- 1iSMART, Qingdao University of Technology, Qingdao, China
- 2Faculty of Environmental Engineering, The University of Kitakyushu, Kitakyushu, Japan
Households are an important sector in carrying out human development activities, accounting for more than 30% of the total global energy consumption. The continued growth of household energy consumption (HEC) and carbon emissions is threatening economic and environmental sustainability. This review focuses on the research in the field of HEC and conducts a bibliometric analysis of research articles from the Web of Science Core Collection since 2000. The results show that: 1) HEC research has undergone rapid development since 2014, and interdisciplinary fusion and collaborative research have become dominant trends. 2) Keyword co-occurrence analysis clearly identifies the current urgent themes, including energy demand and its determinants, environmental impact factors and assessments, and energy-saving technologies and emission reduction measures. 3) The analysis of citations reveals that economic models, such as input-output models and life cycle assessment, are frequently employed in the field of HEC. Based on a summary of household energy-saving and emissions reduction work, this paper critically discusses the limitations of existing measures such as smart home technology, sustainable energy systems, and behavioral interventions. The main directions for promoting household energy-saving development in the future are identified: including improving the security and customer engagement of smart home technology, focusing on the availability and stability of sustainable energy, and paying more attention to low-income and aging households in behavioral intervention measures. One of the important obstacles facing research is how to reduce energy management efficiency and usage costs through technology and policy.
1 Introduction
Climate change and environmental impacts caused by energy consumption and carbon emissions have raised global awareness. In response to the “Paris Agreement”, many countries or regions have developed long-term policies and action plans to reduce greenhouse gas emissions. The EU introduced the “Energy Efficiency Directive”, which aims to reduce total energy consumption, reduce emissions and respond to energy poverty (Energy Efficiency Directive, 2018). The Japanese government submitted its National Autonomous Contribution (NDC), stating that it will reduce greenhouse gas emissions by 26% compared to 2013 by 2030 (Taniguchi-Matsuoka et al., 2020). The U.S. proposed the “CLAEN Futures Act” in the 117th Congress. It sets the goal of reducing greenhouse gas emissions from the U.S. economy by 50% by 2030 compared to 2005, and by 100% by 2050 (CLEAN Future Act, 2021). As the largest developing country, China attaches great importance to addressing climate change and strengthening the implementation of the national autonomous contribution target. It has adopted “reducing CO2 emissions per unit of GDP by 18% in 2025 compared to 2020”as a binding target in the 14th Five-Year Plan (MEE, 2022). At the same time, a series of Energy Conservation and Emission Reduction Policies (ECERP) have been introduced with the aim of controlling the total amount of energy consumption, improving energy efficiency and promoting the green transformation of economic and social development (Luo et al., 2023). India has a target to reduce carbon intensity of its economy to 45% by 2030 (Amin et al., 2023).
Reducing building-related energy use is widely recognized as key to reaching these ambitious targets and facilitating the transition to a low-carbon and sustainable world (Zhao et al., 2023b). The household sector consumes large amounts of energy in the form of heating and cooling, among other things, and accounts for a significant share of greenhouse gas emissions (Goldstein et al., 2020). It plays an important role in reducing carbon footprint and achieving energy sustainability. In the household sector, the widespread use of electricity is driving changes in our lifestyles. In the Kingdom of Saudi Arabia (KSA), electricity consumption in the residential sector accounts for 50% of total production (Alqahtani and Balta-Ozkan, 2021); 26% of U.S. households rely on electricity as their sole energy supply (Michelle Lewis, 2022). The residential sector accounts for about 24% of India’s total electricity consumption (Ramapragada, 2022); which is already close to 40% in China (CESY, 2020). Continued high growth in electricity demand has contributed to a rise in enthusiasm for clean, sustainable energy generation and energy independence. However, about 80% of global energy and 66% of electricity generation still originates from fossil fuels (UNEP, 2022). Not only does this lead to environmental problems such as atmospheric pollution and climate warming, but for countries or regions where fossil fuels are scarce, the issue of energy supply, demand conflicts and energy security are more prominent (Qerimi et al., 2020).
We are at a critical turning point in the energy transition and sustainable development, and it is urgent to achieve energy savings in the household sector. The prerequisite is a comprehensive understanding of trends in household energy consumption (HEC), quantitative analysis of environmental impacts, and actual causes so as to facilitate more sustainable measures to reduce the household carbon footprint (Wang T et al., 2023). Some scholars have reviewed and summarized the research progress in the HEC.
First, the exploration of influencing factors is a topic that has been extensively covered by scholars (Bhattacharjee and Reichard, 2011; Jiang et al., 2020). Mainly evaluated the influence of social and economic factors on household energy consumption. The behavior of family members often has a significant impact on energy consumption, equipment use decisions, etc. (Borozan, 2018) integrated and external factors and individual behavioral features for multiple discussions of causes (Hafner et al., 2019; Adua, 2020); focus on a review of factors that reduce household energy demand from a behavioral and habitual perspective (Su et al., 2023). Developed a dynamic assessment model to quantify the impact of occupant behavior on household energy consumption and carbon emissions. Faced with a range of negative impacts caused by solid fuel use (Elasu et al., 2023), concentrates on exploring the determinants of a clean energy transition (Guta et al., 2022). Pays more attention to the barriers of energy transition for households in the middle-income and low-income countries.
Secondly, The review of quantitative methods as well as energy and carbon reduction programs was also a popular topic (Zhang et al., 2015). Mainly discusses the methods for quantifying household carbon emissions, and related emission reduction measures (Hertwich, 2005). Focuses on the different of the life cycle approach in sustainable consumption (Wiedmann, 2009). Reviews the contribution of input-output approach (IOA) in the work on consumption and emissions accounting (Oladokun and Odesola, 2015). Critically reviews modeling methods and techniques related to household energy consumption and carbon emissions (McAndrew et al., 2021). Systematically examines the effectiveness of a range of household energy efficiency interventions (Liu L et al., 2020). Review focused on the evaluation methods and analysis of influencing factors for HECs.
Some other studies have focused on the prospects of household energy efficiency development. The household sector, recognized as an energy-intensive and high-emission sector, has consistently garnered attention regarding the realization of energy-saving effects. Faced with the continual growth in energy consumption pressures, enhancing residential energy efficiency is regarded as a pivotal means to mitigate energy costs and reduce emissions (Kaufmann et al., 2023; Zheng et al., 2024). Xu L et al. (2021) expressed the pioneering nature of future research in the study. Studies identified behavioral interventions, energy efficiency and energy poverty were identified as possible research frontiers. Especially with the widening scope and spatial disparities of energy poverty, scholars have initiated multidimensional explorations into its influencing factors and policy interventions. The assessment of energy efficiency levels and the projection of energy saving potentials are important prerequisites for the formulation of effective policies (Jia and Wu, 2022; Stojilovska et al., 2022). In study of (Calvin Nsangou et al., 2020), the energy demand Frontier function model was used to estimate the electricity efficiency index of households; the model was applied by (Wang X et al., 2023) to evaluate a 31% energy saving potential for heating in HSCW zones in China. In addition to this, some scholars have focused on the impact of income inequality on the energy efficiency gap to explore ways to reduce energy costs for low-income households (Chaudhuri and Huaccha, 2023).
There is an increasing focus on the impact of HEC. Increasing attention is being paid to accounting for household energy consumption, environmental impact assessment, and implementation of energy efficiency measures. This paper reviews the current literature related to HEC. A descriptive analysis of research trends, the main performance and contributions of countries, regions, organizations, and authors was performed in combination with bibliometric tools. Keyword cluster analysis, citation analysis, etc., were performed in combination with co-occurrence maps.
Compared to previous review articles, the novelty of this paper lies in two aspects. Firstly, it provides a classification and comprehensive review of research methods in Household Energy consumption (HEC). These methods revolve around three aspects: environmental impact assessment, factor analysis of influences, and behavioral analysis. The advantages and disadvantages of their applications are discussed. This provides valuable information for researchers to gain a deep understanding of the development of HEC and offers targeted references for future research method selection and optimization. Another contribution of this paper is the attempt to review and critically discuss recent popular approaches to household energy conservation and emission reduction.
Furthermore, this paper summarizes important themes that have sparked widespread discussion in recent HEC research. These include key factors influencing household energy consumption, the implementation of energy-saving programs and policies, as well as the barriers faced. It also examines the development and optimization effects of smart home technologies and how residents’ consumption behavior and decision-making processes impact energy usage. This provides consumers with information and tools for energy conservation and offers guidance to utilities and governments in formulating energy plans, enabling them to make wiser decisions in sustainable energy production and utilization.
The content of the paper is following: In part 2, explained the literature search sources, databases, and analysis tools; In part 3, the results of the bibliometric analysis are described in terms of journal contribution, disciplinary contribution, literature coupling, keyword co-occurrence, and country/organizational productivity; In part 4, the currently popular research methods are summarized; In part 5, a critical discussion is presented on the aspects of energy efficiency measures that are currently of high interest.
2 Methods, data sources
2.1 Methodology
Bibliometric analysis, with its flexibility and powerful data processing capabilities, is an important tool for exploring the current stage of quantifying and analyzing a discipline or a particular research element. It can track the trends and dynamic hotspots of research topics in an objective and comprehensive manner. However, literature metrics analysis exhibits a certain degree of citation bias, where some innovative perspectives might be overlooked due to the low prominence of authors or journals. Additionally, literature databases often suffer from a time lag, impeding their ability to promptly reflect the latest developments in research. Based on this, this study will be conducted with a combination of bibliometric analysis and manual review.
Firstly, a review and statistical analysis were conducted on literature related to household energy consumption published in peer-reviewed journals over the past 20 years. A descriptive analysis was employed to characterize the current state, trends, and peer contributions. Through manual examination, duplicates and non-academic literature were excluded to ensure the validity of the analytical results. Simultaneously, recent viewpoints and research findings were introduced to discuss prevalent model approaches, dynamic focal points, and research challenges in the field.
2.2 Data sources
The bibliometric literature search of the literature database is performed on the “Web of Science Core Collection database”. The database covers many peer-reviewed, high-quality academic journals containing scientific and technical literature in more than 260 subject areas worldwide, which can provide much of the information needed for interdisciplinary development. It facilitates identification and statistical information for researchers. In addition, a multiple of data extraction formats are supported, providing a complete record of all necessary literature information that can be imported into bibliometric software tools for visual analysis.
The first step in the study was to identify the literature dataset using a keyword search. Using the literature search platform in Web of Science, the final dataset was determined by inputting keywords, date range, and other relevant criteria. The literature collection was exported in January 2023 and underwent subsequent screening processes. The keywords included “energy consumption”, “households”, and “residential”, and the time frame was set from 2000 to 2021. From the conditional search, a total of 791 open access papers were generated. Second, the search results were refined using the filtering function of web of science, and the language was set to English for ease of subsequent understanding. Among the types of literature, research papers are the main contribution to research in the field, accounting for about 85% of the total number of publications, followed by conference proceedings papers with about 13.3%, and other publications belonging to reviews as well as online publications. Since research articles usually provide more pioneering research results and have more information about authors, institutions, and citations. Therefore, only journal articles published in English are considered for further analysis in this paper, totaling 673 articles. The second step involves the article’s indicators, including title, abstract, keywords, references, authors, and organization. This data provides key information about research trends and directions in the HEC. These articles and related information are exported in text format. Finally, we need to further review the exported 673 articles. The main screening objects were withdrawn articles, duplicate articles, and articles that did not match the topic. A final literature dataset of 619 manuscripts was obtained and studied in depth using bibliometric analysis tools. Figure 1 represents the output process of the literature dataset.
2.3 Analysis tools
The selected articles have been identified, and it is necessary to use literature analysis tools for networked and visual analysis of the database. With the application and development of bibliometrics, many different types of knowledge graph tools have been derived. Among them, Citespace, HistCite, VOSViewer, Bibliometrix, etc., are commonly used analysis tools (Xu et al., 2023). Compared with other bibliometric software, VOSViewer has strong graphical presentation capabilities and is suitable for large-scale data; it can create co-authorship, bibliographic coupling and co-citation networks based on citation data, or construct keyword maps using co-occurrence data (Hu et al., 2022). It can also use the thesaurus function for data processing to improve the accuracy of analysis results.
3 Results of bibliometric analysis
3.1 Trends in HEC research
3.1.1 Number of publications
The statistics of article publication trends are presented in Figure 2. The number of annual publications on household and residential energy consumption is generally on the rise. Especially since 2014, which has witnessed a period of rapid growth, with an average annual number of 76.8 articles and an average annual growth rate of 27.5%. This also indicates that energy consumption in the household sector has attracted a great deal of attention from scholars in the face of global climate action, energy depletion, and carbon emission.
3.1.2 Discipline performance
According to the web of science, the research topics were mainly related to 65 different disciplines, and the results are detailed in Figure 3. Interdisciplinary integration has been a common phenomenon in HEC research, with energy fuels ranking first among all disciplines, accounting for about 21.9%. This shows that studies from an energy transition perspective are a key discipline for HEC, such as the development of sustainable energy sources and how to reduce dependence on traditional energy sources. This is followed by Environmental Sciences (12.7%), Environmental Studies (12.4%), and Green and Sustainable Science Technology (8.4%); the negative impacts of HEC and how to improve environmental benefits are also gaining importance. It is worth noting that Economics (7.0%) is also one of the indispensable disciplines in HEC research. It plays an important role in improving pricing measures in the electricity sector and optimizing energy management systems. In addition, disciplines related to building technology, construction, and materials are among the major contributors to HEC. Specifically, they include Building Technology (4.8%), Engineering Civil (3.9%), Engineering Electrical Electronic (3.5%), and Engineering Chemical (2.6%). The remaining other disciplines collectively contributed 20.6%.
3.2 Research contribution
3.2.1 Contribution of journals
The 619 articles were published in 177 different journals, but about 66% of these journals published only one relevant article. The 10 categories of journals that contributed the most to the field are listed, showing their total publications (TP), total citations (TC), and the average number of citations (AC) for the period 2000-2021. Secondly, the impact factor (IF) of journals, which is an important indicator to evaluate the impact of articles, was also examined as one of the objects. The results are shown in Table 1.
