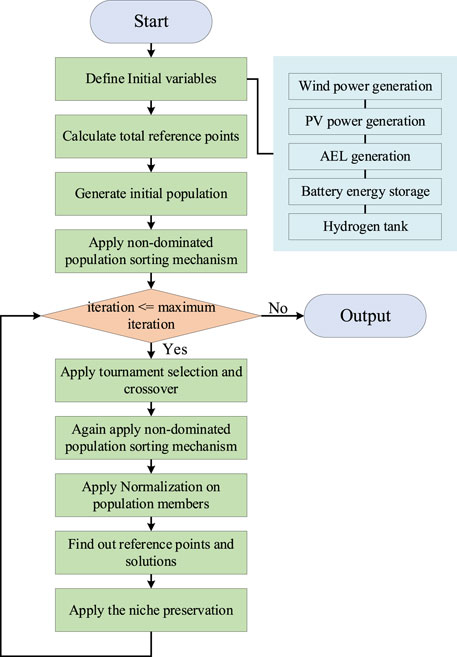
FIGURE 3. To accurately configure the optimal capacity of the system, the NSGA-III algorithm is employed with AEPR, LCOH, and CDER serving as the optimization objectives. The decision variables in this optimization process are the capacity configuration of each equipment within the system.
The economic parameters of the solar-wind green hybrid hydrogen production system in this study including the construction costs, operation costs, and other relevant economic factors associated with the main equipment, are presented in Table 1. In addition, in order to ensure the rationality of the system simulation and capacity configuration optimization process, the technical and operational parameters of each individual device are kept within the design range, as shown in Table 2. Among them, the maximum pressure of one hydrogen tank volume is 5 MPa, and the maximum SOC of one battery is 0.9.
5 Results and discussion
The solar-wind hybrid hydrogen production system enhances the competitiveness of solar and wind energy. In order to improve the thermodynamics and economics potential, the capacity of the solar-wind hybrid hydrogen production system and its dynamic operation characteristics need to be optimized and investigated.
5.1 Analysis of multi-objective optimal capacity optimization results
The meteorological data from a specific region in Taonan, Jilin Province of China is utilized as input for the configuration optimization. The wind speed and solar irradiation data for the area are sampled at hourly intervals over the course of 1 year. Wind speed and solar irradiation are crucial parameters for assessing wind power and solar power potential. The variation trends of these parameters are depicted in Figure 4. It reveals that there is a certain complementarity between wind and insolation, which is better between summer and autumn of resources. Further calculation and analysis of cases are performed based on this weather data.
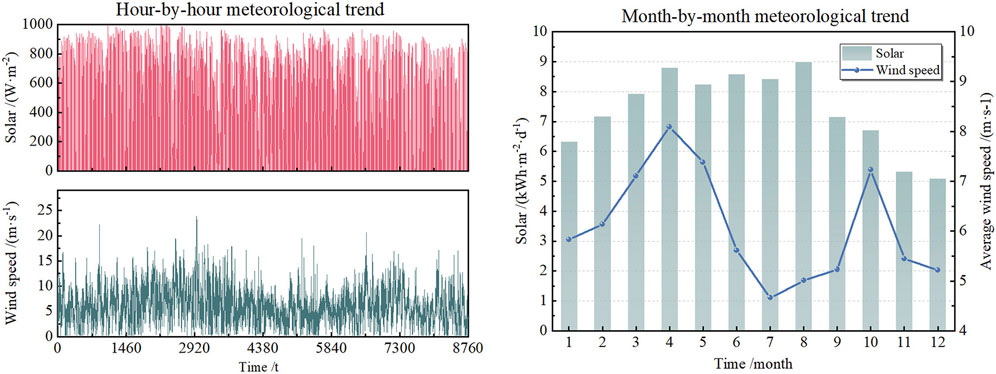
FIGURE 4. To quantitatively assess solar and wind resources in Taonan area of China Jilin Province, the hourly wind speed and solar irradiance data for a specific year were collected and analyzed, to further understand the complementary utilization of wind and solar resources, the monthly variations in wind and solar resources were statistically compared.
Aiming at the grid-connected solar-wind hybrid hydrogen production system, the NSGA-III algorithm is employed to address the capacity optimization configuration problem. The optimization objectives include the levelized cost of hydrogen, the reciprocal of carbon dioxide emission reduction, and the rate of wind and solar curtailment. Considering the diversity and operation of the solution set, the population size is set to 500, and the number of iterations is 100 generations. The resulting optimal solution set is illustrated in Figure 5, the Pareto surface exhibits clear patterns, and the distribution of the target solutions appears wide and uniform, indicating that the distribution of the solution set has diversity.
