- Jožef Stefan Institute, Ljubljana, Slovenia
Previous work done on reactor kinetics and control in load-following operation modes available in open literature is reviewed. The analysis is focused on, however not limited to pressurized water reactors. Different approximations of the time-dependent neutron transport problem as well as different control algorithms are described in detail and compared. Due to lack of published information the majority of the comparisons was done on qualitative level. In order to facilitate future testing and intercomparisons of models and algorithms, two so-called reference scenarios with time-dependent power demand are defined: a scenario to test the limitations of the load-following capabilities of the nuclear facilities and a second, quasi-realistic scenario.
1 Introduction
To generate carbon-free electricity, increased use of renewable energy sources (wind, solar, hydro, etc.) and nuclear power is expected. Due to the fluctuations in electricity generation, influences by external factors such as weather conditions, from renewable energy sources such as solar and wind, an additional source of electricity is needed that can compensate for these fluctuations quickly enough. When the operation of a power plant responds directly to the changing demand for energy supply, it operates in what is known as load-following mode (OECD, 2011). In some parts of the world, e.g., in Germany, the load-following became important in recent years due to introduction of a large share of intermittent sources in the total electricity generation. Additionally, elsewhere, e.g., in France, the share of nuclear power in the electricity production became so important that utilities had to introduce or improve the manoeuvrability of their nuclear power plants (NPPs) in order to be able to adapt the power supply to daily or seasonal variations in electricity demand. An excellent guidance addressing all relevant aspects of non-base-load operation of nuclear power plants is given in (IAEA, 2018).
During load-following operation in nuclear power plants, several qualitatively different effects come into consideration resulting from variations of the reactor power. As a direct consequence, the fuel and moderator temperature profiles change as a function of thermal power, as well as the concentration of the strong neutron absorber nuclide 135Xe and its decay precursor 135I, each with a different characteristic time delay. Indirectly, the power distribution is axially shifted due to feedback effects. These changes must be adequately compensated for so that both the reactor core remains critical and the power distribution remains acceptable. The traditional approach in pressurised water reactors (PWRs) is to compensate for the change in reactivity due to power variations by adjusting the concentration of the soluble boron in water and by moving the control rod banks (Franceschini and Petrovic, 2008).
Compensation of the reactivity by adjusting the boron concentration may lead to increased amounts of radioactive liquid effluent1. On the other hand, compensation by control rods may lead to oscillations in 135Xe concentration with respect to both space and time variables. Whereas it is straightforward to control the reactor power, controlling axial power distribution is a complex process. In addition, predictive software is needed to support the load following, warning the reactor operator in advance of potential violations of operating limits and conditions and suggesting appropriate actions to mitigate the consequences, such as the oscillations in the 135Xe concentration. Such phenomena can significantly affect the ability of the NPP to operate in load-following mode and impose limitations on power and reactivity transients that the NPP can perform. Due to the above phenomena, load following operation also has long term effects on fuel utilisation, operating cycle length, radioactive waste production, and control rod depletion. Therefore, utilisation of both control rods and soluble boron could be optimal. To assess the limitations of individual NPPs in performing load-following manoeuvres, to evaluate the impact of load-following manoeuvres and to develop the best scenarios to mitigate the problems caused by load-following manoeuvres, it is necessary to rely on computer codes performing real-time and on-line calculations of nuclear reactor core parameters.
In the past, load following capabilities of nuclear reactors have been studied in numerous references, which are reviewed in the main part of this paper. They mostly rely on single point kinetics (Aboanber et al., 2014; Arab-Alibeik and Setayeshi, 2005; Ben-Abdennour et al., 1992; Edwards et al., 1990; Elsisi and Abdelfattah, 2020; Hui et al., 2020; Hui and Yuan, 2021; 2022c; d; Khajavi et al., 2002; Khorramabadi et al., 2008; Li and Zhao, 2013a; b, 2014; Liu and Wang, 2014; Mousakazemi, 2019; Nair and Gopal, 1987; Park and Cho, 1992; Torabi et al., 2011; Wang et al., 2019; Zarei et al., 2016) or multi-point kinetics (Ansarifar and Saadatzi, 2015; Eliasi et al., 2011; Hui and Yuan, 2022a; b; Kobayashi and Yoshikuni, 1982; Li, 2014a; b; Li et al., 2014b; Marseguerra et al., 2003; Na et al., 1998b; a; Na, 2001; Onega and Kisner, 1978; Parhizkari et al., 2015; Pradhan et al., 2016; Saadatzi and Ansarifar, 2017; Shimazu, 1995; Winokur and Tepper, 1984; Yadav et al., 2018) or on 1D time-dependent diffusion codes (Christie and Poncelet, 1973; Bauer and Poncelet, 1974; Winokur et al., 1979; Teachman and Onega, 1983; Yoon and No, 1985; Yim and Christenson, 1992; Alten and Danofsky, 1993; Trkov and Ravnik, 1994; Song et al., 1999; Domingos et al., 2003; Ćalić et al., 2016; Kastin et al., 2019), which are sufficient for scoping purposes and for study of general effects and qualitative prediction and description of oscillations of the concentration of the neutron absorber 135Xe. They rely on the calculation of indirect parameters such as the power axial offset or power tilt, but cannot be used for precise calculations of axial and radial power peaking calculations, fuel depletion effects, control rod depletion, cycle length perturbations, etc.
A wide variety of control methods is used, including linear methods like proportional-integral-derivative (PID) control (Mousakazemi, 2019), linear-quadratic-Gaussian control (Edwards et al., 1990; Ben-Abdennour et al., 1992; Li et al., 2014a), minimum-variance control (Na et al., 1998b), model predictive control (receding horizon control) (Na, 2001; Wang et al., 2017), robust control (Torabi et al., 2011), non-linear methods like global linearising control (Park and Cho, 1992), feedback linearization control (Zaidabadi nejad and Ansarifar, 2018; Naimi et al., 2022b), non-linear dynamic inversion (Yadav et al., 2018), non-linear model predictive control (Eliasi et al., 2011; Liu et al., 2016; Ejigu and Liu, 2022), sliding mode control (variable structure control) (Ansarifar and Saadatzi, 2015; Wang et al., 2019; Elsisi and Abdelfattah, 2020; Hui and Yuan, 2022b), control based on artificial neural networks (Na et al., 1998a; Lin and Shen, 2000; Khajavi et al., 2002; Boroushaki et al., 2004; Arab-Alibeik and Setayeshi, 2005; Khorramabadi et al., 2008; Naimi et al., 2022b; Hui and Yuan, 2022d) and/or fuzzy logic (Na et al., 1998a; Khorramabadi et al., 2008; Liu et al., 2016; Zeng et al., 2021), deep learning and artificial intelligence (Lee et al., 2020; 2022; Ejigu and Liu, 2022; Park et al., 2022), fault-tolerant control (Hui and Yuan, 2022d), control based on models with distributed parameters (Winokur et al., 1979; Cho and Grossman, 1983; Ukai et al., 1990; Ye and Turinsky, 1998). While the majority of the publications on PWR control methods is of academic nature, some experimental studies report practical experience with load-following operation of nuclear power plants or discuss the control methods used in practice (Sipush et al., 1976; Meyer et al., 1978; Onoue et al., 2003; Franceschini and Petrovic, 2008; Wei and Zhao, 2015; Zhang et al., 2015; Lee et al., 2020; 2022; Park et al., 2022). In the last decade, modern deep-learning artificial intelligence approaches are gaining traction also in related fault-detection (Hu et al., 2021; Naimi et al., 2022a; Kollias et al., 2022), reactor design (Dzianisau et al., 2022), fuel management (Hassan et al., 2021; Che et al., 2022), and safety analysis (Demazière, Christophe et al., 2020; Gomez-Fernandez et al., 2020; Ayodeji et al., 2022; Racheal et al., 2022). The integration with renewable energy sources and the energy storage systems are additional important emerging research fields (Denholm et al., 2012; Borowiec et al., 2019; Bragg-Sitton et al., 2020; Kim and Alameri, 2020).
