- 1School of Statistics and Data Science, Nankai University, Tianjin, China
- 2Department of Mathematics, University of Management and Technology, Lahore, Pakistan
- 3Center of Research, Faculty of Engineering, Future University in Egypt New Cairo, Cairo, Egypt
- 4Department of Computer Science, College of Science and Humanities at Alghat, Majmaah University, Al-Majmaah, Saudi Arabia
- 5School of Mathematical Sciences, Zhejiang Normal University, Jinhua, Zhejiang, China
The capability to stock energy and manage consumption in the future is one of the keys to retrieving huge quantities of renewable energy on the grid. There are numerous techniques to stock energy, such as mechanical, electrical, chemical, electrochemical, and thermal. The q-rung orthopair fuzzy soft set (q-ROFSS) is a precise parametrization tool with fuzzy and uncertain contractions. In several environments, the attributes need to be further categorized because the attribute values are not disjointed. The existing q-rung orthopair fuzzy soft set configurations cannot resolve this state. Hypersoft sets are a leeway of soft sets (SSs) that use multi-parameter approximation functions to overcome the inadequacies of prevailing SS structures. The significance of this investigation lies in anticipating Einstein-ordered weighted aggregation operators (AOs) for q-rung orthopair fuzzy hypersoft sets (q-ROFHSSs), such as the q-rung orthopair fuzzy hypersoft Einstein-ordered weighted average (q-ROFHSEOWA) and the q-rung orthopair fuzzy hypersoft Einstein-ordered weighted geometric (q-ROFHSEOWG) operators, using the Einstein operational laws, with their requisite properties. Mathematical interpretations of decision-making constrictions are considered able to ensure the symmetry of the utilized methodology. Einstein-ordered aggregation operators, based on prospects, enable a dynamic multi-criteria group decision-making (MCGDM) approach with the most significant consequences with the predominant multi-criteria group decision techniques. Furthermore, we present the solicitation of Einstein-ordered weighted aggregation operators for selecting thermal energy-storing technology. Moreover, a numerical example is described to determine the effective use of a decision-making pattern. The output of the suggested algorithm is more authentic than existing models and the most reliable to regulate the favorable features of the planned study.
1 Introduction
Thermal energy storage (TES) is a technique for storing thermal energy by heating or cooling the storage medium so that the stockpiled energy can be used for consequent central heating and preservation solicitations and power generation. Energy storage systems are used primarily in construction and engineering practices. In these scenarios, almost half of the energy used is for heating, and its mandate can fluctuate daily. Thus, TES schemes can support stability mandates and daily, weekly, and periodic energy resources. They can also diminish ultimate declaration, energy ingestion, carbon dioxide releases, and expenditures while benefitting the whole productivity of energy schemes. Furthermore, the transformation and storing of adaptable renewable energy sources in the arrangement of thermal energy support increases the proportion of renewable energy in the energy mix. TES is mainly significant for power storing, combined with concentrated solar plants, which can stock solar heat to produce electricity without sunlight. TES is essential in several industrial scenarios. For example, one of the real-world problems related to solar energy schemes is the necessity for operational capital through which it can be stockpiled. Comparable difficulties exist in excess heat recovery schemes everywhere, and surplus heat accessibility and exploitation phases fluctuate. Heat storing can also be pragmatic for most kinds of construction where the heating system mandate is extraordinary and electrical energy notices permit heat storing to coexist with other arrangements of the heating system. There are numerous kinds of transaction systems, and several novel improvements are attracting interest in this area. Energy storage technology supports storing energy to avoid future energy complications. The thermal energy storage technique (TEST) is the most important energy technology. Dincer (2002) demonstrated in his study that the TEST is an essential energy storage skill for energy conversion. Monetary concerns act as a stimulus for energy transformation schemes, which makes the TEST even more important. Koçak et al. (2020) found that the TEST is a beneficial procedure with several uses in engineering. This TES solution can potentially escalate thermal energy apparatus usage on an elective foundation. There are commonly four different forms of TEST: sensible TEST, latent TEST, thermochemical TEST, and underground TEST. The prerequisite storage period typically affects the type of TEST used. It is anticipated that it will become one of the most effective thermal uses in this field.
Multi-criteria group decision-making (MCGDM) is the best way to manage suitable alternatives due to all the reliable assumptions, standards, or structures that come with it. A full assessment occurs when realistic intentions and confines are often unclear or imperfect. Zadeh (1965) initiated the notion of fuzzy sets (FSs) to demonstrate this incorrect and inconsistent information. It is imperative to compact with redundant and insecure decision-making (DM) matters. The FS model is used in various fields. Cavallaro (2010) established the fuzzy TOPSIS methodology to assess TES in concerted solar developments. Gumus et al. (2013) presented fuzzy AHP and fuzzy GRA approaches for choosing hydrogen energy systems. Contemporary FSs cannot handle cases when the regular consideration of membership degree (MD) and non-membership degree (NMD) by experts differs in the DM system. Atanassov (1986) addressed these limitations and demonstrated intuitionistic fuzzy sets (IFSs). Wang and Liu (2011) offered fundamental operations and aggregation operators (AOs) under their considered environment. Xu (2007a) furthered the theory of IFS and utilized the score and accuracy function to compute the relationship among two intuitionistic fuzzy numbers. Garg (2018) extended the cosine similarity measures (SMs), aiming to support DM proficiency. Lin et al. (2007) furthered the theory of IFS and demonstrated an advanced multi-criterion decision-making (MCDM) model. De et al. (2000) determined IFS concentration, normalization, and dilation operations. Garg and Kaur (2022) introduced the correlation measures for complex IFSs and utilized their developed measures to resolve MCDM obstacles. An IFS cannot apprehend unstable and confusing facts as it envisages a direct irregularity among MD and NMD. If the panel picks MD and NMD, such as
Yager (2013) proposed that Pythagorean fuzzy sets (PFSs) overcome this deficiency by modifying the fundamental states
The aforementioned structures have a wide range of applications, but none of the above structures can handle alternative parameters. Molodtsov (1999) proposed the soft set (SS) notion to interact with the parametric standards of the alternatives. Maji et al. (2003) introduced several fundamental operations for SSs and discussed their significant properties. Cagman and Enginoglu (2011) extended the SS model to a fuzzy parametrized SS with some important tasks. They also extended the DM methodology to validate their established theory. Ali et al. (2009) introduced several fundamental operations for SSs. Maji et al. (2001a) fused two eminent models, FS and SS, and proposed the fuzzy soft set (FSS) theory. Roy and Maji (2007) extended a theoretical DM tool for FSSs to deal with obscure and invalid information. Maji et al. (2001b) developed the intuitionistic FSS (IFSS) with its complementary properties. Arora and Garg (2018) proposed an MCDM technique for IFSSs to resolve DM complications using their developed AOs. Çağman and Karataş (2013) extended the notion of IFSSs and presented their basic operations with a DM model to resolve real-life difficulties. Muthukumar and Krishnan (2016) proposed some novel SMs with important properties for IFSSs. Peng et al. (2015) constructed the Pythagorean fuzzy soft set (PFSS) with a mixture of PFSs and SSs. Athira et al. (2019) andAthira et al. (2020) extended the idea of PFSSs and introduced entropy and distance measures. Zulqarnain et al. (2022a) proposed Einstein operational laws for PFSSs and developed the Einstein AOs. They used their developed AOs to resolve MAGDM complications. Zulqarnain et al. (2021a) presented the Einstein-ordered averaging operator and developed a DM methodology to determine the supplier selection problem. They also introduced the Einstein-ordered geometric operator and used it to establish the MAGDM technique (Zulqarnain et al., 2022b). Athira et al. (2022) extended SMs for PFSSs with fundamental results and used their developed similarity measures for clustering analysis. Hussain et al. (2020) expanded the PFSS to a q-ROFSS and developed the AOs based on algebraic operational laws. Zulqarnain et al. (2022c) andZulqarnain et al. (2022d) extended the Einstein AOs for q-ROFSSs and established DM methodologies based on their developed operators. Akram et al. (2021b) introduced Yager AOs for q-ROFSSs and developed an MAGDM strategy to resolve DM issues. However, these AOs cannot resolve the MCGDM complexities where any expert considers the sub-parametric value of any parameter.