In terms of TP scores of journals, Energies is the most productive journal with 81 articles, but the TP, AC and IF scores are not high. This is followed by Sustainable development (61 articles) and Energy policy (47 articles). The combined TC and AC scores of the journals were considered, and Energy policy and Applied Energy were ranked first and second, respectively. This indicates that both journals contribute many high-quality articles to HEC. It is worth mentioning that Applied Energy’s IF score (11.446) is the highest score in this journal contribution review process, representing the high influence of the journal in HEC research. Energy and buildings (1,506) also have a good performance in terms of TC and AC score. Energy economics is also one of the representatives of high-quality journals. Although there are only 16 publications, the articles have relatively high TC (726) scores and AC scores (45.38) and the journal has the second highest IF score (9.252). This again confirms the high attention given to research related to energy economics. Other important journals include Sustainable development, IEEE access, Energy, Energy research and social science, Energy efficiency, Environmental research letters, Energy reports.
3.2.2 Country and region
In terms of country/region contributions, a total of 80 countries/regions published papers related to the topic of household energy consumption, with about 25.6% of countries/regions contributing only one article. The 10 countries/regions which are most active in research field are listed in Table.2, based on the score of TP, TC and AC to articles. Joint collaborations (JC) is used to explain the strength of cooperative relationships between countries and is one of the objectives of the review. They are the United States (USA), the United Kingdom, China, Germany, Australia, Netherlands, Spain, Japan, South Korea, Canada, and Italy.
In view of the TP, the USA (141), the United Kingdom (105) and China (102) have the highest scores, accounting for about 51.8% of the total number of published articles. The impact of HEC has attracted high attention from carbon-emitting countries. For TC, the USA (5,474) is far ahead and has a high influence on HEC research. As one of the major countries in global energy consumption, the US has played a significant role in energy policy and technological innovation (Subbiah et al., 2017). This is followed by the United Kingdom (3,276), the Netherlands (2,916). European countries are committed to promoting energy transition and enhancing energy efficiency (De Boeck et al., 2016), and they have played a pivotal role in research on household energy consumption as well. China (2,161) as one of the world’s largest energy-consuming countries, is actively focusing on areas such as renewable energy and smart grids (Gao et al., 2015). Although the Netherlands (81) and Canada (75.72) did not score high in TP, TC and AC scores were second only to the US and the United Kingdom, indicating that they have a strong research capacity and contribute high quality articles regarding the HEC field. In addition, most countries/regions have concentrated their publication productivity in 2017–2018.
The analysis of country/region cooperation was carried out using the full counting calculation method in VOSviewer, and the minimum threshold of published articles was set to 12, which finally filtered out 20 countries/regions that met the above conditions. Figure 4 shows the international cooperation relationships between countries/regions in the field of household energy consumption research. The countries are divided into four different color clusters based on the strength of the relationship, with the red cluster centered on the United States and containing Germany, France, and Switzerland. The green cluster is led by China and the United Kingdom, and includes Japan, Australia, Ireland, and Pakistan. The blue cluster is represented by the Netherlands and Spain, with South Korea, Denmark Sweden, Portugal, and Italy. The red cluster consists of Canada and Finland. Secondly, the circles represent the number of publications, and the connecting lines point out the cooperation relations between countries, with the thickness of each connecting line representing the intensity level of cooperation between countries.
Germany has the densest international cooperation network among the country cooperation networks and maintains cooperation with 16 countries. Although the total number of publications in Germany is only 50, 48 of the articles are collaborative papers. Germany holds a leading position in renewable energy and energy development, and it collaborates with the United States and other European countries in joint research in this field (Andor et al., 2021; Heesen and Madlener, 2021). The USA and the United Kingdom, as the most productive countries, maintain cooperation with 14 countries and have the strongest partnership with China. China also maintains international collaborations with 12 countries, including the USA the United Kingdom, Australia, and Japan, more than 70% of the 102 articles published are collaborative articles. The emissions and climate issues brought about by HEC have attracted global attention. International research cooperation has not only promoted the achievement of global carbon reduction goals, but also enhanced cooperation and understood among different countries.
3.2.3 Organization
A total of 871 organizations published articles on household energy consumption, with nearly 73% of them publishing only one article. The difference in the number of publications between the organizations is not significant, with the University of Oxford and Aalborg University having a relatively high number of publications, with 13 articles each. This is followed by, Delft Univ Technol, Tech Univ Denmark, Tsinghua Univ, and Univ Calif Berkeley, with 11 articles respectively. From the total number of citations of the papers, Carnegie Mellon Univ (634) was the most active, followed by Univ Calif Berkeley (517) and Univ Oxford (509). Five of the selected institutions are from the United States, reflecting the important contribution of the United States in this area of research. Two each are from China, Denmark, and the Netherlands.
By using the full counting method of VOSviewer, by setting the threshold of the minimum number of published articles to 5, a network of partnerships between organizations was obtained, and for organizations with no partnerships, the color of the circles was marked in gray. The results are shown in Figure 5. The organizations are divided into seven clusters according to the strength of their partnerships. Blue is the largest cluster, with Univ Calif Berkeley as the core organization of the cluster and the largest number of collaborating organizations. The second largest cluster is the green cluster headed by Eindhoven Univ Technol, with 5 organizations in partnership. This is followed by the red and yellow clusters, with Trinity Coll Dublin and Univ Maryland as the cores, respectively. Meanwhile, there is a clear cross-collaboration between the blue, green, and orange clusters. In terms of the number of collaborative papers, Univ Calif Berkeley, Eindhoven Univ Technol, Hong Kong Polytech Univ, Trinity Coll Dublin, and Econ & Social Res Inst all have 8 collaborative papers and are jointly ranked first.
3.2.4 Authorship
A total of 2,227 authors contributed to the study of household energy consumption, with only 167 authors having more than 3 papers. Table 3 lists the eight authors who made major contributions and the organizations to which they belonged. Also, their total number of publications, total frequency of citations, and average number of citations per article are concluded. Alberini, Anna from the University of Maryland has published 6 relevant articles with the highest total and average citation frequency, and her research has had a significant impact. Grunewald, Philipp (5 articles) from the University of Oxford is in the second. And Baik, Sung Wook from Sejong University and Ullah, Amin from Oregon State University scored relatively high in terms of the frequency of cited papers and the average number of citations.
Figure 6 further shows the network of collaborative relationships between the authors and the strength of the links. A visual analysis of the authors’ collaboration network is performed using fractionalization in the normalization method. The most active research groups in the research are shown by using different colors, each with 1 or 2 authors at the core. In contrast to national and organizational collaborations, research groups are isolated from each other. Each circle in the figure represents an author, the size of the circle represents the number of publications by each author, and the connecting lines indicate the collaborative relationships between authors. The Blue Cluster constructed by Hanaoka, Tatsuya, Kanamori, Yuko, Masui, Yoshihiko, Xing, and Rui maintain a strong collaborative relationship. In the published articles, all four of them were collaborated. The Green Cluster, headed by Grunewald, Philipp, also formed a collaborative group. Of his five published papers, three belong to the collaborative group. The red cluster led by Alberini, Anna, although she has the largest number of papers, has only two collaborations with Torriti, Jacopo, and Filippini, Massimo. The next closest collaborations are the pink cluster with Baik, Sung wook from Sejong University and the purple cluster with Shen Guofeng, Shu Tao from Peking University.
3.3 Theme evolution
3.3.1 Keyword co-occurrence
The co-occurrence analysis of keywords provides a clear sense of the key research content of a topic at a certain period. Using the Keywords plus function in VOSviewer, 1,231 keywords were selected from the database. To increase the accuracy of the results, the keyword dataset was processed. Firstly, we filtered words that were not relevant to the study topic, such as diffusion, refurbished, heterogeneity, etc. Secondly, we used thesaurus file function to merge and replace words with similar or same meaning, such as household and households, impact and impacts, carbon emissions, dioxide emissions, CO2 emissions, etc. To extract more accurate and valuable information, the minimum frequency of keywords was set to 8, and finally a network map containing 73 keywords was exported Figure 7. Each circle represents a keyword, and the size of the circle is determined by the frequency of keyword occurrence, which is divided into three clusters (Cluster 1,2,3) according to the correlation strength of the keywords, and there is a close relationship between the three clusters.
In Table 4, list the research themes, main keywords, and their frequency of occurrence for three clusters. In the face of global concerns about climate change, HEC research has increasingly focused on sustainable energy utilization and improving energy efficiency. This includes aspects such as the utilization of renewable energy, the development of energy management systems, and technological innovations (Abushnaf et al., 2016). Cluster 1 focuses on household energy efficiency and energy management systems, sustainable energy development and energy storage, and energy efficiency technology improvement and system optimization (Bak and Yoon, 2021; Bataineh and Alrabee, 2018; Chang et al., 2018; Hamilton et al., 2013; Kumar et al., 2023; Xu et al., 2013). The development of data technology has sparked research and discussions on the potential for household electrification. Additionally, residents’ behavior and decision-making processes have emerged as popular topics, exploring how changes in residents’ energy consumption behavior can lead to reductions in energy consumption and emissions (Das et al., 2018).
Cluster 2 focuses on household electricity consumption, demand, and determinants; energy efficiency policies and efficiency studies; residents’ energy consumption behavior and attitudes. Energy efficiency effectiveness has become a key measure in achieving energy savings in the household sector. This includes an assessment of energy efficiency (Hamilton et al., 2013), an exploration of determinants (Adha et al., 2021), and a prediction of future energy savings potential (Bataineh and Alrabee, 2018). The exploration of the determinants affecting energy efficiency is one of the topics that have been widely and continuously studied by scholars, which is of great significance for the development of effective policy planning and the full utilization of the economic benefits of energy (Chang et al., 2018; Bak and Yoon, 2021). Confirmed the significant impact of dwelling characteristics, building envelope performance, etc., on energy efficiency. Occupants’ energy behavior, rational use of equipment, and awareness of energy conservation are also factors that cannot be ignored (Xu et al., 2013; Das et al., 2018). Second, government and local policy interventions have driven improvements in residential energy efficiency (Jafari, 2023; Kumar et al., 2023).
Cluster 3 covers the impacts resulting from household energy consumption, including the increase in carbon emissions, climate change, etc. (Adua et al., 2016); and the analysis of factors affecting household energy and demand, such as urbanization level, lifestyle, income, transportation, etc., (Cao et al., 2019; Mainimo et al., 2022).
3.3.2 Citation analysis
Citation analysis is an activity and method that is based on the citation and referenced relationships between literature, authors, journals, and other sources. It involves studying the network or chain relationships among citations to reveal the development and connections within a field, as well as to provide insights into prospects. The more times an author or article is cited in other scholarly articles, the more influential it is in that discipline, enabling the importance of that article or researcher in any given field to be judged.
A simple method of citation analysis is to count the TC to a paper. However, the comparison of a single condition is often too abstract and causes large errors. However, the comparison of a single condition is often too abstract and causes large errors. According to the spreading pattern of academic literature, generally scientific papers reach the peak of citations within 3–5 years after publication, so recently published articles may not receive higher attention, so we will consider the average number of citations per year of the article (AC/Y) as well as the Normalized citation count of the article (NC). We analyzed the most influential articles in the field over the past 20 years, setting the minimum number of citations to 50, resulting in a total of 34 articles, which are shown in Figure 8, where the size of the points represents the TC, and the shade of the color indicates the year of publication.
Table 5 further shows the top 10 articles and their performance in terms of TC, AC/Y, and NC for the past 20 years of research in HEC. The highest combined score comes from Abrahamse et al. (2005), reviewing research on the effectiveness of interventions to promote energy efficiency in the household. The articles by McLoughlin et al. (2015) and Asensio and Delmas (2015) ranked second and third, respectively, based on AC/Y. McLoughlin et al. (2015) extends the development of clustering methods in characterizing household electricity demand. Asensio and Delmas (2015) investigates the effectiveness of nonprice incentives in motivating energy-saving behaviour. Bin and Dowlatabadi (2005) ranked third in terms of NC, first proposed the Consumer Lifestyle Approach (CLA), which discusses the importance of consumer activities for energy conservation and emission reduction.
4 Research hotspots in HEC
Household activities are attracting more attention for issues such as energy consumption and environmental impact. Research on household energy consumption (HEC) is complex and continuous, involving multiple fields and disciplines. There are variations in the research methods preferred by scholars due to different ways of collecting research data, different scales of research, and different research purposes. Firstly, a filtered search of the dataset was conducted by using the terms model, method, analysis, and quantification to identify the main popular methods and models regarding HEC. This study will discuss the popular research methods in HEC around the mainstream directions of energy use and quantification, environmental impact assessment, exploration of influencing factors. Considering that the behavior, habits, and awareness of households are closely related to changes in household activities and energy consumption, it is necessary to discuss the energy consumption behavior of households.
4.1 Environmental assessment
Environmental assessment plays an important role in the study of HEC, leading to a comprehensive understanding and management of energy use patterns, identification of potential energy waste, and the development of more effective energy management strategies for reducing negative environmental impacts (Dioha, 2018; Bülbül et al., 2023).
4.1.1 Input-output approach
Input-output approach (IOA) is an economic model for comprehensive analysis of the quantitative dependence between inputs and outputs in economic activities. According to different measurement methods, it is divided into physical model and value model, dynamic model and static model; according to the research scope, national model, single-region model, inter-region model, sectoral model and enterprise model can be established.
In the field of household energy consumption, IOA is widely applied to the allocation and utilization of energy, as well as the assessment of potential carbon footprints and economic benefits (Qin et al., 2022; Wang F et al., 2023). In the selected paper collection, the authors used several types of IOA to carry out their studies on energy use in the household sector. Poom and Ahas (2016); Tomas et al. (2020) used the environmentally extended IOA to evaluate household energy use and carbon footprint; (Baltruszewicz et al., 2021; Sato and Narita, 2023); studied the composition and inequalities associated with household energy footprint based on the multi-regional input-output (MRIO) model. Quantifying household indirect and direct energy consumption-demand relationships using IOA is a particularly widely studied topic (Supasa et al., 2017; Singh et al., 2018; Long et al., 2019). In addition, input-output models have established an important role in measuring household carbon emissions, especially in China (Xu L et al., 2021; Tian et al., 2017; Zhang and Lei, 2017).