The objective of this paper is to provide a literature review of the publicly available research on nuclear power plant load following operations, focusing on both neutron kinetics models and on methods/algorithms for reactivity/power control. Based on the existing knowledge, recommendations are given on both the constraints of nuclear power plant load following operations and the methods to monitor and predict them.
In addition, two reference scenarios for load-following operations of NPPs are proposed. The main objective of this work is to facilitate and standardise the testing and comparison of new models and algorithms for NPP load-following.
In Section 2, an overview of the methods to describe the reactor response to power changes is presented. In Section 3, possible strategies for reactor control are described. In Section 4, open questions and possibilities for future work are outlines based on findings from the literature review.
2 Neutron kinetics equations
Time evolution of reactor reactivity and power is a relatively mathematically complicated and computationally demanding problem. In general, a time dependent form of neutron transport equations needs to be solved (Bell and Glasstone, 1970; Duderstadt and Hamilton, 1976):
Despite the exponential increase in the available computational power, even nowadays it is not realistic to solve the above equation in its full generality, using neither deterministic nor stochastic methods. Therefore, different approximations have to be introduced. Transient neutron transport problems are usually treated using the neutron diffusion approximation, either quasi-static or time-dependent, or the point neutron kinetics approximation.
Time-dependent neutron transport is a problem that has been known for decades. A review of methods for space-time reactor kinetics was given e.g., by Chae (1979), with emphasis on different numerical methods and approximations based on the neutron diffusion equation. Diffusion theory methods for spatial kinetics calculations were also reviewed by Sutton and Aviles (1996). One possible way to derive nodal (multipoint) kinetics equations by introducing additional approximations and assumptions into the neutron diffusion equation is outlined by (Shimjith et al., 2010).
2.1 Reactivity feedback effects
In a realistic power reactor, reactivity can be divided into two components: an external one, which can be controlled, and an inherent one, which depends on the state of the system. Inherent physical phenomena affect reactivity during power transients. Reactivity feedback effects occur at different characteristic response times.
• Short-term effects:
– Fuel temperature feedback (time scale:
– Moderator temperature feedback (time scale: s—min).2
• Mid-term effects:
– 135Xe “poisononing” (time scale:
– 149Sm “poisononing” (time scale: days).
• Long-term effects:
– Transmutation of actinides (time scale: weeks—years).
– Fission product build-up (time scale: weeks—years).
In general, the total reactivity feedback effect Δρ can be written as:
where Δρm, Δρf, ΔρX, ΔρS and ΔρBU correspond to the feedback effects of the moderator temperature, fuel temperature, 135Xe build-up, 149Sm build-up and fuel depletion, respectively. For systems with a homogeneous mixture of fuel and moderator, both temperature feedback effects may be treated together.
Depending on the range of time scales of interest [tmin, tmax] for the simulation and the characteristic response time τ, different feedback effect may be treated in different ways.
• If τ ≪ tmin, in a quasi-static approximation.
• If τ ∼ t ∈ [tmin, tmax], explicitly.
• If τ ≫ tmax, as a constant.
Time tmin corresponds to the “resolution” of the simulation, and tmax to the total real time of the simulation. Whereas long-term effects are almost always taken into account indirectly, via some constants, e.g., averaged reaction cross sections, all combinations of treatment of short- and mid-term effects can be found in literature.2.1.1 Fuel temperature feedback
The fuel temperature feedback is primarily a consequence of Doppler-broadening of the resonances in the neutron induced cross sections with increasing temperature, leading to increased reaction rates. For most nuclear fuel types, including UO2 or MOX fuel used in typical PWRs, this increase affects the neutron capture rate significantly more than the fission rate, therefore the net effect on reactivity is negative.
For reactor designs using liquid nuclear fuel, such as e.g. the molten salt reactor, other factors, such as changes in fuel density, may contribute to the total reactivity feedback effect.
For an accurate calculation of the solid fuel temperature profile, the heat transfer equation must be solved using heat source based on a neutron transport calculation. This is computationally intensive but straightforward for fresh fuel. However, for (partially) burned fuel, thermal conductivity is affected by changes at both the microscopic scale (i.e. chemical composition, crystal structure) and the macroscopic scale (i.e. cracks, swelling, fission gas release).
However, the fuel temperature depends primarily on the thermal power density. For systems such as PWRs with fuel rods in the shape of very long thin cylinders, one might assume that the axial heat transfer is negligible compared to that in the radial direction. With the additional assumption that the distribution of the heat source is independent of the power, a linearised model of fuel temperature may be proposed for each axial slice k:
where.
• [Tf] (average) fuel temperature,
• [Tm] moderator/coolant temperature,
• [p] power density,
• [ff] power fraction deposited in fuel,
• [ρf] fuel density,
•
• [Ω] (effective) specific heat transfer coefficient from fuel to coolant.
The effect of fuel temperature on reactor reactivity is expressed as:where αf is the fuel temperature reactivity coefficient, and Tf0 is the initial (or reference) fuel temperature. In general, αf (mildly) depends on fuel temperature, and in a linear approximation, this dependence is ignored.
2.1.2 Moderator temperature feedback
The moderator temperature feedback is a consequence of the shift of the thermal peak in the neutron spectrum, which for increasing temperature leads to lower reaction rates. For liquid moderators, the change in moderator density also affects the ratio between the number of thermal and epithermal/fast neutrons. The second effect might be dominant in some reactor designs such as e.g. PWRs. For most nuclear fuel types, including UO2 or MOX fuel used in typical PWRs, both phenomena more strongly affect the fission rate than neutron capture rate in well-designed systems, therefore the net effect on reactivity is negative.
For an accurate description, liquid moderator temperature may be determined by an explicit thermal-hydraulic calculation. Unlike the fuel temperature, the moderator temperature in systems with cylindrical pressure vessels, such as light water reactors (LWRs) and liquid metal cooled fast reactors (LFRs), is primarily axially dependent. Assuming no radial dependence, the following model of moderator temperature reactivity feedback effect is proposed:
where.
• [ρm] moderator/coolant density,
• [λm] relative moderator/coolant flow rate
•
where αm is the moderator temperature reactivity coefficient, and Tm0 is the initial (or reference) moderator temperature. In general, αm (weakly) depends on the moderator temperature, and in a linear approximation, this dependence is ignored. Due to a typically much narrower interval of possible moderator temperatures, especially for PWRs where moderator temperatures are typically within [290,315] °C, this linear approximation is more easily justifiable compared to the linear approximation for the fuel temperature reactivity effect.
The model in Eq. 5 may have validity for moderator/coolant in PWRs and coolant for some gas-cooled reactor concepts, however it cannot even qualitatively describe the moderator temperature profiles in e.g., boiling water reactors (BWRs). In case of a liquid fuel, as e.g. in molten salt reactors, the same model might be applied for fuel temperature profile. Conversely, in case of a solid moderator, such as e.g., in gas cooled reactors or in TRIGA type research reactors, moderator temperature profile may be described using a model of a similar kind as the one for solid fuel, Eq. 3.
2.1.3 Feedback from 135Xe build-up
135Xe is a relatively short-lived (t1/2,X = ln 2/λX = 9.14 h) fission product with its thermal neutron capture cross section (
Where.
• [I] concentration of 135I,
• [X] concentration of 135Xe,
• [E1] total recoverable energy per fission event,
• [Σf] macroscopic fission cross section.
The 135Xe build-up is inherently non-linear and can only be linearised for small relative temporal variations of the power density. Assuming no migration of 135Xe gas, Eqs 7, 8 are separate for each spatial position, and it is straightforward to average them over any chosen spatial region. The effect of the 135Xe build-up on the reactor reactivity is expressed as:where ν is fission neutron multiplicity, and X0 is the reference (steady-state) 135Xe concentration.