The models with SS configuration compact with single-parameter estimation functions, although hypersoft sets (HSSs), a leeway of SS, contract with multi-parameter approximation. The SS cannot grasp states wherever parameters are divided into further sub-attributes. To overcome such complications, Smarandache (2018) extended the SS to the HSS, the most generalized model to handle the multiple sub-attributes of the deliberated parameters. Rahman et al. (2022a) developed SMs for the possibility of an intuitionistic fuzzy supersoft set (IFHSS). Rahman et al. (2022b) introduced novel operations for fuzzy HSSs and established a MADM structure utilizing their developed operators. Zulqarnain et al. (2021b) presented AOs for IFHSSs engaging their algebraic operational laws. They also introduced the Pythagorean fuzzy hypersoft set (PFHSS) (Zulqarnain et al., 2021c) and discussed their significant properties. Siddique et al. (2021) delivered a creative MCDM system for PFHSSs using their developed AOs. Sunthrayuth et al. (2022) and Zulqarnain et al. (2022e) predicted the Einstein AOs for PFHSSs to obstinacy MCDM impediments and used them for agri-farming and material selection consistently. Zulqarnain et al. (2022f) developed Einstein-ordered AOs for PFHSSs and formulated an MCDM approach to resolve DM complexities.
Khan et al. (2021) extended the q-ROFSS to q-ROFHSS and introduced several fundamental operations. Gurmani et al. (2022) extended the TOPSIS method to q-ROFHSSs built on the correlation coefficient (CC). Khan et al. (2022c) presented the operational laws for q-ROFHSSs and developed the AOs. They also built a DM methodology using their proposed AOs and utilized it in the cryptocurrency market. Zulqarnain et al. (2022g) extended the interaction AOs of q-ROFHSSs to cryptocurrency analysis. A better-integrated organization is of interest to researchers with inadequate, incredible, and irregular facts to debate these flaws. They explained the importance of deliberation; q-ROFHSS is anticipated to play a dynamic role in DM by accumulating affluent cradles in a specific judgment.
1.1 Motivation
The q-ROFHSS is a mixed rational structure of HSSs and q-ROFSs, the basic mathematical tool for dealing with hesitancies, discrepancy, and imperfect details. AOs perform a vital role in DM, so facts concerning communal judgments from various causes can be ascribed to distinctive assessments. Einstein’s operational laws have no application in the literature with the hybridization of HSSs and q-ROFSs. Thus, the prevalent method neither has quantitative concise q-rung orthopair fuzzy hypersoft numbers (q-ROFHSNs) nor is it deliberately correlated with MD and NMD. The effect of MD (NMD) on the subsequent AOs does not interfere with the whole procedure. Furthermore, the model ranks the whole level of the MD (NMD) function, independent of the level of the NMD (MD) function. Therefore, by giving these AOs, the outcomes are obstructive, and consequently, the applicable partiality for alternatives is not determined. Therefore, it is necessary to know how to incorporate these q-ROFHSNs for Einstein operational laws. To resolve such queries, we introduce the q-rung orthopair fuzzy hypersoft Einstein-ordered weighted average (q-ROFHSEOWA) and the q-rung orthopair fuzzy hypersoft Einstein-ordered weighted geometric (q-ROFHSEOWG) operators for q-ROFHSSs. The prevalent Einstein-ordered AOs become the special cases of q-ROFHSSs. Therefore, it is determined that the proposed model is more competent than existing Einstein AOs. Thus, the consequence of the prevalent models is adverse, and the favoritism of the alternative cannot be configured appropriately. Therefore, incorporating these q-ROFHSNs into Einstein’s specification is an exciting subject. The methodologies proposed by Khan et al. (2022c) are inadequate to check the facts on flexible perspectives to achieve well-thought and specific outcomes. For example, we consider the set of two experts, such as
1.2 Contribution
Einstein’s ordered weighted AOs are undoubtedly of interest in terms of the assessed AOs. It has been perceived that the general AO features do not respond to the finding of explicit effects by the DM scheme under apparent conditions. These AOs need to be reformed to eliminate these thorny problems. Therefore, to illuminate the current study of q-ROFHSSs and the aforementioned limitations, we assign Einstein-ordered weighted AOs founded on uncertain facts, with the primary purpose of the research given as follows:
⁃ The Einstein-ordered weighted AOs under q-ROFHSS settings are acquainted with attractive estimation AOs. It is believed that in some states, the main conceptual feature is the lack of sympathetic labeling of particular consequences of the DM process. To overcome such rigorous impairments, we extend both the idea of q-ROFHSSs and some novel AOs for q-ROFHSSs considering the Einstein operational laws.
⁃ q-ROFHSS expertly clarifies the obligation of the multiple sub-attributes of intellectual aspects in DM structures. To ensure this, we use Einstein’s ordered weighted AOs to represent q-ROFHSSs.
⁃ We present the q-ROFHSEOWA and q-ROFHSEOWG operators with their appropriate properties.
⁃ An excellent procedure with the projected AOs is presented to integrate MCGDM anxieties into the q-ROFHSS setting to assert DM negligence and to prioritize the TEST.
⁃ A comprehensive analysis of the advanced MCGDM methodology and predominant approaches is performed to confirm the validity and excellence of the proposed MCGDM approach.
The remainder of this paper is structured as follows: Section 2 contains some basic notions that sustain our organizational development follow-up study. Section 3 anticipates some Einstein operational laws for q-ROFHSNs, as well as introducing the q-ROFHSEOWA, with some significant results and properties. The q-ROFHSEOWG operator with its essential properties is presented in section 4. The MCGDM technique is developed with the proposed AOs, and a mathematical illustration is discussed to certify the practicality of the methodology in section 5. Moreover, a brief sensitivity exploration and comparative studies appear to highlight the advantages of the demonstrated approach in sections 6, 7, respectively.
2 Preliminaries
In the following section, we present some fundamental notions to construct this research.
Definition 2.1. (Molodtsov, 1999) Let
It can also be defined as follows:
Definition 2.2. (Smarandache, 2018) Let
Definition 2.3. (Zulqarnain et al., 2021c) Let
It can also be defined as
Occasionally, the scoring function does not deliver an appropriate result for calculating PFHSNs. It is challenging to draw conclusions about which alternative is informal. To overcome these barriers, accuracy functions are used:
To compare the two PFHSNs
1. If
2. If
• If
• If
Definition 2.4. (Sunthrayuth et al., 2022) Let
1.
2.
3.
4.
These existing AOs for PFHSSs were developed based on algebraic operational laws, and Einstein’s operational laws failed to handle the situation when
Definition 2.5. (Khan et al., 2021) Let
It can also be defined as
Definition 2.6. (Khan et al., 2022c) Let
1.
