- 1Policy Studies Institute, Addis Ababa, Ethiopia
- 2University College London, London, United Kingdom
Wide uses of energy-efficient technologies have the potential to save a significant amount of electricity, which may allow deferral of construction of new power plants. However, in low-income countries, there is low use of energy-efficient technologies. This paper studies the behavioral and socio-economic determinants of urban households’ investment in energy efficiency improvements in Ethiopia. It considers multiple technologies and uses a multivariate probit model to take into account the sequential or simultaneous adoption of energy-efficient technologies. Using a random sample of 1,400 urban households from nine regions in Ethiopia, the study finds that most households (86%) adopt energy-efficient technologies and other energy conservation activities mainly to reduce energy expenditure. Interestingly, at least 12% of the respondents adopt these activities out of concern for the environment and future generations, which is encouraging. The multivariate probit regression result shows that households’ educational status, income or occupational status, access to credit, and age are significant determinants of households’ investment in energy-efficient technologies. The results illustrate there is a need for policies that incentivize or promote firms to sell energy-efficient products, for example, using installment (credit) and also a policy on the language of product specifications–using local language.
1 Introduction
Energy efficiency measures the amount of useful energy (services) that we receive from the use of final energy such as electricity, gas, biomass, etc. Switching to more energy efficient technologies, which consume less energy to deliver the same energy services, offers multiple benefits, such as reduced household energy expenditure and improved productivity, thus better livelihood, and wellbeing of households as the money saved on energy expenditure can be used for other services. Use of energy-efficient technologies in the residential sector has the potential to reduce energy consumption and this makes clean energy more accessible and affordable to many households and enterprises as the same generation capacity can be used to serve more households and enterprises due to lower demand. Further, diffusion of energy-efficient technologies has the added advantage of reducing greenhouse gas emissions.
Globally, the emissions of greenhouse gas increased by 31% during 2000–2010 and 10% during 2010–2019 (IEA, 2021). Emissions shrank by more than 5% between 2019 and 2020, as the COVID-19 pandemic cut energy demand. In 2021, emissions rebounded past pre-pandemic levels, growing more than 6% in tandem with economic stimulus and the roll-out of vaccines (IEA, 2023). According to EIA (2019), recent global energy consumption is driven by increasing use of electricity in the residential and commercial building sectors and these two sectors account for around 20% of total global energy consumption (EIA, 2019). This highlights the important role that energy-efficient technologies can play in emissions reduction. In Ethiopia, the household sector accounted for 33% of total electricity consumption (MoWIE, 2015) with most urban households using multiple electrical appliances. Eludoyin et al. (2022) estimate that increased sale of efficient technologies under the 2030 MEPS (Minimum Energy Performance Standard) has the potential for ∼30% in cumulative savings in residential electricity demand between 2017 and 2065. Therefore, energy efficiency improvements will be important for Ethiopia since it curbs demand growth, thereby reducing additional power capacity needs and facilitating cheaper and faster energy access to populations. Although numerous energy efficient technologies exist, there remain important barriers to the adoption of such technologies. A greater understanding of the determinants of households’ use of energy efficient technologies in Ethiopia is therefore required.
There are a growing number of studies in developing countries on households use of energy-efficient technologies (e.g., Alem et al., 2014; Hassen, 2015; Emodi et al., 2022). Previous studies identified income, education, age, gender, and other socio-economic characteristics of household members as important determinants of households’ use of energy-efficient technologies (e.g., Amacher et al., 1992; Adrianzén, 2013; Alem et al., 2014; Jeuland et al., 2014; Hassen, 2015). In developed countries, studies concentrate on the effect of dwellings, attitudes towards environmental problems or energy saving measures, households’ knowledge about their energy consumption, and the cost and performance of energy conservation measures and renewable technologies (Sardianou and Genoudi, 2013; Ameli and Brandt, 2015). To date, studies in developing countries have focused on socio-economic characteristics, but less on behavioral factors.
This paper focuses on urban households’ behavioral and socio-economic determinants of investment in energy-efficient technologies in Ethiopia. The efficient technologies include biomass stoves for cooking and electric devices for all household services. Although there are a growing number of studies on energy-efficient technologies in Ethiopia and other countries in sub-Saharan African (SSA), this research adds value to the literature in this field in two ways. First, unlike many studies in SSA, this study focuses on households’ use of multiple energy technologies that may use different fuels. Previous studies in SSA focus on only one technology, for example, the adoption of an improved biomass stove or a liquified petroleum gas (LPG) stove (e.g., Beyene and Koch, 2013; Bensch&Peters, 2011; 2012; Bensch et al., 2015). A household will use multiple appliances for different services and the decision to buy an efficient energy appliance may depend on other technologies used within a household. A household may purchase multiple related energy-efficient technologies or may make a sequential decision in the investment of technologies. Second, this study includes the effect of households’ motives for engaging in energy efficiency behaviors and energy-efficient technologies. Households may adopt energy-efficient behaviors and technologies for purely economic reasons, i.e., to reduce expenditure on energy, or out of concern for the environment, or both. To the best of our knowledge, studies in SSA countries have yet to take the effect of these factors into account.
This paper addresses the following research question: what motivates and influences urban households in Ethiopia to invest in energy efficiency and energy efficient technologies? To answer this question, 1,400 households were randomly selected from an urban area—Addis Ababa.
The rest of the paper is structured as follows: Section 2 provides a review of literature on determinants of energy efficiency, Section 3 discusses the conceptual framework and empirical strategy of the study, Section 4 presents the data used for the study, and Section 5 and Section 6 provides the descriptive and econometrics analysis of the study and the last section concludes.
2 Literature review
Improving access to affordable and reliable forms of modern energy is an essential part of economic development, especially for developing countries (Modi et al., 2005; Barnes et al., 2011; Ekouevi and Tuntivate, 2012). However, electricity service provision is typically limited in SSA countries. Addressing this challenge will require a massive expansion of electricity access through both on and off-grid solutions.
