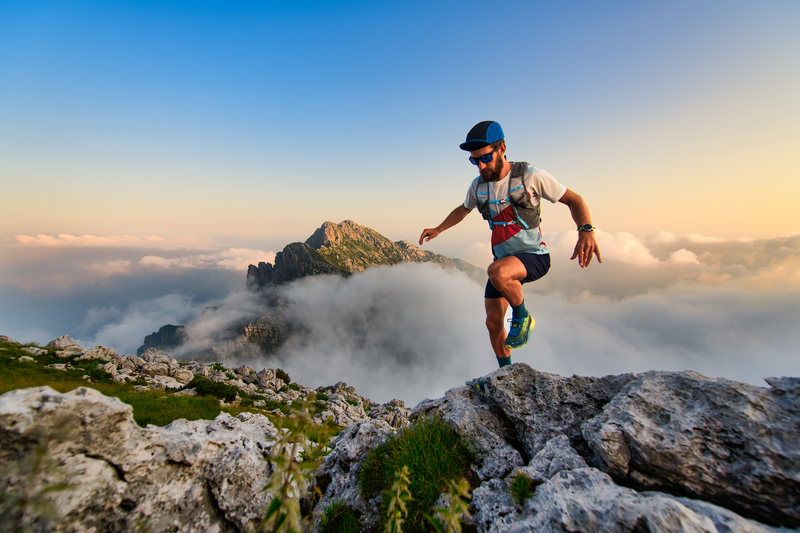
94% of researchers rate our articles as excellent or good
Learn more about the work of our research integrity team to safeguard the quality of each article we publish.
Find out more
ORIGINAL RESEARCH article
Front. Energy Res. , 03 February 2023
Sec. Process and Energy Systems Engineering
Volume 11 - 2023 | https://doi.org/10.3389/fenrg.2023.1113814
This article is part of the Research Topic Machine Learning to Support Low Carbon Energy Transition View all 7 articles
Clean energy utilization is important for the improvement of energy structure. At present, photothermal and electrothermal conversion technologies are becoming increasingly applied in many homes, which can thus be regarded as green residences. To meet the heating demand of green residences, solar hot water systems and electricity-to-heat (E2H) conversion devices, such as ground source heat pumps electric heating stoves and electric water heaters are widely installed to provide a clean form of heat. Besides, common loads, such as lighting, washing, and electric vehicles are daily loads in green residences. The above electric and thermal loads are regarded as multi-energy heterogeneous loads MEHLs can be used to decarbonize green residences by optimizing energy dispatch through flexible control. In this study, a novel energy structure of green residences was extended through the combination of SHWs, E2H, GSHPs, and EVs, as well as rooftop photovoltaic systems. Then, to minimize carbon emissions, a residential energy dispatching model was designed from day-ahead and real-time scales and a low-carbon-oriented multi-energy heterogeneous loads coordinated control strategy was proposed. Finally, to mitigate the residents’ loss of comfort caused by MEHL control, the indoor environment and water tank temperatures and the state of charge of EVs were regarded as special constraints. The simulation revealed that the proposed strategy can reduce carbon emissions by 33.07% and meet the basic demand for residential heat and electricity. Additionally, the strategy has good applicability for decarbonizing green residences.
With global warming becoming an increasingly serious problem, it is urgently important to build a clean, low-carbon, safe, and efficient energy system that utilizes wind, solar, and other renewable energies. In response to this, electric power substitution has been proposed as a great scheme in many regions (Niu et al., 2017; Lv et al., 2018; Zhang et al., 2022) and aims to use electricity to generate heat or cold to meet terminal energy usage. This method can alleviate the environmental pollution caused by coal, oil, and other primary fossil energy. The promotion of “electric power substitution” has effectively contributed to the transformation of clean energy structures and alleviated pressure on the environment. Based on this scheme, not only is the energy consumption structure optimized but flexible load control services are extended. With the help of advanced information technology, the Internet of Things and edge computing technology (Hua et al., 2022a) and residential smart devices, such as EHSs, EWHs, and air conditioners (ACs), can be aggregated, forming an energy pool that can be controlled by various communication methods and contribute to power services through peak shaving and valley filling (Wang et al., 2018; Song et al., 2020; Yan and Zhang, 2021), frequency and voltage regulation (Saxena et al., 2022; Wang et al., 2017; Wang et al., 2019), renewable energy consumption (Li et al., 2021; Cai et al., 2022), and other scenarios. This is widely known as load control technology. For example, Wei et al. (2017) proposed a hierarchical distributed load control strategy based on thermostatically controlled loads (TCLs) in poor communication environments, which can be used to eliminate the fluctuation of renewable energy. Zhang et al. (2022) established a state estimation method and control strategy based on the Fokker Planck model to solve the heterogeneity from TCLs. Kiani et al. (2021), by taking TCLs and EVs as total virtual energy storage, built a unified state space model to evaluate controllable potential from the load population. Additionally, Tao et al. (2022) established an evaluation model based on data-driven methods to assess the controllable potential, and a real-time load control strategy was designed to provide auxiliary service.