2.
3.
4.
Remark 2.1.
1. If
2. If
Let
If
If
○ If
○ If
Definition 2.7. (Klement et al., 2004)Einstein operations comprise the Einstein product and Einstein sum, which are examples of t-norm and t-conorm, respectively, and can be defined as follows:
The aforementioned Einstein product
3 Einstein-ordered weighted average aggregation operator for q-rung orthopair fuzzy hypersoft sets
This section introduces a novel Einstein-ordered weighted average AO for q-ROFHSNs with the most necessary properties.
Definition 3.1. Let
1.
2.
3.
4.
Definition 3.2. Let
In this manuscript,
Theorem 3.1. Let
Proof. We demonstrate this by mathematical induction.For
For
So, Eq. 3.2 is true for
For
So, it holds for
Example 3.1. Let
The associated ordered position matrix using Eq. 2.5 is given as follows:
As we know,
For
3.1 Properties of the q-rung orthopair fuzzy hypersoft Einstein-ordered weighted average operator
3.1.1 Idempotency
Let
Proof. As
3.1.2 Boundedness
Let
Proof. Let
Let
We have
Let q-ROFHSEOWA
From the aforementioned discussion, we have the following consequences.If
If
3.1.3 Homogeneity
Prove that q-ROFHSEOWA
Proof. Let
So, q-ROFHSEOWA
3.1.4 Monotonicity
Let
Proof. Let
where
Let
where,
(ii):
From 3.5 and 3.6, we get
So, it proved that
4 Einstein-ordered weighted geometric aggregation operator for q-rung orthopair fuzzy hypersoft sets
The following section will introduce the Einstein-ordered weighted geometric operator for q-ROPFHSSs with some important possessions.
Definition 4.1. Let
where
Theorem 4.1. Let
where
Proof. We use mathematical induction to demonstrate the aforementioned result.For
For
Eq. 4.2 is true for
So, it holds for
Example 4.1. Let
The related ordered position matrix using Eq. 2.5 is as follows:
As we know,
For
4.1 Properties of the q-rung orthopair fuzzy hypersoft Einstein-ordered weighted geometric operator
4.1.1 Idempotency
Let
Proof. As we know that
4.1.2 Boundedness
Let
Proof. Let
Again,
We have
Let q-ROFHSEOWG
From the aforementioned procedure, we have the following consequences. If
If
q-ROFHSEOWG
4.1.3 Homogeneity
Prove that q-ROFHSEOWG
Proof. Let
So, q-ROFHSEOWG
4.1.4 Monotonicity
Let
Proof. Similar to 3.2.4.
5 Multi-criteria group decision model for q-rung orthopair fuzzy hypersoft sets
To substantiate the inference of the established Einstein-ordered weighted AOs, there is a DM method to eradicate MCGDM constraints. We also used the developed approach to select the most appropriate TEST.
5.1 Proposed multi-criteria group decision approach
Let
Step 1. Compute the decision matrices for each alternate in terms of q-ROFHSNs
Step 2. Obtain the ordered position matrices for each alternative using the score function.
Step 3. Convert the cost type aspects into benefit types using the normalization rule.
Step 4. With settled Einstein-ordered weighted AOs, compute the collective decision matrix
Step 5. For the ranking of alternatives, find the score values using Eq. 2.5.
Step 6. Analyze the most apt TEST based on the maximum score value
Step 7. Compute the ordering of the substitutes.The flowchart of the proposed algorithm is given in the following Figure 1.
5.2 Application of the proposed multi-criteria group decision approach
The energy storage method is intended to store energy when fabrication surpasses the mandate and to provide power according to consumers’ desires. They can easily conserve energy resources and overcome inconstant fabrication from renewable energy sources such as solar and wind, increase the productivity of energy systems, and decrease carbon dioxide emissions. It focuses on diminutive heat storage schemes. The purpose of energy storing is to apprehend energy and deliver it professionally for upcoming usage. Energy storage technology has numerous important advantages: enhanced solidity of power eminence, consistency of power quantity, etc. In the modern era, given the current energy crisis, energy storage has become the core focus of study in engineering and academia. The storing procedure is a necessary constituent of energy-proficient and justifiable energy systems. Energy storage is a cross-cutting problem that depends on several capabilities. Energy storage cooperation stimulates the widespread familiarity, management, and interdisciplinary organization of work agendas and exploration intentions. The energy storage technique generally supports accomplishing energy controlling on-demand sideways, addressing differences in power claims, power stock worth, and long-term dependability. Incorporating energy-storing classifications can clearly show the influence and energy experiments challenged by customary schemes. Energy storage types of machinery require a variety of forms, from large-scale power groups and transmission-based strategies to linkage circulation systems. Although different types of energy storing expertise exist, it can be seen that each storing method accomplishes its goal differently owing to its intrinsically different storing methods. Currently, people are gradually being attracted to the enlargement of energy-storing knowledge. Soon, storage capability intensities in established states can be amplified by 15–25%, although this rate may increase in emerging states. In this way, the important role of power engineering can be amended for better results. In the current circumstances, pushed hydro storage may be superior to other energy-storing arrangements that are not as effective in terms of large-scale energy storage. CAES schemes can participate with impelled hydropower schemes. However, their development may only be seen in states with high ethical values and auspicious geographical circumstances. Battery-operated expertise can support the energy market and storage, satisfying the need for electricity on demand.
Similarly, in recent years, in small and medium enterprises, flywheels, flow batteries, fuel cells, TES, etc., have become keys in the energy division. Their rapid payback makes them beneficial for several short- and long-term solicitations, particularly in terms of electrical superiority. In short, the energy productivity of extraordinary energy concentration and energy storing machinery, growing storage paybacks, solidity, dependability, energy preservation, and ecological sustainability predictions marks them consistently as the best choice for cumulative energy requirements. Due to the proliferation in heat, thermal energy is produced due to the pileup of atoms and spots. TES philosophy is used in several arenas of biological disciplines. Essentially, there are three skills for TES. The graphical representation of TES techniques is provided in Figure 2.
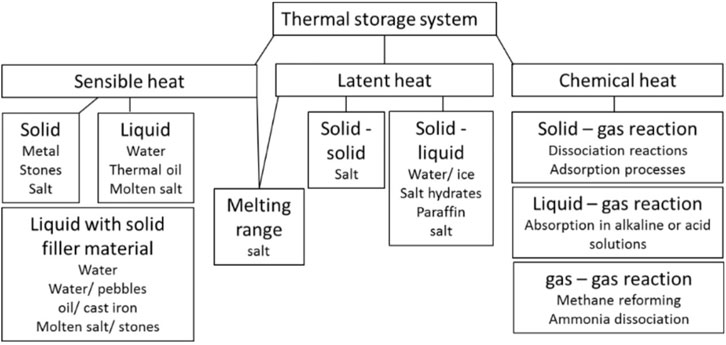
FIGURE 2. Thermal energy storage overview (Source: https://www.beilstein-journals.org/bjnano/content/figures/2190-4286-6-154-1.png?scale=2.0&max-width=1024&background=FFFFFF).