Globally, however, the residential sector accounts for one-fifth of energy consumption through, for example, demand for heating, cooling, and lighting services. It is therefore not surprising that energy efficiency in the residential market has gained importance in recent years. International institutions (e.g., OECD, 2003; European Commission, 2011; IEA, 2013b) also recommend that energy efficiency is the best mechanism to reduce energy demand and at the same time to contribute to low-carbon development. Hence, taking energy-efficiency enhancing measures is vital both to households and to electricity service providers.
However, there might be unintended results arising from the adoption of energy-efficient technologies. For instance, an increase in the availability of energy-efficient technologies might bring an increase in household energy consumption (Kowsari and Zerriffi, 2011; Morton and Griffiths, 2012), which is known as the rebound effect. Hence, increasing the use of energy-efficient appliances may directly increase energy use (Steg, 2008; Oikonomou et al., 2009) which will inflate energy demand and expenditure. Household adoption of energy-efficient technologies may play a significant role in reducing energy consumption and maintaining economic development. Moreover, an increase in energy-efficient household electric appliances will also play a significant contribution to alleviate environmental impacts arising from residential electricity demand (Baldini et al., 2018). Several studies have examined the adoption of energy efficient technologies and behaviors in different parts of the world, and these are described in more detail below.
i. Household investment in energy efficiency and efficient technologies adoption
Over the past few decades, energy efficiency and managing energy demand have become a global priority. However, the potential cost-savings of energy efficient technologies alone have not been sufficient to drive their adoption or to change behavior. Rather, technologies must be aligned with the interests of users and user regulators, and also with energy efficiency standards and incentives. Increasing energy efficiency also requires efforts to raise awareness across industrial sectors and household consumers (World Energy Council, 2016).
Economic theories suggest that households’ expenditure on energy conservation activities and the use of renewable energy sources will be determined by: the ability of the households to purchase energy conservation inputs; their incentives to invest in energy-conserving appliances; the climatic conditions of where they live; and the age of the household head (Long, 1993). The other important factor to be considered is consumers risk preferences, which play a significant role in the adoption of energy-efficient technologies (Qiu et al., 2014).
Demographic and socio-economic factors, such as income, type of dwelling, and number of habitats living in a household, determine the energy use of a given household (Bedir et al., 2013; Jones and Lomas, 2016; Girod et al., 2017). Hence, the adoption of energy-efficient appliances and their use in one way or another can be affected by those specified demographic and socio-economic characteristics of a given household. Various types of energy-saving appliances are in use across different parts of the world. Despite the potential for energy saving and economic benefits from these technologies, like compact fluorescent light bulbs, their adoption rate by the residential sector is limited.
A review of the literature by Urban and Ščasný (2012) reveals several variables which determine household energy adoption behavior. These include socio-demographic characteristics (age, gender, education, household size, and presence of children in the household), economic variables (household income, ownership of the dwelling, home size), and also structural variables (energy metering, prices of energy, available energy sources). Apart from lighting, household cooking consumes more energy than any other end-use service in low-income developing countries (IEA, 2006; Daioglou et al., 2012). Based on bottom-up model projections in selected developing countries, cooking activities consume most of the household’s energy demand which is dominated by traditional fuels (Daioglou et al., 2012).
To help the poor segment of the society and alleviate environmental degradation, improved-cooking technologies were introduced in most parts of Ethiopia. In evaluating the adoption rate of improved-cooking technologies in Ethiopia, Beyene and Koch, 2013 examine reasons for the adoption of cooking technologies and the speed of adoption using “duration analysis” for Mirte and Lakech cookstoves - two new cooking technologies in urban Ethiopia. The result of the duration analysis shows that adoption rates steadily increased over time. Product price, household income, and household wealth were the most important determinants of adoption. Conversely, the availability of substitute technologies tended to hinder the adoption of the specified cookstove technologies. The study also identified large differences in the adoption of technologies among different regions of the country. In Addis Ababa, Takama et al., 2015 also found that marginal willingness to pay for high-quality fuels and stoves tended to increase with wealth increases.
Most of the research undertaken in Ethiopia has been focused on households’ fuel choices and their determinants in different parts of the country. There is a lack of evidence on energy efficiency and adoption of energy-efficient appliances and technologies. Among the studies that deals with fuel choices, Abebaw (2007) identifies a non-linear relationship between per capita income and per capita fuelwood consumption in Jimma town, Ethiopia. Per capita fuelwood consumption is inversely related to family size and education of the household head. On the other hand, households that have higher incomes are likely to diversify their fuel choices rather than substituting one particular fuel type with another (Mekonnen, 2009).
The underlying socio-economic drivers of residential fuel choices are also assessed by Guta (2012) in Ethiopia. The results of the study show that households’ fuel choice is mainly constrained by limited access to commercial fuels and efficient cookstoves. In addition, supply dependency, affordability, and consumer preferences play a significant role in determining fuel choices. The study also found that households are expected to develop inter-fuel substitution and switching behavior conditional on access to modern energy technologies. Hence, it explains the availability of energy-efficient technologies in Ethiopia is rudimentary.
Technology adoption decisions by households vary depending on the type of technologies. For instance, Guta (2018) found that wealthy households are more likely to adopt solar energy technology as compared to poor households in Woliso town, Oromia State, Ethiopia. The study also identified that male-headed households were less likely to adopt solar energy technology compared to female-headed counterparts. Other important factors which determined the adoption of solar technology included household wealth, education, and awareness.
Fuelwood choices and their determinants in African households follow similar patterns to those of Ethiopia’s. A study by Jumbe & Angelsen (2011) determines households’ choice of fuelwood sources and the environmental consequences of fuelwood collection choices in Malawi. The result of their study shows that the size and species composition and the distance to the source are the most important determinants of fuelwood choice. Factors that determine a household’s energy-efficient use includes income, education, age of the household and age of the house, past investment, and perceived energy cost, which influence homeowners’ preference for a particular type of energy efficiency measures (Nair et al., 2010). An interesting finding from Emodi et al. (2022) is that aside from income–education, household size, and internet access are essential drivers of urban household fuel choices in Africa.
ii. Household behavioral motivations for engaging in energy efficiency and energy efficient technologies
Households’ behavioral motivations in the decision to use energy-efficient technologies and appliances is an important pillar to address energy efficiency and efficient use of energy. Moreover, in assessing the energy consumption of households to develop energy-saving strategies and measures, individual-level behavioral assessment and approaches are important (Vassileva et al., 2012). A review by Pothitou et al. (2016) found that individual energy perception gaps are affected by psychological, habitual, structural, and cultural variables both at a societal and individual level of analysis. These kinds of measures can help to manage effective and efficient policy implementation concerning energy efficiency and efficient use of energy resources. It is also revealed that social influence plays a vital role as a driver of behavior to adopt new technologies (Venkatesh et al., 2003).