An electric power substitution scheme brings more flexibility to power system operation. However, with the increasing number of alternative loads, the demand for power grid capacity is becoming greater. In particular, networks in rural areas struggle to support a large number of alternative electric loads and meet the energy demands of residential users. To solve these problems, clean heating is increasingly being used. In such scenarios, solar is converted directly into heat, and efficient GSHPs are used as a supplementary heat source to generate warm air and hot water for residents. The entire process is clean and low carbon. Clean heating has been applied widely across the world, such as in Germany, Denmark, China, and Austria (Tschopp et al., 2020). Moreover, in China, 21 provinces are required to install SHW systems in residential buildings and utilize photothermal energy (NEA China, 2016).
All the above-mentioned clean heating scenarios coexist in a small residential electrothermal integrated energy system, which can thus be regarded as green residences. This system provides both a thermal and an electric energy service. For thermal demands, SHW systems, E2H conversion devices, and GSHPs are installed, while for the electric demands, common loads, such as lighting, washing, and EV, are regarded as daily loads. The above electric and thermal loads are regarded as MEHLs. To optimize energy dispatch, a great deal of research has focused on load control for improving flexibility and economy. For example, Gao et al. (2021) proposed an incentive demand response strategy for residential users based on evolutionary game theory, which realizes users’ load control when their willingness is time-varying. Wang et al. (2023) put forward an optimal scheduling model based on chance-constrained programming by combining EVs with electrothermal loads. Shao et al. (2019) considered the transferability of load and the alternativity of energy, and a price-based comprehensive demand response strategy was designed for thermoelectric load control. Li Z et al. (2022), Li L et al. (2022) presented a distributed and real-time economic dispatch strategy, in which TCLs are collected to form a virtual and flexibly controlled battery to support the optimal operation of a power system. Additionally, Zheng J et al. (2020), Zheng S et al. (2020) proposed an incentive demand response strategy for both the upregulation and downregulation of multi-energy systems by considering the energy alternative effect and the coupling effect of users’ behaviors. To make use of MEHLs in the special clean heating scenario, some related studies have been carried out. For example, Wang L et al. (2022) conducted multi-level scale-up research of distributed clean building heating, in which electric heat storage is used as a typical MEHL to optimize the operational cost of heating a building. Coen et al. (2021) analyzed and optimized heterogeneous thermal loads as one type of MEHL to reduce heat exhaustion in buildings. These studies show the advantages and applicability of load control technology in improving dispatch economy, but they focus on the optimization of energy supply quantity, and few put forward specific load control actions. The loss of load control actions will result in an inadequate and inaccurate grasp of users’ comfort satisfaction.
Similar to the research above, load control technology is also adopted in clean heating scenarios in this paper. However, two aspects are addressed differently. First, MHEL control technology is extended in this paper (Coen et al., 2021; Sun et al., 2021) and is evolved from load control and involves more loads of different energy types. Second, MHEL control actions are conducted in the optimization to achieve accurate control. Generally, the related research in such MHEL scenarios can be seen in studies by Nordgård-Hansen et al. (2022) and the Ma et al. (2021). Among them, Nordgård-Hansen et al. (2022) established an investment and operation model for RPV and GSHP systems in a single house in Norway. This heating scenario is similar to that in our study, but the load control actions are not involved. Ma et al. (2021) combined EVs, electric heating, and cooling loads, and then designed a load dispatching strategy by minimizing the comprehensive operating cost, which consists of electricity cost, gas cost, and carbon emissions. However, the dispatching time scale is 1 h. The residents’ cooling and heating levels may exceed the endurance limits within 1 h. To coordinate energy dispatch and residents’ comfort, a novel low-carbon-oriented multi-time-scale MHEL control strategy is proposed in this study, which contains the following contributions.
1) The energy structure described by Nordgård-Hansen et al. (2022) is extended by installing solar accumulators and GSHPs, which lead to a more complex and energy-coupled scenario, and the residential energy use pattern is more flexible with the participation of EVs.
2) A joint DA and RT energy dispatching strategy is proposed, in which a stochastic scheduling model is established and the expected carbon emission cost is minimized in different and uncertain scenarios.
3) The multi-time-scale dispatching strategy is built in the coordinated control of MEHLs in the warm house system, where the RT indoor temperature status and EV’s SOC are considered to avoid dissatisfaction from residents.
4) The solving method of the dispatching strategy is novel in that it transfers the recursive temperature and SOC constraints into approximate linear constraints, and the original constraints are taken as the verification to analyze the reasonability of the proposed dispatching strategy.