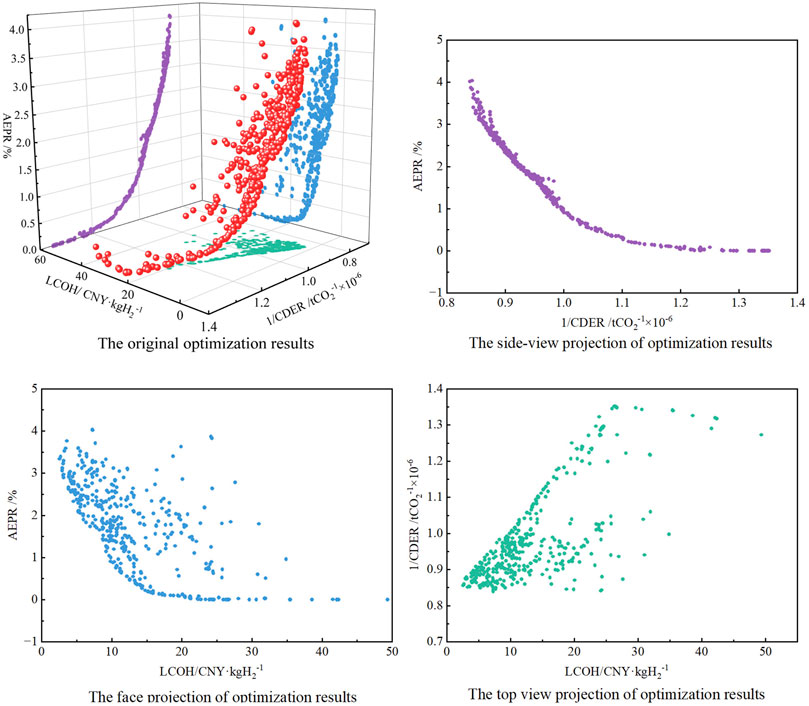
FIGURE 5. The optimization results of the capacity configuration were obtained using the NSGA-III algorithm, with a population size of 500 and 100 generations of iterations. To determine the optimal capacity configuration under multi-objective optimization, it is necessary to comprehensively consider the system in the AEPR, CDER and LCOH, the performance of AEPR, CDER and LCOH in the multi-objective capacity configuration are evaluated.
Based on the diversity scheme resulting from the capacity configuration optimization, a final optimization scheme can be selected through analysis. As for the side-view projection of optimization results, it is a curve depicting the reciprocal of carbon emission reduction and the rate of wind and solar curtailment, showing a clear negative correlation trend. Specifically, there is a positive correlation between carbon emission reduction and the rate of wind and solar curtailment. As the rate of wind and solar curtailment decreases, the carbon emission reduction also decreases. This trend indicates that although reducing the rate of solar and wind curtailment allows for increased utilization of renewable energy, it results in a decrease in the overall carbon emission reduction, reflecting a reduction in the overall level of comprehensive accessibility and carbon emission reduction.
Based on the face projection of optimization results and the top view projection of optimization results, it can be seen that among the schemes with lower LCOH, there are variations in the wind and solar curtailment rate and carbon emissions, but the three optimization objectives exhibit a non-linear relationship with each other. Consequently, a single scheme cannot simultaneously achieve the optimal solution for all three objectives. Therefore, a weighting method is employed to select the scheme. Firstly, the scheme is preliminarily selected based on the requirement of the solar-wind hybrid hydrogen production system, that is, f ≤ [25, 1.0 × 10−6, 5]. Then, the solution of the Pareto solution sets are normalized. Finally, the weighted method is used to get the final scheme. Given the significance of economy in the design process, a weight ω = [0.6, 0.2, 0.2] is applied to sort and select normalized schemes. By calculating the fitness of the solution in the Pareto solution set, the scheme with the minimum fitness is selected as the design scheme. Finally, the corresponding objective function values are f = [16.69, 9.76 × 10−7, 3.32], and the configuration of each device is shown in Table 3. Through the NSGA-III algorithm, the capacity configuration scheme for the solar-wind hybrid hydrogen production system is determined based on these three categories of indicators.
5.2 Performance analysis of solar-wind hybrid hydrogen production system
Through the application of the multi-objective optimization algorithm, an optimized scheme for solar-wind hybrid hydrogen production has been obtained. Based on the capacity optimization configuration results, a comprehensive analysis of the scheme is conducted using various performance indicators. The calculation results are presented in Table 4. Notably, the LCOH is determined to be 16.69 CNY/kgH2, and Initial cost is 5.60×109 CNY. To ensure economic viability, a recommended sales price of 27.80 CNY/kgH2 is proposed, along with a payback period of 12.18 years and a total investment profit rate of 10.60%.
Otherwise, under this specific capacity configuration scale, the system demonstrates notable environmental performance indicators, with a CER of 1.02×106 tCO2. A predominant contribution of renewable energy sources and the grid primarily serves the role of load regulation, resulting in a high proportion of 98.51% for green hydrogen ratio, further enhancing its environmental friendliness. Concerning energy utilization parameters, the wind and solar energy curtailment rate is recorded at 3.32%, indicating satisfactory utilization of scenic power resources. Moreover, the average load factor of the electrolyzer stands at 64.77%, falling within the conventional range for electrolyzers. Thus, it is evident that the proposed capacity configuration method yields a comprehensive and well-performing solution for a solar-wind hybrid hydrogen production system, meanwhile to further enhance the economic benefits, as the cost of related equipment decreases. Therefore, it is crucial to emphasize the carbon reduction advantages of green hydrogen production and integrate them into the overall economic evaluation of the project through measures like carbon taxation and carbon trading. This would ultimately contribute to the reduction of green hydrogen production costs.