In determining the main factors which affect consumers’ choice and their behavioral attitudes in OECD countries, households’ propensity to invest in clean energy technologies mainly depends on home ownership, income, and the social context of the households’ energy practices (Ameli & Brandt, 2015). Homeowners and high-income earning households are more likely to invest in clean technologies than renters. Similarly, social context - such as membership in environmental and non-governmental organizations - helps the adoption of clean technologies. In Denmark, socioeconomic, demographic, and behavioral variables were found to determine household choices in energy-efficient appliances (Baldini et al., 2018). The study further investigates that income is a weak predictor of adoption of energy efficient appliances. Similar findings from Emodi et al. (2022) for Africa that no significant relationship between the price and expenditure on different fuels in urban households. Even though the Danish population is wealthy and well-educated, information campaigns have been largely ineffective in driving high-efficiency investments.
Even though there are economic benefits of adopting energy-efficient technologies and changing behaviors, diffusion of energy-efficient technologies remains limited. Factors, such as the perceived risk of the return on energy efficiency investments and attitudes towards energy efficiency can cause slow diffusion of energy-saving appliances and technologies (Hirst and Brown, 1990).
Weber (1997) summarizes institutional, market-related, organizational, and behavioral barriers that can explain the slow diffusion of energy efficiency. This shows how relevant behavioral factors are in technology diffusion and the efficient use of electricity. Reddy and Painuly (2004) identified factors that can be considered as barriers to the diffusion of efficient technologies, which include: information asymmetry, lack of financial support, perceived technological risks, institutional and regulatory barriers, market barriers/market failures and behavioral factors. Adkins et al. (2010) suggest that market- and community-based models of LED lighting technology dissemination have the potential to increases technology diffusion in developing countries. Community-based activities have a positive impact on the energy-saving behavior of five Asian cities (Hori et al., 2013). The study also found that global warming consciousness, environmental behavior, and social interactions significantly affect energy-saving behaviors.
Pricing and payment options also influence individual’s behavior and increase adoption of efficient appliances. Beyene et al. (2022) examine the impacts of pre-paid metering on electricity consumption, ownership of appliances, level of satisfaction, and cooking behavior in Addis Ababa. The results indicate that pre-paid customers have significantly lower electricity consumption compared to those with traditional meters and it had positive and significant impacts significant impact on ownership of energy-efficient lights. On the other hand, Hassen et al. (2022) examined the price elasticity of demand for electricity in urban Ethiopia and found that demand was highly inelastic due to a lower share of income being spent on energy.
The other factor which affects energy-saving behavior is cultural behavior. A study by Quaglione et al. (2017) in Italy emphasized the significance of cultural capital, specifically, individuals’ participation with pro-environmental behavior, which was a relevant additional driver of electricity-saving behaviors. The study found that individuals who participate in some cultural activities show a higher degree of adoption of electricity-saving behavior in their homes. Likewise, individuals’ environmental concerns play a significant role in shaping domestic energy-saving behavior (Abrahamse and Steg, 2009; Martinsson et al., 2011; Han et al., 2013; Gram-Hanssen, 2014; Zhou and Yang, 2016). Lifestyle and behavioral aspects can also be a barrier to energy use reduction activities in the residential sector. Quaglione et al. (2017), therefore recommended that policymakers should focus on individual consumers’ responsibility in climate change policies and their action with regard to energy efficiency.
Our review of the literature on behavioral motivations found that existing empirical evidence is concentrated in developed economies and some SSA countries, but not Ethiopia. To the best of our knowledge, no study in Ethiopia has examined the determinants of households’ decision to adopt combinations of energy-efficient technologies nor the effect of households’ behavioral motives. This research therefore aims to fill this gap by providing insights into the factors which determine households’ decisions to adopt energy-efficient appliances.
3 Conceptual framework and empirical strategy
Households’ adoption decisions for a single energy efficient technology or multiple energy efficient technologies can be modeled using a random utility framework. Let
Household decisions to transit from the state of non-adoption to the state of adoption of energy efficient technology in isolation or as a package j on household h if
Where
One may try to identify the effect of observable socio-economic characteristics on energy efficient technologies using a single equation method such as the simple probit method. However, this assumes that the error terms are independently and identically distributed across energy efficient technologies. This assumes no interdependency among energy-efficient technologies. However, a household may adopt two or more technologies sequentially or simultaneously. This means that household decisions for various energy-efficient technologies are interrelated. A single equation estimation approach could cause bias and inefficiency in the parameters if the interdependence is observed and/or if unobserved heterogeneity is correlated among these energy-efficient technologies in the adoption decisions (Greene, 2008). A Multivariate Probit Method (MVP) (non-linear seemingly unrelated simultaneous equation) that allows correlation among the unobserved disturbances is best suited to the adoption of interrelated energy-efficient technologies and appliances. Following Teklewold et al. (2013); Ndiritu et al. (2014), the interdependence of energy-efficient technologies in both adoption decisions can be tested by looking at the sign and significance of the off-diagonal elements of the variance-covariance matrix of a Multivariate Probit Model (MVP).
4 Data
The study is based on phone survey data collected from nine regions of Ethiopia1 during July-September 2020. The sample households were from urban areas of these nine regions. In selecting the sample households, we followed a sampling frame applied by the World Bank’s Multi-Tier Frame Work (MTF), a survey that was conducted in 2016/2017. The MTF survey covers both rural and urban areas of the country, whereas this study only covers urban areas due to limited mobile phone availability in rural areas, which would have led to a sample selection problem. In urban areas, mobile phone access is 100% and all urban MTF sample households have a mobile phone number. The respondents were incentivized by transferring a 100 birr (US $1.85) mobile card for the time they spent on the interview.