The structure of the article is as follows. The section entitled “System Model of a Green Residence Equipped with MEHLs” depicts the extended structure of MEHLs in an independent green residential house and their basic models. The subsequent section “Low-Carbon-Oriented MEHL Dispatch” presents the multi-time-scale load dispatching strategy with the coordinated control of MEHLs. Finally, simulation results and conclusions are developed in the last two sections.
Most residents require heat and electricity, so an integrated energy system is needed to meet these demands. To make the heating process clean and economic, a house energy system equipped with photothermal, electrothermal, and photovoltaic devices and a GSHP and an EV was designed and is shown in Figure 1. This system can not only bring about clean heating, but also increase the flexibility of the house energy system.
In the proposed system structure, the energy supply was mainly provided by solar accumulators, RPV systems, and the external power grid, while the heat demands included air heating and hot water. For indoor air heating, the GSHP system was adopted, which can generate lots of heat from small amounts of electricity. Besides, the EHS was equipped to realize electricity-to-heat (E2H) conversion and meet the air heating demand. For the hot water, the solar accumulators were connected to the water system to form the SHW system to provide hot water on sunny days preferentially. Additionally, an EWH system was installed to provide hot water when the sun was not shining.
The proposed structure was grid friendly as the energy system was directly connected to the external power grid. As both solar-thermal and solar-electric conversion efficiency are limited in cloudy and rainy weather, it is necessary to purchase electricity from the external power grid. On the contrary, when the RPV output exceeds the demand of electricity and E2H conversion, the house owner can sell the excess electricity to the power grid. Apart from the power interaction, flexibly controlled MEHLs can optimize the operation of the house system and the external power system. With the improvement of intelligence and the information level, the hot water load, heating load, and the EV can be used as flexible loads. When the power supply is tight, the usage time and practical operation power of these loads can be adjusted flexibly, contributing to peak shaving, frequency stability, and other auxiliary services of the power grid.
In this study, we mainly attempt low-carbon energy dispatching. From the structure shown in Figure 1, carbon emissions were mainly generated by the gas combustion from the external power grid. Therefore, the residents should use energy from solar accumulators and RPV systems as much as possible and optimize the operation process of MEHLs to reduce carbon emissions in the house system. This process is usually performed by home energy management systems (EMS). The default in this study was that the green residence was equipped with an EMS, which was responsible for communication, remote control, load monitoring, etc.
A GSHP is a high-efficient E2H device that uses little electricity to provide lots of heat. Generally, the coefficient of performance (COP) is an effective index to measure electricity consumption and heat supply. The physical power model of a GSHP can be depicted as follows (Marmaras et al., 2016):
where
Besides the GSHP, thermostatically controlled load is another type of E2H equipment, including EWHs, EHSs, ACs, and so on (Peirelinck et al., 2021; Wang C et al., 2022; Huang et al., 2019). They have resistivity characteristics when heating. The electric power and thermal power of the E2H conversion process should meet a certain proportion, which can be briefly described as:
where
The SHW system directly converts solar energy into heat. The output is mainly affected by the heat collection efficiency, plate area, and solar radiation intensity. Its physical model can be described as (Li et al., 2023):
where
RPV systems are a type of common distributed power unit, and their power generation can be described as follows (Soto et al., 2006):
where
The objective function for the operation of green residences is minimizing carbon emissions. Generally, carbon emissions are mainly caused by the burning of fossil fuels. In the proposed energy structure, the energy of a green residence is primarily provided by solar. The photoelectrical and photothermal conversion based on solar energy is clean and zero carbon. Therefore, if the house is energy self-sufficient, carbon emission will not occur. However, when the energy from solar cannot meet the energy demand, residents need to purchase electricity from the external power grid. It is supposed that the power from the external power grid is generated by gas turbines, which is the main cause of carbon emissions. Therefore, the carbon emission is strongly dependent on the generation of gas turbines.
Normally, a quadratic function is used to describe the relationship of the operation cost and the power generation of gas turbines (He et al., 2023). Therefore, the cost of carbon emission is also quadratically dependent on the power generation of gas turbines, as the amount of carbon emission is linearly dependent on the consumption of gas. As such, if the residents purchase electricity/power from the power grid, the cost of the carbon emission can be calculated by quadratic functions. In DA and RT stages, it is inevitable that residents will purchase or sell electricity to maintain the power balance. As a result, the objective function should contain two-time scales. Meanwhile, considering that inaccurate power forecasts will affect the calculation of carbon emissions, a stochastic scheduling method based on a scenario tree is adopted to reduce the uncertainty of prediction. Therefore, the objective function can be described as follows:
where
Generally, the relationship between heat load and temperature in a house can be described by the ETP model (Hu et al., 2017), as shown in Eq. 10. Considering the various heating sources in the proposed structure, the total thermal power meets Eq. 11.