In the solar-wind hybrid hydrogen production design scheme, the initial investment cost plays a significant role, as depicted in Figure 6. To ensure the stability of hydrogen production in the electrolytic cell and mitigate the fluctuations of wind and solar energy, an ample amount of energy storage equipment is employed. Among these, the battery construction cost constitutes 27.34% of the total cost, while the hydrogen storage equipment exhibits high capacity, the unit equipment cost is low, accounting for only 0.33% of the total construction cost. The construction cost distribution reveals that the photovoltaic, wind turbine, and electrolyzer components contribute 36.99%, 12.66% and 22.68%, respectively. The electricity utilized for hydrogen production is predominantly sourced from wind power, photovoltaic, and the grid. Specifically, wind power and photovoltaic sources contribute 23.66% and 73.02%, while the grid occupies a marginal portion of 3.32%. This distribution underscores the substantial reliance on solar and wind energy sources in providing energy for the system solution, resulting in predominantly green hydrogen production.
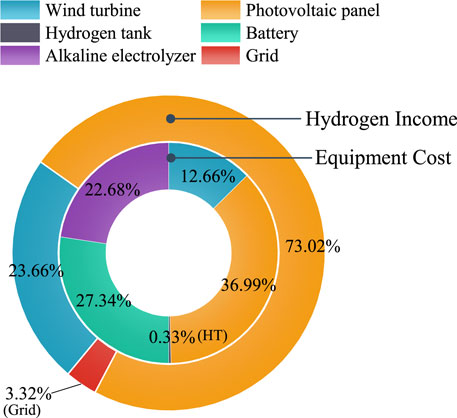
FIGURE 6. To assess the initial investment of the solar-wind hybrid hydrogen production system, the initial investment combined with the capacity configuration optimization results. of each major equipment and the distribution of the hydrogen consumption electricity sources were calculated.
Due to the variations of solar-wind resources throughout the year, the monthly solar-wind power generation and hydrogen production levels differ. Therefore, Figure 7 illustrates the calculated monthly solar-wind power generation and the hydrogen production load of the electrolytic cell. Compared with the two electricity generation, solar-wind power is the main power supply of the system. Concurrently, the power generation of photovoltaic and wind power is the highest in April, which is 1.17 × 105 MWh and 5.76 × 104 MWh respectively, while the power generation is the lowest in November and December. In contrast, the monthly load of the electrolytic cell remained basically stable in the range of 9.06×104–1.03×105 MWh. In order to maintain the hydrogen production and solar-wind utilization rate of the electrolytic cell, as for the transmission power, when the power generation is sufficient, the excess power is connected to the grid, and the maximum power on the grid in April is 6.35×104 MWh. Concurrently, it supplies power to the electrolytic cell when it is insufficient, and the maximum power supply in December is 8.65×103 MWh.
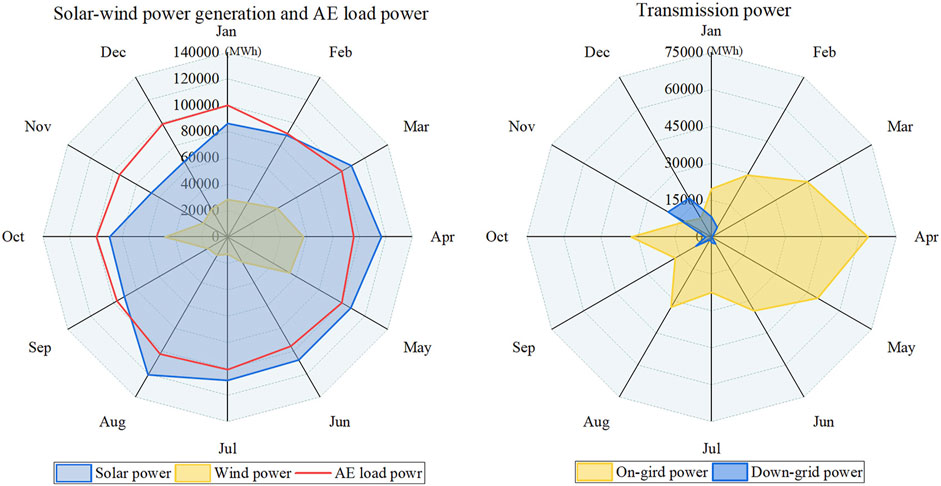
FIGURE 7. Due to the difference of wind and solar resources in each month, the wind and solar power generation and the corresponding electrolytic tank load power in different months are analyzed, and the power grid is used as the power regulation method of the system. Further analysis of the monthly power grid up and down.
Further comparing the hydrogen production for each month, as shown in Figure 8, the hydrogen production is consistent with the trend of the load power of the electrolytic cell. The highest hydrogen production in March is 1,713 tons, and the lowest hydrogen production in February is 1,536 tons.
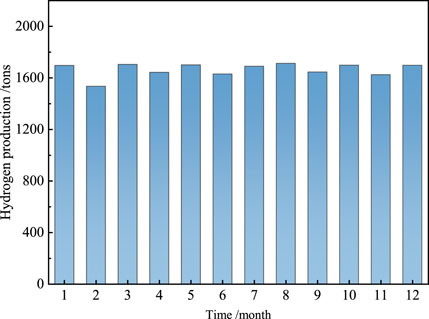
FIGURE 8. In order to analyze the hydrogen production under different solar-wind resources in different months, the changes of hydrogen production in different months were compared.
Moreover, the annual economic cost recovery relies primarily on the output of electrolytic hydrogen production and grid-connected solar-wind hybrid power generation. Thus, a detailed analysis of daily electrolytic hydrogen production and solar-wind hybrid power generation throughout the year is conducted, as illustrated in Figure 9. The average daily hydrogen production is 54.76 tons of H2, the amount of hydrogen produced per day exhibits lower fluctuations around the average. Simultaneously, as the solar-wind hybrid power generation is capable of fulfilling the hydrogen production capacity, the surplus power is integrated into the grid. According to the power integration, the average daily online power is 1,007 MWh, accounting for 8.92% of the total solar and wind hybrid power generation, thereby further enhancing the utilization rate of solar and wind power. However, seasonal variations have an impact, the daily grid-connected power generation fluctuates significantly.