The MTF survey used a nationally representative sample of households, which were selected based on a two-stage cluster sampling approach. In the first stage, it selected primary sampling units, i.e., the Enumeration Areas (EA). It used the Ethiopian Central Statistics Agency (CSA) EA, which were used for the population and housing census conducted in 2007. A total of 337 EA were chosen using probability proportional to size sampling technique, i.e., proportional to the size of the population. In the second stage, 12 households from each EA were chosen using systemic random sampling method, except for Addis Ababa where 20 households were chosen systematically to include informal settlements. If the EA is under national grid electricity, nine electricity-connected households and three non-connected households2 were selected to make the total number of households for that EA = 12. A total of 4,317 households were used for the baseline MTF survey, and about 2,500 households were from urban areas.
In this study, due to resource limitations, we did not survey all MTF urban households. We randomly selected 1,500 households from the list of 2,500 MTF sample households. Due to COVID-19 and travel restrictions related to the pandemic, it was not possible to conduct the survey face-to-face. Hence, we used a phone survey method. On average the phone interview took about 45 min. For most of the households, the interview was non-stop (uninterrupted interview) while for others it required a second call. A second phone call was undertaken in mid-August/2020 for those households whose phone network did not work properly during the first survey period. About 100 of the 1,500 declined to participate in the second call, but this dropout is not systemic to the observed socio-economic characteristics of the households. Thus, this study is based on a sample of 1,400 urban households from all regions of the country.
5 Descriptive analysis
Table 1, Table 2, Table 3 show the socio-economic characteristics of the sample households. From Table 1, it can be seen that, on average, the sample households have either a high school or primary level of education. Table 2 shows the disaggregated level of education of the respondents. About 76% of the sample households have a high school or less than a high school level of education, which has an implication on their knowledge about energy efficient technologies. Most of the energy-efficient technologies are imported, which means that the labels detailing the energy-efficiency are likely to be in English. Households with primary or no formal education or some with high school will therefore face difficulties in understanding the labels.
Further, about 40% of the sampled households were either casual workers or unemployed (i.e., dependent on other family members for income and/or rent). This also has implications for the ability of participants to purchase energy efficient products. Casual workers in Ethiopia have subsistence wage income and will have difficulty to cover the upfront cost or accessing credit to buy the technologies. Unemployed household heads that depend on remittances or on the income of other family members may also face challenges in the decision to buy the items. They need approval from these other family members to purchase the item in cash or on credit. In line with this, Table 1 shows that only 30% of the households have access to credit.
From Table 1, about 43% of the household heads are women. Table 1 also shows that about 60% of the respondents are currently married and spending about 75% of their monthly income on food and non-food items.
Table 4 shows households’ energy expenditure. As can be seen from the table, households’ mean monthly energy expenditure is about 243.5 birr (1 USD = 36 birr), which constitutes about 4% of households’ income. This implies that households in Ethiopia spend only a smaller fraction of their income on energy. Of all energy sources, electricity expenditure is the highest, and accounts for 66% of total energy expenditure. Households also use biomass fuels in addition to electricity, which has a health implications from indoor air pollution and leads to greenhouse gas emissions.
Table 5 shows whether households adopted energy conservation activities, which included shifting baking and cooking time, adopting energy-efficient technologies, turning off light bulbs and other appliances when not in use, and reducing the number of light bulbs. Table 5 shows that the majority of respondents (61%) did one or more energy conservation activities. These activities have the advantage of reducing the load during peak hours.
Households may have different motivations to conserve energy. The motivations could be to reduce energy expense, to conserve natural resources and/or to protect future generations or to reduce greenhouse gas emissions. Respondents were asked about their main motivation to use energy conservation activities. About 86% of the respondents responded that their main motivation was to reduce expenses (Table 6). About 12% of the respondents were mainly motivated by concern about the climate and future generations, which is encouraging.
Table 7 shows the percentage of respondents using energy-efficient technologies for various end-use services. Almost all (92%) of the respondents used energy-efficient light bulbs. This is unexpected, because the Government of Ethiopia has banned the import and local production of incandescent light bulbs. Further, between 2009 and 2012 there was a free and subsidized distribution of CFL light bulbs by the Ethiopian Electric Utility. This may have created awareness and increased uptake and continued use of energy-efficient light bulbs.
About 34% of the respondents used energy-efficient electronic appliances, most likely purchased in recent years. Recently purchased electronic appliances are mostly Energy Star as less energy inefficient electronic appliances are no longer sold on the market. In Ethiopia, second-hand markets for electronic appliances are not common and hence households will only be able to buy new, more efficient appliances.
Sample households were asked to list the main barriers to the adoption of energy-efficient appliances. 65% of the respondents reported that they face difficulties in identifying efficient appliances because the description of product specifications is not in local languages. Other barriers included access to credit and availability of energy-efficient appliances, which were reported by 30% and 5% of respondents respectively. In most European and Latin American countries, imported products have product descriptions in the national language of importing country. However, in Ethiopia almost all imported products have product descriptions in non-Ethiopian languages. Further, lack of access to installment-based credit is also one of the barriers to the low use of energy-efficient electronic appliances.
Intra-household decision-making power is one of the key variables in the decision to purchase energy-efficient appliances. For example, where because households mostly use biomass fuels and women are responsible for cooking and biomass collection, the men have less interest to spend money on energy-efficient biomass stoves as this does not affect his monthly expenditure. Thus, it will be in the interest of women to buy more efficient stoves. The more women have power in household decision making, the more likely it will be that energy-efficient stoves are purchased. Alem et al. (2020) found that rural wives with higher decision-making power are more likely to buy energy-efficient technologies. However, Addis Ababa case is different. This is mainly because most urban households depend on purchased energy sources and hence both men and women are motivated to buy energy-efficient appliances to reduce energy expenses. Consistent with our expectation, Table 8 shows that most urban households decide either together (66%) or the wife makes decisions about purchasing energy-efficient appliances (24%).