Many studies have confirmed the contribution of IOA models in promoting energy efficiency and in developing energy efficiency policies of household (Stagnitta et al., 2020). However, some studies have also shown that energy efficiency improvements are instead a potential risk to retard energy efficiency effects. And IOA models also play an important role in estimating and explaining the energy rebound effect (Thomas and Azevedo, 2013; Wen et al., 2018).
4.1.2 Life-cycle assessment
Life-cycle assessment (LCA) is a tool for evaluating the environmental impact of a product or service from production, use to disposal. It can be used to assess the overall environmental impact of a household, including buildings, equipment, water use and waste management (García-Valiñas et al., 2023). LCA has been widely used in the building sector and is one of the most important tools used to assess the building performance (Utama and Gheewala, 2009), energy sustainability in buildings (Vogt Gwerder et al., 2019; Walzberg et al., 2019), building environmental impact and stress (Vega-Azamar et al., 2017). Secondly, it has also been widely deployed to evaluate home energy efficiency improvements and explore improved pathways to energy conservation. Nichols and Kockelman (2014) used LCA to confirm that changes in the built environment and increases in population density can promote improvements in home energy efficiency, and (Stephan and Crawford, 2016) quantified the implied energy due to changes in house size that improve the effectiveness of building energy efficiency regulations.
With the emergence of home energy management systems, LCA plays an equally important role in assessing the environmental impacts of smart buildings and home automation (Louis et al., 2015; Louise and Pongracz, 2017).
4.2 Influencing factors analysis
Large population sizes and rapidly growing economies lead to large energy consumption and carbon emissions in the residential sector (Xu L et al., 2021), which are threatening environmental sustainability. Identifying and analyzing the key drivers affecting household energy consumption (HEC) has proven to be an important step in improving energy efficiency and pursuing sustainable energy strategies (Wang et al., 2015).
The analysis of factors influencing HEC typically considers two perspectives: internal and external factors within households. Internal factors mainly encompass socio-economic factors, population dynamics, and residential environmental factors (Mashhoodi et al., 2020; Amber et al., 2021). In terms of socio-economic factors, characteristics of household members are considered a key factor discussed by many scholars. Apart from individual behavior and attitudes, factors such as household size and age composition of occupants are recognized as important determinants influencing HEC. There are studies that confirm the overburden of older households in terms of energy supply and expenditure (Berrill et al., 2021), and the phenomenon of aging has been explained as a determinant of household energy use efficiency (Pais-Magalhaes et al., 2020). With rapid economic development, household sizes are further shrinking, and small households or nuclear families are becoming the mainstream. This trend is expected to drive a significant increase in residential energy demand in the future (Zeng et al., 2021). In addition to this, the type of residential building and the environment also have an impact on energy consumption patterns to a certain extent (Namazkhan et al., 2020; Moadab et al., 2021).
Among the external factors, energy policies as well as climate conditions are the main perspectives discussed by scholars. The implementation of time-of-use electricity pricing plans (Kwon et al., 2020) and energy-efficient appliance subsidy policies (Nie et al., 2021) play a significant role in controlling electricity consumption and reducing energy costs. Climate conditions are a major driving factor in HEC and have been widely applied in estimating household energy use. In developed countries such as Europe and the United States, the number of heating and cooling degree days is an important indicator for measuring household heating and cooling demand (Adua et al., 2016; Alberini et al., 2019). In emerging economies, Li established temperature-electricity response functions to predict the impact of climate change on electricity consumption. Additionally, (Bezerra et al., 2021), developed and applied end-use models to assess the changes in HEC due to temperature and relative humidity.
Furthermore, under the global decarbonization goals, climate change has driven increased attention to the sustainable development of future residential energy and the formulation of related policies. For example, in Jordan, the implementation of this goal is reflected in the retrofitting of existing housing for improved energy efficiency (Bataineh and Alrabee, 2018). In India, increasing the supply of low-income housing is considered an avenue for achieving climate mitigation (Khosla et al., 2019). A number of methods or models have been used to develop the analysis of the factors affecting the drivers of household energy consumption, as shown in Table 6.
4.2.1 Regression analysis
Economic methods are increasingly used in HEC research. It can help researchers understand household demand for energy and consumption behavior. The elastic response to energy under different economic conditions is analyzed, as well as the impact of price, income and other factors on HEC (Guo et al., 2023). Regression and econometric methods are frequently used methods. It useful to deal with and analyze investigate large data sets (Greenblatt et al., 2013; Copiello and Gabrielli, 2017). Hassen et al. (2023) used a multivariate probit model to discuss the behavioral and socio-economic factors that influence energy efficiency technologies in urban households. Shi et al. (2020) developed a LASSO regression model to identify the main drivers of direct or indirect HCEs. Belaid and Garcia (2016) conducted an empirical study on the main factors that motivate energy saving behavior in French households through econometric modeling. Zhang and Wen (2021) develop a Panel Smoothed Transition Regression (PSTR) model and explore the driving effects of urbanization level and income level on household electricity consumption according to empirical data. In addition, Least squares method (LMS) (Besagni and Borgarello, 2019) and Quantile regression model (Tilov et al., 2020) are frequently used to explain the effect of factors such as social-economic and household characteristics on HEC.
4.2.2 Decomposition analysis
Decomposition analysis as an analytical framework for studying the characteristics of changes in things, quantifying environmental impacts and relative contributions, has been widely used in environmental economic research. Structural decomposition analysis (SDA) and Index decomposition analysis (IDA) are the two main categories of decomposition analysis. SDA enables a more detailed description of the energy demand and consumption structure based on input-output models (Du et al., 2018; Kim et al., 2015) which also means a higher request for data. While the IDA method is more adaptable and easier to use, the Logarithmic Mean Divisia Index (LMDI) method is the most popular (Zhao et al., 2023a). Most studies have used LMDI to quantitatively identify the effects of different factors on changes in energy consumption (Roman-Collado and Jose Colinet, 2018; Meng et al., 2019; Reuter et al., 2019). In China, scholars paid attention to use LMDI to comprehensively analyze the differences in inter-provincial (Li et al., 2020; Rong et al., 2021) and urban-rural (Chen et al., 2019; Yang et al., 2020a) of energy consumption and carbon emissions in the household sector. In recent years, some scholars have started to focus on the decoupling analysis of REC, Yang et al. (2020b) and Zhang and Bai (2018) combined Tapio decoupling model and LMDI method to evaluate the decoupling relationship between energy consumption and residential income. In addition, the Kaya identity is one of the main methods of decomposition analysis. Chen and Lin (2015) and Serrano et al. (2017) used the Kaya identity to evaluate the effect of economic, policy and demographic factors on the environmental impact.
4.2.3 Other method
The augmented Stochastic Impacts by Regression on Population, Affluence and Technology (STIRPAT) model is also an important method for exploring the factors influencing household energy consumption (Ding et al., 2016). Investigated the spatial heterogeneity of household energy consumption and its influencing factors in China using the STIRPAT model, and the results confirmed that income level is the main factor contributing to the spatial effect. Zhou and Liu (2016); Bai et al. (2019) used the STIRPAT model to assess the effects of population, income, and urbanization on energy-related CO2. Other methods include numerical modeling, such as structural equation modeling (SEM) (Boukarta and Berezowska-Azzag, 2018), Multiple Discrete Continuous Extreme Value (MDCEV) model (Pinjari and Bhat, 2021), Cost-benefit analysis (CBA) (Maljkovic et al., 2022).
4.3 Behavioral analysis
The consumption of energy generated by household activities is highly behavioral (Long et al., 2019). The lifestyles of residents present a serious threat to energy sustainability. User behavior is considered one of the most significant factors influencing household energy consumption. As the main driver of household activities, more research focuses on exploring user consumption behavior to enhance energy efficiency and reduce environmental impact (Eirinaki et al., 2022). Therefore, exploring the factors influencing households’ consumption behavior is essential to achieve energy efficiency in households. It is worth mentioning that due to the global COVID-19 pandemic in recent years, social distancing and home lockdown measures have exacerbated household energy stress, and behavioral analysis of occupants during the pandemic has generated much attention from scholars as well as policymakers (Ludtke et al., 2021; Tleuken et al., 2021). Secondly, during the COVID-19 era, there has been a significant emphasis on energy efficiency and the willingness to purchase energy-saving home appliances (Jamil et al., 2022). Due to the complexity of consumer behavior, which is also influenced by various factors, scholars have adopted different research approaches from different research perspectives.
4.3.1 Theory of planned behavior
The theory of planned behavior (TPB), which consists of the elements of attitudes, subjective norms, perceptual behavioral control, and behavioral intentions and behaviors (Ajzen, 1991), is widely used in behavior-related research fields to predict, and understand human behavior (Ajzen, 1985). The theory assumes that behavioral intention is the most direct factor influencing behavior, and that behavioral intention is in turn influenced by attitudes, subjective norms, and perceived behavioral control. It has been confirmed that TPB and its extended theory contribute to the explanation of household energy saving behavior and to the intervention of residential energy behavior (Schwartz et al., 2015; Ru et al., 2018).
Since the theory is mainly based on human behavioral intentions to make judgments, the data sources for the research are mainly obtained through customer questionnaires. The use of TPB theory to explore and identify the role of consumer attitudes, subjective norms, and perceived behavioral control on building energy efficiency behaviors and intentions is commonly studied (Liu X et al., 2020; Ru et al., 2018). Khurshid et al. (2023) based on Pakistani household data, combined Structural Equation Modeling (SEM) and TPB to gain insights into the psychological factors and emotions driving energy-saving behaviors. Tan et al. (2017) studied the indirect effects of residents’ willingness to purchase energy-efficient appliances through the extrapolation of personal moral norms in TPB. Adnan and Shahrina (2021) and Masrahi et al. (2021) based on the TPB research model, predicted consumers’ willingness to use renewable energy.
4.3.2 Consumer lifestyle approach
Bin and Dowlatabadi (2005) first proposed the Consumer Lifestyle Approach, which aims to develop a comprehensive consumer-oriented assessment framework for quantifying the environmental impacts of consumer lifestyle factors on energy use and resulting impacts. At the same time, it defines the direct and indirect impacts of consumer activities or use of products from the consumer’s perspective and is widely used to estimate trends in energy consumption and carbon footprint in the household sector. Wang and Yang (2014) used the CLA method to estimate the indirect energy consumption of households in urban and rural areas of China. Wang and Zhang (2015) analyzed the characteristics of direct and indirect household energy consumption in rural China for the period 1988-2011. As carbon emission research fever continues to rise, numerous scholars have analyzed the quantity and trends of household carbon emissions using the CLA method (Chen et al., 2019; Xu et al., 2016). And the combination of STIRPAT model (Jin et al., 2019; Wang et al., 2018; Wang and Yang, 2014) to explore the main influences of household carbon emissions has been favored by many researchers.
4.3.3 Cluster analysis
Cluster analysis is a statistical analysis method that simplifies and mines data by using different algorithms. The sample data are divided into relatively homogeneous clusters, where objects in the same cluster have high similarity and different clusters are heterogeneous from each other. Commonly used clustering algorithms include K-means, K-medoids and CLARANS (Han et al., 2022). K-means algorithm is one of the relatively simple and widely used methods (Fernandes et al., 2017; Trotta et al., 2020), its provides a new perspective for identifying occupant behavior and understanding energy use in buildings (Yu et al., 2011). It has also proven to be important in improving and adjusting the usage patterns of residential air conditioning and saving energy costs (An et al., 2018; Mitra et al., 2021). With the rise of machine learning, clustering analysis has also gained popularity and has been widely applied to household meter data in recent studies (Roberts et al., 2019; Satre-Meloy et al., 2020), providing a basis for understanding residential electricity demand and explaining the driving forces affecting electricity consumption patterns. Cluster analysis has been beneficial in exploring residential water end-use consumption categories (Makki et al., 2015), land use policies, and improving public transportation services (Zahabi et al., 2012).
4.3.4 Other methods
With the implementation of Demand Response Programs (DRPs), Household Energy Management systems (HEMS) and its key role in achieving the Sustainable Development Goals (Ramos et al., 2019). It is especially important to monitor the energy use behavior of occupants (AlSkaif et al., 2018), especially in identifying the behavioral habits of electricity users (Granell et al., 2015; Gajowniczek and Zabkowski, 2017; Khosla et al., 2019). Nakano and Washizu (2019) Kim et al. (2019) analyze and predict the characteristics of household electricity consumption under different behavioral patterns by using the Survey on Time Use. Sadeghianpourhamami et al. (2016) and Haben et al. (2016) based on smart meter data, conduct in-depth analysis of household behavior, and use it as a basis to build an energy load prediction model. Ouyang et al. (2009) and Wu et al. (2017) developed their studies based on the data from microdata surveys. In addition, neural network framework has been applied to analyze and understand residential electricity consumption habits and predict energy loads on the household sector (Justo et al., 2017; Alhussein et al., 2020; Estebsari and Rajabi, 2020).
5 Exploration of household energy saving
The large share of the household sector in global energy consumption and the implied potential for energy savings is attracting more and more attention (An et al., 2018). In response to global climate action, many countries have actively taken steps to promote sustainable development of household energy and enhance energy efficiency. The EU, for instance, has implemented energy taxation policies aimed at modifying electricity consumption through energy price adjustments. France has provided financial support, including the quelle energie premium and credits from the Family Allowance Fund, to incentivize citizens to reduce energy consumption (Orset, 2021). The Chinese government has established energy efficiency standards and regulations to promote the energy efficiency of energy-consuming products (Tao and Yu, 2011).
However, how to develop and implement public measures to reduce energy consumption is not just a goal for governments or utilities anymore (Rutland and Aylett, 2008). For the supply side, it is effective by promoting renewable and clean energy, green energy-saving technologies to occupants (Duan et al., 2023). For the demand side, how to change their own awareness and participation behavior, and flexible adoption of smart technologies and green energy is important to reduce energy costs and achieve low carbon households. Focusing on energy-saving technologies, energy transition, and behavioral interventions, it discusses the contribution of energy-saving and emission reduction measures in the household sector and the facing challenges.