5.2.1 Sensible TEST
Sensible heat storage (SHS) is a system where energy is contained by increasing or reducing the heat of the stored substance. This storage medium can be a solid or a liquid. Water is economical and one of the most frequently used mediums. The graphical representation of the sensible TEST is displayed in Figure 3. Sensible thermal energy storage (STES) is used in numerous mechanisms, for example, construction and solar power plants. In solar preservation, solar food drying, and solar cooking, STES is the easiest and most established method of heat storage. Sensible thermal energy (STE) is stored by fluctuating the heat of STES ingredients, such as water, oil, rock beds, bricks, sand, or clay. There is no stage evolution through heat modifications in STES ingredients. SHS is easy to control and rationally exclusive. It is the best traditional, developed, and broadly used TES resolution. However, it achieves less energy-storing compactness than other TES alternatives, namely, latent heat storage (LHS) and thermochemical heat storage (TCHS) (Zhao et al., 2020). In this process, the transference of energy to storage liquids or solids suggests the identical alteration in the warmth of the medium. One of the benefits of this approach is that the storing and discharge of stored heat (charge and discharge phases) can be continually made easier through the accumulation of huge capacities to achieve this goal (Chandel and Agarwal, 2017).
Furthermore, this scheme often benefits from specific features of the stockpiled substance, such as its particular high temperature (Rathore and Shukla, 2019). It is necessary to know two core features of SHS ingredients to increase the storing ability (MJ•
5.2.2 Latent TEST
The LHS system implies storing energy in phase change materials (PCMs). Thermal energy is stored and released when the storage material changes the segments. The benefit of LHS schemes is that they are usually condensed. For heat-storing, the PCM size is considerably smaller than the size of the SHS. This is essential since it affects the usage of fewer separations and is functional everywhere around the world. Other benefits of PCMs comprise the capability to stock huge volumes of heat with minor temperature alterations and being robust to severe operative temperatures since they can handle exertion under isothermal circumstances and have extraordinary storing compactness. Compared to SHS systems, LHS systems have almost five to ten times greater storing capacity. The stage alteration can be from solid to liquid, solid to gas, solid to solid, liquid to gas, and vice versa. In solid-stage alteration, heat is stockpiled as the substantial transmission from one sparkler organization to another. This stage conversion, i.e., solid-to-solid alteration, has less latent heat: high latent heat has issues and high capacity deviations connected with solid-to-gas and liquid-to-gas transformation. However, considerable variations in size can arise due to the requirement for a larger vessel. In several circumstances, this makes this method unrealistic. For the causes stated formerly, the best stage alteration is the transition from solid to liquid as it is associated with minor changes in volume, although the latent heat of the solid to liquid growth is lesser than that of liquid to gas. Solid to liquid material alteration is also economical as a thermal energy storing mode. The PCM itself cannot be reused as a heat transmission mode. An isolated heat transmission cause should be retrieved, with a heat exchanger in the interior, to transfer energy from the basis to the PCM and the PCM to the load. Assuming that the PCM’s thermal dispersal is usually small, consideration must be given to the role of the temperature exchanger (see Figure 4).
Compared to SHS, LHS systems have a moderately good energy solidity and have been used for low- and medium-temperature scenarios, for example, thermal regulation in buildings (De Gracia and Cabeza, 2015). Therefore, from the perspective of excessive heat, LHS systems aim to incorporate prodigious heat-concentrated solar power demonstrations. The low thermal conductivity, low thermal constancy, and restrained temperature of PCMs found in present LHS systems are critical barriers to their use, even if they have significant energy-storing strengths. In customary concealed storage systems, there are different methods to progress thermal enactment through organs, flowing unlike PCMs and embalmed PCMs (Jegadheeswaran and Pohekar, 2009). However, these approaches trade off PCMs’ retention capacity with their size, resulting in a reduction in the energy storage capability through the charging/discharge of the LHS system (Lin et al., 2018). One viable substitute is metal PCMs with unusual thermal conductivity, sentimental warmth, and abundant thermal solidity. Generally, the melt’s latent heat and the mixture material’s thermal constancy rise with the growth in the soppy heat (Bauer et al., 2012). Hence, there is an increasing need to understand PCMs in terms of unexpected melting point heats.
5.2.3 Thermochemical TEST
TCHS is one of the potential TES techniques in the procedure of revocable thermochemical reactions. The main significant benefit of TCHS is that the enthalpy of the reaction is considerably more than the heat. Thus, the storing compactness is superior in organic responses, with energy stockpiled in biochemical bonds among molecules forming fragments. At the atomic level, energy storage contains energy related to the orbital of electrons. In addition to whether the chemical captures or discharges the reaction energy, there is no global measurement of the quantity of energy through the reaction. The purpose of incorporating the TCHS technique used by the solar thermal system (STS) is to increase the amount of solar energy and thus increase the productivity of the STS. The excess heat delivered by the summer stock accumulation controls the TCHS. In the wintertime, stored heat can be reprocessed to overcome the excessive heat mandate of the system. Regarding the heat mandate of the system and the extent of the TCHS, a fossil-fuel-assisted STS using a proportion of more than 50% can be used, as well as a pure STS. Figure 5 displays a schematic diagram of TCHS combined with an STS. TCHS is linked to the water shield storage usually integrated in the STS through solar circuits and heat exchangers. The core component of TCHS is the heat and mass transfer that arises through the charge and discharge of the TCHS reactor. Dependent on the regulatory policy, the best reactor is a fixed bed reactor. The material comes into the reactor from above, and passage over the reactor is determined by gravity. Air moves in the reactor and passes moisture and heat to and from the reactor. For air-to-air heat alteration, entering fresh air is heated by hot air departure in TCHS, which controls the productivity of TCHS as heat damage by the gas stream is reduced. The heat entering the reactor is transmitted from the airflow to the solar circuit via air-to-water heat exchange in TCHS, releasing heat. For material preservation, the airflow is absorbed in the opposite way and reprocessed to transference reformative heat from the solar circuit to the reactor through the heat exchange airflow.
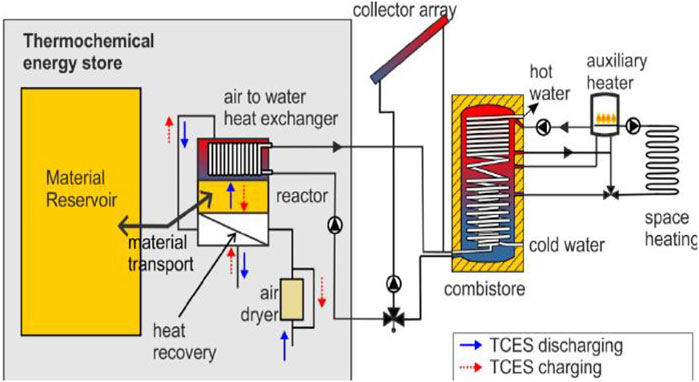
FIGURE 5. Thermochemical heat storage technique [Figure 1 (Mette et al., 2013)].
TCHS uses a series of reactions related to physical and chemical developments (for a provisional organization, see McNaught and Wilkinson, 1997). However, in this organization, adsorption is used to accumulate various physical properties and can lead to misinterpretations. We intentionally limit the organization development to out-of-phase reactions, including mechanisms of two or additional stages, as uniform responses are rarely used for TES. Prioritizing TES techniques is a significant initiative in this modern era. Industrial innovativeness is based on a vision, financial planning, environmental concerns, and the frequent lack of materials. The best design chooses the most appropriate TES technique by considering the most feasible financial plan. Several unreliable DM practices require prioritizing TES techniques, predominant AOs, and other DM methodologies in this context. These AOs need to be restructured to address these concerns. We recommend specific innovative ordered AOs considering the Einstein operational laws for accumulating helpful q-ROFHSNs. Considering that mentioned previously, DM discernment can characterize all configurations. Attention is first needed on prioritizing the TES technique and then on other features’ impacts to identify the multiple sub-parameters and ingredients for DM. The q-ROFHSS model and projected Einstein-ordered AOs can be used in this context.