6 Econometrics results
Results from the maximum likelihood estimation of the MVP are presented in Tables 9, 10. The Wald chi-square (
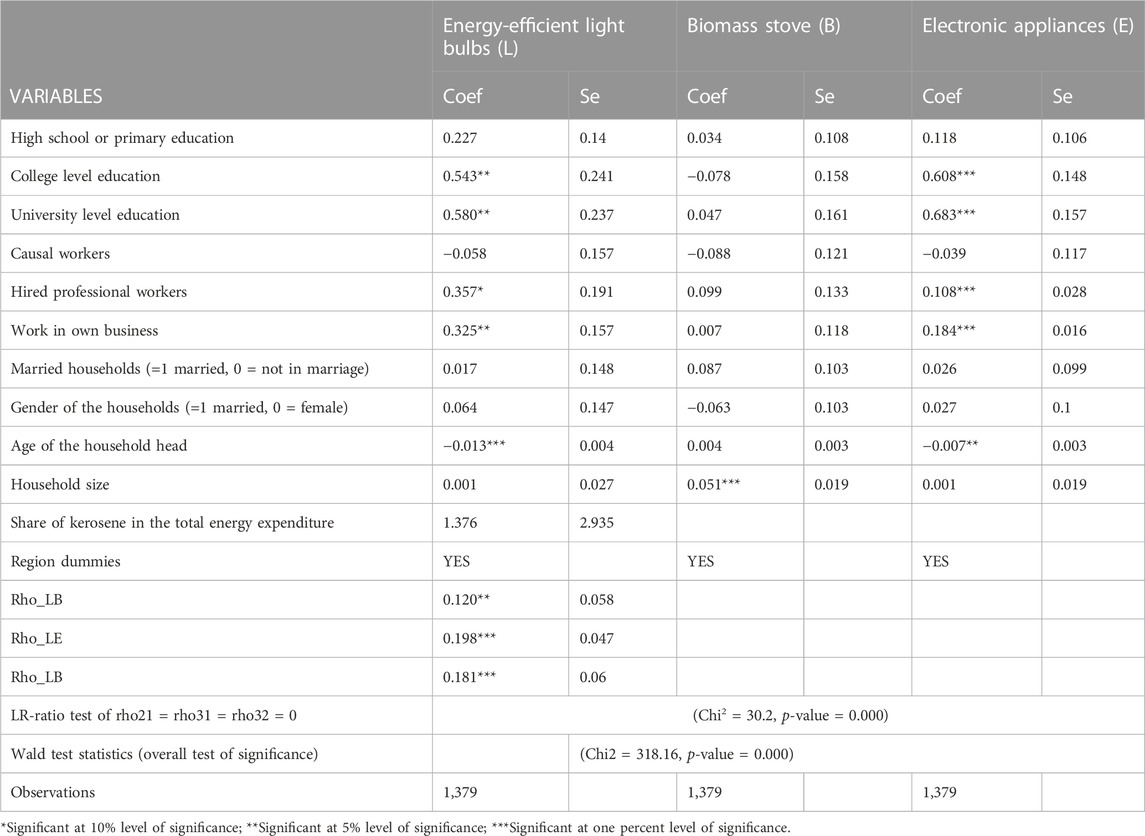
TABLE 9. Multivariate Probit Regression of the Determinants of Energy Efficient Technologies (household occupation is an indicator of income).
Both Tables 9, 10 and show the results of determinants of adoption of the three technologies. The two tables are different with respect to the income variable. In Table 9, we use households’ occupations as an indicator of income while in Table 10, we use monthly income. The reason we used households’ occupations as an indicator of income is that households usually underreport income during surveys. Expenditure is preferred because households are less sensitive to expenditure questions than income questions. Usually, expenditures from surveys are greater than income as income is expected to be understated but in our survey average income is greater than average expenditure. Hence, we use the occupation and income in our regressions. Table 10 also includes the effect of access to credit.
Concerning the determinants of socio-economic characteristics, Tables 9, 10 show that education is a significant determinant of households’ adoption of energy-efficient technologies. More specifically, in both Tables 9, 10, households with college and university level education are more likely to adopt energy-efficient light bulbs and electronic appliances. This is more likely to be related to knowledge about the benefits of energy-efficient technologies and it is also more likely to be related to their English language knowledge as the products imported to Ethiopia are likely to be labelled in English. Previous studies also documented the importance of education in the adoption of energy-efficient appliances (Alem et al., 2014; Hassen, 2015).
With respect to income, Table 9 shows that households who are professionals or who own their businesses are more likely to adopt energy-efficient light bulbs and electronic appliances. These households are more likely to be higher income households than the causal laborers or those that depend on remittances or property rent (labeled as unemployed in the data). Consistent with the results in Table 9, the income variable in Table 10 is positive and statistically significant showing that households with higher income are more likely to afford to buy energy-efficient bulbs and electronic appliances. A variable related to this is the credit variable. Access to credit is one of the barriers to the adoption of energy-efficient technologies. Unlike households in Europe and US, wholesalers in Ethiopia do not provide installment-based credit purchases. In line with this, the result in Table 10 shows that households who have access to credit are more likely to adopt energy-efficient technologies, specifically light bulbs and electronic appliances.
Both Tables 9, 10 also show that younger households are more likely to adopt energy-efficient technologies than older households. This can be related to the acceptance of modern technologies. Younger generations are more likely to accept modern technologies, which are labelled with an Energy Star, and modern lifestyles than older generations.
In this analysis, we do not find the gender variable to be statistically significant for the three energy-efficient technologies. As discussed in the descriptive statistics, women with higher decision-making power are more likely to adopt energy-efficient appliances as the burden of cooking and fuel collection rests on women. However, in Addis Ababa, where both men and women generate income for the household, the man has the incentive to adopt energy-efficient technologies to reduce monthly energy expenses. Thus, the insignificance of the gender variable may mean that both men and women have similar incentives to adopt energy-efficient technologies. The status of the marriage of the households is not found to be a significant determinant of the adoption of energy-efficient technologies.