5.1 Transitioning towards smart energy
The emergence of information and communication technologies has paved the way for the construction of smart cities (Liaqat et al., 2021). And the development of smart cities cannot be achieved without efficient energy services. Residential customers as the main users of electrical energy, have a strong influence on electricity demand due to the randomness of their household activities and the flexibility of their appliance usage patterns (Wang et al., 2022). The promotion and application of smart control systems based on energy monitoring, management, and data analysis play a crucial role in optimizing energy utilization and providing personalized recommendations (Alsaigh et al., 2023; Paneru and Tarigan, 2023). The development and diffusion of smart home devices such as smart thermostats, smart lighting and smart appliances support the monitoring and management of energy behavior in the household (Alhussein et al., 2020; Moadab et al., 2021), to better control energy use and improve energy efficiency. Figure 9 shows the application of a HEMS in a typical residence (Zhao et al., 2021).
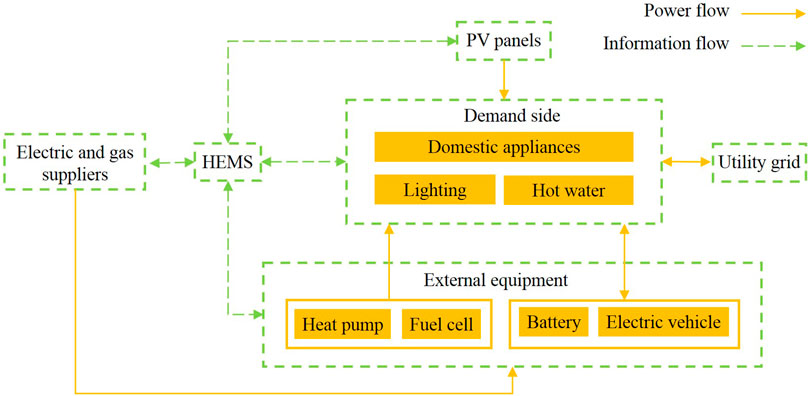
FIGURE 9. Basic framework of HEMS used in typical household (Zhao et al., 2021).
First, smart home technology allows seamless automation and control of various home systems from a single access point, such as lighting, heating, cooling, etc., improving quality of life and convenience. In terms of energy management, it can help control electricity usage through automated systems, reducing energy bills and a smaller carbon footprint (Louis et al., 2015; Pang et al., 2021). The data monitoring function of smart meters can effectively explore customers’ electricity consumption demand, which also supports the utility companies to optimize customer management, adjust electricity cost and policy innovation (Gholami et al., 2021). Moreover, (EL Jaouhari et al., 2019), proposed the possibility of smart technologies in establishing the interaction between energy, wellbeing, and health management through the study.
Although smart homes offer unlimited possibilities for future smart energy consumption in the household sector and promote the achievement of energy saving and emission reduction goals, there are still many obstacles to their promotion and popularization in households at this stage. Residential customers need to pay a high initial cost for the introduction of a smart home, which discourages many homeowners. In addition to reducing operational and service costs through technological means (Iqbal et al., 2019), deepening customers’ understanding of the value for smart services (Gonçalves and Patrício, 2022) and providing incentives to push the program forward. This will require more in-depth research and surveys (Srivastava et al., 2019). Second, as smart home systems connect more ports, security risks are put to the test (Komninos et al., 2014). The setup and maintenance of smart home technology requires a certain level of technical expertise, and customers inevitably have difficulty setting up and troubleshooting system issues. Thus, it is especially important to help customers gain a deeper understanding of the service and learn about it (Hollebeek et al., 2019), and to support them in taking on new roles.
5.2 Transitioning renewable energy
The continuous growth of energy demand and the transitional dependence on fossil fuels for power generation are the main perpetrators of negative impacts such as climate change and environmental degradation. The adoption of green energy and technologies at the household level is recognized as an effective tool for achieving sustainable economic development in the future (Eirinaki et al., 2022). Sustainable development goals are driving a deep energy transition among the demand side, to reduce the use of carbon energy (Elmorshedy et al., 2021). The rapid growth in electricity consumption has contributed to the widespread adoption of clean and renewable energy sources (Leitao et al., 2021). The use of renewable energy, mainly solar, wind and hydro power, emits almost no pollutants into the atmosphere, and produces no greenhouse gas emissions, which has the advantage of coping with climate change and effectively improves environmental benefits. Secondly, renewable energy comes from domestic sources, effectively reducing the dependence on energy imports, thus improving energy security (Dioha, 2018; Khan et al., 2020). Solar energy plays a crucial role in renewable energy and holds significant importance for achieving energy diversification and independence. The United States has implemented solar energy policies, such as investment tax credits to incentivize the development of renewable energy (Heng et al., 2020). In China, Japan, and India are gradually increasing green financing for renewable energy to support sustainable development (Rahmani et al., 2023).
In the household sector, sustainable energy management measures are rapidly developing (Heinisch et al., 2019; Puranen et al., 2021). Renewable energy systems consisting of photovoltaics, micro wind turbines, etc., are becoming increasingly popular (Camargo et al., 2019) and are playing a critical role in the energy transition (Palm and Eriksson, 2018). Although the contribution of renewable energy systems in saving household energy bills as well as environmental benefits has been proven, the high cost of equipment and the stability of energy storage systems have hindered its popularity in the household sector (Kiprop et al., 2019). For users, the high upfront costs and the risks to be taken are one of the main barriers (Ellabban and Alassi, 2019). For national governments, overly heavy energy allowances impose a financial burden (Hancevic et al., 2017). The analysis of technical, energy and economic benefits for renewable energy systems is a topic of focus for scholars (Lazzeroni et al., 2021). Alqahtani and Balta-Ozkan (2021) evaluated the optimal size of PV, battery storage capacity by building a techno-economic model. Figaj et al. (2020) attempted to build a new hybrid power system to reduce the reliance on traditional energy sources for household cooling and heating, but the results of the final simulation showed that it was less economic and competitive than the conventional system due to the high cost of the system components.
In addition, as most renewable energy systems are intermittent, and require energy storage systems to provide a continuous energy supply, their energy flexibility and balance of supply are yet to be tested (He et al., 2021; Li et al., 2017). Integrating microgrids to build a power sharing framework is one of the reliable methods available (Quiggin et al., 2012). Microgrids are small systems that integrate distributed energy sources, energy storage systems, and energy conversion, and monitoring devices into one. It helps to optimize generation, distribution, and consumption (AlSkaif et al., 2017). However, as energy storage and distribution systems, it is still a challenge to utilize renewable energy sources within the microgrid to generate electricity (Bashir et al., 2018), optimize community energy planning, and reduce operational and investment costs (David Mina-Casaran et al., 2021; Xu et al., 2022).
5.3 Enhancing energy-saving intentions
The molding of occupants’ behaviors toward greater energy-efficient is identified as a crucial approach in decreasing household energy consumption (Urquizo et al., 2017). Especially during the recent pandemic, with increased time spent at home, some households have more frequently utilized devices such as air conditioning, water heaters, and electricity, contributing to the rise in residential energy consumption levels. Beyond the replacement of high-efficiency appliances and the adoption of energy-saving technologies, there is a growing emphasis on the shift in individual energy-saving awareness and behavior (Bae et al., 2023; Conradie et al., 2023).
The factors influencing energy-saving behavior are diverse, including socio-economic factors, technological convenience, policy constraints, and individual awareness and attitudes (Cvetković et al., 2021; Nguyen et al., 2023). Among them, individual awareness and attitudes are crucial for the initiation and maintenance of energy-saving behavior (Xu Q et al., 2021). The balance between economic costs and benefits is a crucial prerequisite for implementing decisions related to energy-saving behavior, especially in low-income households (Hottman and Monarch, 2020; Birkmann et al., 2022). Due to the diversity of household characteristics, there is a higher demand for the availability and convenience of energy-saving technologies, which directly impacts behavioral decisions (Mills and Schleich, 2012; Adua, 2020). Additionally, incentives from policies and constraints from regulations can often encourage residents to adopt more energy-efficient behaviors to a certain extent (Ren et al., 2020). Stringent energy efficiency standards are common in European countries to drive households to adopt more efficient heating and cooling systems (DESNZ, 2023); the United States encourages the implementation of energy tax credits to encourage households to invest in energy efficiency improvements (Adebayo and Alola, 2023); and Japan incentivizes households to use smart technologies to improve energy management efficiency (Zhao et al., 2021).
Taking into account these factors, researchers and policymakers often implement behavior intervention policies to motivate occupants change habits, promoting the adoption of more sustainable energy practices. Behavioral interventions are usually carried out through awareness and education, information feedback, incentive measures, and social norms. Knowledge dissemination and education is a key first step in modeling eco-energy behavior (Grodek-Szostak et al., 2021), usually through information campaigns, public services, or workshops. Studies have confirmed that implementing education and awareness campaigns can significantly reduce energy waste (Song and Leng, 2020). Zhang J et al. (2021) evaluated the positive impact of low-carbon knowledge promotion on energy saving behavior by constructing an integrated “knowledge-intention-behavior” model.
Providing real-time feedback to households on their energy use is an incentive to reduce energy consumption (Ruokamo et al., 2022). Most of the information feedback is currently done through smart grid tools, or other monitoring tools (Kendel et al., 2017; Ozawa et al., 2017; Lazowski et al., 2018) combined with regression analysis, found the impact of energy feedback reports on household lifestyles, and contributed to a reduction in household electricity consumption. The economic incentives and government financial subsidy policies for reducing energy consumption, can motivate households to adopt energy efficiency measures and increase welfare (Vuelvas and Ruiz, 2017; Zhang G et al., 2021). In the face of rapid residential electrification, increasing the share of energy-saving appliances in households is one of the effective strategies. However, emerging small appliances have not yet attracted much attention as ownership is not saturated (Cabeza et al., 2018). Interventions based on social norms play an equally important role in shaping energy-efficient behavior (Alberini and Filippini, 2018; Ru et al., 2018). Most of the mitigation measures lack heterogeneity in the consumer population, which is not substantial for low-income, elderly households (Trotta et al., 2020).
6 Conclusion
The household sector is a major contributor to the increase of energy consumption and carbon emissions in a country, and the negative environmental impacts caused by its consumption activities and household behavior are seriously hindering sustainable economic and social development. This paper combines a bibliometric approach to summarize and review current work related to HEC. Analysis on the overall structure of the screened 619 papers was conducted, with descriptive statistics on research clusters and major contributors related to the topic, etc. A discussion is focused on the current mainstream research methods, relevant measures regarding household energy efficiency, and attempts to explore the values played and challenges faced by HEC in the global carbon reduction movement. This paper found that,
1. Although HEC studies have been widely conducted, less in-depth studies have been conducted on developing countries. The trend towards reducing carbon emissions and addressing climate change has sparked a global research fervor in the field of household energy efficiency and renewable energy. However, there are variations in focus across different countries and regions. Cold regions such as Canada and the United States prioritize addressing heating demand under extreme climatic conditions, while Japan concentrates on adjusting energy policies and innovating energy-efficient technologies. In developing countries like India, optimizing energy structures and traditional consumption patterns emerge as prevalent trends.
2. For accounting methods or approaches, the IOA has standardized datasets, is relatively easy to use, and is easily adaptable to other methods. But it can only output the environmental impact of the product or service itself and cannot predict the subsequent economic or social impact. Also, the modeling is highly dependent on comprehensive national or local economic data and is not applicable to all regions of the study. LCA can reflect environmental, social, and economic impacts throughout the life cycle, but data collection and practical application are time-consuming and laborious.
3. For analysis methods, TPB and CLA both focus on the influence of consumer behavior on energy consumption by establishing a structural framework. The TPB focuses more on factors such as individual awareness and perceived behavior, The CLA focuses more on the role of social and cultural factors in shaping consumer behavior. Decomposition analysis is easy to understand and highly applicable. It has a prominent position in exploring HEC drivers and can identify and quantify the impact of different factors on energy consumption and environmental change.
4. For technology, many empirical studies have demonstrated the potential of technologies such as smart homes and renewable energy systems to play a role in household energy sustainability, high operational costs, technical and system barriers, lack of customer knowledge and the risks to be taken, etc., make diffusion extremely difficult.
Finally, this paper puts forward the future research direction of HEC. It is important to evaluate the effectiveness of energy policies and energy standards for the future development of HEC, particularly in developing countries. About household energy consumption behavior, low-income or elderly households will be the key focus. The energy consumption generated by small appliances cannot be ignored and more statistics need to be adopted to compensate. Similarly, we identified limitations in the study. The research on household energy consumption behavior is characterized by a singular data source, predominantly relying on observational data gathered through methods such as questionnaire surveys and interviews. The findings of these studies lack universality. In addition, the definition of HEC is not comprehensive, and the energy consumption of small appliances is often ignored, which hinders the accounting of HEC.
Author contributions
The manuscript was written through the contributions of all authors. TW: conceptualization, methodology, visualization, and writing–original draft. QZ: conceptualization, supervision, conceptualization, and writing–review and editing. WG: supervision, and writing–review and editing. XH: software, and visualization. All authors contributed to the article and approved the submitted version.
Funding
This work was supported by International Science and Technology Cooperation Project of the Ministry of Housing and Urban-Rural Development, China (2020-H-002) and the Kitakyushu Innovative Human Resource and Regional Development Program (JST grant code: JPMJSP2149).
Conflict of interest
The authors declare that the research was conducted in the absence of any commercial or financial relationships that could be construed as a potential conflict of interest.
Publisher’s note
All claims expressed in this article are solely those of the authors and do not necessarily represent those of their affiliated organizations, or those of the publisher, the editors and the reviewers. Any product that may be evaluated in this article, or claim that may be made by its manufacturer, is not guaranteed or endorsed by the publisher.