Space-time oscillations of the 135Xe concentration, imprecisely also referred to simply as “xenon oscillations”, are a well-known phenomenon which might also occur during or after other, non-load-following, transients (Gyorey, 1962; Lellouche, 1962; Randall and John, 1962; Shotkin and Abernathy, 1963; Christie and Poncelet, 1973; Bauer and Poncelet, 1974; El-Bassioni and Poncelet, 1974; Onega and Kisner, 1978; Schulz and Lee, 1980; Kobayashi and Yoshikuni, 1982; Moon and Han, 1982; Teachman and Onega, 1983; Gondal and Axford, 1986; Berkan et al., 1991; Alten and Danofsky, 1993; Shimazu, 1995; Tiwari et al., 1996; Song and Cho, 1997; Song et al., 1999; Domingos et al., 2003; Marseguerra et al., 2003; Obaidurrahman and Doshi, 2011; Chang, 2016; Pradhan et al., 2016; Zarei et al., 2016). Whereas principles regarding the neutron kinetics are the same for the load-following operations, the objective functions for the control methods differ—typically, axial offsets of the thermal power density, 135Xe and 135I concentrations are observed.
2.1.4 Feedback from 149Sm build-up
149Sm is a stable fission product with its thermal neutron capture cross section (
Where.
• [P] concentration of 149Pm,
• [S] concentration of 149Sm,
• [E1] total recoverable energy per fission event,
• [Σf] macroscopic fission cross section.
Assuming no migration of 149Sm, Eqs 10, 11 are separate for each spatial position, and it is straightforward to average them over any chosen spatial region. The effect of 149Sm build-up on reactor reactivity is expressed as:where S0 is the initial (or reference) 149Sm concentration.
In practice, due to a much lower neutron capture cross section and cumulative fission yield, and a longer half-life of its precursor, the reactivity feedback effect caused by 149Sm is almost always small compared to the one caused by 135Xe. An exception is the reactor restart after a shutdown of several days or weeks.
2.1.5 Long-term effects from fuel depletion
The material composition of the nuclear fuel is changing significantly during its life in a reactor. There are multiple predominant effects.
• The removal (predominantly by fission and neutron capture) of fissile 235U (and plutonium in case of MOX fuel),
• The conversion of 238U into plutonium and other higher actinides,
• The formation of other nuclides, which can be categorised as actinides or fission products, that are important for fuel performance,
• In case of use of so-called “burnable poisons” (Evans et al., 2022), gradual removal of absorber nuclides typically present in the “burnable poisons”, such as 10B, 113Cd, 155,157Gd, etc.
As the nuclear fuel depletes, the reactor core reactivity decreases for most conventional fuel types and reactors. Therefore, to keep the reactor in a critical state for the desired time period of the operation cycle, the reactor core must start up with considerable excess reactivity. This excess reactivity must be offset with negative reactivity sources to make the core critical at the desired power level. Because the spatial profile of the fuel burnup has a strong influence on reactivity, variations in axial burnup are also important to criticality safety and must be addressed. As is typical for LWRs, the fuel burnup is slightly higher at the bottom of the fuel assembly than at the top. This variation is predominantly caused by the difference in the moderator density (sometimes referred to as the “axial offset”). The cooler (higher density) water at the assembly inlet results in a higher local fission rate (which subsequently results in a higher local burnup) than at the assembly outlet with the warmer moderator.
• Slow change of integral parameters
– Effective delayed neutron fraction βeff. For UO2 fuel the production of 239Pu in a reactor makes it the dominant fissile nuclide towards the end of the cycle. Since plutonium isotopes on average produce fewer delayed neutrons than 235U, the value of βeff typically decreases with burnup.
– Fuel temperature reactivity coefficient αf.
– Fuel thermal conductivity Ω. Thermal conductivity of the uranium oxide fuel is reduced with irradiation damage and progressive build-up of fission products (Ronchi et al., 2004).
– Macroscopic cross section. In thermal reactors, the macroscopic fission cross section typically decreases with burnup, whereas the macroscopic neutron capture cross section typically increases with burnup.
– Secondary: average fission neutron multiplicity
2.2 Reactivity control
In contrast to the reactivity feedback effects, which are inherent, automatic and inevitable, mechanisms exist to control reactivity externally, manually or automatically, on different time scales.
Practically all types of nuclear reactors include some sort of control rods which introduce an external reactivity ρCR, which is primarily a function of their position, however non-negligible secondary effects might be present depending on the operational conditions. This control mechanism has a typical response time of the order of seconds.
In addition, some nuclear reactor designs enable other ways of external reactivity control. For example, in PWRs reactivity may be changed externally by altering the concentration of boric acid in the water coolant/moderator. The negative reactivity effect from the boron is roughly proportional to the concentration of boric acid, however it depends also on other parameters such as moderator density and temperature. The typical response time of this control mechanism is minutes to hours. Due to boiling water in the BWR reactor core, the boric acid cannot be used for reactivity control.
2.3 One-energy-group point kinetics
In the past, both the spatial and energy dependence of neutrons were often even completely removed from the analysis of transients. In other words, it was assumed that the neutron population at each point in the neutron multiplication system was subject to an equal relative change in time. The general theory, explanations and justifications are available e.g. in Refs. (Keepin, 1965; Stacey, 1969; Hetrick, 1971; Ott and Neuhold, 1985).
In one-energy-group point kinetics equations, the delayed neutron precursor nuclides are typically merged into either 6 groups (Shibata et al., 2011; Brown et al., 2018) or 8 groups (Plompen et al., 2020), based on the half-lives of the precursor nuclides, or into a single group (Hui et al., 2020; Hui and Yuan, 2021; 2022c; d; Liu and Wang, 2014; Mousakazemi, 2019; Wang et al., 2019).
With following definitions.
• [n] population of prompt neutrons,
• [c] population of delayed neutron precursor nuclides,
• [ρ(t)] system reactivity,
• [β] effective delayed neutron fraction,
• [λ] effective decay constant of delayed neutron precursors,
• [Λ] prompt neutron generation time,
In some cases, other number of delayed neutron precursor groups may be used, such as e.g., 3 (Khorramabadi et al., 2008) or 8 (Plompen et al., 2020).In the basic form of the point kinetics equations, reactivity is a free parameter that can be controlled externally ρ(t) = ρext, i.e. no (inherent) feedback effects are simulated. In general,
where Δρ(t) includes all reactivity feedback effects. The latter can be, as indicated above, treated explicitly, i.e. in the form of additional (often differential) equations, in a quasi-static approximation, i.e. in form of a function of the state parameter(s), or as a constant or a slowly varying function of time.
For slow transients, prompt jump approximation may be introduced by assuming Λ → 0 (Ott and Neuhold, 1985). Following this assumption, Eqs 13, 14 simplify to:
In studies of the load-following operations, the one energy group point reactor kinetics approximation was frequently used in literature, both without (Torabi et al., 2011; Park and Cho, 1992; Li and Zhao, 2013a; b; Ben-Abdennour et al., 1992; Elsisi and Abdelfattah, 2020; Khajavi et al., 2002) and with the 135Xe reactivity feedback (Nair and Gopal, 1987; Edwards et al., 1990; Arab-Alibeik and Setayeshi, 2005; Khorramabadi et al., 2008; Li and Zhao, 2014; Zarei et al., 2016).
2.4 Multi-energy-group point kinetics
In principle, one might assume that while the neutron population in any point in the neutron multiplication system is subject to equal relative change in time, there might still be spectral changes in time. In theory, such conditions can be achieved by a change in the system that may be homogeneously introduced, e.g., by change of boric acid concentration in the coolant of a PWR reactor. However, in reality, spatial heterogeneity effects typically prevail over spectral effects and therefore this option was not frequently used in literature.
A notable exception is the work of Aboanber et al. (2014), where a two-energy-group point kinetic model with one group of delayed neutron precursors was used to study the reactor dynamics following external reactivity insertions.
2.5 One-energy-group multi-region kinetics
Some reactivity feedback parameters, especially the 135Xe concentration, may affect the spatial power distribution within the reactor core, thereby invalidating the point reactor approximation. Therefore, neutron population has to be tracked in multiple regions. This can in general be treated using two possible approaches.
1. To generalise the point reactor kinetics equations to multiple “points” (corresponding to geometrical regions in the system).