In Eqs 10, 11,
where
The thermal storage of the hot water tank is similar to that of the heating system in a house, so the ETP model is also adopted in the hot water system. However, the parameters of the model are different. Its expression is as follows:
In Eqs 13–15,
The SOC evolution process of EVs can be described as follows (Li Z et al., 2022):
where
where
The COP of a GSHP in operating conditions should not exceed the rated COP (Nordgård-Hansen et al., 2022), which is affected by the temperature of borehole heat exchangers (BHEs) and surrounding soil, so that the operating COP should meet the following constraints:
where
Similar to the energy constraints in energy management scenarios (Hua et al., 2019a; Deng et al., 2023), supply-demand balance is the basic rule for optimization. Both the balance of electric power supply and demand in multi-time scales and that of the thermal power should be met in this system. For electric power, balance constraints are as follows:
For thermal power, balance constraints are as follows:
where the superscripts
In the proposed house system, the electric power is partly provided by the RPV and partly provided by external power grid. Here, each variable regarding electric power consumption is divided into two independent variables to make the energy flow clearer. Therefore, the following energy flow constraints should be met in the optimization:
where the meanings of
In the proposed strategy, the optimization in the RT stages is based on that in the DA stages, i.e., the RT optimization is an adjustment according to DA results. So, for the variables
where
As mentioned above, the proposed strategy contributes to the low-carbon operation of the house system by controlling MEHLs. As residents’ air heating, water heating, and EV charging process will change, temperature constraints and EV charging constraints need to be involved, which are as follows:
For the indoor air heating demand, it is necessary to ensure that the temperature is within the range of the user’s satisfaction, considering that an environment that is too hot or too cold is not suitable for living. The temperature of the house should meet the constraint in Eq. 37. For the hot water demand, there is also a requirement that the temperature is tolerable, and this is shown in the constraint (Eq. 38).
EV needs to be charged to the expected SOC before leaving, shown as:
where
Eqs 1–39 show that the model is non-linear, which presents two difficulties that need to be solved. First, in the GSHP model, the
where
Second, the relationship between temperature and thermal power in the water heating and air heating process are non-linear, which is shown in Eqs 10, 12, 37 for house heating and Eqs 13, 15, 38 for water heating. It is difficult to solve these temperature constraints with the objective function directly because of the non-linearity. To solve the problem, the predictions for air heating load and water heating load based on historical temperature data are made and used as thermal demands in the optimization periods. Then, the temperature constraints can transfer into approximate thermal power constraints, as follows:
where
Finally, when the optimized results are obtained based on the modified constraints in Eqs 40–42, the temperature constraints (10), (12), (37), (13), (15), and (38) will be the verification condition for the results. If the results are not feasible, then the coefficients
To verify the advantages of the proposed strategy, two cases, with and without the proposed strategy, were set to make a thorough comparison in terms of external electricity consumption, carbon emission, house system operation, and residents’ comfort. The two cases are shown in Table 1.
The Monte Carlo method was used to simulate the heating process both in the house and in the water tank. Based on the historical temperature data, which are easily visible in the EMS system, the indoor air heating and hot water demands were calculated according to the temperature data. Additionally, the EV charging process was simulated and the charging demand in each period was predicted. The thermal power and electric power demands are shown in Figure 3, in which QH represents the thermal power used for air heating, QW represents thermal power used for water heating, and EV represents the charging power.
The curves of solar thermal output used for SHW and PV, as well as the common load in the DA stages, are depicted in Figure 4. In a shorter time scale, random noises were also added to simulate the uncertainty from DA and RT prediction.
The proposed strategy involves various parameters, such as carbon emission cost coefficients, MEHL rated values, and controllable coefficients and initialization of MEHLs. Their settings can be seen in Table 2.
As the carbon emission of a single house is relatively small, the proposed strategy rarely generates a profit. However, in the case of the large-scale promotion of MEHLs, the benefits are very optimistic. In this section, 100,000 distributed houses with MEHLs were set and aggregated into a flexible and controllable group to show the benefits afforded by large-scale promotion. Table 3 shows that the proposed strategy can bring large economic benefits in terms of carbon emission cost.
By comparing the carbon emission cost in Case 1 and Case 2, the proposed strategy can save up to 33.07% of carbon emission cost, whether it be a single house or a house group. As such, the proposed strategy can greatly reduce the carbon emissions of the house system with MEHLs and promote clean and low-carbon operation.
The carbon emission cost distribution in the DA periods is described in Figure 5. As observed in Case 1, the highest carbon emission occurred from 8 a.m. to 2 p.m., as did the highest electricity purchase, as EV charging demand is large and relatively concentrated during this period. Normally, the EV starts to charge upon arrival and the charging process is undisturbed. Therefore, in such concentrated charging periods, the house system needs to buy large amounts of electricity from external thermal power plants, resulting in a concentrated distribution of high carbon emission cost. However, in our proposed strategy, i.e., in Case 2, from 8 a.m. to 2 p.m., the electricity purchase and the carbon emission cost were reduced after optimization. Although from 2 p.m. to 7 p.m., the carbon emission cost was higher than that in Case 1, the cost of the full optimization period is obviously lower, which is a great advantage of our strategy.