References
Abrahamse, W., Steg, L., Vlek, C., and Rothengatter, T. (2005). A review of intervention studies aimed at household energy conservation. J. Of Environ. Psychol. 25 (3), 273–291. doi:10.1016/j.jenvp.2005.08.002
Abushnaf, J., Rassau, A., and Gornisiewicz, W. (2016). Impact on electricity use of introducing time-of-use pricing to a multi-user home energy management system. Int. Trans. Electr. Energy Syst. 26 (5), 993–1005. doi:10.1002/etep.2118
Adebayo, T. S., and Alola, A. A. (2023). Drivers of natural gas and renewable energy utilization in the USA: how about household energy efficiency-energy expenditure and retail electricity prices? Energy 283, 129022. doi:10.1016/j.energy.2023.129022
Adha, R., Hong, C.-Y., Firmansyah, M., and Paranata, A. (2021). Rebound effect with energy efficiency determinants: a two-stage analysis of residential electricity consumption in Indonesia. Sustain. Prod. Consum. 28, 556–565. doi:10.1016/j.spc.2021.06.019
Adnan, N., and Shahrina, M. N. (2021). A comprehensive approach: diffusion of environment-friendly energy technologies in residential photovoltaic markets. Sustain. Energy Technol. Assessments 46, 101289. doi:10.1016/j.seta.2021.101289
Adua, L. (2020). Reviewing the complexity of energy behavior: technologies, analytical traditions, and household energy consumption data in the United States. Energy Res. Soc. Sci. 59, 101289. doi:10.1016/j.erss.2019.101289
Adua, L., York, R., and Schuelke-Leech, B. (2016). The human dimensions of climate change: a micro-level assessment of views from the ecological modernization, political economy and human ecology perspectives. Soc. Sci. Res. 56, 26–43. doi:10.1016/j.ssresearch.2015.10.003
Ajzen, I. (1985). From intentions to actions: a theory of planned behavior. Berlin, Heidelberg: Springer.
Ajzen, I. (1991). The theory of planned behavior. Theor. Cognitive Self-Regulation 50 (2), 179–211. doi:10.1016/0749-5978(91)90020-T
Alberini, A., and Filippini, M. (2018). Transient and persistent energy efficiency in the US residential sector: evidence from household-level data. ENERGY Effic. 11 (3), 589–601. doi:10.1007/s12053-017-9599-z
Alberini, A., Prettico, G., Shen, C., and Torriti, J. (2019). Hot weather and residential hourly electricity demand in Italy. ENERGY 177, 44–56. doi:10.1016/j.energy.2019.04.051
Alhussein, M., Aurangzeb, K., and Haider, S. (2020). Hybrid CNN-lstm model for short-term individual household load forecasting. IEEE ACCESS 8, 180544–180557. doi:10.1109/ACCESS.2020.3028281
Alqahtani, N., and Balta-Ozkan, N. (2021). Assessment of rooftop solar power generation to meet residential loads in the city of neom, Saudi Arabia. Energies 14 (13), 3805. doi:10.3390/en14133805
Alsaigh, R., Mehmood, R., and Katib, I. (2023). AI explainability and governance in smart energy systems: a review. Front. Energy Res. 11, 1071291. doi:10.3389/fenrg.2023.1071291
AlSkaif, T., Lampropoulos, I., van den Broek, M., and van Sark, W. (2018). Gamification-based framework for engagement of residential customers in energy applications. Energy Res. Soc. Sci. 44, 187–195. doi:10.1016/j.erss.2018.04.043
AlSkaif, T., Luna, A., Zapata, M., Guerrero, J., and Bellalta, B. (2017). Reputation-based joint scheduling of households appliances and storage in a microgrid with a shared battery. Energy Build. 138, 228–239. doi:10.1016/j.enbuild.2016.12.050
Amber, K. P., Ahmad, R., Farmanbar, M., Bashir, M. A., Mehmood, S., Khan, M. S., et al. (2021). Unlocking household electricity consumption in Pakistan. Buildings 11 (11), 566. doi:10.3390/buildings11110566
Amin, A., Bte Mohamed Yusoff, N. Y., Yousaf, H., Peng, S., Işık, C., Akbar, M., et al. (2023). The influence of renewable and non-renewable energy on carbon emissions in Pakistan: evidence from stochastic impacts by regression on population, affluence, and technology model. Front. Environ. Sci. 11, 1182055. doi:10.3389/fenvs.2023.1182055
An, J., Yan, D., and Hong, T. (2018). Clustering and statistical analyses of air-conditioning intensity and use patterns in residential buildings. Energy Build. 174, 214–227. doi:10.1016/j.enbuild.2018.06.035
Andor, M. A., Bernstein, D. H., and Sommer, S. (2021). Determining the efficiency of residential electricity consumption. Empir. Econ. 60 (6), 2897–2923. doi:10.1007/s00181-020-01967-4
Asensio, O., and Delmas, M. (2015). Nonprice incentives and energy conservation. Proc. Of Natl. Acad. Of Sci. Of U. S. A. 112 (6), E510–E515. doi:10.1073/pnas.1401880112
Bae, C., Lee, J. Y., Kim, D., and Chun, C. (2023). A study on the impact of residents’ energy usage behavior on heating energy consumption based on smart meter data and surveys. Energy Build. 300, 113634. doi:10.1016/j.enbuild.2023.113634
Bai, Y., Deng, X., Gibson, J., Zhao, Z., and Xu, H. (2019). How does urbanization affect residential CO2 emissions? An analysis on urban agglomerations of China. J. Of Clean. Prod. 209, 876–885. doi:10.1016/j.jclepro.2018.10.248
Bak, J., and Yoon, S. (2021). Dwelling infiltration and heating energy demand in multifamily high-rise and low-energy buildings in Korea. Renew. Sustain. Energy Rev. 148, 111284. doi:10.1016/j.rser.2021.111284
Baltruszewicz, M., Steinberger, J., Ivanova, D., Brand-Correa, L., Paavola, J., and Owen, A. (2021). Household final energy footprints in Nepal, Vietnam and Zambia: composition, inequality and links to well-being. Environ. Res. Lett. 16 (2), 025011. doi:10.1088/1748-9326/abd588
Bashir, A. A., Kasmaei, M. P., Safdarian, A., and Lehtonen, M. (2018). Matching of local load with on-site PV production in a grid-connected residential building. ENERGIES 11 (9), 2409. doi:10.3390/en11092409
Bataineh, K., and Alrabee, A. (2018). Improving the energy efficiency of the residential buildings in Jordan. Buildings 8 (7), 85. doi:10.3390/buildings8070085
Belaid, F., and Garcia, T. (2016). Understanding the spectrum of residential energy-saving behaviours: French evidence using disaggregated data. Energy Econ. 57, 204–214. doi:10.1016/j.eneco.2016.05.006
Berrill, P., Gillingham, K. T., and Hertwich, E. G. (2021). Drivers of change in US residential energy consumption and greenhouse gas emissions, 1990-2015. Environ. Res. Lett. 16 (3), 034045. doi:10.1088/1748-9326/abe325
Besagni, G., and Borgarello, M. (2019). The socio-demographic and geographical dimensions of fuel poverty in Italy. Energy Res. Soc. Sci. 49, 192–203. doi:10.1016/j.erss.2018.11.007
Bezerra, P., da Silva, F., Cruz, T., Mistry, M., Vasquez-Arroyo, E., Magalar, L., et al. (2021). Impacts of a warmer world on space cooling demand in Brazilian households. Energy Build. 234, 110696. doi:10.1016/j.enbuild.2020.110696
Bhattacharjee, S., and Reichard, G. (2011). Socio-economic factors affecting individual household energy consumption: a systematic review. Energy Sustain. 54686, 891–901. doi:10.1115/ES2011-54615
Bin, S., and Dowlatabadi, H. (2005). Consumer lifestyle approach to US energy use and the related CO2 emissions. Energy Policy 33 (2), 197–208. doi:10.1016/S0301-4215(03)00210-6
Birkmann, J., Liwenga, E., Pandey, R., Boyd, E., Djalante, R., Gemenne, F., et al. (2022). Poverty, livelihoods and sustainable development.
Borozan, D. (2018). Regional-level household energy consumption determinants: the european perspective. Renew. Sustain. Energy Rev. 90, 347–355. doi:10.1016/j.rser.2018.03.038
Boukarta, S., and Berezowska-Azzag, E. (2018). Assessing households’ gas and electricity consumption: a case study of djelfa, Algeria. Quaest. Geogr. 37 (4), 111–129. doi:10.2478/quageo-2018-0034
Brounen, D., Kok, N., and Quigley, J. M. (2012). Residential energy use and conservation: economics and demographics. Eur. Econ. Rev. 56 (5), 931–945. doi:10.1016/j.euroecorev.2012.02.007
Bülbül, H., Topal, A., Özoğlu, B., and Büyükkeklik, A. (2023). Assessment of determinants for households’ pro-environmental behaviours and direct emissions. J. Clean. Prod. 415, 137892. doi:10.1016/j.jclepro.2023.137892
Cabeza, L. F., Urge-Vorsatz, D., Urge, D., Palacios, A., and Barreneche, C. (2018). Household appliances penetration and ownership trends in residential buildings. Renew. Sustain. Energy Rev. 98, 1–8. doi:10.1016/j.rser.2018.09.006
Calvin Nsangou, J., Kenfack, J., Nzotcha, U., and Tamo, T. T. (2020). Assessment of the potential for electricity savings in households in Cameroon: a stochastic frontier approach. Energy 211, 118576. doi:10.1016/j.energy.2020.118576
Camargo, L. R., Nitsch, F., Gruber, K., Valdes, J., Wuth, J., and Dorner, W. (2019). Potential analysis of hybrid renewable energy systems for self-sufficient residential use in Germany and the Czech republic. Energies 12 (21), 4185. doi:10.3390/en12214185
Cao, Q., Kang, W., Xu, S., Sajid, M. J., and Cao, M. (2019). Estimation and decomposition analysis of carbon emissions from the entire production cycle for Chinese household consumption. J. Of Environ. Manag. 247, 525–537. doi:10.1016/j.jenvman.2019.06.044
Chang, C., Zhu, N., Yang, K., and Yang, F. (2018). Data and analytics for heating energy consumption of residential buildings: the case of a severe cold climate region of China. Energy Build. 172, 104–115. doi:10.1016/j.enbuild.2018.04.037
Chaudhuri, K., and Huaccha, G. (2023). Who bears the energy cost? Local income deprivation and the household energy efficiency gap. Energy Econ. 127, 107062. doi:10.1016/j.eneco.2023.107062
Chen, C., Liu, G., Meng, F., Hao, Y., Zhang, Y., and Casazza, M. (2019). Energy consumption and carbon footprint accounting of urban and rural residents in Beijing through Consumer Lifestyle Approach. Ecol. Indic. 98, 575–586. doi:10.1016/j.ecolind.2018.11.049
Chen, Y., and Lin, S. (2015). Study on factors affecting energy-related per capita carbon dioxide emission by multi-sectoral of cities: a case study of Tianjin. Nat. Hazards 77 (2), 833–846. doi:10.1007/s11069-015-1632-y
CLEAN Future Act (2021). 117th congress. Available at: https://www.congress.gov/.
Conradie, P., Van Hove, S., Pelka, S., Karaliopoulos, M., Anagnostopoulos, F., Brugger, H., et al. (2023). Why do people turn down the heat? Applying behavioural theories to assess reductions in space heating and energy consumption in Europe. Energy Res. Soc. Sci. 100, 103059. doi:10.1016/j.erss.2023.103059
Copiello, S., and Gabrielli, L. (2017). Analysis of building energy consumption through panel data: the role played by the economic drivers. Energy Build. 145, 130–143. doi:10.1016/j.enbuild.2017.03.053
Cvetković, D., Nešović, A., and Terzić, I. (2021). Impact of people’s behavior on the energy sustainability of the residential sector in emergency situations caused by COVID-19. Energy Build. 230, 110532. doi:10.1016/j.enbuild.2020.110532
Daioglou, V., van Ruijven, B. J., and van Vuuren, D. P. (2012). Model projections for household energy use in developing countries. Energy 37 (1), 601–615. doi:10.1016/j.energy.2011.10.044
Das, R., Richman, R., and Brown, C. (2018). Demographic determinants of Canada’s households’ adoption of energy efficiency measures: observations from the Households and Environment Survey, 2013. Energy Effic. 11 (2), 465–482. doi:10.1007/s12053-017-9578-4
David Mina-Casaran, J., Fernando Echeverry, D., Arturo Lozano, C., and Navarro-Espinosa, A. (2021). On the value of community association for microgrid development: learnings from multiple deterministic and stochastic planning designs. Appl. Sciences-Basel 11 (14), 6257. doi:10.3390/app11146257
De Boeck, L., Van Asch, S., De Bruecker, P., and Audenaert, A. (2016). Comparison of support policies for residential photovoltaic systems in the major EU markets through investment profitability. Renew. Energy 87 (1), 42–53. doi:10.1016/j.renene.2015.09.063
Ding, Y., Qu, W., Niu, S., Liang, M., Qiang, W., and Hong, Z. (2016). Factors influencing the spatial difference in household energy consumption in China. Sustainability 8 (12), 1285. doi:10.3390/su8121285
Dioha, M. (2018). Modelling the impact of Nigeria household energy policies on energy consumption and CO2 emissions. Eng. Thail. 22 (6), 1–19. doi:10.4186/ej.2018.22.6.1
Du, W., Cohen, A., Shen, G., Ru, M., Shen, H., and Tao, S. (2018). Fuel use trends for boiling water in rural China (1992-2012) and environmental health implications: a national cross-sectional study. Environ. Sci. Technol. 52 (21), 12886–12894. doi:10.1021/acs.est.8b02389
Duan, M., Li, L., Liu, X., Pei, J., and Song, H. (2023). Turning awareness into behavior: meta-analysis of household residential life energy transition behavior from the dual perspective of internal driving forces and external inducing forces. Energy 279, 128072. doi:10.1016/j.energy.2023.128072
Eirinaki, M., Varlamis, I., Dahihande, J., Jaiswal, A., Pagar, A. A., and Thakare, A. (2022). Real-time recommendations for energy-efficient appliance usage in households. Front. Big Data 5, 972206. doi:10.3389/fdata.2022.972206
Elasu, J., Ntayi, J. M., Adaramola, M. S., and Buyinza, F. (2023). Drivers of household transition to clean energy fuels: a systematic review of evidence. Renew. Sustain. Energy Transition 3, 100047. doi:10.1016/j.rset.2023.100047
El Jaouhari, S., Jose Palacios-Garcia, E., Anvari-Moghaddam, A., and Bouabdallah, A. (2019). Integrated management of energy, wellbeing and health in the next generation of smart homes. Sensors 19 (3), 481. doi:10.3390/s19030481
Ellabban, O., and Alassi, A. (2019). Integrated Economic Adoption Model for residential grid-connected photovoltaic systems: an Australian case study. Energy Rep. 5, 310–326. doi:10.1016/j.egyr.2019.02.004
Elmorshedy, M. F., Elkadeem, M. R., Kotb, K. M., Taha, I. B. M., and Mazzeo, D. (2021). Optimal design and energy management of an isolated fully renewable energy system integrating batteries and supercapacitors. Energy Convers. Manag. 245, 114584. doi:10.1016/j.enconman.2021.114584
Energy Efficiency Directive (2018). European commission. Available at: https://energy.ec.europa.eu/.