As expected, results in Tables 9, 10 shows that household size is positive and significant for biomass energy efficient technology, but insignificant for lightbulbs and electronic appliances. Greater family size means a household must cook more food and hence has higher energy expenditure. Hence, households with greater family sizes have an incentive to adopt energy-efficient cooking technologies. However, for lighting and electronic appliances such as TV, the services are shared among household members. Hence, if a light is turned on, it gives the same service to one or more people in the same room. Hence, family size is not expected to be significant for lighting. Finally, most of the other socio-economic variables are not significant for energy-efficient biomass technologies, could be due to lower cost for biomass compared to that of modern energy.
7 Conclusions and policy implications
This paper studies the determinants of and motivations for household investment in energy-efficient technologies and other conservation activities. It used a random sample of 1,400 urban households in Addis Ababa, drawing on a phone survey which was conducted during July-September 2020. An MPV regression method was used to capture the simultaneity or sequential adoption of multiple energy-efficient technologies.
Most of the energy-efficient technologies in Ethiopia are imported and product descriptions, including their energy efficiency, is typically in English. Since most households (76%) have a low level of education this means the majority will not be able to read and understand the product description and efficiency details. We argue that product descriptions and labels must be provided in the local language. This implies that the government needs to set a new law, which mandates that product specifications are provided in the local language.
While household expenditure on energy constitutes about 4% of income, which is relatively low, capital cost remains a barrier. Only 30% of households have access to credit for the purchase of energy-efficient products. Therefore, access to credit is important in the use of energy-efficient technologies.
Despite these barriers, the majority of respondents (61%) adopted one or more energy efficiency and conservation activity, including shifting baking and cooking times, adopting energy-efficient technologies, turning off lightbulbs and other appliances when not in use, and reducing the number of light bulbs. About 86% of households used energy efficiency and conservation activities to reduce energy expenses. It is encouraging to see that about 12% of the sample were motivated by concern for the climate and future generations.
Significant determinants of households’ investment in energy-efficient technologies are educational status, income or occupational status, access to credit, and age. Gender is not a significant determinant of the adoption of energy-efficient technologies, as both men and women in urban areas share similar incentives to reduce energy expenditure.
Marginal effects are a useful way to describe the average effect of changes in explanatory variables on the change in the probability of outcomes in probit method. However, when analysing the data, we did not calculate the marginal effect which is a shortcoming in this study.
Data availability statement
The raw data supporting the conclusion of this article will be made available by the authors, without undue reservation.
Ethics statement
The studies involving human participants were reviewed and approved by Policy Studies Institute, Ethiopia. The participants provided their written informed consent to participate in this study.
Author contributions
SH: Developed the survey questionnaire, carried out the analysis and drafted the manuscript. GA: Project lead, developed overall research framework, contributed to the methodology and reviewed the manuscript. RS: Contributed to the development of survey questionnaire, conducted the survey and processed the data.
Funding
Foreign Commonwealth and Development Office—UKAID supported this work under the project “Energy system development pathways for Ethiopia (PATHWAYS)”. This is part of the Applied Research Program on Energy for Economic Growth (EEG), led by Oxford Policy Management. Grant reference no. A0534.
Conflict of interest
The authors declare that the research was conducted in the absence of any commercial or financial relationships that could be construed as a potential conflict of interest.
Publisher’s note
All claims expressed in this article are solely those of the authors and do not necessarily represent those of their affiliated organizations, or those of the publisher, the editors and the reviewers. Any product that may be evaluated in this article, or claim that may be made by its manufacturer, is not guaranteed or endorsed by the publisher.
Author disclaimer
The views expressed in this paper do not necessarily reflect the UK government’s official policies.
References
Abebaw, D. (2007)., Household determinants of fuelwood choice in urban Ethiopia: A case study of iimma townJ. Dev. Areas. 41. Published by : College of Business, Tennessee. The Journal of Developing Areas, 117–126. doi:10.1353/jda.2008.0009 https://www.jstor.org/stable/40376161
Abrahamse, W., and Steg, L. (2009). How do socio-demographic and psychological factors relate to households' direct and indirect energy use and savings? J. Econ. Psychol. 3m bxd bnxessasa0 30, 711–720. doi:10.1016/j.joep.2009.05.006
Adkins, E., Eapen, S., Kaluwile, F., Nair, G., and Modi, V. (2022). Off-grid energy services for the poor: Introducing LED lighting in the Millennium Villages Project in Malawi. Energy Policy 38, 1087–1097. doi:10.1016/j.enpol.2009.10.061
Adrianzén, (2013). Improved cooking stoves and firewood consumption: Quasi-experimental evidence from the Northern Peruvian Andes. Ecol. Econ. 89, 135–143. doi:10.1016/j.ecolecon.2013.02.010
Alam, S. S., Nik Hashim, N. H., Rashid, M., Omar, N. A., Ahsan, N., and Ismail, M. D. (2014). Small-scale households renewable energy usage intention: Theoretical development and empirical settings. Renew. Energy 68, 255–263. doi:10.1016/j.renene.2014.02.010
Alem, Y., Hassen, S., and Köhlin, G. (2014). Adoption and disadoption of electric cookstoves in urban Ethiopia: Evidence from panel data. Resour. Energy Econ. 38, 110–124. doi:10.1016/j.reseneeco.2014.06.004
Amacher, G. S., Hyde, W. F., and Joshee, B. R. (1992). The adoption of consumption technologies under uncertainty: A case of improved stoves in Nepal. J. Econ. Dev. 17 (2), 93–105.
Ameli, N., and Brandt, N. (2015). Determinants of households’ investment in energy efficiency and renewables: Evidence from the OECD survey on household environmental behaviour and attitudes. Environ. Res. Lett. 10 (4), 044015. doi:10.1088/1748-9326/10/4/044015
Baldini, M., Trivella, A., and Wente, J. W. (2018). The impact of socioeconomic and behavioural factors for purchasing energy efficient household appliances: A case study for Denmark. Energy Policy 120, 503–513. doi:10.1016/j.enpol.2018.05.048
Barnes, D. F., Khandker, S. R., and Samad, H. A. (2011). Energy poverty in rural Bangladesh. Energy Policy 39 (2), 894–904. doi:10.1016/j.enpol.2010.11.014
Bedir, M., Hasselaar, E., and Itard, L. (2013). Determinants of electricity consumption in Dutch dwellings. Energy Build. 58 (1), 194–207. doi:10.1016/j.enbuild.2012.10.016
Bensch, G., and Peters, J. (2012). A recipe for success? Randomized free distribution of improved cooking stoves in Senegal. Ruhr Econ Pap 0325 Westfälischesinstitutfürwirtschaftsforsch. Ruhr-Universität Boch Univ Dortm Univ Duisbg. 3.