In other periods, i.e., from midnight to 7.30 a.m. and from 7 p.m. to midnight, the output of the RPV system was relatively low, and most of the energy for hot water and air heating was obtained from the external power grid. To reduce the carbon emission cost of the system, hot water load and heating load were downregulated slightly in Case 2. This means that the proposed strategy can use MEHLs for a lower-carbon optimization and reduce carbon emission costs.
Correspondingly, Figure 6 depicts the incremental carbon emission cost distribution in RT periods compared with the costs of the DA periods. The incremental costs were mainly caused by the prediction errors of photoelectricity, photothermal energy, and residential load. Figure 6 shows that, when there were deviations and uncertainties in RT periods, the carbon emission cost distribution fluctuated less and was more stable in Case 2. In our strategy, the fluctuating outputs of the RPV system and photothermal energy were considered, and MEHLs in the house could match such outputs through flexible control. However, in Case 1, these deviations between the DA and RT periods could only be eliminated through external power purchases. Therefore, the fluctuation range of carbon emission costs at different times was large and the total emission cost was high.
The DA dispatching results of MEHLs in the house system in two cases are compared in Figure 7. The top two panels show that, in Case 2, the operation power of the GSHP is higher than that in Case 1 from 1 p.m. to 4 p.m., while the operation power of the EHS is lower. In the proposed strategy, air heating demand was met by the GSHP, and the electric power of the GSHP was downregulated during most of the optimization periods due to the flexible and moderate control of indoor heating load.
Besides the air heating load supplied by the GSHP and EHS, control of the MHELs in the proposed strategy was also applied to the hot water load and EV. For hot water, shown in the third figure panel, when the photothermal energy was low, the heat supply for hot water load was moderately downregulated. Thus, the EWH, as the supplier in low-photothermal conditions, consumed less electric power, whereas in high-photothermal conditions, the hot water load was met by the SHW system rather than the EWH in our strategy. For the EV, shown in the figure panel at the bottom, the charging power from 8 a.m. to 12 p.m. was downregulated while the charging time was extended and the charging power after 12 p.m. was upregulated to consume more photoelectricity.
In the RT periods, the dispatching and operation of the MEHLs showed a similar trend as that in the DA periods, as shown in Figure 8. Compared with Case 1, the main changes in Case 2 can be summarized with respect to the following three aspects. First, the power of EHS was reduced and the GSHP was fully used to meet air heating demand within adjustable ranges. Second, photothermal energy was fully utilized at noon to increase hot water temperature, and then the heat supply for water in other periods was adjusted within acceptable ranges. Finally, the EV was charged with more flexible power during the entirety of the high-photoelectric periods.
The proposed strategy could meet the electric and thermal demand shown in Figure 3. As shown in Figures 7, 8 above, EV charging was not concentrated between 8 a.m. and 12 p.m. but was completed within a longer time period. Figure 9 depicts the heat supply for air heating and hot water in our strategy. Figure 9 shows that the heat supply for air heating during each period was downregulated, but the total supply was within an acceptable range. The heat supply for hot water in high-photothermal periods exceeded the original demand, and in these periods, more hot water was stored in the tank to maintain a higher temperature over a longer time scale in case the heat supply was downregulated in low-photothermal periods. Therefore, although the energy supply was adjusted after MEHL control, residents’ energy demand could still be met.
Actually, residents’ comfort depends on the temperature when it comes to hot water and air heating load, and also depends on the EV’s SOC when leaving. Therefore, the temperature of hot water, indoor air, and the final SOC were the comfort indexes and were compared in two cases, which are shown in Figure 10. As shown in uppermost figure panel, the indoor temperature rose relatively slowly compared with that in Case 1, but it stayed within the threshold range of deviation acceptable to residents. Meanwhile, in the middle panel, the water temperature fluctuated around the lower limit of deviation acceptable to residents before 8 a.m. then rose to the preset range and dropped at night; these fluctuations are strongly influenced by photothermal energy during the full optimization cycle. Finally, the bottom figure shows that, although the charging time increased, the SOC rose to 100% before the user left. Therefore, the user’s travel demand could be met and no discomfort would generate.
Therefore, the proposed low-carbon-oriented MEHL coordinated control strategy not only has strong advantages in terms of carbon emission costs but also ensures living and traveling standards that residents are satisfied with. It shows reliable supportability in the supply of hot water, indoor air heating, and EV charging when MEHLs are controlled flexibly.