Estebsari, A., and Rajabi, R. (2020). Single residential load forecasting using deep learning and image encoding techniques. Electronics 9 (1), 68. doi:10.3390/electronics9010068
Fernandes, M., Viegas, J., Vieira, S., and Sousa, J. (2017). Segmentation of residential gas consumers using clustering analysis. Energies 10 (12), 2047. doi:10.3390/en10122047
Figaj, R., Zoladek, M., and Goryl, W. (2020). Dynamic simulation and energy economic analysis of a household hybrid ground-solar-wind system using TRNSYS software. Energies 13 (14), 3523. doi:10.3390/en13143523
Filippini, M., and Pachauri, S. (2004). Elasticities of electricity demand in urban Indian households. Energy Policy 32 (3), 429–436. doi:10.1016/S0301-4215(02)00314-2
Gajowniczek, K., and Zabkowski, T. (2017). Electricity forecasting on the individual household level enhanced based on activity patterns. PLOS ONE 12 (4), e0174098. doi:10.1371/journal.pone.0174098
Gao, B., Liu, X., Zhang, W., and Tang, Y. (2015). Autonomous household energy management based on a double cooperative game approach in the smart grid. Energies 8 (7), 7326–7343. doi:10.3390/en8077326
García-Valiñas, M., Arbués, F., and Balado-Naves, R. (2023). Assessing environmental profiles: an analysis of water consumption and waste recycling habits. J. Environ. Manag. 348, 119247. doi:10.1016/j.jenvman.2023.119247
Gholami, R., Nishant, R., and Emrouznejad, A. (2021). Modeling residential energy consumption: an application of IT-based solutions and big data analytics for sustainability. J. Glob. Inf. Manag. 29 (2), 166–193. doi:10.4018/JGIM.2021030109
Goldstein, B., Gounaridis, D., and Newell, J. (2020). The carbon footprint of household energy use in the United States. Proc. Natl. Acad. Of Sci. Of U. S. A. 117 (32), 19122–19130. doi:10.1073/pnas.1922205117
Gonçalves, L., and Patrício, L. (2022). From smart technologies to value cocreation and customer engagement with smart energy services. Energy Policy 170, 113249. doi:10.1016/j.enpol.2022.113249
Granell, R., Axon, C., and Wallom, D. (2015). Impacts of raw data temporal resolution using selected clustering methods on residential electricity load profiles. IEEE Trans. Power Syst. 30 (6), 3217–3224. doi:10.1109/TPWRS.2014.2377213
Greenblatt, J., Hopkins, A., Letschert, V., and Blasnik, M. (2013). Energy use of US residential refrigerators and freezers: function derivation based on household and climate characteristics. Energy Effic. 6 (1), 135–162. doi:10.1007/s12053-012-9158-6
Grodek-Szostak, Z., Malinowski, M., Suder, M., Kwiecien, K., Bodziacki, S., Vaverkova, M. D., et al. (2021). Energy conservation behaviors and awareness of polish, Czech and Ukrainian students: a case study. Energies 14 (18), 5599. doi:10.3390/en14185599
Guo, J., Xu, Y., Qu, Y., Wang, Y., and Wu, X. (2023). Exploring factors affecting household energy consumption in the internet era: empirical evidence from Chinese households. Energy Policy 183, 113810. doi:10.1016/j.enpol.2023.113810
Guta, D., Baumgartner, J., Jack, D., Carter, E., Shen, G., Orgill-Meyer, J., et al. (2022). A systematic review of household energy transition in low and middle income countries. Energy Res. Soc. Sci. 86, 102463. doi:10.1016/j.erss.2021.102463
Haben, S., Singleton, C., and Grindrod, P. (2016). Analysis and clustering of residential customers energy behavioral demand using smart meter data. IEEE Trans. Smart Grid 7 (1), 136–144. doi:10.1109/TSG.2015.2409786
Hafner, R. J., Elmes, D., and Read, D. (2019). Promoting behavioural change to reduce thermal energy demand in households: a review. Renew. Sustain. Energy Rev. 102, 205–214. doi:10.1016/j.rser.2018.12.004
Hamilton, I. G., Steadman, P. J., Bruhns, H., Summerfield, A. J., and Lowe, R. (2013). Energy efficiency in the British housing stock: energy demand and the homes energy efficiency database. Energy Policy 60, 462–480. doi:10.1016/j.enpol.2013.04.004
Han, J., Pei, J., and Tong, H. (2022). Data mining: concepts and techniques. Waltham, MA: Morgan kaufmann.
Hancevic, P. I., Nunez, H. M., and Rosellon, J. (2017). Distributed photovoltaic power generation: possibilities, benefits, and challenges for a widespread application in the Mexican residential sector. Energy Policy 110, 478–489. doi:10.1016/j.enpol.2017.08.046
Hassen, S., Anandarajah, G., and Seifemichael, R. (2023). Behavioral and socio-economic determinants of urban households’ investment in energy efficient technologies: evidence from Ethiopia. Front. Energy Res. 11, 1135291. doi:10.3389/fenrg.2023.1135291
He, Y., Zhou, Y., Yuan, J., Liu, Z., Wang, Z., and Zhang, G. (2021). Transformation towards a carbon-neutral residential community with hydrogen economy and advanced energy management strategies. Energy Convers. Manag. 249, 114834. doi:10.1016/j.enconman.2021.114834
Heesen, F., and Madlener, R. (2021). Revisiting heat energy consumption modeling: household production theory applied to field experimental data. Energy Policy 158, 112511. doi:10.1016/j.enpol.2021.112511
Heinisch, V., Odenberger, M., Goransson, L., and Johnsson, F. (2019). Organizing prosumers into electricity trading communities: costs to attain electricity transfer limitations and self-sufficiency goals. Int. J. Energy Res. 43 (13), 4720. doi:10.1002/er.4720
Heng, Y., Lu, C.-L., Yu, L., and Gao, Z. (2020). The heterogeneous preferences for solar energy policies among US households. Energy Policy 137, 111187. doi:10.1016/j.enpol.2019.111187
Hertwich, E. G. (2005). Life cycle approaches to sustainable consumption: a critical review. Environ. Sci. Technol. 39 (13), 4673–4684. doi:10.1021/es0497375
Hollebeek, L. D., Srivastava, R. K., and Chen, T. (2019). SD logic–informed customer engagement: integrative framework, revised fundamental propositions, and application to CRM. J. Acad. Mark. Sci. 47, 161–185. doi:10.1007/s11747-016-0494-5
Hottman, C. J., and Monarch, R. (2020). A matter of taste: estimating import price inflation across U.S. income groups. J. Int. Econ. 127, 103382. doi:10.1016/j.jinteco.2020.103382
Hu, Y., Zhang, Q., Hu, S., Xiao, G., Chen, X., Wang, J., et al. (2022). Research progress and prospects of ecosystem carbon sequestration under climate change (1992–2022). Ecol. Indic. 145, 109656. doi:10.1016/j.ecolind.2022.109656
Iqbal, M., Sajjad, M., Amin, S., Haroon, S., Liaqat, R., Khan, M., et al. (2019). Optimal scheduling of residential home appliances by considering energy storage and stochastically modelled photovoltaics in a grid exchange environment using hybrid grey wolf genetic algorithm optimizer. Appl. Sciences-Basel 9 (23), 5226. doi:10.3390/app9235226
Jafari, H. (2023). Energy storage by improving energy-efficiency of electricity home appliances under governmental supporting policies: a game-theoretic approach. J. Energy Storage 63, 106972. doi:10.1016/j.est.2023.106972
Jamil, K., Dunnan, L., Awan, F. H., Jabeen, G., Gul, R. F., Idrees, M., et al. (2022). Antecedents of consumer’s purchase intention towards energy-efficient home appliances: an agenda of energy efficiency in the post COVID-19 era. Front. Energy Res. 10, 863127. doi:10.3389/fenrg.2022.863127
Jia, W., and Wu, S. (2022). Spatial differences and influencing factors of energy poverty: evidence from provinces in China. Front. Environ. Sci. 10, 921374. doi:10.3389/fenvs.2022.921374
Jiang, L., Xue, B., Xing, R., Chen, X., Song, L., Wang, Y., et al. (2020). Rural household energy consumption of farmers and herders in the Qinghai-Tibet Plateau. Energy 192, 116649. doi:10.1016/j.energy.2019.116649
Jin, X., Li, Y., Sun, D., Zhang, J., and Zheng, J. (2019). Factors controlling urban and rural indirect carbon dioxide emissions in household consumption: a case study in beijing. Sustainability 11 (23), 6563. doi:10.3390/su11236563
Justo, D., Minussi, C., and Lotufo, A. (2017). Behavioral similarity of residential customers using a neural network based on adaptive resonance theory. Sustain. Cities Soc. 35, 483–493. doi:10.1016/j.scs.2017.08.029
Kaufmann, M., Veenman, S., Haarbosch, S., and Jansen, E. (2023). How policy instruments reproduce energy vulnerability—a qualitative study of Dutch household energy efficiency measures. Energy Res. Soc. Sci. 103, 103206. doi:10.1016/j.erss.2023.103206
Kendel, A., Lazaric, N., and Marechal, K. (2017). What do people `learn by looking’ at direct feedback on their energy consumption? Results of a field study in Southern France. Energy Policy 108, 593–605. doi:10.1016/j.enpol.2017.06.020
Khan, Z., Ullah, A., Ullah, W., Rho, S., Lee, M., and Baik, S. (2020). Electrical energy prediction in residential buildings for short-term horizons using hybrid deep learning strategy. Appl. Sciences-Basel 10 (23), 8634. doi:10.3390/app10238634
Khosla, R., Sircar, N., and Bhardwaj, A. (2019). Energy demand transitions and climate mitigation in low-income urban households in India. Environ. Res. Lett. 14 (9), 095008. doi:10.1088/1748-9326/ab3760
Khurshid, N., Shah, A., and Munir, F. (2023). Impact of socio-demographic, psychological and emotional factors on household direct and indirect electricity saving behavior: a case study of Pakistan. J. Clean. Prod. 429, 139581. doi:10.1016/j.jclepro.2023.139581
Kim, S., Jung, S., and Baek, S. (2019). A model for predicting energy usage pattern types with energy consumption information according to the behaviors of single-person households in South Korea. Sustainability 11 (1), 245. doi:10.3390/su11010245
Kim, Y.-G., Yoo, J., and Oh, W. (2015). Driving forces of rapid CO2 emissions growth: a case of Korea. Energy Policy 82, 144–155. doi:10.1016/j.enpol.2015.03.017
Kiprop, E., Matsui, K., and Maundu, N. (2019). The role of household consumers in adopting renewable energy technologies in Kenya. Environments 6 (8), 95. doi:10.3390/environments6080095
Komninos, N., Philippou, E., and Pitsillides, A. (2014). Survey in smart grid and smart home security: issues, challenges and countermeasures. IEEE Commun. Surv. Tutorials 16 (4), 1933–1954. doi:10.1109/COMST.2014.2320093
Kumar, P., Caggiano, H., Shwom, R., Felder, F. A., and Andrews, C. J. (2023). Saving from home! How income, efficiency, and curtailment behaviors shape energy consumption dynamics in US households? Energy 271, 126988. doi:10.1016/j.energy.2023.126988
Kwon, Y., Kim, T., Baek, K., and Kim, J. (2020). Multi-objective optimization of home appliances and electric vehicle considering customer’s benefits and offsite shared photovoltaic curtailment. Energies 13 (11), 2852. doi:10.3390/en13112852
Lazowski, B., Parker, P., and Rowlands, I. H. (2018). Towards a smart and sustainable residential energy culture: assessing participant feedback from a long-term smart grid pilot project. Energy Sustain. Soc. 8, 27. doi:10.1186/s13705-018-0169-9
Lazzeroni, P., Mariuzzo, I., Quercio, M., and Repetto, M. (2021). Economic, energy, and environmental analysis of PV with battery storage for Italian households. Electronics 10 (2), 146. doi:10.3390/electronics10020146
Leitao, J., Fonseca, C., Gil, P., Ribeiro, B., and Cardoso, A. (2021). A compressive receding horizon approach for smart home energy management. IEEE Access 9, 100407–100435. doi:10.1109/ACCESS.2021.3093277
Li, H., Zhao, Y., Wang, S., and Liu, Y. (2020). Spatial-temporal characteristics and drivers of the regional residential CO2 emissions in China during 2000-2017. J. Clean. Prod. 276, 124116. doi:10.1016/j.jclepro.2020.124116
Li, R., Dane, G., Finck, C., and Zeiler, W. (2017). Are building users prepared for energy flexible buildings? A large-scale survey in The Netherlands. Appl. Energy 203, 623–634. doi:10.1016/j.apenergy.2017.06.067
Liaqat, R., Sajjad, I., Waseem, M., and Alhelou, H. (2021). Appliance level energy characterization of residential electricity demand: prospects, challenges and recommendations. IEEE Access 9, 148676–148697. doi:10.1109/ACCESS.2021.3123196
Liu, L., Qu, J., Maraseni, T. N., Niu, Y., Zeng, J., Zhang, L., et al. (2020). Household CO2 emissions: current status and future perspectives. Int. J. Environ. Res. Public Health 17 (19), 7077. doi:10.3390/ijerph17197077
Liu, X., Wang, Q., Wei, H., Chi, H., Ma, Y., and Jian, I. (2020). Psychological and demographic factors affecting household energy-saving intentions: a TPB-based study in northwest China. Sustainability 12 (3), 836. doi:10.3390/su12030836
Long, Y., Yoshida, Y., Meng, J., Guan, D., Yao, L., and Zhang, H. (2019). Unequal age-based household emission and its monthly variation embodied in energy consumption—a cases study of Tokyo, Japan. Appl. Energy 247, 350–362. doi:10.1016/j.apenergy.2019.04.019
Louis, J.-N., Calo, A., Leiviskä, K., and Pongrácz, E. (2015). Environmental impacts and benefits of smart home automation: life cycle assessment of home energy management system. 8th Vienna Int. Conf. Math. Model. 48 (1), 880–885. doi:10.1016/j.ifacol.2015.05.158
Louise, J., and Pongracz, E. (2017). Life cycle impact assessment of home energy management systems (HEMS) using dynamic emissions factors for electricity in Finland. Environ. Impact Assess. Rev. 67, 109–116. doi:10.1016/j.eiar.2017.08.009
Ludtke, D., Luetkemeier, R., Schneemann, M., and Liehr, S. (2021). Increase in daily household water demand during the first wave of the covid-19 pandemic in Germany. Water 13 (3), 260. doi:10.3390/w13030260
Luo, J., Yang, J., Wan, S., Zhao, J., and Liang, J. (2023). Can energy conservation and emission reduction policies affect household carbon emissions? Evidence from China. Front. Energy Res. 11, 1138467. doi:10.3389/fenrg.2023.1138467
Mainimo, E. N., Okello, D. M., Mambo, W., and Mugonola, B. (2022). Drivers of household demand for cooking energy: a case of Central Uganda. Heliyon 8 (3), e09118. doi:10.1016/j.heliyon.2022.e09118
Makki, A., Stewart, R., Beal, C., and Panuwatwanich, K. (2015). Novel bottom-up urban water demand forecasting model: revealing the determinants, drivers and predictors of residential indoor end-use consumption. Resour. Conservation Recycl. 95, 15–37. doi:10.1016/j.resconrec.2014.11.009
Maljkovic, D., Lenz, N., and Zikovic, S. (2022). The pitfalls of shared metering: does the self-interest in district heating systems cause tragedy of the commons. Energy Res. Soc. Sci. 83, 102335. doi:10.1016/j.erss.2021.102335
Mashhoodi, B., Stead, D., and van Timmeren, A. (2020). Spatializing household energy consumption in The Netherlands: socioeconomic, urban morphology, microclimate, land surface temperature and vegetation data. Data Brief 29, 105118. doi:10.1016/j.dib.2020.105118
Masrahi, A., Wang, J., and Abudiyah, A. (2021). Factors influencing consumers’ behavioral intentions to use renewable energy in the United States residential sector. Energy Rep. 7, 7333–7344. doi:10.1016/j.egyr.2021.10.077
McAndrew, R., Mulcahy, R., Gordon, R., and Russell-Bennett, R. (2021). Household energy efficiency interventions: a systematic literature review. Energy Policy 150, 112136. doi:10.1016/j.enpol.2021.112136
McLoughlin, F., Duffy, A., and Conlon, M. (2012). Characterising domestic electricity consumption patterns by dwelling and occupant socio-economic variables: an Irish case study. Energy Build. 48, 240–248. doi:10.1016/j.enbuild.2012.01.037
McLoughlin, F., Duffy, A., and Conlon, M. (2015). A clustering approach to domestic electricity load profile characterisation using smart metering data. Appl. Energy 141, 190–199. doi:10.1016/j.apenergy.2014.12.039
MEE (2022). Progress on the implementation of China’s nationally determined contributions. Ministry of Ecology and Environment of People’s Republic of China https://www.mee.gov.cn/.