Bensch &Peters, G. J. Combating deforestation? - impacts of improved stove dissemination on charcoal consumption in urban Senegal. Proceedings of the German Development Economics ConferenceBerlin. 2011. Research Committee Development Economics. VereinfürSocialpolitik.
BenschGrimm, G. M., and Peters, J. (2015). Why do households forego high returns from technology adoption? Evidence from improved cooking stoves in Burkina Faso. J. Econ. Behav. Organ. 116, 187–205. doi:10.1016/j.jebo.2015.04.023
Beyene, A. D., Jeuland, M., Sebsibie, S., Hassen, S., Mekonnen, A., Meles, T. H., et al. (2022). Pre-paid meters and household electricity use behaviors: Evidence from Addis Ababa, Ethiopia. Energy Policy 170, 113251. doi:10.1016/j.enpol.2022.113251
Beyene, A. D., and Koch, S. F. (2013). Clean fuel-saving technology adoption in urban Ethiopia. Energy Econ. 36, 605–613. doi:10.1016/j.eneco.2012.11.003
Daioglou, V., van Ruijven, B. J., and van Vuuren, D. P. (2012). Model projections for household energy use in developing countries. Energy 37 (1), 601–615. doi:10.1016/j.energy.2011.10.044
Ekouevi, K., and Tuntivate, V. (2012). World Bank Studies. Washington, D.C.: The World Bank.Household energy access for cooking and heating: Lessons learned and the way forward
Eludoyin, F. O., Anandarajah, G., Broad, O., Hassen, S., and Seifemichael, R. (2022). Modelling residential electricity demand in Ethiopia: A mixed methods approach. EEG Work Pap.
Emodi, N. V., Haruna, E. U., Abdu, N., Mortaya, S. D. A., Dioha, M., and Abraham-Ducka, M. C. (2022). Urban and rural household energy transition in Sub-Saharan Africa: Does spatial heterogeneity reveal the direction of the transition? Energy Policy 168, 113118. doi:10.1016/j.enpol.2022.113118
European Commission (2011). COM (2011) 109 final. Communication from the commission to the European parliament, the Council, the European economic and social committee and the committee of the regions. Brussels: Energy Efficiency Plan.
Girod, B., Stucki, T., and Woerter, M. (2017). How do policies for efficient energy use in the household sector induce energy-efficiency innovation? An evaluation of European countries. Energy Policy 103 (1), 223–237. doi:10.1016/j.enpol.2016.12.054
Gram-Hanssen, K. (2014). New needs for better understanding of household's energy consumption - behaviour, lifestyle or practices? Archit. Eng. Des. Manag. 10 (1–2), 91–107. doi:10.1080/17452007.2013.837251
Guta, D. D. (2012). Application of an almost ideal demand system (AIDS) to Ethiopian rural residential energy use: Panel data evidence. Energy Policy 50, 528–539. doi:10.1016/j.enpol.2012.07.055
Guta, D. D. (2018). Determinants of household adoption of solar energy technology in rural Ethiopia. J. Clean. Prod. 204, 193–204. doi:10.1016/j.jclepro.2018.09.016
Gwavuya, S. G., Abele, S., Barfuss, I., Zeller, M., and Müller, J. (2012). Household energy economics in rural Ethiopia: A cost-benefit analysis of biogas energy. Renew. Energy 48, 202–209. doi:10.1016/j.renene.2012.04.042
Han, Q., Nieuwenhijsen, I., de Vries, B., Blokhuis, E., and Schaefer, W. (2013). Intervention strategy to stimulate energy-saving behavior of local residents. Energy Policy 52, 706–715. doi:10.1016/j.enpol.2012.10.031
Hassen, S. (2015). PhD thesis chapter. Sweden: University of Gothenburg. Intra-household bargaining power and demand for clean and energy efficient technologies: Evidence from rural Ethiopia
Hasses, S., Beyene, A., Jeuland, M., Mekonnen, A., Meles, T., H., Sebsibie, S., et al. (2022). Effect of electricity price reform on households’ electricity consumption in urban Ethiopia. Util. Policy 79 (2022), 101445. doi:10.1016/j.jup.2022.101445
Hirst, E., and Brown, M. A. (1990). Closing the efficiency gap: Barriers to the efficient use of energy. Resour. Conserv. Recycl. 3 (4), 267–281. doi:10.1016/0921-3449(90)90023-w
Hori, S., Kondo, K., Nogata, D., and Ben, H. (2013). The determinants of household energy-saving behavior: Survey and comparison in five major Asian cities. Energy Policy 52, 354–362. doi:10.1016/j.enpol.2012.09.043
IEA (2023). CO2 emissions in 2022. https://iea.blob.core.windows.net/assets/3c8fa115-35c4-4474-b237-1b00424c8844/CO2Emissionsin2022.pdf.
IEA (2021). Greenhouse gas emissions from energy data explorer. https://www.iea.org/data-and-statistics/data-tools/greenhouse-gas-emissions-from-energy-data-explorer.
IEA (2013b). Transition to sustainable buildings. Strategies and opportunities to 2050. Paris: International Energy Agency.