In this study, an MEHL coordinated control strategy was proposed to optimize the house energy system with GSHPs and solar. Low-carbon emission is regarded as the optimization target, and a multi-time-scale house energy dispatching model was established and coordinated with MEHL control. The proposed strategy was applied to a house system consisting of photothermal and photoelectric energy. The simulation results of two cases showed that the proposed strategy can reduce carbon emissions and meet residents’ energy demand when MEHLs are controlled. The main conclusion can be highlighted as follows:
The proposed strategy can reduce carbon emissions by up to 33.07%. The saving is made through the reduction of external electricity, and inner-system photoelectric and photothermal energy is fully used and optimized by MEHL control over longer time periods.
In the proposed strategy, MEHL control changes the temperature distribution of the water tank and the indoor environment, but the deviation of temperature will not significantly affect residents’ living comfort. Similarly, EV charging status changes but will not affect the traveling satisfaction of users.
Future research will focus on the data-driven technology application in our scenarios. Owing to the non-linear and recursive characteristics of the original model, the data-driven methods described by Tao et al. (2022), Hua et al. (2019a), Hua et al. (2019b) and Hua et al. (2022b) are good references for solving such problems.
The original contributions presented in the study are included in the article/Supplementary Material, further inquiries can be directed to the corresponding author.
ZL–model research, YS–scenarios research, JL–solution, KX–model research, SL–simulation, YS–writting and checking.
This work was supported by Science and Technology Projects from the State Grid Corporation (SGJSYF00LJJS2200006).
The authors declare that the research was conducted in the absence of any commercial or financial relationships that could be construed as a potential conflict of interest.
The handling editor HH declared a past co-authorship with the reviewer DL.
All claims expressed in this article are solely those of the authors and do not necessarily represent those of their affiliated organizations, or those of the publisher, the editors and the reviewers. Any product that may be evaluated in this article, or claim that may be made by its manufacturer, is not guaranteed or endorsed by the publisher.
Cai, Q., Xu, Q., Qing, J., Shi, G., and Liang, Q. (2022). Promoting wind and photovoltaics renewable energy integration through demand response: Dynamic pricing mechanism design and economic analysis for smart residential communities. Energy 261, 125293. Part B. doi:10.1016/j.energy.2022.125293
Coen, T., François, B., and Gerard, P. (2021). Analytical solution for multi-borehole heat exchangers field including discontinuous and heterogeneous heat loads. Energy Build. 253, 111520. doi:10.1016/j.enbuild.2021.111520
Deng, Y., Mu, Y., Wang, X., Jin, S., He, K., Jia, H., et al. (2023). Two-stage residential community energy management utilizing EVs and household load flexibility under grid outage event. Energy Rep. 9, 337–344. doi:10.1016/j.egyr.2022.10.414
Gao, B., Chen, C., Qin, Y., Liu, X., and Zhu, Z. (2021). Evolutionary game-theoretic analysis for residential users considering integrated demand response. J. Mod. Power Syst. Clean Energy 9 (6), 1500–1509. doi:10.35833/MPCE.2019.000030
He, Y., Li, Z., Zhang, J., Shi, G., and Cao, W. (2023). Day-ahead and intraday multi-time scale microgrid scheduling based on light robustness and MPC. Int. J. Electr. Power Energy Syst. 144, 108546. doi:10.1016/j.ijepes.2022.108546
Hu, J., Cao, J., Chen, M., Jie, Y., Yong, T., Yang, S., et al. (2017). Load following of multiple heterogeneous tcl aggregators by centralized control. IEEE Trans. Power Syst. 32 (4), 3157–3167. doi:10.1109/TPWRS.2016.2626315
Hua, H., Li, Y., Wang, T., Li, W., Dong, N., and Cao, J. (2022a). Edge computing with artificial intelligence: A machine learning perspective. ACM Computing Surveys [preprint] Available at: https://dl.acm.org/doi/10.1145/3555802 (Accessed August 16, 2022).