Meng, M., Wu, S., Zhou, J., and Wang, X. (2019). What is currently driving the growth of China’s household electricity consumption? A clustering and decomposition analysis. Sustainability 11 (17), 4648. doi:10.3390/su11174648
Michelle Lewis (2022). 26% of US households use electricity as their only energy source – but there’s a caveat. https://electrek.co/.
Mills, B., and Schleich, J. (2012). Residential energy-efficient technology adoption, energy conservation, knowledge, and attitudes: an analysis of European countries. Energy Policy 49, 616–628. doi:10.1016/j.enpol.2012.07.008
Mitra, D., Chu, Y., and Cetin, K. (2021). Cluster analysis of occupancy schedules in residential buildings in the United States. Energy Build. 236, 110791. doi:10.1016/j.enbuild.2021.110791
Moadab, N., Olsson, T., Fischl, G., and Aries, M. (2021). Smart versus conventional lighting in apartments-Electric lighting energy consumption simulation for three different households. Energy Build. 244, 111009. doi:10.1016/j.enbuild.2021.111009
Nakano, S., and Washizu, A. (2019). In which time slots can people save power? An analysis using a Japanese survey on time use. Sustainability 11 (16), 4444. doi:10.3390/su11164444
Namazkhan, M., Albers, C., and Steg, L. (2020). A decision tree method for explaining household gas consumption: the role of building characteristics, socio-demographic variables, psychological factors and household behaviour. Renew. Sustain. Energy Rev. 119, 109542. doi:10.1016/j.rser.2019.109542
Nguyen, T. T., Nguyen, D. N., and Pham, H. T. L. (2023). Survey data on energy-saving policies, energy price, crisis and household energy-saving behavior. Data Brief 51, 109646. doi:10.1016/j.dib.2023.109646
Nichols, B., and Kockelman, K. (2014). Life-cycle energy implications of different residential settings: recognizing buildings, travel, and public infrastructure. Energy Policy 68, 232–242. doi:10.1016/j.enpol.2013.12.062
Nie, H., Zhou, T., Lu, H., and Huang, S. (2021). Evaluation of the efficiency of Chinese energy-saving household appliance subsidy policy: an economic benefit perspective. Energy Policy 149, 112059. doi:10.1016/j.enpol.2020.112059
Oladokun, M. G., and Odesola, I. A. (2015). Household energy consumption and carbon emissions for sustainable cities–A critical review of modelling approaches. Int. J. Sustain. Built Environ. 4 (2), 231–247. doi:10.1016/j.ijsbe.2015.07.005
Orset, C. (2021). Is information a good policy instrument to influence the energy behaviour of households? Energy Econ. 102, 105451. doi:10.1016/j.eneco.2021.105451
Ouyang, J., Gao, L., Yan, Y., Hokao, K., and Ge, J. (2009). Effects of improved consumer behavior on energy conservation in the urban residential sector of hangzhou, China. J. Asian Archit. Build. Eng. 8 (1), 243–249. doi:10.3130/jaabe.8.243
Ozawa, A., Furusato, R., and Yoshida, Y. (2017). Tailor-made feedback to reduce residential electricity consumption: the effect of information on household lifestyle in Japan. Sustainability 9 (4), 528. doi:10.3390/su9040528
Pais-Magalhaes, V., Moutinho, V., and Robaina, M. (2020). Determinants of the household electricity consumption efficiency of an ageing population: evidence for the EU-28. Energy Rep. 6, 415–422. doi:10.1016/j.egyr.2020.11.193
Palm, J., and Eriksson, E. (2018). Residential solar electricity adoption: how households in Sweden search for and use information. Energy Sustain. Soc. 8, 14. doi:10.1186/s13705-018-0156-1
Paneru, C. P., and Tarigan, A. K. M. (2023). Reviewing the impacts of smart energy applications on energy behaviours in Norwegian households. Renew. Sustain. Energy Rev. 183, 113511. doi:10.1016/j.rser.2023.113511
Pang, Z., Chen, Y., Zhang, J., O’Neill, Z., Cheng, H., and Dong, B. (2021). How much HVAC energy could be saved from the occupant-centric smart home thermostat: a nationwide simulation study. Appl. Energy 283, 116251. doi:10.1016/j.apenergy.2020.116251
Pinjari, A., and Bhat, C. (2021). Computationally efficient forecasting procedures for Kuhn-Tucker consumer demand model systems: application to residential energy consumption analysis. J. Choice Model. 39, 100283. doi:10.1016/j.jocm.2021.100283
Poom, A., and Ahas, R. (2016). How does the environmental load of household consumption depend on residential location? Sustainability 8 (9), 799. doi:10.3390/su8090799
Puranen, P., Kosonen, A., and Ahola, J. (2021). Techno-economic viability of energy storage concepts combined with a residential solar photovoltaic system: a case study from Finland. Appl. Energy 298, 117199. doi:10.1016/j.apenergy.2021.117199
Qerimi, D., Dimitrieska, C., Vasilevska, S., and Rrecaj, A. A. (2020). Modeling of the solar thermal energy use in urban areas. Civ. Eng. Journal-Tehran 6 (7), 1349–1367. doi:10.28991/cej-2020-03091553
Qin, X., Wu, H., Zhang, X., and Wang, W. (2022). The widening wealth inequality as a contributor to increasing household carbon emissions. Front. Earth Sci. 10, 872806. doi:10.3389/feart.2022.872806
Quiggin, D., Cornell, S., Tierney, M., and Buswell, R. (2012). A simulation and optimisation study: towards a decentralised microgrid, using real world fluctuation data. Energy 41 (1), 549–559. doi:10.1016/j.energy.2012.02.007
Rahmani, A., Mashayekh, J., Aboojafari, R., and Bonyadi Naeini, A. (2023). Determinants of households’ intention for investment in renewable energy projects. Renew. Energy 205, 823–837. doi:10.1016/j.renene.2023.01.096
Ramapragada, P., Tejaswini, D., Garg, V., Mathur, J., and Gupta, R. (2022). Investigation on air conditioning load patterns and electricity consumption of typical residential buildings in tropical wet and dry climate in India. Energy Inf. 5, 61. doi:10.1186/s42162-022-00228-1
Ramos, J., Moreno, M., Delgado, M., Dominguez, S., and Cabeza, L. (2019). Potential of energy flexible buildings: evaluation of DSM strategies using building thermal mass. Energy Build. 203, 109442. doi:10.1016/j.enbuild.2019.109442
Ren, G., Sunikka-Blank, M., and Zhang, X. (2020). Young urban households in Shanghai, China: characteristics of energy use and attitudes. Sustain. Cities Soc. 60, 102174. doi:10.1016/j.scs.2020.102174
Reuter, M., Patel, M. K., and Eichhammer, W. (2019). Applying ex post index decomposition analysis to final energy consumption for evaluating European energy efficiency policies and targets. Energy Effic. 12 (5), 1329–1357. doi:10.1007/s12053-018-09772-w
Roberts, M., Haghdadi, N., Bruce, A., and MacGill, I. (2019). Characterisation of Australian apartment electricity demand and its implications for low-carbon cities. Energy 180, 242–257. doi:10.1016/j.energy.2019.04.222
Roman-Collado, R., and Jose Colinet, M. (2018). Are labour productivity and residential living standards drivers of the energy consumption changes? Energy Econ. 74, 746–756. doi:10.1016/j.eneco.2018.07.030
Rong, Y., Jia, J., Ju, M., Chen, C., Zhou, Y., and Zhong, Y. (2021). Multi-perspective analysis of household carbon dioxide emissions from direct energy consumption by the methods of logarithmic mean Divisia index and sigma convergence in Central China. Sustainability 13 (16), 9285. doi:10.3390/su13169285
Ru, X., Wang, S., and Yan, S. (2018). Exploring the effects of normative factors and perceived behavioral control on individual’s energy-saving intention: an empirical study in eastern China. Resour. Conservation Recycl. 134, 91–99. doi:10.1016/j.resconrec.2018.03.001
Ruokamo, E., Merilainen, T., Karhinen, S., Raiha, J., Suur-Uski, P., Timonen, L., et al. (2022). The effect of information nudges on energy saving: observations from a randomized field experiment in Finland. Energy Policy 161, 112731. doi:10.1016/j.enpol.2021.112731
Rutland, T., and Aylett, A. (2008). The work of policy: actor networks, governmentality, and local action on climate change in portland, Oregon. Environ. Plan. D Soc. Space 26 (4), 627–646. doi:10.1068/d6907
Sadeghianpourhamami, N., Demeester, T., Benoit, D., Strobbe, M., and Develder, C. (2016). Modeling and analysis of residential flexibility: timing of white good usage. Appl. Energy 179, 790–805. doi:10.1016/j.apenergy.2016.07.012
Sato, I., and Narita, D. (2023). Use of nested multi-regional input–output analysis for the evaluation of subnational emission reduction policies: a case of Japanese prefectures. Sustain. Prod. Consum. 37, 294–305. doi:10.1016/j.spc.2023.03.003
Satre-Meloy, A., Diakonova, M., and Grunewald, P. (2020). Cluster analysis and prediction of residential peak demand profiles using occupant activity data. Appl. Energy 260, 114246. doi:10.1016/j.apenergy.2019.114246
Schwartz, D., Bruine de Bruin, W., Fischhoff, B., and Lave, L. (2015). Advertising energy saving programs: the potential environmental cost of emphasizing monetary savings. J. Exp. Psychol. Appl. 21 (2), 158–166. doi:10.1037/xap0000042
Serrano, S., Uerge-Vorsatz, D., Barreneche, C., Palacios, A., and Cabeza, L. F. (2017). Heating and cooling energy trends and drivers in Europe. Energy 119, 425–434. doi:10.1016/j.energy.2016.12.080
Sharma, A. (2023). Economic Survey 2023: India aims 45% reduction in emission intensity of its GDP by 2030 from 2005 levels. Available at: https://www.cnbctv18.com/.