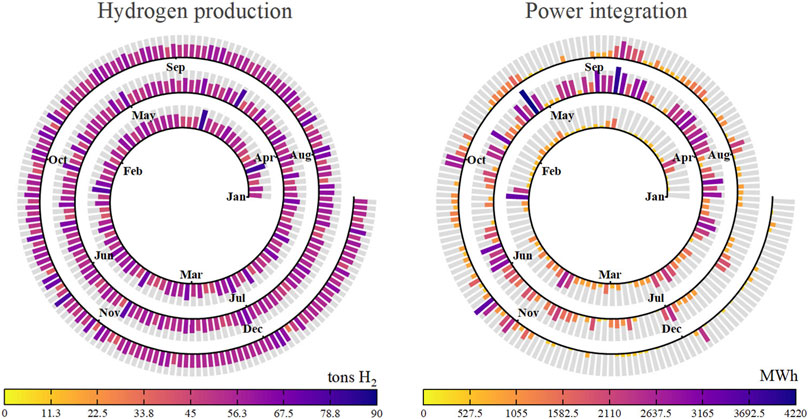
FIGURE 9. The annual economic cost recovery is a crucial factor in evaluating the performance of the system. It can be divided into two main components. The first component is the annual benefit derived from hydrogen production. To analyze this, the daily hydrogen production capacity of the system was assessed. The second component involves analyzing the daily electricity sales of the system. This can be examined by assessing the annual grid connection volume of the electrolytic cell hydrogen production and solar-wind hybrid power generation.
5.3 Dynamic operation analysis of solar-wind hybrid hydrogen production system
For the solar-wind hybrid hydrogen production system with the selected capacity configuration scheme, dynamic operation analysis is performed on typical weeks representing each month by the seasons.
The power generation profiles of wind power and photovoltaic systems during these weeks, highlighting their complementary characteristics. The dynamic operation analysis of the system is carried out for the typical weeks of the specific months of season. The dynamic behaviors observed during three typical weeks in spring, and three typical weeks in summer, as shown in Figure 10, exhibit similar patterns. Notably, there is a favorable complementarity between PV and wind power generation. SOC of the energy storage device remains relatively stable, while SOH experiences significant fluctuations. This is attributed to the ample availability of solar and wind power generation, causing the battery to reach its upper limit of energy storage. Consequently, the primary objective of ensuring a stable hydrogen supply is primarily achieved through the utilization of hydrogen storage equipment. This operational strategy prioritizes the adjustment of the hydrogen output rate and the smoothing of hydrogen production fluctuations through hydrogen storage equipment. Additionally, the charging and discharging of the battery are employed to regulate the electrolytic hydrogen production rate, further enhancing the stability of the solar and wind complementary hydrogen production system.
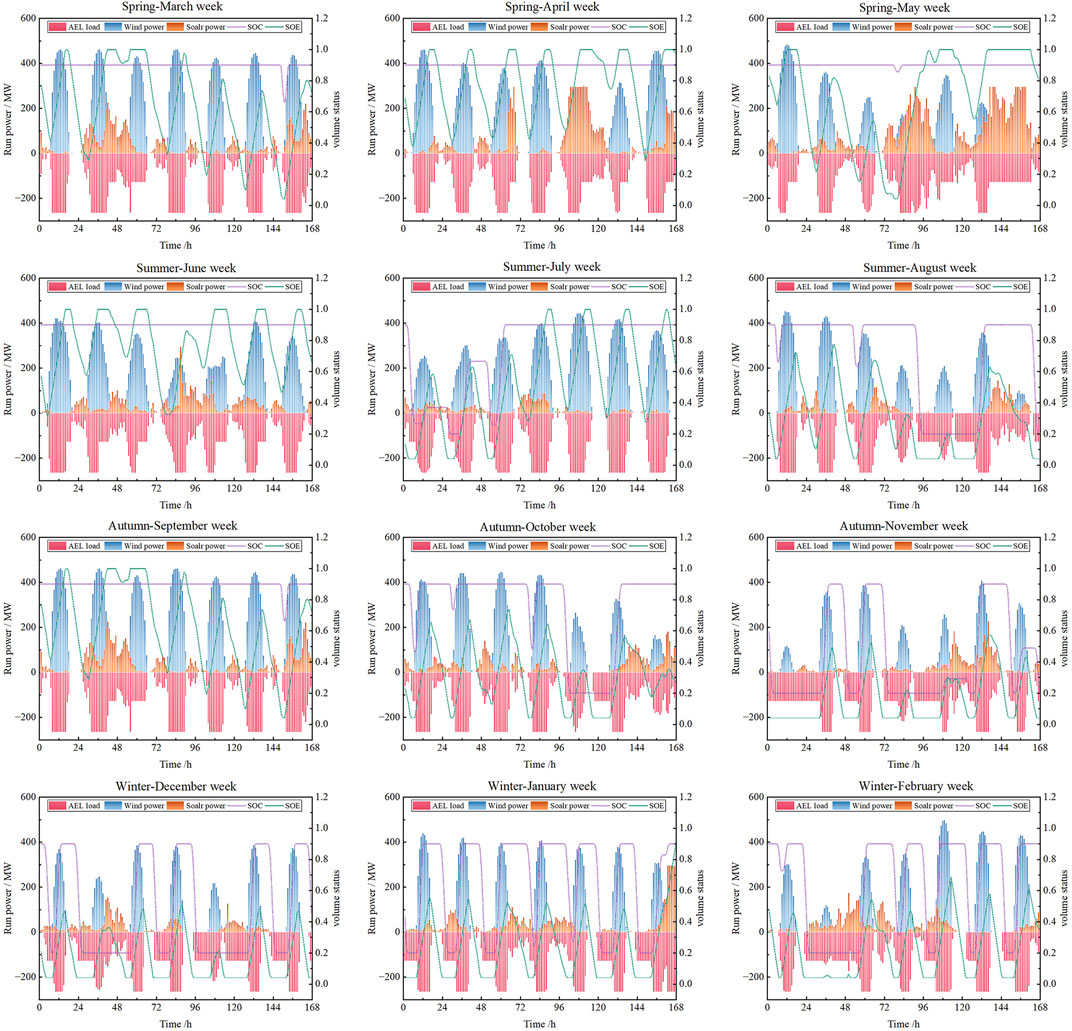
FIGURE 10. The fluctuations of the solar-wind will affect the stable operation of the energy storage device, so the key to formulating a scheduling strategy that matches the volatility is to accurately evaluate the year-round complementarity of the solar-wind, the solar-wind complementarity of typical weeks in four seasons of spring, summer, autumn, winter is evaluated.