Regarding the best features to contemplate when choosing an effective TES method in DM, it is necessary to select a process with a primary transmission of TES, focusing on rationalization configurations inherent to solicitation. MCGDM considers making decisions in the presence of several, often inconsistent, criteria. The different criteria can have a disparate scope and comparative weight. Some measures can be controlled scientifically, while others can only be intuitively determined. It is possible to use many methodologies to explain and overcome MCGDM obstacles. The MCGDM approach provides insights into numerous managerial difficulties. The primary aim of this study is to select the most appropriate TES technique based on Einstein-ordered weighted AOs under the q-ROFHSS environment.
5.3 Numerical example
Let
5.3.1 Using the q-rung orthopair fuzzy hypersoft Einstein-ordered weighted average operator
Step 1. Compute the decision matrices for each alternate in terms of q-ROFHSNs (their predilections are given in Table 1).
Step 2. Using the score function, obtain the ordered position matrices for each alternative (Table 2).
Step 3. There is no need to normalize since the parameters are the same.
Step 4. Determine the collective aggregated values of alternatives from Table 2 using the q-ROFHSEOWA operator given as:
Step 5. From Eq. 2.5, find the score values, such as
Step 6.
Step7. Investigate the ordering of the substitutes:
5.3.2 Using the q-rung orthopair fuzzy hypersoft Einstein-ordered weighted geometric operator
Steps 1–3. Similar to 5.3.1.
Step 4. Determine the collective aggregated values of alternatives from Table 2 using the q-ROFHSEOWG operator given as
Step 5. From Eq. 2.5, find the score values, such as
Step 6.
Step 7. Investigate the ordering of the substitutes:
6 Sensitivity analysis
This section evaluates the proposed approach and prevailing methodologies to confirm the practicality of the scheme.
6.1 Influence on the alternatives’ rank of the deviancy of for the q-rung orthopair fuzzy hypersoft Einstein-ordered weighted average operator
The organization training shows that
The proposed method makes fuzzy information easier to describe and makes it more flexible in combining facts with factors. When assembling some sequences, numerous amalgam structures of FS are converted into the special detail of q-ROFHSS (see Table 5). The parameter
6.2 Influence on alternatives’ rank of the deviancy of for the q-rung orthopair fuzzy hypersoft Einstein-ordered weighted geometric operator
To minimize the impact of “
7 Comparative analysis and discussion
This section compares the proposed model and predominant techniques to justify the practicality of our presented model.
7.1 Supremacy of the planned approach
The proposed scheme is effective and robust. We formulated an innovative MCGDM approach using q-ROFHSEOWA and q-ROFHSEOWG operators. The developed methodology in this research is more effective than prevalent methods and compatibility contracts for MCGDM problems. The proposed methodology is versatile and familiar, with disparities, accountabilities, and changes allowing for different outputs. Unlike models with explicit taxonomic comportment, there is a conventional alteration to the projected scheme classification to accommodate its perspective. Methodological studies and estimations consider that the consequences of prevalent approaches are similar to hybrid systems. Furthermore, after adding some suitable conditions, numerous amalgam configurations of FSs become the q-ROFHSS. Adding infrequent and blurred facts to the current practical plan is unexpected. Data concerning prosperity provide a more complete and reasonable description. Through the DM procedure, there are many fabricated and troubling details. Thus, our proposed methodology will be superior to several amalgam FS scenarios. Table 5 presents the feature analysis of our developed and prevalent approaches.
7.2 Comparative analysis
To demonstrate the efficacy of the established approach, we linked the inferences gained from some well-known systems. Table 6 summarizes the comparison between our developed model and existing AOs. Zulqarnain et al. (2022a) used the PFSEWA and PFSEWG operators to analyze the parametric values of the substitute. However, these Einstein AOs are ineffective in compacting with sub-parameters of the alternatives. Also, the Einstein-ordered AOs (Zulqarnain et al., 2021a; Zulqarnain et al., 2022b) for PFSSs cannot compute the alternatives’ multiple sub-attributes. The AOs presented in Zulqarnain et al. (2021b) under the IFHSS environment can diminish with the sub-parameters of substitutes. However, these AOs fail to deal with the decision outcomes when the sum of
Therefore, we have the right to be surprised by the exploitation and unreliability of the DM procedure for the prevailing operators we have recognized. Intentional sustenance for this method-related action has a slight influence on adverse reasons. In this way, it relaxes the organization of unreliable and assumed details in the amplification of DM. Figure 8 graphically demonstrates the comparison analysis.
The aforementioned discussion and comparative studies show that the present study can play a significant role in the scientific community in terms of assessing better alternatives. It is the most generalized mathematical model to discuss the sub-parameters of the alternatives in DM problems.
7.3 Advantages of the proposed research
In the following section, we highlight the advantages of the proposed approach:
• The proposed technique takes into account the concept of parameterization in aggregation and discusses the importance of DM constraints with q-ROFHSS. The MD and NMD replication scenarios of constant parameterization have a certain degree of notation and rationality. This approach has an outstanding ability to demonstrate computational operations in an incredible world with these capabilities.
• Since the model highlights an in-depth investigation of the set of values of the parameter and its associated sub-parameters, it supports decision-makers in DM labeling combinations and making reliable decisions.
8 Conclusion
The lack of contemplation of complex conditions in the features can obstruct some of the multifaceted inferences of MCGDM. The mathematical demonstration in MCGDM exploits all special effects while being of interest under the limits of finance, superiority, and welfare boundaries. It is necessary to limit the investigation to make decisions at the highest level and capture the need for decisions. In factual DM, estimates of alternative details recognized by professionals are often inaccurate, asymmetrical, and insignificant, so q-ROFHSNs are used to calculate this impulsive information. The core objective of this research is to perform the Einstein operational laws for q-ROFHSS. Using our developed Einstein operational laws, we propose q-ROFHSEOWA and q-ROFHSEOWG operators with their preferred properties. In addition, the DM approach is expected to resolve MCGDM bottlenecks based on proven operators. To illustrate the strength of the presented methodology, we provide a mathematical description of the TES technique. This article also provides a comprehensive analysis of some contemporary models. Finally, based on the results obtained, it can be concluded that the approach proposed in this research is undeniably the most specific and feasible system to clarify MCGDM issues. Future investigations should focus on defining Bonferroni mean AOs, distance, and similarity measures with their conforming characteristics. Researchers could also integrate q-ROFHSNs with other MCGDM approaches and further practical solicitations in medical diagnosis, material selection, pattern recognition, information fusion, and supply chain management problems. Several configurations can be developed for q-ROFHSS, such as topological, algebraic, and ordered structures, with their DM techniques. Moreover, it can be extended to the T-spherical fuzzy hypersoft set, interval-valued T-spherical fuzzy hypersoft set, and interval-valued q-ROFHSS, with their algebraic and Einstein operations. Several other decision-making methodologies can be developed considering settings such as TOPSIS, VIKOR, AHP, and MABAC.
Data availability statement
The original contributions presented in the study are included in the article/supplementary materials; further inquiries can be directed to the corresponding author.