Shi, S., Li, H., Ding, X., and Gao, X. (2020). Effects of household features on residential window opening behaviors: a multilevel logistic regression study. Build. Environ. 170, 106610. doi:10.1016/j.buildenv.2019.106610
Singh, V., Henriques, C., and Martins, A. (2018). Fostering investment on energy efficient appliances in India A multi perspective economic input-output lifecycle assessment. Energy 149, 1022–1035. doi:10.1016/j.energy.2018.01.140
Song, S., and Leng, H. (2020). Modeling the household electricity usage behavior and energy-saving management in severely cold regions. Energies 13 (21), 5581. doi:10.3390/en13215581
Srivastava, A., Van Passel, S., and Laes, E. (2019). Dissecting demand response: a quantile analysis of flexibility, household attitudes, and demographics. Energy Res. Soc. Sci. 52, 169–180. doi:10.1016/j.erss.2019.02.011
Stagnitta, R., Rocco, M., and Colombo, E. (2020). A complementary approach to traditional energy balances for assessing energy efficiency measures in final uses: the case of space heating and cooling in Argentina. Sustainability 12 (16), 6563. doi:10.3390/su12166563
Stephan, A., and Crawford, R. (2016). The relationship between house size and life cycle energy demand: implications for energy efficiency regulations for buildings. Energy 116, 1158–1171. doi:10.1016/j.energy.2016.10.038
Stojilovska, A., Guyet, R., Mahoney, K., Gouveia, J. P., Castaño-Rosa, R., Živčič, L., et al. (2022). Energy poverty and emerging debates: beyond the traditional triangle of energy poverty drivers. Energy Policy 169, 113181. doi:10.1016/j.enpol.2022.113181
Su, S., Ding, Y., Li, G., Li, X., Li, H., Skitmore, M., et al. (2023). Temporal dynamic assessment of household energy consumption and carbon emissions in China: from the perspective of occupants. Sustain. Prod. Consum. 37, 142–155. doi:10.1016/j.spc.2023.02.014
Subbiah, R., Pal, A., Nordberg, E. K., Marathe, A., and Marathe, M. V. (2017). Energy demand model for residential sector: a first principles approach. IEEE Trans. Sustain. Energy 8 (3), 1215–1224. doi:10.1109/TSTE.2017.2669990
Supasa, T., Hsiau, S., Lin, S., Wongsapai, W., and Wu, J. (2017). Household energy consumption behaviour for different demographic regions in Thailand from 2000 to 2010. Sustainability 9 (12), 2328. doi:10.3390/su9122328
Tan, C., Ooi, H., and Goh, Y. (2017). A moral extension of the theory of planned behavior to predict consumers’ purchase intention for energy-efficient household appliances in Malaysia. Energy Policy 107, 459–471. doi:10.1016/j.enpol.2017.05.027
Taniguchi-Matsuoka, A., Shimoda, Y., Sugiyama, M., Kurokawa, Y., Matoba, H., Yamasaki, T., et al. (2020). Evaluating Japan’s national greenhouse gas reduction policy using a bottom-up residential end-use energy simulation model. Appl. Energy 279, 115792. doi:10.1016/j.apenergy.2020.115792
Tao, J., and Yu, S. (2011). Implementation of energy efficiency standards of household refrigerator/freezer in China: potential environmental and economic impacts. Appl. Energy 88 (5), 1890–1905. doi:10.1016/j.apenergy.2010.11.015
Thomas, B., and Azevedo, I. (2013). Estimating direct and indirect rebound effects for US households with input-output analysis Part 1: theoretical framework. Ecol. Econ. 86, 199–210. doi:10.1016/j.ecolecon.2012.12.003
Tian, J., Lumbreras, J., Andrade, C., and Liao, H. (2017). Key sectors in carbon footprint responsibility at the city level: a case study of Beijing. Int. J. Clim. Change Strategies Manag. 9 (6), 749–776. doi:10.1108/IJCCSM-01-2017-0009
Tilov, I., Farsi, M., and Volland, B. (2020). From frugal Jane to wasteful John: a quantile regression analysis of Swiss households’ electricity demand. Energy Policy 138, 111246. doi:10.1016/j.enpol.2020.111246
Tleuken, A., Tokazhanov, G., Serikbay, A., Zhalgasbayev, K., Guney, M., Turkyilmaz, A., et al. (2021). Household water and energy consumption changes during COVID-19 pandemic lockdowns: cases of the kazakhstani cities of almaty, shymkent, and atyrau. Buildings 11 (12), 663. doi:10.3390/buildings11120663
Tomas, M., Lopez, L., and Monsalve, F. (2020). Carbon footprint, municipality size and rurality in Spain: inequality and carbon taxation. J. Clean. Prod. 266, 121798. doi:10.1016/j.jclepro.2020.121798
Trotta, G., Gram-Hanssen, K., and Jorgensen, P. (2020). Heterogeneity of electricity consumption patterns in vulnerable households. Energies 13 (18), 4713. doi:10.3390/en13184713
UNEP (2022). Renewable energy – powering a safer future. https://www.unep.org/.
Urquizo, J., Calderon, C., and James, P. (2017). Metrics of urban morphology and their impact on energy consumption: a case study in the United Kingdom. Energy Res. Soc. Sci. 32 (SI), 193–206. doi:10.1016/j.erss.2017.03.011
Utama, A., and Gheewala, S. H. (2009). Indonesian residential high rise buildings: a life cycle energy assessment. Energy Build. 41 (11), 1263–1268. doi:10.1016/j.enbuild.2009.07.025
Vega-Azamar, R., Romero-Lopez, R., Oropeza-Garcia, N., Glaus, M., Hausler, R., and Silva-Poot, H. (2017). Emergy evaluation of dwelling operation in five housing units of Montreal island, Canada. Sustainability 9 (4), 663. doi:10.3390/su9040663
Verma, P., and Raghubanshi, A. S. (2018). Urban sustainability indicators: challenges and opportunities. Ecol. Indic. 93, 282–291. doi:10.1016/j.ecolind.2018.05.007
Vogt Gwerder, Y., Marques, P., Dias, L. C., and Freire, F. (2019). Life beyond the grid: a Life-Cycle Sustainability Assessment of household energy needs. Appl. Energy 255, 113881. doi:10.1016/j.apenergy.2019.113881
Vuelvas, J., and Ruiz, F. (2017). Rational consumer decisions in a peak time rebate program. Electr. Power Syst. Res. 143, 533–543. doi:10.1016/j.epsr.2016.11.001
Walzberg, J., Dandres, T., Merveille, N., Cheriet, M., and Samson, R. (2019). Accounting for fluctuating demand in the life cycle assessments of residential electricity consumption and demand-side management strategies. J. Clean. Prod. 240, 118251. doi:10.1016/j.jclepro.2019.118251
Wang, F., Xu, B., Si, Y., Shang, Y., Zhang, W., Cai, B., et al. (2023). Components and drivers of household water footprint inequality in China. Sustain. Prod. Consum. 43, 1–14. doi:10.1016/j.spc.2023.10.009
Wang, Q., Liu, P., Yuan, X., Cheng, X., Ma, R., Mu, R., et al. (2015). Structural evolution of household energy consumption: a China study. Sustainability 7 (4), 3919–3932. doi:10.3390/su7043919
Wang, T., Zhao, Q., Gao, W., and He, X. (2023). Subdividing end-use energy consumption based on household characteristics and climate conditions: insights from urban China. Front. Energy Res. 11, 1267975. doi:10.3389/fenrg.2023.1267975
Wang, W., and Zhang, M. (2015). Direct and indirect energy consumption of rural households in China. Nat. Hazards 79 (3), 1693–1705. doi:10.1007/s11069-015-1921-5
Wang, X., Ding, C., Zhou, M., Cai, W., Ma, X., and Yuan, J. (2023). Assessment of space heating consumption efficiency based on a household survey in the hot summer and cold winter climate zone in China. Energy 274, 127381. doi:10.1016/j.energy.2023.127381
Wang, Y., Fu, Y., Lin, H., Sun, Q., Scartezzini, J., and Wennersten, R. (2022). Uncertainty modeling of household appliance loads for smart energy management. Energy Rep. 8, 232–237. doi:10.1016/j.egyr.2021.11.097
Wang, Y., Yang, G., Dong, Y., Cheng, Y., and Shang, P. (2018). The scale, structure and influencing factors of total carbon emissions from households in 30 provinces of China-based on the extended STIRPAT model. ENERGIES 11 (5), 1125. doi:10.3390/en11051125
Wang, Z., and Yang, L. (2014). Indirect carbon emissions in household consumption: evidence from the urban and rural area in China. J. Clean. Prod. 78, 94–103. doi:10.1016/j.jclepro.2014.04.041
Wen, F., Ye, Z., Yang, H., and Li, K. (2018). Exploring the rebound effect from the perspective of household: an analysis of China’s provincial level. Energy Econ. 75, 345–356. doi:10.1016/j.eneco.2018.08.018
Wiedmann, T. (2009). A review of recent multi-region input–output models used for consumption-based emission and resource accounting. Ecol. Econ. 69 (2), 211–222. doi:10.1016/j.ecolecon.2009.08.026
Wu, J., Hou, B., Ke, R., Du, Y., Wang, C., Li, X., et al. (2017). Residential fuel choice in rural areas: field research of two counties of north China. Sustainability 9 (4), 609. doi:10.3390/su9040609
Xu, L., Geng, Y., Wu, D., Zhang, C., and Xiao, S. (2021). Carbon footprint of residents’ housing consumption and its driving forces in China. Energies 14 (13), 3890. doi:10.3390/en14133890
Xu, L., Liu, J., Pei, J., and Han, X. (2013). Building energy saving potential in Hot Summer and Cold Winter (HSCW) Zone, China—influence of building energy efficiency standards and implications. Energy Policy 57, 253–262. doi:10.1016/j.enpol.2013.01.048
Xu, Q., Hwang, B.-G., and Lu, Y. (2021). Exploring the influencing paths of behavior-driven household energy-saving intervention – household energy saving option (HESO). Sustain. Cities Soc. 71, 102951. doi:10.1016/j.scs.2021.102951
Xu, T., Gao, W., Qian, F., and Li, Y. (2022). The implementation limitation of variable renewable energies and its impacts on the public power grid. Energy 239, 121992. doi:10.1016/j.energy.2021.121992
Xu, X., Gou, X., Zhang, W., Zhao, Y., and Xu, Z. (2023). A bibliometric analysis of carbon neutrality: research hotspots and future directions. Heliyon 9 (8), e18763. doi:10.1016/j.heliyon.2023.e18763
Xu, X., Han, L., and Lv, X. (2016). Household carbon inequality in urban China, its sources and determinants. Ecol. Econ. 128, 77–86. doi:10.1016/j.ecolecon.2016.04.015
Yang, Y., Jia, J., and Chen, C. (2020a). Residential energy-related CO2 emissions in China’s less developed regions: a case study of jiangxi. Sustainability 12 (5), 2000. doi:10.3390/su12052000
Yang, Y., Jia, J., Devlin, A., Zhou, Y., Xie, D., and Ju, M. (2020b). Decoupling and decomposition analysis of residential energy consumption from economic growth during 2000-2017: a comparative study of urban and rural guangdong, China. ENERGIES 13 (17), 4461. doi:10.3390/en13174461
Yu, Z., Haghighat, F., Fung, B., Morofsky, E., and Yoshino, H. (2011). A methodology for identifying and improving occupant behavior in residential buildings. Energy 36 (11), 6596–6608. doi:10.1016/j.energy.2011.09.002
Zahabi, S., Miranda-Moreno, L., Patterson, Z., and Barla, P. (2012). Evaluating the effects of land use and strategies for parking and transit supply on mode choice of downtown commuters. J. Transp. Land Use 5 (2), 103–119. doi:10.5198/jtlu.v5i2.260
Zeng, Y., Yang, H., Wang, Z., and Li, L. (2021). Impacts of family household dynamics on residential energy demands in Hebei Province of China. GENUS 77 (1), 35. doi:10.1186/s41118-021-00148-0
Zhang, G., Zhang, C., and Nie, H. (2021). An overview of China’s energy labeling policy portfolio: China’s contribution to addressing the global goal of sustainable development. Sage Open 11 (1), 215824402098885. doi:10.1177/2158244020988858
Zhang, J., Ma, L., and Li, J. (2021). Why low-carbon publicity effect limits? The role of heterogeneous intention in reducing household energy consumption. Energies 14 (22), 7634. doi:10.3390/en14227634
Zhang, J., Zhang, L., Qin, Y., Wang, X., and Zheng, Z. (2019). Impact of residential self-selection on low-carbon behavior: evidence from zhengzhou, China. Sustainability 11 (23), 6871. doi:10.3390/su11236871
Zhang, L., and Wen, X. (2021). Nonlinear effect analysis of electricity price on household electricity consumption. Math. Problems Eng. 2021, 1–13. doi:10.1155/2021/8503158
Zhang, M., and Bai, C. (2018). Exploring the influencing factors and decoupling state of residential energy consumption in Shandong. J. Of Clean. Prod. 194, 253–262. doi:10.1016/j.jclepro.2018.05.122
Zhang, X., Luo, L., and Skitmore, M. (2015). Household carbon emission research: an analytical review of measurement, influencing factors and mitigation prospects. J. Of Clean. Prod. 103, 873–883. doi:10.1016/j.jclepro.2015.04.024
Zhang, Y., and Lei, Y. (2017). Research on the carbon emissions of Beijing residents based on the input-output model. Pol. J. Of Environ. Stud. 26 (5), 2397–2406. doi:10.15244/pjoes/69441
Zhao, Q., Gao, W., Su, Y., and Wang, T. (2023a). Carbon emissions trajectory and driving force from the construction industry with a city-scale: a case study of Hangzhou, China. Sustain. Cities Soc. 88, 104283. doi:10.1016/j.scs.2022.104283
Zhao, Q., Gao, W., Su, Y., Wang, T., and Wang, J. (2023b). How can C&D waste recycling do a carbon emission contribution for construction industry in Japan city? Energy Build. 298, 113538. doi:10.1016/j.enbuild.2023.113538
Zhao, X., Gao, W., Qian, F., and Ge, J. (2021). Electricity cost comparison of dynamic pricing model based on load forecasting in home energy management system. Energy 229, 120538. doi:10.1016/j.energy.2021.120538
Zheng, J., Dang, Y., and Assad, U. (2024). Household energy consumption, energy efficiency, and household income–Evidence from China. Appl. Energy 353, 122074. doi:10.1016/j.apenergy.2023.122074
Keywords: bibliometric analysis, household energy consumption, environmental assessment, mitigation measures, household emissions reduction
Citation: Wang T, Zhao Q, Gao W and He X (2024) Research on energy consumption in household sector: a comprehensive review based on bibliometric analysis. Front. Energy Res. 11:1209290. doi: 10.3389/fenrg.2023.1209290
Received: 20 April 2023; Accepted: 13 December 2023;
Published: 10 January 2024.
Edited by:
João Pedro Gouveia, New University of Lisbon, PortugalReviewed by:
Usep Surahman, Universitas Pendidikan Indonesia, IndonesiaJanez Dolšak, University of Ljubljana, Slovenia
Copyright © 2024 Wang, Zhao, Gao and He. This is an open-access article distributed under the terms of the Creative Commons Attribution License (CC BY). The use, distribution or reproduction in other forums is permitted, provided the original author(s) and the copyright owner(s) are credited and that the original publication in this journal is cited, in accordance with accepted academic practice. No use, distribution or reproduction is permitted which does not comply with these terms.
*Correspondence: Qinfeng Zhao, emhhb3FpbmZlbmcwMTIyQDE2My5jb20=