In the autumn typical weeks and the winter typical weeks shown in Figure 10, both wind and PV power generation are comparatively lower, with less noticeable complementarity between the two. The generation capacity of wind and solar power is significantly lower during the winter typical weeks. In contrast to typical weeks in spring and summer, batteries and hydrogen storage devices are now adjusted more frequently. Additionally, there are instances where the steady hydrogen output criteria cannot be met by energy storage and wind and solar power generation. In these situations, the electrolyzer receives power from the power grid to meet the standard. In this system, the grid can effectively control the load power of the electrolyzer, ensure a consistent output of hydrogen, and absorb solar power generation, increasing the rate at which solar power is utilized.
However, due to the region’s superior photovoltaic resources compared to wind resources, the former generates more electricity than the latter. The calculations reveal that during typical weeks in spring and summer, the average power generation of photovoltaic is 24,208 MWh and 26,589 MWh, respectively, which is much higher than the average power generation of photovoltaic typical weeks in autumn and summer (17,135 MWh and 17,022 MWh), while during the typical weeks in summer, the average power generation of wind power is 10,425 MWh, which is much higher than the average power generation of typical weeks in other seasons (3,451 MWh in spring, 4,298 MWh in autumn and 4,575 MWh in winter). Despite notable variability in the power generation capacity of power throughout different seasons, the electrolytic hydrogen production system can ensure basic operation through the regulation of energy storage devices and grid infrastructure. Its typical weekly load power remains stable, reaching 22,766 MWh, 24,208 MWh, 23,171 MWh and 22,123 MWh across all seasons, thereby guaranteeing the consistent hydrogen production.
As mentioned above, in order to achieve stable hydrogen output, according to the dynamic operation strategy, the smoothing ability of low-cost hydrogen storage equipment in wind and solar output scenarios is first fully utilized. This reduces the use of the battery and the frequency of charge and discharge, thereby improving its operating life. At the same time, combined with the power grid to adjust, it also ensures the system power balance and meets the load demand of electrolytic water hydrogen production. Consequently, this solar-wind hybrid hydrogen production strategy is well-suited to leverage its advantages in large-scale hydrogen production scenarios.
6 Conclusion
This study focuses on optimizing the capacity configuration of a solar-wind green hybrid hydrogen production system using the NSGA-III algorithm with the goal of achieving a comprehensive index. The main conclusions are summarized as follows:
(1) The capacity configuration optimization of a solar-wind hybrid hydrogen production system can be achieved by employing the NSGA-III algorithm and the optimization method of comprehensive performance objective function. The resulting solution set offers a diverse distribution, enabling the selection of a design scheme that meets the design requirements in a comprehensive manner.
(2) This study determined the multi-index optimal scheme using a specific method. With the addition income of surplus solar/wind power by transmitting to the grid, the internal rate of return of the system reaches to 8% when the hydrogen sale price is suggested to 27.80 CNY/kgH2. Additionally, this system achieves the carbon emission reduction of 1.02×106 tCO2, and the abandoned energy power rate reduced to 3.32%.
(3) Through the cooperative hydrogen production strategy proposed in this paper, the demand for hydrogen production of 20,000 tons per year can be met. At the same time, the adjustment ability of hydrogen storage equipment and battery is fully utilized. Finally, the stability of hydrogen output is improved, and the hydrogen production load of electrolytic water in the typical cycle of four seasons is within the range of 22,123 MWh-24,208 MWh.
This study reveals that the capacity configuration method of solar-wind hybrid hydrogen production based on comprehensive performance index can meet the demand of large-scale hydrogen production throughout the year, and provide technical and methodological suggestions and guidance for the formulation of solar-wind hydrogen production scheme with favorable comprehensive performance.
Data availability statement
The original contributions presented in the study are included in the article/Supplementary Material, further inquiries can be directed to the corresponding author.