Hua, H., Qin, Y., Hao, C., and Cao, J. (2019b). Optimal energy management strategies for energy Internet via deep reinforcement learning approach. Appl. Energy 239, 598–609. doi:10.1016/j.apenergy.2019.01.145
Hua, H., Qin, Y., Hao, C., and Cao, J. (2019a). Stochastic optimal control for energy Internet: A bottom-up energy management approach. IEEE Trans. Industrial Inf. 15 (3), 1788–1797. doi:10.1109/TII.2018.2867373
Hua, H., Qin, Z., Dong, N., Qin, Y., Ye, M., Wang, Z., et al. (2022b). Data-driven dynamical control for bottom-up energy Internet system. IEEE Trans. Sustain. Energy 13 (1), 315–327. doi:10.1109/TSTE.2021.3110294
Huang, T., Sun, Y., Li, B., and Hao, J. (2019). A collaborative fuzzy control strategy of aggregated regenerative electric heaters for real-time energy balance in micro-grid. IEEE Access 7, 79388–79400. doi:10.1109/ACCESS.2019.2916072
Kiani, S., Sheshyekani, K., and Dagdougui, H. (2021). A unified state space model for aggregation and coordination of large-scale TCLs and EVs for frequency regulation. Electr. Power Syst. Res. 195, 107181. doi:10.1016/j.epsr.2021.107181
Li, J., Wei, S., Dong, Y., Liu, X., and Novakovic, V. (2023). Technical and economic performance study on winter heating system of air source heat pump assisted solar evacuated tube water heater. Appl. Therm. Eng. 221, 119851. doi:10.1016/j.applthermaleng.2022.119851
Li, L., Dong, M., Song, D., Yang, J., and Wang, Q. (2022). Distributed and real-time economic dispatch strategy for an islanded microgrid with fair participation of thermostatically controlled loads. Energy 261, 125294. Part B. doi:10.1016/j.energy.2022.125294
Li, Z., Sun, Y., and Anvari-Moghaddam, A. (2021). “A consumer-oriented incentive mechanism for EVs charging in multi-microgrids based on price information sharing,” in Proceeding of the IEEE International Conference on Environment and Electrical Engineering and 2021 IEEE Industrial and Commercial Power Systems Europe (EEEIC/I&CPS Europe), 1–6. doi:10.1109/EEEIC/ICPSEurope51590.2021.9584651
Li, Z., Sun, Y., Yang, H., and Anvari-Moghaddam, A. (2022). A consumer-oriented incentive strategy for EV charging in multiareas under stochastic risk-constrained scheduling framework. IEEE Trans. Industry Appl. 58 (4), 5262–5274. doi:10.1109/TIA.2022.3174527
Lv, J., Zhou, L., Zhang, S., Liu, S., Si, L., and Ye, F. (2018). “Design and practice of trading mode promoting clean energy wide-scale consumption based on electric power alternative,” in Proceedings of 2018 4th International Conference on Green Materials and Environmental Engineering (GMEE 2018), 383–389. doi:10.12783/dteees/gmee2018/27541
Ma, T., Pei, W., Xiao, H., Zhang, G., and Ma, S. (2021). “The energy management strategies of residential integrated energy system considering integrated demand response,” in Proceeding of the IEEE/IAS Industrial and Commercial Power System Asia (I&CPS Asia), 188–193. doi:10.1109/ICPSAsia52756.2021.9621462
Marmaras, J., Burbank, J., and Kosanovic, D. (2016). Primary-secondary de-coupled ground source heat pump systems coefficient of performance optimization through entering water temperature control. Appl. Therm. Eng. 96, 107–116. doi:10.1016/j.applthermaleng.2015.10.027
NEA China (2016). The 13th Five-Year Plan for development of solar energy. Available online at: http://zfxxgk.nea.gov.cn/auto87/201612/t20161216_2358.htm (Accessed December 8, 2016).
Niu, D., Song, Z., and Xiao, X. (2017). Electric power substitution for coal in China: Status quo and SWOT analysis. Renew. Sustain. Energy Rev. 70, 610–622. doi:10.1016/j.rser.2016.12.092
Nordgård-Hansen, E., Kishor, N., Midttømme, K., Kjær Risinggård, V., and Kocbach, J. (2022). Case study on optimal design and operation of detached house energy system: Solar, battery, and ground source heat pump. Appl. Energy 308, 118370. doi:10.1016/j.apenergy.2021.118370
Peirelinck, T., Hermans, C., Spiessens, F., and Deconinck, G. (2021). Domain randomization for demand response of an electric water heater. IEEE Trans. Smart Grid 12 (2), 1370–1379. doi:10.1109/TSG.2020.3024656
Saxena, A., Kumar, S., Shankar, R., and Parida, S. K. (2022). “Demand response strategy in a multi-microgrid integrating renewable sources for improved frequency regulation,” in Proceeding of the 2022 IEEE IAS Global Conference on Emerging Technologies (GlobConET), 77–83. doi:10.1109/GlobConET53749.2022.9872339
Shao, C., Ding, Y., Siano, P., and Lin, Z. (2019). A framework for incorporating demand response of smart buildings into the integrated heat and electricity energy system. IEEE Trans. Industrial Electron. 66 (2), 1465–1475. doi:10.1109/TIE.2017.2784393
Song, M., Sun, W., Shahidehpour, M., Yan, M., and Gao, C. (2020). Multi-time scale coordinated control and scheduling of inverter-based TCLs with variable wind generation. IEEE Trans. Sustain. Energy 12 (1), 46–57. doi:10.1109/TSTE.2020.2971271