2. To simplify the neutron transport/diffusion equation to a (very) coarse spatial grid.
In the first approximation, two-region kinetics can be used to qualitatively describe the coupled space-time 135Xe oscillations. Typically, the reactor core is divided in the upper and the lower half since the heterogeneity is often introduced by partial insertion of the control rods in the upper half of the core for PWRs and in the lower half of the core for BWRs. Additionally, reactivity feedback parameters, such as moderator density and fuel burnup, primarily have an axial rather than a radial asymmetry. The two-region reactor kinetics can be derived starting from the point kinetics equations, e.g. based on Eq. 14. A typical form is.
It is worth to emphasise the coupling coefficient αS, which determines the relative coupling of the two-halves of the system, and the two externally controlled reactivities ρext,1 and ρext,2. This is a fundamental qualitative change compared to the single point kinetics. Unless a constraint is added without introduction of new free parameters (e.g., if there was only one possibility for the reactivity control), the additional degree of freedom essentially means that there are multiple possibilities to follow the prescribed reactor power. This opens a completely new topic of control strategies and methods, which is discussed in Section 3.
In literature, the 2-point reactor kinetics was applied numerous times (Ansarifar and Saadatzi, 2015; Eliasi et al., 2011; Hui and Yuan, 2022a; Li, 2014a; b; Li et al., 2014b; Na et al., 1998b; a; Na, 2001; Parhizkari et al., 2015; Saadatzi and Ansarifar, 2017; Yadav et al., 2018), optionally using the prompt jump approximation (Onega and Kisner, 1978; Kobayashi and Yoshikuni, 1982; Winokur and Tepper, 1984; Marseguerra et al., 2003) or even the quasi-static approximation (Shimazu, 1995), and most frequently taking into account a single or three delayed neutron precursor groups. 2-point kinetics model may be coupled with a thermal hydraulic calculation (Pradhan et al., 2016) for a more precise description of the changes in the coolant/moderator temperature and density.
There are three basic approaches for the multi-region reactor kinetics (Sutton and Aviles, 1996): direct methods, space-time factorisation methods, and modal methods. The direct methods are computationally more intensive with a higher fidelity, therefore they are most frequently used with an increasing trend. They may further be divided in finite difference, coarse-mesh and nodal methods. The finite difference methods typically use a more refined spatial grid compared to the other two, which is compensated by essential use of effective diffusion homogenisation (EDH) (Trkov and Ravnik, 1994; Ćalić et al., 2016) approach in coarse-mesh methods. A major difference is also in the basic philosophy: whereas the finite difference and coarse-mesh methods are based on a top-down approach, i.e. by simplification of the diffusion equation, the nodal methods are essentially bottoms-up, i.e. by expanding the point reactor kinetics equations.
The nodal multi-point kinetics model was used in different forms.
• Prompt jump approximation (Ukai et al., 1990); linearised (Cho and Grossman, 1983).
• 1 delayed neutron group (Liu et al., 2016; Chakraborty et al., 2018; 2019)—linearised (Tiwari et al., 1996; Talange et al., 2006).
• 3 delayed neutron groups (Zaidabadi nejad and Ansarifar, 2017; Zaidabadi nejad and Ansarifar, 2018).
1-group 1D coarse-mesh (axial) diffusion was used with explicit treatment of the prompt neutrons e.g., by Domingos et al. (2003); Kastin et al. (2019), or in a quasi-static approximation (Christie and Poncelet, 1973; Bauer and Poncelet, 1974; Teachman and Onega, 1983; Yoon and No, 1985; Yim and Christenson, 1992; Alten and Danofsky, 1993; Song et al., 1999). A rare example of a 1D radial time-dependent one-energy-group diffusion equation was studied by Winokur et al. (1979). Franceschini and Petrovic (2008) used a 1D one-energy-group “NDA-approved Westinghouse code”, however no further details were revealed.
On the other hand, the modal expansion was used in earlier decades of computational reactor physics, both in a non-linear (Gyorey, 1962; Canosa and Brooks, 1966) and a linearised form (Lellouche, 1962; Schulz and Lee, 1980).
Mascolino and Haghighat (2019) used a fundamentally different approach to neutron kinetics, the so-called fission matrix (FM) method, implemented in the code RAPID (Walters et al., 2015; Mascolino, 2021). The calculation of the FM coefficients is based on continuous energy Monte Carlo simulations, and the coefficients are defined for many spatial regions, integrated over the neutron energy and for one delayed neutron precursor group.
2.6 Multi-energy-group multi-region kinetics
For the thermal reactors, the 2-energy-group approach, where the thermal neutrons are treated separately from the rest, i.e. epithermal and fast neutrons, is frequently used for static analyses on the reactor core level, usually in combination with the neutron diffusion equation. A similar method can also be applied for dynamic problems.
Ye and Turinsky (1998) used a 1D 2-group core quasi-static model with the Taylor expansion of variables such as the coolant density, the fuel temperature and the soluble boron concentration as a function of the fuel burnup in control rod insertion.
Similarly, Moon and Han (1982) used the 1D 2-group quasi steady-state diffusion code DD1D with 135Xe and 149Sm feedback.
Gondal and Axford (1986) developed a 2-group diffusion model with 2D plane geometry and 135Xe and 149Sm feedback, with Fourier transform.
Shimjith et al. (2010) used a more rigorous 3D nodal 2-group diffusion model with the neutron kinetics and linearised equations for 135Xe/135I.
The code SIMULATE-3 (Smith, 2007) was e.g., used by Lin and Shen (2000) using a 2-energy-group approximation without modelling the reactivity feedback due to 135Xe build-up.
For more accurate calculations, a larger number of energy groups and more geometric details should be introduced, however in practice this approach is due to an increased computational expense frequently combined with a less detailed treatment of the time-dependent phenomena.
The Multipurpose Analyzer for Static and Transient Effects of Reactor (MASTER) code (Cho et al., 2002) includes capabilities for transient PWR and BWR reactor core analysis. It was used to solve the 3D time dependent diffusion equation (Na et al., 2004; 2005), however without 135Xe and 149Sm feedback.
The Studsvik code SIMULATE5 is a 3D, steady-state, multi-group nodal code for the analysis of both PWRs and BWRs. Apart from the diffusion approximation it may also use the P3 approximation, i.e. where the Legendre expansion of the angular distribution of the neutron population up to the third order is used, and it includes a coupling module with thermal-hydraulic feedback.
The DYNCO nodal diffusion code for dynamic VVER simulations was used e.g., by (Boroushaki et al., 2003) to study the transient behaviour as a result of the control rod movement.
The code LoadF (Trkov, 1997; Trkov, 1998; Trkov et al., 1998; Trkov and Kromar, 1998) for transient and load-following operations of PWR reactors is based on the GNOMER few-group diffusion code (Trkov, 1994), which is used for whole-core power distribution calculations in the quasi-static approximation. It includes a thermal-hydraulic module. Later, Merljak et al. (2017) added a neutron kinetics module to GNOMER, which was however not incorporated into LoadF. LoadF includes capabilities for 3D modelling of control rod movement and reactivity feedback from fuel and moderator temperature and 135Xe and 149Sm build-up. LoadF was verified (Trkov, 2012) and optimised (Trkov and Kurinčič, 2001) for the use in the Krško NPP.
The code TRIKIN (Obaidurrahman et al., 2010) is a 3D multi-group time-dependent diffusion code with a 1D lumped thermal-hydraulic feedback module. With an added 135Xe build-up module it was used by (Obaidurrahman and Doshi, 2011) to study the spatial stability of the system.
3 Control strategies
Following the load with a PWR is a challenging control problem, since the response of a PWR to power and reactivity changes depends on many parameters and is non-linear, with dynamics of a wide range of time scales from prompt neutrons to fuel depletion. For the simulation of PWRs, studies of control strategies mostly use non-linear models of various complexity levels. For a detailed evaluation of the spatial power densities, studies with spatial 3D models are required. However, simple control evaluations that can demonstrate 135Xe “poisoning” effects can be carried out using single-point (1P) dynamics models. At least two-point (2P) models are required for the demonstrationof the oscillations of the axial power offset.