Author contributions
WS: Conceptualization, Investigation, Writing–original draft. WZ: Formal Analysis, Investigation, Writing–original draft. QL: Formal Analysis, Investigation, Visualization, Writing–original draft. ZY: Investigation, Software, Writing–review and editing. YH: Formal Analysis, Investigation, Writing–original draft. ZB: Investigation, Writing–review and editing.
Funding
The author(s) declare financial support was received for the research, authorship, and/or publication of this article. The authors appreciate the financial support provided by Shandong Provincial Natural Science Foundation of China (ZR2022YQ58), and the Fundamental Research Funds for the Central Universities (No. 22CX07006A).
Conflict of interest
WS, WZ, and ZY were employed by the Company Powerchina Huadong Engineering Corporation Limited.
The remaining authors declare that the research was conducted in the absence of any commercial or financial relationships that could be construed as a potential conflict of interest.
Publisher’s note
All claims expressed in this article are solely those of the authors and do not necessarily represent those of their affiliated organizations, or those of the publisher, the editors and the reviewers. Any product that may be evaluated in this article, or claim that may be made by its manufacturer, is not guaranteed or endorsed by the publisher.
References
Acosta-Silva, Y. J., Torres-Pacheco, I., Matsumoto, Y., Toledano-Ayala, M., Soto-Zarazúa, G. M., Zelaya-Ángel, O., et al. (2019). Applications of solar and wind renewable energy in agriculture: a review. Sci. Prog. 102 (2), 127–140. doi:10.1177/0036850419832696
Al-Buraiki, A. S., and Al-Sharafi, A. (2022). Hydrogen production via using excess electric energy of an off-grid hybrid solar/wind system based on a novel performance indicator. Energy Convers. Manag. 254, 115270. doi:10.1016/j.enconman.2022.115270
Al-Ghussain, L., Ahmad, A. D., Abubaker, A. M., Hovi, K., Hassan, M. A., and Annuk, A. (2023). Techno-economic feasibility of hybrid PV/wind/battery/thermal storage trigeneration system: toward 100% energy independency and green hydrogen production. Energy Rep. 9, 752–772. doi:10.1016/j.egyr.2022.12.034
Almutairi, K., Hosseini Dehshiri, S. S., Hosseini Dehshiri, S. J., Mostafaeipour, A., Jahangiri, M., and Techato, K. (2021). Technical, economic, carbon footprint assessment, and prioritizing stations for hydrogen production using wind energy: a case study. Energy Strategy Rev. 36, 100684. doi:10.1016/j.esr.2021.100684
Amry, Y., Elbouchikhi, E., Le Gall, F., Ghogho, M., and El Hani, S. (2023). Optimal sizing and energy management strategy for EV workplace charging station considering PV and flywheel energy storage system. J. Energy Storage 62, 106937. doi:10.1016/j.est.2023.106937
Behzadi, A., and Sadrizadeh, S. (2023). A rule-based energy management strategy for a low-temperature solar/wind-driven heating system optimized by the machine learning-assisted grey wolf approach. Energy Convers. Manag. 277, 116590. doi:10.1016/j.enconman.2022.116590
Chaichan, W., Waewsak, J., Nikhom, R., Kongruang, C., Chiwamongkhonkarn, S., and Gagnon, Y. (2022). Optimization of stand-alone and grid-connected hybrid solar/wind/fuel cell power generation for green islands: application to koh samui, southern Thailand. Energy Rep. 8, 480–493. doi:10.1016/j.egyr.2022.07.024
Deymi-Dashtebayaz, M., Baranov, I. V., Nikitin, A., Davoodi, V., Sulin, A., Norani, M., et al. (2022). An investigation of a hybrid wind-solar integrated energy system with heat and power energy storage system in a near-zero energy building-A dynamic study. Energy Convers. Manag. 269, 116085. doi:10.1016/j.enconman.2022.116085
Emrani, A., Berrada, A., Arechkik, A., and Bakhouya, M. (2022). Improved techno-economic optimization of an off-grid hybrid solar/wind/gravity energy storage system based on performance indicators. J. Energy Storage 49, 104163. doi:10.1016/j.est.2022.104163
Fang, R., and Liang, Y. (2019). Control strategy of electrolyzer in a wind-hydrogen system considering the constraints of switching times. Int. J. Hydrogen Energy 44 (46), 25104–25111. doi:10.1016/j.ijhydene.2019.03.033
Fosso Tajouo, G., Tiam Kapen, P., and Djanna Koffi, F. L. (2023). Techno-economic investigation of an environmentally friendly small-scale solar tracker-based PV/wind/Battery hybrid system for off-grid rural electrification in the mount bamboutos, Cameroon. Energy Strategy Rev. 48, 101107. doi:10.1016/j.esr.2023.101107
Graça Gomes, J., Jiang, J., Chong, C. T., Telhada, J., Zhang, X., Sammarchi, S., et al. (2023). Hybrid solar PV-wind-battery system bidding optimisation: A case study for the iberian and Italian liberalised electricity markets. Energy 263, 126043. doi:10.1016/j.energy.2022.126043