Soto, W., Klein, S., and Beckman, W. (2006). Improvement and validation of a model for photovoltaic array performance. Sol. Energy 80 (1), 78–88. doi:10.1016/j.solener.2005.06.010
Sun, Y., Li, Z., Bao, H., Liu, C., and Pang, P. (2021). Analysis of multi energy heterogeneous load regulation framework and key technologies under clean heating mode. Proc. Chin. Soc. Electr. Eng. 41 (20), 6827–6842. (in Chinese). doi:10.13334/j.0258-8013.pcsee.210798
Tao, Y., Qiu, J., and Lai, S. (2022). A data-driven management strategy of electric vehicles and thermostatically controlled loads based on modified generative adversarial network. IEEE Trans. Transp. Electrification 8 (1), 1430–1444. doi:10.1109/tte.2021.3109671
Tschopp, D., Tian, Z., Berberich, M., Fan, J., Perers, B., and Furbo, S. (2020). Large-scale solar thermal systems in leading countries: A review and comparative study of Denmark, China, Germany and Austria. Appl. Energy 270, 114997. doi:10.1016/j.apenergy.2020.114997
Wang, C., Wang, B., Cui, M., and Wei, F. (2022). Cooling seasonal performance of inverter air conditioner using model prediction control for demand response. Energy Build. 256, 111708. doi:10.1016/j.enbuild.2021.111708
Wang, H., Xing, H., Luo, Y., and Zhang, W. (2023). Optimal scheduling of micro-energy grid with integrated demand response based on chance-constrained programming. Int. J. Electr. Power Energy Syst. 144, 108602. doi:10.1016/j.ijepes.2022.108602
Wang, J., Zhang, H., and Zhou, Y. (2017). Intelligent under frequency and under voltage load shedding method based on the active participation of smart appliances. IEEE Trans. Smart Grid 8 (1), 353–361. doi:10.1109/TSG.2016.2582902
Wang, L., Guo, L., Ren, J., and Kong, X. (2022). Using of heat thermal storage of PCM and solar energy for distributed clean building heating: A multi-level scale-up research. Appl. Energy 321, 119345. doi:10.1016/j.apenergy.2022.119345
Wang, M., Mu, Y., Jiang, T., Jia, H., Li, X., Hou, K., et al. (2018). Load curve smoothing strategy based on unified state model of different demand side resources. J. Mod. Power Syst. Clean Energy 6 (3), 540–554. doi:10.1007/s40565-017-0358-0
Wang, M., Mu, Y., Li, F., Jia, H., Li, X., Shi, Q., et al. (2019). State space model of aggregated electric vehicles for frequency regulation. IEEE Trans. Smart Grid 1 (2), 981–994. doi:10.1109/TSG.2019.2929052
Wei, W., Wang, D., Jia, H., Wang, C., Zhang, Y., and Fan, M. (2017). Hierarchical and distributed demand response control strategy for thermostatically controlled appliances in smart grid. J. Mod. Power Syst. Clean Energy 5 (1), 30–42. doi:10.1007/s40565-016-0255-y
Yan, Y., and Zhang, Z. (2021). “Peak shaving potential of residential areas considering energy consumption characteristics,” in Proceeding of the 2021 IEEE 4th International Electrical and Energy Conference (CIEEC), 1–6. doi:10.1109/CIEEC50170.2021.9510894
Zhang, Z., Liu, H., Wang, C., and Deng, H. (2022). “Impact of electric heating technology on power grid of city,” in Proceedings of 2022 China International Conference on Electricity Distribution (CICED), 556–559. doi:10.1109/CICED56215.2022.9929120
Zheng, J., Laparra, G., Zhu, G., and Li, M. (2020). Aggregate power control of heterogeneous tcl populations governed by fokker–planck equations. IEEE Trans. Control Syst. Technol. 28 (5), 1915–1927. doi:10.1109/TCST.2020.2968873
Keywords: multi-energy heterogeneous loads (MEHL), low-carbon, electrothermal energy, residential energy dispatching, clean heating
Citation: Li Z, Sun Y, Li J, Xiong K, Liang S and Shen Y (2023) A low-carbon-oriented multi-time-scale dispatching strategy of multi-energy heterogeneous loads in clean heating scenarios of green residences. Front. Energy Res. 11:1113814. doi: 10.3389/fenrg.2023.1113814
Received: 01 December 2022; Accepted: 18 January 2023;
Published: 03 February 2023.
Edited by:
Haochen Hua, Hohai University, ChinaCopyright © 2023 Li, Sun, Li, Xiong, Liang and Shen. This is an open-access article distributed under the terms of the Creative Commons Attribution License (CC BY). The use, distribution or reproduction in other forums is permitted, provided the original author(s) and the copyright owner(s) are credited and that the original publication in this journal is cited, in accordance with accepted academic practice. No use, distribution or reproduction is permitted which does not comply with these terms.
*Correspondence: Yaqi Shen, anNudHN5cTE5OTRAMTYzLmNvbQ==
Disclaimer: All claims expressed in this article are solely those of the authors and do not necessarily represent those of their affiliated organizations, or those of the publisher, the editors and the reviewers. Any product that may be evaluated in this article or claim that may be made by its manufacturer is not guaranteed or endorsed by the publisher.
Research integrity at Frontiers
Learn more about the work of our research integrity team to safeguard the quality of each article we publish.