For control design, local linearisation about the operating point using the first-order Taylor series expansion is often used. Near criticality conditions where a PWR is normally operated, local dynamics of an integrating character (with one pole at the origin) are present. In addition, non-minimum-phase (unstable zero) dynamics of the 135Xe concentration may be observed. Thus, the choice of the applicable control methods is restricted, and care must be taken that all internal signals have a stable behaviour. Control design approaches can be classified to linear and non-linear design methods; there is also an intermediate way where non-linear control is designed by blending of local linear controllers.
The control approaches differ in the choice of actuators. PWRs are generally controlled by adjusting the reactivity via the control rods and/or the boric acid concentration. Often, rough approximations are used, where control is performed with a single or two control rods, although this cannot be considered realistic for load-following control over a wide range of power levels. In practice, the control rods are typically grouped in several rod banks acting in pre-defined coordination (e.g., in overlap). For reducing axial offset issues, some strategies make use of part-length and/or part-strength control rods. In some publications, the actuator velocity rather than the actuator position is chosen as the control input.
Further differences between the approaches can be noticed in the choice of controlled output variables. For automatic feedback control, only the process quantities which can be reliably measured or estimated from the available sensors may be considered. The main controlled variable is the reactor thermal power, mostly used in the relative form (to the maximum nominal power) corresponding to the relative average neutron density. In practical applications, the coolant outlet temperature may be used in the same sense. Additional controlled variables may be used for control of the axial power profile, most commonly the power axial offset (AO) or the axial normalized power (neutron flux) difference ΔI
where p is the power, relative to the nominal power, n the relative neutron density, ϕ the relative neutron flux, and the indices T and B denote the top and bottom half of the reactor, respectively.
• In simplified approaches based on the 1P models, only the relative power is controlled to its set-point (Ben-Abdennour et al., 1992; Torabi et al., 2011; Li and Zhao, 2014; Wang et al., 2017).
• Commonly, the relative power and the AO are controlled to their set-points (Li et al., 2014b; Ansarifar and Saadatzi, 2015; Zaidabadi nejad and Ansarifar, 2018). The set-point value for the AO is in most cases a linear function of the relative power set-point.
• The relative power is controlled to its set-point, while the AO is demanded to be in a prescribed (typically, 5%) vicinity of its set-point (Sipush et al., 1976; Meyer et al., 1978; Onoue et al., 2003; Boroushaki et al., 2004; Zhang et al., 2015). AO control may be turned off while the AO value is within the allowed interval (Lee et al., 2012). Optimization-based approaches may consider the allowed interval as a constraint in an optimization problem (Eliasi et al., 2011).
• Alternative to control of the AO, some approaches use separate control of the relative power in two (or more) model nodes (Na et al., 1998b; Mousakazemi, 2019).
• Approaches based on distributed-parameter models may attempt to control the axial shape of the power profile (Winokur et al., 1979; Cho and Grossman, 1983; Ukai et al., 1990).
Most publications on PWR control consider the isolated problem of the reactor core thermal power (and possibly axial power distribution) control; some also address the heat exchanger and the steam turbine (Park and Cho, 1992; Naimi et al., 2022b).
In many nuclear power plants, PWRs are operated with manual control, where operators directly adjust actuator positions. This is feasible because the time-scale of the challenging dynamics is relatively slow (seconds to minutes). Manual control may be demanded in nuclear power plants due to safety protocols. However, it may not be convenient in load-following regimes with frequent load changes, because adjustments are required frequently and transcribing them manually does not necessarily contribute to safety. Automatic feedback control is routinely used in many safety-critical applications. Certainly, such control must undergo rigorous testing procedures. The majority of the reported control approaches is described at a conceptual level, and their practical applicability is questionable. Some of the practical load-following strategies have been tested with fixed daily set-point patterns, and in fact implemented with manual operator control (Sipush et al., 1976). Manual control may be assisted with an automatic estimation and prediction of important non-measured states for the decision support (Sipush et al., 1976; Trkov et al., 1998).
In the following subsections, automatic feedback control strategies relevant to load-following operation of PWRs are listed and grouped according to the predominant control approach. This should however not be considered as a strict classification, because many strategies blend different approaches.
3.1 Linear PID control
PID (proportional/integral/derivative) control is the most widespread form of automatic feedback control (Oka and Suzuki, 2013; Kerlin and Upadhyaya, 2019). Its simplest form, the proportional controller, computes the control signal u(t) (the manipulated variable) as u(t) = KPe(t), where KP is the proportional gain and e(t) is the error signal between the reference output and the system output signals, e(t) = yr(t) − y(t). The full PID control adds integral and derivative terms,
• Mousakazemi (2019) presented a control scheme where two PID controllers are used for the control of the neutron densities in the top and the bottom part of the reactor represented by a 2P model. The parameters of the two PID controllers are optimised using a genetic algorithm (GA), where the cost function comprises the settling time and the ITAE performance index evaluated in several regions of a load-following scenario. The stability of the control system is analysed using a Lyapunov-like function.
3.2 Linear state-feedback based control
State-feedback control is commonly used with state-space models, where the current state of a dynamic system is described with a state vector x(t). Then, a convenient control law for computing the control signal u(t) becomes u(t) = K(t)x(t), where K(t) is the controller gain matrix (which is often constant). The closed-loop pole assignment (placement) is a common technique for determining the gain matrix. Continuous-time and discrete-time implementations are possible.
System states often cannot be measured, and control can only be implemented from the measured outputs (output feedback). In such case, a state observer (estimator) may be used to reconstruct the system state estimate
A linear-quadratic (LQ) optimal controller is a particular form of a state-feedback controller where the controller gain is determined by minimizing a cost function imposed on a quadratic cost function penalizing the system state x(t) and the control input u(t) over an infinite future horizon. Assuming a linear stabilisable system without any signal constraints, a stable control law with the controller gain matrix K(t) can be computed via the Riccati equation. Most commonly, its steady-state solution is used as a static gain. For the output-feedback case, its dual approach, the optimal state estimator (Kalman filter) is used for the estimation of
The basic form of the state-(output-)feedback control results in driving the system state (output) vector to zero, possibly with a steady-state offset. Extensions may be required for tracking non-zero reference signals and for the elimination of steady-state offsets due to non-zero-mean disturbances.
• Edwards et al. (1990) presented an approach named State Feedback Assisted Classical Control (SFAC). SFAC combines a “classical control scheme”, using PI control with the temperature feedback, with a state-feedback controller with an observer, both based on a linearised state-space model and tuned with the pole assignment.
• Li and Zhao (2014) described a load-following control scheme based on process dynamics linearised in 5 operating points, using state-feedback control tuned using a robust pole assignment method and a Kalman filter, using fuzzy logic for soft switching among the local linear controllers. Global stability of the system is analyzed using Lyapunov functions. Simulation results in load-following using a 1P non-linear PWR model are presented.
• Li (2014a) compared two state-feedback control approaches based on linear robust pole assignment on a 2P non-linear PWR simulation model, controlled using a two-region power control rod and an axial offset (AO) control rod. In Case 1, a linearised single-variable linear model is used, and state-feedback control is implemented by utilizing the robust pole assignment method with an additional integrator, for control of power where the same control signal is applied to both actuators. In Case 2, a linearised 2-input 2-output model is used, and an integral decoupling control system with a dynamic controller is devised for control of power and axial offset using the two rod actuators separately. For the state estimation, a Kalman filter is used in both cases. In Li et al. (2014b), the approach was upgraded by using 10 local models linearised at different power levels and the same number of local controllers with smooth switching, and global stability of the system is established via a Lyapunov function.
• Ben-Abdennour et al. (1992) presented a state-feedback control scheme for the PWR reactor, represented with a 1P non-linear model, with a Linear-Quadratic Gaussian (LQG) optimal controller, comprising a LQ optimal state controller and a Kalman filter for state estimation. For LQG tuning, the Loop Transfer Recovery technique is used due to its effectiveness in accommodating plant uncertainty. The simulation performance in a load-following regime is compared to the state-feedback assisted control.