Han, Y., Shi, K., Qian, Y., and Yang, S. (2023). Design and operational optimization of a methanol-integrated wind-solar power generation system. J. Environ. Chem. Eng. 11 (3), 109992. doi:10.1016/j.jece.2023.109992
Hussain, B., Asif Ali Naqvi, S., Anwar, S., and Usman, M. (2023). Effect of wind and solar energy production, and economic development on the environmental quality: is this the solution to climate change? Gondwana Res. 119, 27–44. doi:10.1016/j.gr.2023.01.012
Hutchinson, A. J., and Gladwin, D. T. (2023). Capacity factor enhancement for an export limited wind generation site utilising a novel Flywheel Energy Storage strategy. J. Energy Storage 68, 107832. doi:10.1016/j.est.2023.107832
Izadi, A., Shahafve, M., and Ahmadi, P. (2022). Neural network genetic algorithm optimization of a transient hybrid renewable energy system with solar/wind and hydrogen storage system for zero energy buildings at various climate conditions. Energy Convers. Manag. 260, 115593. doi:10.1016/j.enconman.2022.115593
Jaber, J. O., Jaber, Q. M., Sawalha, S. A., and Mohsen, M. S. (2008). Evaluation of conventional and renewable energy sources for space heating in the household sector. Renew. Sustain. Energy Rev. 12 (1), 278–289. doi:10.1016/j.rser.2006.05.004
Kakavand, A., Sayadi, S., Tsatsaronis, G., and Behbahaninia, A. (2023). Techno-economic assessment of green hydrogen and ammonia production from wind and solar energy in Iran. Int. J. Hydrogen Energy 48 (38), 14170–14191. doi:10.1016/j.ijhydene.2022.12.285
Kamani, D., and Ardehali, M. M. (2023). Long-term forecast of electrical energy consumption with considerations for solar and wind energy sources. Energy 268, 126617. doi:10.1016/j.energy.2023.126617
Kiehbadroudinezhad, M., Merabet, A., Rajabipour, A., Cada, M., Kiehbadroudinezhad, S., Khanali, M., et al. (2022). Optimization of wind/solar energy microgrid by division algorithm considering human health and environmental impacts for power-water cogeneration. Energy Convers. Manag. 252, 115064. doi:10.1016/j.enconman.2021.115064
Kumar, J, C. R., and Majid, M. A. (2020). Renewable energy for sustainable development in India: current status, future prospects, challenges, employment, and investment opportunities. Energy, Sustain. Soc. 10 (1), 1–36. doi:10.1186/s13705-019-0232-1
Lim, T., and Kim, S.-K. (2022). Non-precious hydrogen evolution reaction catalysts: stepping forward to practical polymer electrolyte membrane-based zero-gap water electrolyzers. Chem. Eng. J. 433, 133681. doi:10.1016/j.cej.2021.133681
Liu, L., Zhai, R., and Hu, Y. (2023). Performance evaluation of wind-solar-hydrogen system for renewable energy generation and green hydrogen generation and storage: energy, exergy, economic, and enviroeconomic. Energy 276, 127386. doi:10.1016/j.energy.2023.127386
Lu, J., Li, M., and Li, Q. (2023). Modeling and optimal design of a grid-independent solutions based on solar-hydrogen storage feeding green building by optimization algorithm. J. Energy Storage 62, 106844. doi:10.1016/j.est.2023.106844
Lv, X., Li, X., and Xu, C. (2023). A robust optimization model for capacity configuration of PV/battery/hydrogen system considering multiple uncertainties. Int. J. Hydrogen Energy 48 (21), 7533–7548. doi:10.1016/j.ijhydene.2022.11.220
Nasrabadi, A. M., and Korpeh, M. (2023). Techno-economic analysis and optimization of a proposed solar-wind-driven multigeneration system; case study of Iran. Int. J. Hydrogen Energy 48 (36), 13343–13361. doi:10.1016/j.ijhydene.2022.12.283
Pan, G., Gu, W., Hu, Q., Wang, J., Teng, F., and Strbac, G. (2021). Cost and low-carbon competitiveness of electrolytic hydrogen in China. Energy & Environ. Sci. 14 (9), 4868–4881. doi:10.1039/d1ee01840j
Praveenkumar, S., Agyekum, E. B., Ampah, J. D., Afrane, S., Velkin, V. I., Mehmood, U., et al. (2022). Techno-economic optimization of PV system for hydrogen production and electric vehicle charging stations under five different climatic conditions in India. Int. J. Hydrogen Energy 47 (38), 38087–38105. doi:10.1016/j.ijhydene.2022.09.015
Prestat, M. (2023). Corrosion of structural components of proton exchange membrane water electrolyzer anodes: a review. J. Power Sources 556, 232469. doi:10.1016/j.jpowsour.2022.232469
Rezaei, M., Mostafaeipour, A., Qolipour, M., and Arabnia, H. R. (2018). Hydrogen production using wind energy from sea water: a case study on southern and northern coasts of Iran. Energy & Environ. 29 (3), 333–357. doi:10.1177/0958305x17750052
Roesch, M., Bauer, D., Haupt, L., Keller, R., Bauernhansl, T., Fridgen, G., et al. (2019). Harnessing the full potential of industrial demand-side flexibility: an end-to-end approach connecting machines with markets through service-oriented IT platforms. Appl. Sci. 9 (18), 3796. doi:10.3390/app9183796
Sharma, H., Mandil, G., Monnier, É., Cor, E., and Zwolinski, P. (2023). Sizing a hybrid hydrogen production plant including life cycle assessment indicators by combining NSGA-III and principal component analysis (PCA). Energy Convers. Manag. X 18 (13), 100361. doi:10.1016/j.ecmx.2023.100361