Author contributions
Conceptualization, RZ; methodology, RZ; validation, IS, SE, and MS; formal analysis, SE and JM; investigation, JM, SH, and MS; writing—original draft preparation, IM and RZ; visualization, IM and MS; supervision, RZ and SE; project administration, SE and JM; funding acquisition, JM and SE. All authors have read and agreed to the published version of the manuscript.
Acknowledgments
The author extends their appreciation to the deputyship for Research & Innovation, Ministry of Education in Saudi Arabia for funding this research work through the project number (IFP-2020-138).
Conflict of interest
The authors declare that the research was conducted in the absence of any commercial or financial relationships that could be construed as a potential conflict of interest.
Publisher’s note
All claims expressed in this article are solely those of the authors and do not necessarily represent those of their affiliated organizations, or those of the publisher, the editors, and the reviewers. Any product that may be evaluated in this article, or claim that may be made by its manufacturer, is not guaranteed or endorsed by the publisher.
References
Akram, M., Alsulami, S., Karaaslan, F., and Khan, A. (2021). q-Rung orthopair fuzzy graphs under Hamacher operators. J. Intelligent Fuzzy Syst. 40 (1), 1367–1390. doi:10.3233/jifs-201700
Akram, M., Shahzadi, G., Butt, M. A., and Karaaslan, F. (2021). A hybrid decision making method based on q-rung orthopair fuzzy soft information. J. Intelligent Fuzzy Syst. 40 (5), 9815–9830. doi:10.3233/jifs-202336
Ali, M. I., Feng, F., Liu, X., Min, W. K., and Shabir, M. (2009). On some new operations in soft set theory. Comput. Math. Appl. 57 (9), 1547–1553. doi:10.1016/j.camwa.2008.11.009
Arora, R., and Garg, H. (2018). A robust aggregation operators for multi-criteria decision-making with intuitionistic fuzzy soft set environment. Sci. Iran. 25 (2), 931–942.
Atanassov, K. (1986). Intuitionistic fuzzy sets. Fuzzy Sets Syst. 20, 87–96. doi:10.1016/s0165-0114(86)80034-3
Athira, T. M., John, S. J., and Garg, H. (2020). A novel entropy measure of Pythagorean fuzzy soft sets. AIMS Math. 5 (2), 1050–1061. doi:10.3934/math.2020073
Athira, T. M., John, S. J., and Garg, H. (2019). Entropy and distance measures of Pythagorean fuzzy soft sets and their applications. J. Intelligent Fuzzy Syst. 37 (3), 4071–4084. doi:10.3233/jifs-190217
Athira, T. M., John, S. J., and Garg, H. (2022). Similarity measures of Pythagorean fuzzy soft sets and clustering analysis. Soft Comput., 1–16. doi:10.1007/s00500-022-07463-4
Bauer, T., Steinmann, W. D., Laing, D., and Tamme, R. (2012). Thermal energy storage materials and systems. Annu. Rev. Heat Transf. 15, 131–177. doi:10.1615/annualrevheattransfer.2012004651
Borri, E., Tafone, A., Zsembinszki, G., Comodi, G., Romagnoli, A., and Cabeza, L. F. (2020). Recent trends on liquid air energy storage: A bibliometric analysis. Appl. Sci. 10 (8), 2773. doi:10.3390/app10082773
Cagman, N., and Enginoglu, S. (2011). FP-soft set theory and its applications. Ann. Fuzzy Math. Inf. 2 (2), 219–226.
Çağman, N., and Karataş, S. (2013). Intuitionistic fuzzy soft set theory and its decision making. J. Intelligent Fuzzy Syst. 24 (4), 829–836. doi:10.3233/ifs-2012-0601
Cárdenas-Ramírez, C., Jaramillo, F., and Gómez, M. (2020). Systematic review of encapsulation and shape-stabilization of phase change materials. J. Energy Storage 30, 101495. doi:10.1016/j.est.2020.101495
Cavallaro, F. (2010). Fuzzy TOPSIS approach for assessing thermal-energy storage in concentrated solar power (CSP) systems. Appl. Energy 87 (2), 496–503. doi:10.1016/j.apenergy.2009.07.009
Chandel, S. S., and Agarwal, T. (2017). Review of current state of research on energy storage, toxicity, health hazards and commercialization of phase changing materials. Renew. Sustain. Energy Rev. 67, 581–596. doi:10.1016/j.rser.2016.09.070
De Gracia, A., and Cabeza, L. F. (2015). Phase change materials and thermal energy storage for buildings. Energy Build. 103, 414–419. doi:10.1016/j.enbuild.2015.06.007
De, S. K., Biswas, R., and Roy, A. R. (2000). Some operations on intuitionistic fuzzy sets. Fuzzy sets Syst. 114 (3), 477–484. doi:10.1016/s0165-0114(98)00191-2
Dincer, I. (2002). Thermal energy storage systems as a key technology in energy conservation. Int. J. energy Res. 26 (7), 567–588. doi:10.1002/er.805
Garg, H., Ali, Z., Mahmood, T., and Ali, M. R. (2022). TOPSIS-method based on generalized dice similarity measures with Hamy mean operators and its application to decision-making process. Alexandria Eng. J. 65, 383–397. doi:10.1016/j.aej.2022.10.043
Garg, H. (2018). An improved cosine similarity measure for intuitionistic fuzzy sets and their applications to decision-making process. Hacettepe J. Math. Statistics 47 (6), 1578–1594.