• Li and Zhao (2013a) described a multi-model state-feedback control approach with 5 local models linearised from a 1P non-linear PWR model at different power levels where for each local model a LQG controller is designed and combined with a PID controller, where PID control parameters are tuned using Improved adaptive genetic algorithm (IAGA) to yield the best performance in a load-following scenario. A fuzzy supervision mechanism is used for switching among the local controllers. Global stability analysis is performed using a Lyapunov function. In a similar context, Li and Zhao (2013b) presents another variant of LQG state-feedback control where a controlled plant is augmented with an additional integrator, so that the LQG controller can remove the steady-state error for input commands and disturbances that are non-zero in the steady-state, such as the step changes. The LQG controller is tuned using the Loop Transfer Recovery approach aimed at improving the robustness. The principle of flexibility control, which uses a fuzzy supervision mechanism to combine the local linear controllers, is used to maintain control of the non-linear core at a random power level. Case 2 in Li (2014b) expands the LQG/LTR approach to the 2-input 2-output control problem where the reactor power and AO are controlled via the power control rod and the AO control rod.
3.2.1 Linear minimum variance control
The minimum-variance controller is a simple form of optimal control which attempts to minimize the variance of the system output (or, in the tracking version, the tracking error between the system output and its set-point signal) at one point in the future (k steps ahead). For predicting the system output at this future point, a system model is used.
•Na et al. (1998a) described a multivariable adaptive control algorithm applied to the axial neutron flux shape control in a PWR. The reactor model used for computer simulations is a 2P model based on the non-linear 135Xe and 135I balance equations and the neutron diffusion equation with non-linear power reactivity feedback. The reactor core is axially divided into two regions, and each region has one manipulated input (the change in absorption macroscopic cross section between two time steps) and one controlled output (the deviation of the normalized neutron flux from its steady state) and is coupled with the other region. The controlled process is described by a matrix polynomial linear model. A weighted k-step-ahead minimum-variance controller is designed to achieve a compromise between the fast responses and the amount of control effort. Integral control action is added to remove the steady-state offset. A recursive scheme based on the generalized least-squares method parameters is used for direct adaptation of the controller.
3.2.2 Linear receding horizon control/model predictive control
Receding horizon control (RHC)/model predictive control (MPC) algorithms can be seen as an extension of the minimum-variance control algorithms, where a quadratic cost function penalizing the system state/output and the control action is computed over a whole horizon of future points instead of a single one. The future system response to changes in the manipulated variables is predicted by using a model, which may be in state-space, polynomial or impulse- or step-response form, respectively. At each sampling instant, an optimization problem for a future horizon is solved. In the absence of constraints, this is a least-squares optimization problem, with an analytical solution in the form of a matrix gain. However, the MPC problem setup conveniently allows imposing constraints on process signals, and in the constrained case with linear constraints this results in a quadratic program (QP). Following the receding-horizon principle, the first of the computed sequence of the optimal future control moves is implemented as the current control input. The rest are discarded, as the procedure is repeated in the next time step. Due to a computer implementation, most practical implementations are discrete-time; but it is also possible to use continuous-time models and cost functions. Originally, the application of QP-type online optimization with state/output constraints was computationally feasible only for processes with slow dynamics, with sampling times measured in hours. Recently, specialized QP solvers are approaching the millisecond range.
Due to the constraint-handling ability and the straightforward design of control schemes with multiple manipulated and controlled variables, MPC has become the advanced control of choice in many industries. However, a constraint on signals must be placed very carefully, because conflicting constraints may render the MPC optimization problem unfeasible. Due to this, practical implementations use prioritization or softening of constraints. Several theoretically advanced methods address the stability and feasibility and improve the robustness of MPC by combining a finite-horizon MPC controller with an LQ controller and by using the theory of invariant sets.
• Na (2001) applied receding horizon control (RHC, also known as model predictive control—MPC) to the axial power distribution control in a PWR. The reactor model used for computer simulations is a 2P non-linear model that describes axial distribution for the one-dimensional diffusion equation and the 135I and 135Xe balance equations using trigonometric functions. Then, averages for the upper and the lower half of the reactor are computed. The reactor core is axially divided into two regions, and each region has one input and one output and is coupled with the other region. An equation of the steady-state neutron flux amplitude is derived and solved by maintaining the reactor nearly critical using a variational estimate of the eigenvalues of the one-dimensional diffusion equation. For the implementation of RHC, a linearised model in the form of a matrix polynomial model was obtained through the linear identification of the non-linear 135Xe oscillation model using a conventional parameter estimation algorithm. The outputs of the model are the two normalized neutron fluxes, controlled to their respective target values, while variation in absorber cross sections between the two neighboring time steps for both reactor halves are used as manipulated variables in the time-differential form.
• Na et al. (2004) proposed a study of an adaptive model predictive control (MPC) method for control of the reactor power level and of the axial shape index (ASI) for Korea Standard Nuclear Power Plants (KSNPs). A 3D nuclear reactor analysis code MASTER is used for simulation of the PWR response. The reactor has 5 power control rod banks, and 2 part-length control rod banks, which have only the bottom part filled with the neutron absorber material. The MPC cost function penalizes deviations of the predicted output signal from its set-point during the prediction horizon and the changes of the manipulated variable during the control horizon. For model predictions, a linear polynomial Controlled Auto-Regressive and Integrated Moving Average (CARIMA) model is used. The parameters of the CARIMA model are estimated at every time step to reflect the time-varying conditions of the plant, using a recursive parameter estimation algorithm. In the MPC power level control scheme, the adaptive MPC controller controls the PWR power to its set-point in a load-following scenario by manipulating the power control rods. The 5 power control rod banks are used with a fixed overlap, so that they are controlled as a single manipulated variable. ASI is not controlled and exceeds the allowed limits. Boric acid concentration is constant. In the MPC ASI control scheme, the adaptive MPC controller regulates ASI by manipulating the part-length control rods. Boric acid concentration is adjusted to maintain the desired power. The power control rods are withdrawn. For both schemes, simulations with and without constraints on control inputs and outputs are provided. In Na et al. (2005), the approach is extended to the two-input two-output integrated MPC power level and axial power distribution control. The control scheme also makes use of boric acid concentration adjustment, automatically related to the power control rod movement, but does not exceed the boric acid treatment capacity. Lee et al. (2012) further extends the MPC approach with a support vector regression (SVR) model to predict the future outputs based on previous inputs and outputs. SVR modelling is a machine learning approach that searches for the network weights of an artificial neural network with a kernel function by solving a non-convex unconstrained minimization problem. The model is trained with a learning algorithm originating in the statistical learning theory and in the structural risk minimization. Due to the non-linear model, the objective function of a MPC control algorithm with multiple objectives is non-convex and may have multiple local minima. A genetic algorithm is employed for optimization of the MPC cost function. The MPC controller manipulates 5 full-strength and 2 part-strength control banks, using a sequence with a fixed overlap. It may operate in the single or the dual control mode, depending on the ASI error. While the ASI error is below 5%, the single mode is used, where only the power is controlled. Otherwise, both the power level and ASI are controlled. Additionally, automatic adjustment of the boric acid concentration is performed using a set of rules, depending on the full-strength control rod position and the power tracking error.
• Wang et al. (2017) proposed a MPC method for core power control in a PWR. For the power prediction, a linear state-space model is obtained from a 1P non-linear model (not considering 135Xe dynamics) using linearisation and time-discretization. The minimization of the MPC cost function is a constrained optimization problem, solved by a quadratic programming (QP) solver.
3.3 Linear robust control
A control system is generally deemed robust if its performance is relatively insensitive to changes in the dynamics. Closed-loop control systems are often designed for certain nominal system dynamics. However, the performance is likely to change due to system non-linearity or time-variation, and the change in the performance may depend a lot on the design and the tuning of the controller. The aim of the robust control design is to ensure system stability, or performance to given specifications, despite a predefined uncertainty of the dynamic system, by control design. There is a wide variety of robust control design approaches, many of them being enhancements of other control methods.