Jeuland, M. A., Bhojvaid, V., Kar, A., Lewis, J. J., Patange, O., Pattanayak, S. K., et al. (2014). Preferences for improved cook stoves: Evidence from north villages. DUKE Environ. ENERGY Econ. Work. Pap. Ser. 52, 287–298. doi:10.1016/j.eneco.2015.11.010
Jones, R. V., and Lomas, K. J. (2016). Determinants of high electrical energy demand in UK homes: Appliance ownership and use. Energy Build. 117 (1), 71–82. doi:10.1016/j.enbuild.2016.02.020
Jumbe, C. B. L., and Angelsen, A. (2011). Modeling choice of fuelwood source among rural households in Malawi: A multinomial probit analysis. Energy Econ. 33 (5), 732–738. doi:10.1016/j.eneco.2010.12.011
Kowsari, R., and Zerriffi, H. (2011). Three dimensional energy profile:. Energy Policy 39 (12), 7505–7517. doi:10.1016/j.enpol.2011.06.030
Long, J. E. (1993). An econometric analysis of residential expenditures on energy conservation and renewable energy sources. Energy Econ. 15 (4), 232–238. doi:10.1016/0140-9883(93)90012-G
Martinsson, J., Lundqvist, L. J., and Sundström, A. (2011). Energy saving in Swedish households. The (relative) importance of environmental attitudes. Energy Policy 39, 5182–5191. doi:10.1016/j.enpol.2011.05.046
Mekonnen, A. (2009). IN ECONOMICS No 399 determinants of household fuel choice in major cities in Ethiopia alemu mekonnen gunnar köhlin.Determinants of household fuel choice in major cities in Ethiopia WORKING PAPERS
Modi, V., McDade, S., Lallement, D., and Saghir, L. (2005). Energy services for the millennium development goals. New York: UNDP and The World Bank.
Morton, G., and Griffiths, N. 2012. “Achieving energy efficiency through behavioural change – what does it take?” European environment agency (AEA) report.
Nair, G., Gustavsson, L., and Mahapatra, K. (2010). Factors influencing energy efficiency investments in existing Swedish residential buildings. Energy Policy 38 (6), 2956–2963. doi:10.1016/j.enpol.2010.01.033
Ndiritu, W. S., Kassie, M., and Shiferaw, B. (2014). Are there systematic gender di_erences in the adoption of sustainable agricultural intensi_cation practices? Evidence from Kenya. Food Policy 49 (1), 117–127. doi:10.1016/j.foodpol.2014.06.010
Oikonomou, V., Becchis, F., Steg, L., and Russolillo, D. (2009). Energy saving and energy efficiency concepts for policy making. Energy Policy 37 (11), 4787–4796. doi:10.1016/j.enpol.2009.06.035
Pothitou, M., Kolios, A. J., Varga, L., and Gu, S. (2016). A framework for targeting household energy savings through habitual behavioural change. Int. J. Sustain. Energy 35 (7), 686–700. doi:10.1080/14786451.2014.936867
Qiu, Y., Colson, G., and Grebitus, C. (2014). Risk preferences and purchase of energy-efficient technologies in the residential sector. Ecol. Econ. 107, 216–229. doi:10.1016/j.ecolecon.2014.09.002
Quaglione, D., Cassetta, E., Crociata, A., and Sarra, A. (2017). Exploring additional determinants of energy-saving behaviour: The influence of individuals’ participation in cultural activities. Energy Policy 108, 503–511. doi:10.1016/j.enpol.2017.06.030
Reddy, S., and Painuly, J. P. (2004). Diffusion of renewable energy technologies — Barriers and stakeholders' perspectives. Renew. Energy 29, 1431–1447. doi:10.1016/j.renene.2003.12.003
Sardianou, E., and Genoudi, P. (2013). Which factors affect the willingness of consumers to adopt renewables energies? Renew. Energy 57, 1–4. doi:10.1016/j.renene.2013.01.031
Steg, L. (2008). Promoting household energy conservation. Energy Policy 36 (12), 4449–4453. doi:10.1016/j.enpol.2008.09.027
Takama, T., Tsephel, S., and Johnson, F. X. (2015). Evaluating the relative strength of product-speci fi c factors in fuel switching and stove choice decisions in Ethiopia. A discrete choice model of household preferences for clean cooking alternatives. Energy Econ. 34 (6), 1763–1773. doi:10.1016/j.eneco.2012.07.001
Teklewold, H., Kassie, M., and Shiferaw, B. (2013). Adoption of multiple sustainable agricultural practices in rural Ethiopia. J. Agric. Econ. 64 (3), 597–623. doi:10.1111/1477-9552.12011
Urban, J., and Ščasný, M. (2012). Exploring domestic energy-saving: The role of environmental concern and background variables. Energy Policy 47, 69–80. doi:10.1016/j.enpol.2012.04.018
Vassileva, I., Wallin, F., and Dahlquist, E. (2012). Analytical comparison between electricity consumption and behavioral characteristics of Swedish households in rented apartments. Appl. Energy 90 (1), 182–188. doi:10.1016/j.apenergy.2011.05.031, No.
Venkatesh, V., Morris, M. G., Davis, G. B., and Davis, F. D. (2003). User acceptance of informa-tion technology: Toward a unified view. MIS Q. 27, 425–478. doi:10.2307/30036540
Weber, L. (1997). Some reflections on barriers to the efficient use of energy. Energy Policy 25 (10), 833–835. doi:10.1016/s0301-4215(97)00084-0
World Energy Council (2016). “World energy trilemma 2016 defining measures to accelarate the energy transition,” in World energy Council report. http://www.worldenergy.org/publications/2016/world-energy-trilemma-2016-defining-measures-to-accelerate-the-energy-transition/.
Keywords: energy efficient technologies, adoption, multivariate probit, urban, Ethiopia
Citation: Hassen S, Anandarajah G and Seifemichael R (2023) Behavioral and socio-economic determinants of urban households’ investment in energy efficient technologies: evidence from Ethiopia. Front. Energy Res. 11:1135291. doi: 10.3389/fenrg.2023.1135291
Received: 31 December 2022; Accepted: 21 April 2023;
Published: 31 July 2023.
Edited by:
Constantinos S. Psomopoulos, University of West Attica, GreeceReviewed by:
Seife Ayele, University of Sussex, United KingdomWojciech Florkowski, University of Georgia, Griffin Campus, United States
Copyright © 2023 Hassen, Anandarajah and Seifemichael. This is an open-access article distributed under the terms of the Creative Commons Attribution License (CC BY). The use, distribution or reproduction in other forums is permitted, provided the original author(s) and the copyright owner(s) are credited and that the original publication in this journal is cited, in accordance with accepted academic practice. No use, distribution or reproduction is permitted which does not comply with these terms.
*Correspondence: Gabrial Anandarajah, Zy5hbmFuZGFyYWphaEB1Y2wuYWMudWs=