Garg, H., and Kaur, G. (2022). Algorithm for solving the decision-making problems based on correlation coefficients under cubic intuitionistic fuzzy information: A case study in watershed hydrological system. Complex and Intelligent Syst. 8 (1), 179–198. doi:10.1007/s40747-021-00339-4
Gumus, A. T., Yayla, A. Y., Çelik, E., and Yildiz, A. (2013). A combined fuzzy-AHP and fuzzy-GRA methodology for hydrogen energy storage method selection in Turkey. Energies 6 (6), 3017–3032. doi:10.3390/en6063017
Gurmani, S. H., Chen, H., and Bai, Y. (2022). Extension of TOPSIS method under q-rung orthopair fuzzy hypersoft environment based on correlation coefficients and its applications to multi-attribute group decision-making. Int. J. Fuzzy Syst., 1–14. doi:10.1007/s40815-022-01386-w
Hussain, A., Ali, M. I., Mahmood, T., and Munir, M. (2020). q-Rung orthopair fuzzy soft average aggregation operators and their application in multi-criteria decision-making. Int. J. Intelligent Syst. 35 (4), 571–599. doi:10.1002/int.22217
Hussain, A., Ullah, K., Alshahrani, M. N., Yang, M. S., and Pamucar, D. (2022). Novel Aczel–Alsina operators for Pythagorean fuzzy sets with application in multi-attribute decision making. Symmetry 14 (5), 940. doi:10.3390/sym14050940
Jana, C., Garg, H., and Pal, M. (2022). Multi-attribute decision making for power Dombi operators under Pythagorean fuzzy information with MABAC method. J. Ambient Intell. Humaniz. Comput., 1–18. doi:10.1007/s12652-022-04348-0
Javed, M., Javeed, S., Ullah, K., Garg, H., Pamucar, D., and Elmasry, Y. (2022). Approach to multi-attribute decision-making problems based on neutrality aggregation operators of T-spherical fuzzy information. Comput. Appl. Math. 41 (7), 310. doi:10.1007/s40314-022-01985-1
Jegadheeswaran, S., and Pohekar, S. D. (2009). Performance enhancement in latent heat thermal storage system: A review. Renew. Sustain. energy Rev. 13 (9), 2225–2244. doi:10.1016/j.rser.2009.06.024
Khan, M. R., Wang, H., Ullah, K., and Karamti, H. (2022). Construction material selection by using multi-attribute decision making based on q-rung orthopair fuzzy Aczel–Alsina aggregation operators. Appl. Sci. 12 (17), 8537. doi:10.3390/app12178537
Khan, R., Ullah, K., Pamucar, D., and Bari, M. (2022). Performance measure using a multi-attribute decision-making approach based on complex T-spherical fuzzy power aggregation operators. J. Comput. Cognitive Eng. 1 (3), 138–146. doi:10.47852/bonviewJCCE696205514
Khan, S., Gulistan, M., Kausar, N., Kousar, S., Pamucar, D., and Addis, G. M. (2022). Analysis of cryptocurrency market by using q-rung orthopair fuzzy hypersoft set algorithm based on aggregation operators. Complexity 2022, 1–16. doi:10.1155/2022/7257449
Khan, S., Gulistan, M., and Wahab, H. A. (2021). Development of the structure of q-rung orthopair fuzzy hypersoft set with basic operations. Punjab Univ. J. Math. 53 (12), 881–892. doi:10.52280/pujm.2021.531204
Klement, E. P., Mesiar, R., and Pap, E. (2004). Triangular norms. Position paper I: Basic analytical and algebraic properties. Fuzzy sets Syst. 143 (1), 5–26. doi:10.1016/j.fss.2003.06.007
Koçak, B., Fernandez, A. I., and Paksoy, H. (2020). Review on sensible thermal energy storage for industrial solar applications and sustainability aspects. Sol. Energy 209, 135–169. doi:10.1016/j.solener.2020.08.081
Kumar, S., and Garg, H. (2022). Some novel point operators and multiple rounds voting process based decision-making algorithm under picture fuzzy set environment. Adv. Eng. Softw. 174, 103274. doi:10.1016/j.advengsoft.2022.103274
Lin, L., Yuan, X. H., and Xia, Z. Q. (2007). Multicriteria fuzzy decision-making methods based on intuitionistic fuzzy sets. J. Comput. Syst. Sci. 73 (1), 84–88. doi:10.1016/j.jcss.2006.03.004
Lin, Y., Jia, Y., Alva, G., and Fang, G. (2018). Review on thermal conductivity enhancement, thermal properties and applications of phase change materials in thermal energy storage. Renew. Sustain. energy Rev. 82, 2730–2742. doi:10.1016/j.rser.2017.10.002
Lizana, J., Chacartegui, R., Barrios-Padura, A., and Valverde, J. M. (2017). Advances in thermal energy storage materials and their applications towards zero energy buildings: A critical review. Appl. Energy 203, 219–239. doi:10.1016/j.apenergy.2017.06.008
Mahmood, T., Ullah, K., Khan, Q., and Jan, N. (2019). An approach toward decision-making and medical diagnosis problems using the concept of spherical fuzzy sets. Neural Comput. Appl. 31 (11), 7041–7053. doi:10.1007/s00521-018-3521-2
Maji, P. K., Biswas, R., and Roy, A. R. (2001). Intuitionistic fuzzy soft sets. J. Fuzzy Math. 9, 677–692.
Maji, P. K., Biswas, R., and Roy, A. R. (2003). Soft set theory. Comput. Math. Appl. 45 (4-5), 555–562. doi:10.1016/s0898-1221(03)00016-6
McNaught, A. D., and Wilkinson, A. (1997). IUPAC compendium of chemical terminology. 2nd ed. Hoboken, NJ, USA: Wiley Blackwell.
Mette, B., Kerskes, H., Drück, H., Badenhop, T., Salg, F., and Gläser, R. (2013). “Thermochemical energy storage as an element for the energy turnaround,” in 8th int. Renew. Energy storage conf. Exhib.(IRES 2013 (Berlin, 1–10.
Molodtsov, D. (1999). Soft set theory—First results. Comput. Math. Appl. 37 (4-5), 19–31. doi:10.1016/s0898-1221(99)00056-5
Muthukumar, P., and Krishnan, G. S. S. (2016). A similarity measure of intuitionistic fuzzy soft sets and its application in medical diagnosis. Appl. Soft Comput. 41, 148–156. doi:10.1016/j.asoc.2015.12.002
Peng, X. D., Yang, Y., Song, J., and Jiang, Y. (2015). Pythagorean fuzzy soft set and its application. Comput. Eng. 41 (7), 224–229.
Rahman, A. U., Saeed, M., and Garg, H. (2022). An innovative decisive framework for optimized agri-automobile evaluation and HRM pattern recognition via possibility fuzzy hypersoft setting. Adv. Mech. Eng. 14 (10), 168781322211321. doi:10.1177/16878132221132146
Rahman, A. U., Saeed, M., Khalifa, H. A. E. W., and Afifi, W. A. (2022). Decision making algorithmic techniques based on aggregation operations and similarity measures of possibility intuitionistic fuzzy hypersoft sets. AIMS Math. 7 (3), 3866–3895. doi:10.3934/math.2022214
Rahman, K., Abdullah, S., Ahmed, R., and Ullah, M. (2017). Pythagorean fuzzy Einstein weighted geometric aggregation operator and their application to multiple attribute group decision making. J. Intelligent Fuzzy Syst. 33 (1), 635–647. doi:10.3233/jifs-16797
Rathore, P. K. S., and Shukla, S. K. (2019). Potential of macroencapsulated PCM for thermal energy storage in buildings: A comprehensive review. Constr. Build. Mater. 225, 723–744. doi:10.1016/j.conbuildmat.2019.07.221
Riaz, M., Farid, H. M. A., Karaaslan, F., and Hashmi, M. R. (2020). Some q-rung orthopair fuzzy hybrid aggregation operators and TOPSIS method for multi-attribute decision-making. J. Intelligent Fuzzy Syst. 39 (1), 1227–1241. doi:10.3233/jifs-192114
Roy, A. R., and Maji, P. K. (2007). A fuzzy soft set theoretic approach to decision making problems. J. Comput. Appl. Math. 203 (2), 412–418. doi:10.1016/j.cam.2006.04.008
Sarkar, A., Biswas, A., and Kundu, M. (2022). Development of q-rung orthopair trapezoidal fuzzy Einstein aggregation operators and their application in MCGDM problems. J. Comput. Cognitive Eng. 1 (3), 109–121.
Siddique, I., Zulqarnain, R. M., Ali, R., Jarad, F., and Iampan, A. (2021). Multicriteria decision-making approach for aggregation operators of Pythagorean fuzzy hypersoft sets. London, United Kingdom: Computational Intelligence and Neuroscience.
Smarandache, F. (2018). Extension of soft set to hypersoft set, and then to plithogenic hypersoft set. Neutrosophic Sets Syst. 22, 168–170.