• Torabi et al. (2011) presented a robust power control system for the nuclear reactor using the Quantitative Feedback Theory method (QFT). The PWR plant is represented with a 1P non-linear model. By using model identification from input-output data of simulated experiments using the non-linear model that sweeps the power range of load-following operation with a superimposed pseudo-random binary signal, two uncertain second-order linear models are generated, where parameter intervals are determined rather than specific parameter values. The first model is generated with the 1P-kinetic model with one delayed neutron precursor group and a constant reactivity worth of control rods along the vertical axis, and the other one is a 1P-kinetic model with six delayed neutron precursor groups and a variable reactivity worth of control rods along the axis. Robust QFT control design aims to design a controller that stabilizes the system for any parameter values within the specified intervals of the model uncertainty.
3.4 Non-linear control
Non-linear control is a heterogeneous group of control methods that do not rely on the assumption that a linearised approximation of a system can be used for control design, or apply an inherently non-linear control law.
• Park and Cho (1992) introduced the control method called Model-based Controller with Adaptive Proportional-Integral gains (MCAPI), which may be considered as a subset of non-linear global linearising control (GLC). MCAPI is designed for a single-input single-output control system. A gain adaptation algorithm for the PI gains based on the Lyapunov’s second method is used. An open-loop observer is used to estimate the non-measurable state variables. The effect of estimation error in the open-loop observer due to mismatches in the initial conditions and system parameters is compensated by an uncertainty estimator. MCAPI is used for PWR control, which also includes the steam generator. A 1P non-linear model of the primary PWR loop is used, without considering the 135Xe and the fuel depletion effects. The reactor coolant system model is divided into five nodes to simulate the energy balance between fuel and coolant and the transport delays between reactor core and steam generator. The steam generator model comprises heat transfer between the reactor coolant system and the secondary side. The turbine load variation is performed by changing the steam flow to the turbine. Non-linearity in the heat transfer between the fuel and the coolant is considered. The reactor control system consists of two channels: temperature and power deviations from their respective set-points. The output of these two channels is used to drive the control rods. The total tracking error signal sent to the rod controller is given by the weighted sum of the temperature deviation and the power deviation.
• Park and Cho (1993) proposed a hybrid control strategy consisting of a time-optimal (coarse) and a model-based adaptive (fine) controllers, applicable to the power-tracking control of small research or spacecraft nuclear reactors in which the point kinetics equations are valid. The method is based on a 1P non-linear model of the PWR, where the 135Xe dynamics are not considered. During the coarse control stage, where the power output is not yet close to its set-point, the maximum allowed control effort is used to direct the system toward the switching boundary near the desired power level. At this boundary, the control is switched to the fine control stage, adaptive proportional-integral-feedforward (PIF) controller. Feedforward action from the power set-point is used to decrease power over-/under-shoots at non-smooth set-point changes. As the feedforward signal, an estimate of the temperature feedback reactivity using inverse neutron kinetics and prompt-jump approximation are used. The PI control gains and the feedforward gain are adapted with a direct adaptation approach based on Lyapunov’s second method.
• Zaidabadi nejad and Ansarifar. (2018) presented robust feedback-linearisation control (FLC) for the axial power distribution for PWR in load-following operation, with the aim of improving the load-following capability in the presence of parameter uncertainty and disturbances. The approach is based on a non-linear 4P reactor model with parameters fitted to the VVER-1000 PWR. The negative reactivity insertion at the top of the PWR core is controlled using the first control rod banks, which are assumed to travel through the top, and reactivity insertion at the bottom of the core is affected by the second control rod bank, which is assumed to travel through the bottom of the core. The conventional FLC comprises two cascade control loops, of which the inner non-linear controller eliminates the non-linear terms of the process dynamics, and the outer loop controls the linearised system. However, the conventional FLC is not applicable to systems with unstable internal dynamics. Non-minimum phase response of the 135Xe concentration is evident in the PWR model simulations. Hence, at the second step of controller design, the desirable control law according to feedback-linearisation is combined with a dynamic SMC for the robust FLC. The performance of the robust FLC is compared to the SMC. SMC can be applied to PWR control separately, however the control signal exhibits considerable chattering, a high activity of the control action, and boundary layer thickness expansion. A decrease in the chattering phenomena leads to a large steady-state tracking offset.
• Yadav et al. (2018) designed a non-linear dynamic inversion (NDI) based controller design coupled with constrained optimization for the load following operations in a PWR, with the aim of PWR over a wide range of reactor power with bounded 135Xe oscillations and satisfying the operational constraints imposed by the reactivity worth of the control devices and allowable fuel and coolant temperatures. NDI is a state-feedback non-linear control technique which uses exact feedback linearisation. Using the inverse system model it calculates the required control input to follow a desired output trajectory. A two-point non-linear PWR model is used. Two banks of control rods are considered, where the first control rod bank enters from the top half of the reactor, and the second control rod bank from the bottom and is assumed not to exceed beyond the central plane. The NDI controller is designed for the two-input-two-output system and controls the power in the top and in the bottom halves of the PWR to their respective set-points. A reduced-order observer for the non-linear plant is used for the estimation of the 135Xe, 135I and the delayed neutron precursor concentrations which are not measured. The observer gain is chosen so that the error dynamics is Lyapunov stable. The controller parameters are determined using constrained optimization to minimize the absolute value of the difference between the neutron densities of the top and bottom half of the PWR while meeting operational constraints over the whole power range of the load-following operation.
• Eliasi et al. (2011) proposed a robust non-linear MPC for the PWR load-following operation problem that ensures the 135Xe oscillations are kept bounded within the operational limits. The controller imposes restricted state constraints on the predicted trajectory during optimization which guarantees robust satisfaction of the state constraints using the invariant set approach, without solving a min–max optimization problem most commonly used in robust MPC. The controller is based on a 2P non-linear model of the PWR reactor. The model uncertainty is considered in the form of a vector of bounded uncertainties and bounded disturbance, containing the power and the one-group delayed neutron precursor, 135I and 135Xe concentrations for the two reactor halves, respectively. The reactor is controlled via a single control rod. Specifically, control rod speed is used as the manipulated variable. The MPC cost function penalizes deviation of the system state from its desired value and the control effort. The axial offset is not regulated to a set-point, but rather treated as a robust state constraint of the controller optimization problem. For the analysis of the stability properties of the closed-loop system in the presence of bounded persistent disturbances and state-dependent uncertainties, the characterization of the input-to-state stability (ISS) in terms of Lyapunov functions is used.
• Ansarifar and Saadatzi (2015) presented the design of a sliding mode control (SMC) for the load following operation problem in a way that ensures that the 135Xe oscillations are kept bound within the operational limits by using a constant axial offset (AO) strategy. SMC is a variable structure control (VSC) system. A sliding mode is a motion on a discontinuity set of a dynamic system and is characterized by a feedback control law and a decision rule known as the switching function. A stable switching surface is defined, and SMC design chooses the control law in a way as to satisfy an equation which draws the state of the system towards the sliding surface within a finite time for any initial condition. Once the state trajectory reaches the sliding surface, it remains on it for the rest of the time, hence the sliding condition makes the sliding surface an invariant set. The control rule is discontinuous along the switching surface and chattering occurs in the vicinity of the sliding surface. Various techniques are used to reduce chattering. Here, the boundary layer approach is used, which replaces the sign () function with the tanh () function. The reactor core is represented with a 2P non-linear nuclear reactor model with a three delayed neutron precursor groups. Two banks of control rods are considered; the first control rod bank is assumed to travel through the top to reach the bottom of the core, and the second control rod bank only affects the top of the core and is assumed to control the AO. Control rod velocities are considered as manipulated variables. The controller is designed to reduce the tracking errors of the desired relative power and the axial flux difference (ΔI), respectively. The stability analysis is given by means of the Lyapunov approach. Saadatzi and Ansarifar (2017) extend the previous SMC design with a more accurate model with three delayed neutron precursor groups and with a sliding mode observer with robust properties, which is used to estimate the 135Xe concentration and the densities of the delayed neutron precursors that cannot be measured; Zaidabadi nejad and Ansarifar (2017) further developed the adaptive robust SMC control based on the multi-point nodal kinetics reactor model with 2, 4, 6, or 8 axial nodes representing a VVER-1000 PWR reactor. The model is validated with experimental measurements of a fast rod drop accident. It is observed that the basic SMC design exhibits acceptable tracking performance in the presence of parametric uncertainty only at the expense of high gains and control chattering. Therefore, the SMC controller is combined with an online parameter estimator, where the adaptation laws for updating parameters are generated using the Lyapunov approach; Wang et al. (2019) presented a SMC control strategy for power control in modular high-temperature gas-cooled reactors (MHTGR) based on a 1P non-linear nuclear reactor model without considering the 135Xe dynamics, represented as a Takagi-Sugeno fuzzy model, where an adaptive algorithm is used to reduce the chattering phenomenon of SMC. Hui et al. (2020); Hui and Yuan (2021, 2022b,c) described several variants of SMC control of the PWR power based on a 1P non-linear nuclear reactor model without considering the 135Xe dynamics, where non-linear state estimation of the non-measured states of the model and output disturbances was used, using different approaches for the suppression of the SMC chattering. In Hui and Yuan (2022a), a related approach was based on a 2P non-linear PWR model also considering the 135Xe and 135I dynamics, where the AO is controlled in addition to the reactor power.