Shen, Y., Li, X., Wang, N., Li, L., and Hoseyni, A. (2021). Introducing and investigation of a pumped hydro-compressed air storage based on wind turbine and alkaline fuel cell and electrolyzer. Sustain. Energy Technol. Assessments 47, 101378. doi:10.1016/j.seta.2021.101378
Shin, H., Jang, D., Lee, S., Cho, H.-S., Kim, K.-H., and Kang, S. (2023). Techno-economic evaluation of green hydrogen production with low-temperature water electrolysis technologies directly coupled with renewable power sources. Energy Convers. Manag. 286, 117083. doi:10.1016/j.enconman.2023.117083
Shiva Kumar, S., and Lim, H. (2022). An overview of water electrolysis technologies for green hydrogen production. Energy Rep. 8, 13793–13813. doi:10.1016/j.egyr.2022.10.127
Song, S., Lin, H., Sherman, P., Yang, X., Chen, S., Lu, X., et al. (2022). Deep decarbonization of the Indian economy: 2050 prospects for wind, solar, and green hydrogen. iScience 25 (6), 104399. doi:10.1016/j.isci.2022.104399
Superchi, F., Papi, F., Mannelli, A., Balduzzi, F., Ferro, F. M., and Bianchini, A. (2023). Development of a reliable simulation framework for techno-economic analyses on green hydrogen production from wind farms using alkaline electrolyzers. Renew. Energy 207, 731–742. doi:10.1016/j.renene.2023.03.077
Tang, O., Rehme, J., and Cerin, P. (2022). Levelized cost of hydrogen for refueling stations with solar PV and wind in Sweden: on-grid or off-grid? Energy 241, 122906. doi:10.1016/j.energy.2021.122906
Teferra, D. M., Ngoo, L. M. H., and Nyakoe, G. N. (2023). Fuzzy-based prediction of solar PV and wind power generation for microgrid modeling using particle swarm optimization. Heliyon 9 (1), e12802. doi:10.1016/j.heliyon.2023.e12802
Temiz, M., and Dincer, I. (2022). Development and assessment of an onshore wind and concentrated solar based power, heat, cooling and hydrogen energy system for remote communities. J. Clean. Prod. 374, 134067. doi:10.1016/j.jclepro.2022.134067
Wahbah, M., Mohandes, B., El-Fouly, T. H. M., and El Moursi, M. S. (2022). Unbiased cross-validation kernel density estimation for wind and PV probabilistic modelling. Energy Convers. Manag. 266, 115811. doi:10.1016/j.enconman.2022.115811
Wang, R., Yang, L., Wang, X., and Zhou, Y. (2023). Low carbon optimal operation of integrated energy system based on concentrating solar power plant and power to hydrogen. Alexandria Eng. J. 71, 39–50. doi:10.1016/j.aej.2023.03.038
Wang, Z., Zhang, X., and Rezazadeh, A. (2021). Hydrogen fuel and electricity generation from a new hybrid energy system based on wind and solar energies and alkaline fuel cell. Energy Rep. 7, 2594–2604. doi:10.1016/j.egyr.2021.04.060
Wappler, M., Unguder, D., Lu, X., Ohlmeyer, H., Teschke, H., and Lueke, W. (2022). Building the green hydrogen market – current state and outlook on green hydrogen demand and electrolyzer manufacturing. Int. J. Hydrogen Energy 47 (79), 33551–33570. doi:10.1016/j.ijhydene.2022.07.253
Wu, Y., and Zhang, T. (2021). Risk assessment of offshore wave-wind-solar-compressed air energy storage power plant through fuzzy comprehensive evaluation model. Energy 223, 120057. doi:10.1016/j.energy.2021.120057
Zhang, F., Wang, B., Gong, Z., Zhang, X., Qin, Z., and Jiao, K. (2023). Development of photovoltaic-electrolyzer-fuel cell system for hydrogen production and power generation. Energy 263, 125566. doi:10.1016/j.energy.2022.125566
Zhang, P., Li, Y., Tang, Y., Zhang, R., Li, H., and Gu, J. (2023). Multi-objective optimization and dynamic response predictions of an articulated offshore wind turbine. Ocean. Eng. 273, 114017. doi:10.1016/j.oceaneng.2023.114017
Nomenclature
Keywords: solar-wind hybrid power, hydrogen production, capacity optimization, comprehensive performance, NSGA-III
Citation: Su W, Zheng W, Li Q, Yu Z, Han Y and Bai Z (2023) Capacity configuration optimization for green hydrogen generation driven by solar-wind hybrid power based on comprehensive performance criteria. Front. Energy Res. 11:1256463. doi: 10.3389/fenrg.2023.1256463
Received: 10 July 2023; Accepted: 17 August 2023;
Published: 30 August 2023.
Edited by:
Jianli Zhou, Xinjiang University, ChinaReviewed by:
Jiaming He, North China Electric Power University, ChinaRui Jing, Xiamen University, China
Copyright © 2023 Su, Zheng, Li, Yu, Han and Bai. This is an open-access article distributed under the terms of the Creative Commons Attribution License (CC BY). The use, distribution or reproduction in other forums is permitted, provided the original author(s) and the copyright owner(s) are credited and that the original publication in this journal is cited, in accordance with accepted academic practice. No use, distribution or reproduction is permitted which does not comply with these terms.
*Correspondence: Zhang Bai, YmFpemhhbmdAdXBjLmVkdS5jbg==