Sunthrayuth, P., Jarad, F., Majdoubi, J., Zulqarnain, R. M., Iampan, A., and Siddique, I. (2022). A novel multicriteria decision-making approach for Einstein weighted average operator under Pythagorean fuzzy hypersoft environment. J. Math. 2022, 1–24. doi:10.1155/2022/1951389
Tarragona, J., de Gracia, A., and Cabeza, L. F. (2020). Bibliometric analysis of smart control applications in thermal energy storage systems. A model predictive control approach. J. Energy Storage 32, 101704. doi:10.1016/j.est.2020.101704
Thao, N. X., and Smarandache, F. (2019). A new fuzzy entropy on Pythagorean fuzzy sets. J. Intelligent Fuzzy Syst. 37 (1), 1065–1074. doi:10.3233/jifs-182540
Ullah, K. (2021). Picture fuzzy Maclaurin symmetric mean operators and their applications in solving multiattribute decision-making problems. Math. Problems Eng. 2021, 1–13. doi:10.1155/2021/1098631
Wang, L., and Li, N. (2020). Pythagorean fuzzy interaction power Bonferroni mean aggregation operators in multiple attribute decision making. Int. J. Intelligent Syst. 35 (1), 150–183. doi:10.1002/int.22204
Wang, W., and Liu, X. (2011). Intuitionistic fuzzy geometric aggregation operators based on Einstein operations. Int. J. Intelligent Syst. 26 (11), 1049–1075. doi:10.1002/int.20498
Wei, G., and Lu, M. (2018). Pythagorean fuzzy power aggregation operators in multiple attribute decision making. Int. J. Intelligent Syst. 33 (1), 169–186. doi:10.1002/int.21946
Xiao, F., and Ding, W. (2019). Divergence measure of Pythagorean fuzzy sets and its application in medical diagnosis. Appl. Soft Comput. 79, 254–267. doi:10.1016/j.asoc.2019.03.043
Xu, Z. S. (2007a). Intuitionistic fuzzy aggregation operators. IEEE Trans. Fuzzy Syst. 15, 1179–1187. doi:10.1109/tfuzz.2006.890678
Yager, R. R. (2016). Generalized orthopair fuzzy sets. IEEE Trans. Fuzzy Syst. 25 (5), 1222–1230. doi:10.1109/tfuzz.2016.2604005
Yager, R. R. (2013). Pythagorean membership grades in multi-criteria decision making. IEEE Trans. Fuzzy Syst. 22 (4), 958–965. doi:10.1109/tfuzz.2013.2278989
Zadeh, L. A. (1965). Fuzzy sets. Fuzzy Sets, Inf. Control 8, 338–353. doi:10.1016/s0019-9958(65)90241-x
Zhang, Q., Hu, J., Feng, J., Liu, A., and Li, Y. (2019). New similarity measures of Pythagorean fuzzy sets and their applications. IEEE Access 7, 138192–138202. doi:10.1109/access.2019.2942766
Zhang, X. (2016). A novel approach based on similarity measure for Pythagorean fuzzy multiple criteria group decision making. Int. J. Intelligent Syst. 31 (6), 593–611. doi:10.1002/int.21796
Zhang, X., and Xu, Z. (2014). Extension of TOPSIS to multiple criteria decision making with Pythagorean fuzzy sets. Int. J. Intelligent Syst. 29 (12), 1061–1078. doi:10.1002/int.21676
Zhao, Y., Zhao, C. Y., Markides, C. N., Wang, H., and Li, W. (2020). Medium-and high-temperature latent and thermochemical heat storage using metals and metallic compounds as heat storage media: A technical review. Appl. Energy 280, 115950. doi:10.1016/j.apenergy.2020.115950
Zulqarnain, R. M., Ali, R., Awrejcewicz, J., Siddique, I., Jarad, F., and Iampan, A. (2022). Some Einstein geometric aggregation operators for q-rung orthopair fuzzy soft set with their application in MCDM. IEEE Access 10, 88469–88494. doi:10.1109/access.2022.3199071
Zulqarnain, R. M., Rehman, H. K. U., Awrejcewicz, J., Ali, R., Siddique, I., Jarad, F., et al. (2022). Extension of Einstein average aggregation operators to medical diagnostic approach under q-rung orthopair fuzzy soft set. IEEE Access 10, 87923–87949. doi:10.1109/access.2022.3199069
Zulqarnain, R. M., Siddique, I., Ahmad, S., Iampan, A., Jovanov, G., Vranješ, Đ., et al. (2021). Pythagorean fuzzy soft Einstein ordered weighted average operator in sustainable supplier selection problem. Math. Problems Eng. 2021, 1–16. doi:10.1155/2021/2559979
Zulqarnain, R. M., Siddique, I., Ali, R., Awrejcewicz, J., Karamti, H., Grzelczyk, D., et al. (2022). Einstein ordered weighted aggregation operators for Pythagorean fuzzy hypersoft set with its application to solve MCDM problem. IEEE Access 10, 95294–95320. doi:10.1109/access.2022.3203717
Zulqarnain, R. M., Siddique, I., Ali, R., Jarad, F., and Iampan, A. (2022). Einstein weighted geometric operator for Pythagorean fuzzy hypersoft with its application in material selection. Henderson, NV, United States: CMES-Computer Modeling in Engineering and Sciences. doi:10.32604/cmes.2023.023040
Zulqarnain, R. M., Siddique, I., Ali, R., Pamucar, D., Marinkovic, D., and Bozanic, D. (2021). Robust aggregation operators for intuitionistic fuzzy hypersoft set with their application to solve MCDM problem. Entropy 23 (6), 688. doi:10.3390/e23060688
Zulqarnain, R. M., Siddique, I., and Ei-Morsy, S. (2022). Einstein-ordered weighted geometric operator for Pythagorean fuzzy soft set with its application to solve MAGDM problem. London, United Kingdom: Mathematical Problems in Engineering.
Zulqarnain, R. M., Siddique, I., and Gurmani, S. H. (2022). Extension of interaction aggregation operators for the analysis of cryptocurrency market under q-rung orthopair fuzzy hypersoft set. IEEE Access 10, 126627–126650. doi:10.1109/access.2022.3224050
Zulqarnain, R. M., Siddique, I., Jarad, F., Hamed, Y. S., Abualnaja, K. M., and Iampan, A. (2022). Einstein aggregation operators for Pythagorean fuzzy soft sets with their application in multiattribute group decision-making. J. Funct. Spaces 2022, 1–21. doi:10.1155/2022/1358675
Keywords: q-rung orthopair fuzzy hypersoft set, q-rung orthopair fuzzy hypersoft Einstein-ordered weighted average operator, q-rung orthopair fuzzy hypersoft Einstein-ordered weighted geometric operator, multi-criteria group decision making, thermal energy storage techniques
Citation: Mushtaq I, Siddique I, Eldin SM, Majdoubi J, Gurmani SH, Samar M and Zulqarnain RM (2023) Prioritization of thermal energy storage techniques based on Einstein-ordered aggregation operators of q-rung orthopair fuzzy hypersoft sets. Front. Energy Res. 11:1119463. doi: 10.3389/fenrg.2023.1119463
Received: 12 December 2022; Accepted: 16 January 2023;
Published: 28 March 2023.
Edited by:
Lorenzo Ferrari, University of Pisa, ItalyReviewed by:
Tahir Mahmood, International Islamic University, PakistanRifaqat Ali, King Khalid University, Saudi Arabia
Copyright © 2023 Mushtaq, Siddique, Eldin, Majdoubi, Gurmani, Samar and Zulqarnain. This is an open-access article distributed under the terms of the Creative Commons Attribution License (CC BY). The use, distribution or reproduction in other forums is permitted, provided the original author(s) and the copyright owner(s) are credited and that the original publication in this journal is cited, in accordance with accepted academic practice. No use, distribution or reproduction is permitted which does not comply with these terms.
*Correspondence: Jihen Majdoubi, ai5tYWpkb3ViaUBtdS5lZHUuc2E=; Rana Muhammad Zulqarnain, cmFuYXp1bHFhcm5haW43Nzc3QGdtYWlsLmNvbQ==