• Elsisi and Abdelfattah (2020) discussed optimal design of a state-feedback variable structure controller (VSC) for PWR control, where the VSC parameters (switching vector elements and feedback gains) are optimized using the lightning search algorithm (LSA), and compares it to a VSC tuned using a genetic algorithm (GA). Both LSA and GA are heuristic optimisation approaches aimed at solving non-linear optimisation problems which may have multiple local optima. A Lyapunov function is used for the purpose of ensuring stability. A 1P non-linear PWR model is used, with 6 precursor groups for simulation and only one for the control design. The performance of the two approaches in a load-following cycle is evaluated using the IAE and ISE performance indices.
• Na et al. (1998b) applied a neuro-fuzzy control algorithm for the core power distribution in a PWR. Fuzzy controllers (FC) facilitate the creation of non-linear controllers heuristically by forming tables containing local control rules. The designer selects input fuzzy variables that characterize system non-linearity and subdivides each of them into distinct regions softly by using partially overlapping (here, Gaussian) membership functions. With the first-order Sugeno–Takagi type FC, the rule output according to each of the table cells consists of linear combinations of the input variables. The joint FC output is computed by blending the active rules according to the affinity of the inputs to their membership functions. The rule base may be built heuristically from control experience of an expert. However, an alternative interpretation of the FC structure as a form of an artificial neural network (ANN) allows data-driven tuning of the FC from input-output data sequences using an optimization algorithm, in this case the back-propagation algorithm. The reactor model used for computer simulations is a 2P non-linear model based on the non-linear 135Xe and 135I balance equations and the neutron diffusion equation having non-linear power reactivity feedback, with parameters set for the Oconee Unit 2 power plant. Two FCs are used. In particular, the input fuzzy variables for the lower FC are the difference between the normalised neutron flux in the lower half of the reactor from its target, and the difference in absorber cross section between two neighboring time steps in the lower half of the reactor. The input fuzzy variables for the upper FC are the difference between the normalised neutron flux in the upper half of the reactor from its target, and the difference in absorber cross section between two neighbouring time steps in the upper half of the reactor. The interaction between the regions of the reactor core is treated by a decoupling scheme.
• In Lin and Shen (2000), an artificial neural network (ANN) -based control technique was applied to control a PWR in load-follow operations. Direct inverse control architecture was adopted in which the ANN was trained off-line to learn the inverse model of the PWR. The training data were generated by the 3D core simulation model SIMULATE-3. Two ANN controllers were designed by an adaptive neuro-fuzzy inference system (ANFIS): one for control of total core power by manipulating the change in boron concentration, and the other for the control of the axial power distribution by manipulating the provided control rod position. Each of the ANN controllers had 4 inputs and one output. The dynamic nature of the controllers is due to using time-difference variables for some of the ANN inputs. The 135Xe concentration was not considered as an input variable to the ANN controller to simplify the controller design. An additional feedback controller was added to compensate the impact of the changes of the 135Xe concentration. The center target strategy, which maintained AO as close as possible to its target value, was applied to control the axial power distribution. In addition, to reduce the total volume of the wastewater, the so-called minimum boron strategy was also applied, which reduced the variations of the boron concentration by permitting AO to vary within a target band.
• Khajavi et al. (2002) presented an ANN-based control scheme for PWR load-following control. For simulation, the PWR is represented with a 1P non-linear model, controlled with a single control rod manipulated variable. The system response obtained with a previous control design based on a robust optimal self-tuning regulator (ROSTR) response is used for training of the ANNs. The ANN-based control scheme comprises 11 single-input-single-output ANNs of the multi-layer perceptron type. The outputs of the ANNs represent 5 feedback gains, 5 observer gains and 1 feedforward gain. The ANNs are trained using the Levenberg-Marquardt method. The computation time using the ANN-based scheme is shorter than the one with the ROSTR method.
• Arab-Alibeik and Setayeshi (2005) proposed an adaptive ANN-based inverse controller for the power of a PWR reactor in the load-following operation. For the process simulation, a 1P non-linear PWR model including 135Xe dynamics is used. A direct-based control approach attempts to attain the best possible closed-loop response in the form of a unity transfer function, where the ANN training effectively produces an inverse plant model. The approach does not appear to consider the issue of the non-minimum phase 135Xe dynamics that do not have a stable inverse. The ANN is of the recursive multi-layer perceptron type. Its output is the control signal for the control rod position, and its inputs are: the power reference signal, the current value and the past values of the power, and the past values of the control signal. The ANN is trained using the Levenberg-Marquardt method. The approach relies on the on-line adaptation of the ANN parameters to compensate for the effects of the noise and the disturbances in the system. Small offsets remain between the power output ant its reference in the steady state.
• Boroushaki et al. (2003, 2004) described an on-line intelligent core controller (ICC) for the load-following operations, based on a heuristic control algorithm, using a recurrent multi-input–multi-output ANN. A 3D core calculation code DYNCO is used to represent the VVER type 320 PWR and to train the ANN. 3 out of 10 control rod groups (CRG) are used to control the PWR power, while the boric acid concentration is kept constant. The macroscopic neutron absorption cross section of the control rods was reduced by the coefficients of 0.5, 0.4, and 0.3, respectively. This ICC includes: an ANN core model (used to predict the core dynamic behaviours), a manoeuvre generator for the CRGs, and a fuzzy critic that considers all of the possible CRGs manoeuvres and proposes an optimum CRGs manoeuvre for the next time interval. The ANN model may be updated with real plant data at any time interval. A multi-layered perceptron topology is used. With the recurrent ANN approach, dynamics are introduced into the model by feeding back past ANN outputs among model inputs, so that the ANN structure corresponds to a NARX structure (Non-linear AutoRegressive with eXogenous inputs) with 4 outputs (135Xe concentration, thermal power and its axial offset, and core reactivity), and 33 inputs. The fuzzy critic is a 3-input fuzzy system with a singleton fuzzifier, a product inference engine with a table of if-then rules, and a centre-of-gravity defuzzifier, using Gaussian membership functions. To avoid the 135Xe oscillations, the ICC is designed to enforce the constant axial offset control strategy, where the normalized axial offset is controlled to within 5% from the target value which depends on the relative power. The fuzzy critic aims to keep the axial offset in the allowed band, rather than controlling it to the exact target value. The constraints of the minimum and maximum overlap between the CRGs are enforced.
• Naimi et al. (2022b) presented a controller based on a recurrent ANN model combining feedback linearisation and MPC. The process, originally represented with a 1P PWR reactor core model without considering the 135Xe dynamics, is identified to an recurrent ANN, trained using a quasi-Newton optimisation algorithm. The ANN is used for feedback linearisation, and the linearised system is controlled using MPC. The described technique was used for five different subsystems: a reactor core-power loop, a steam generator loop, a pressurizer-pressure loop, a pressurizer-level loop, and a turbine-speed loop.
• Khorramabadi et al. (2008) designed a neuro-fuzzy approach to reactor core power control based on the so-called emotional learning. With the emotional learning concept, a fuzzy critic evaluates the present situation, and provides the emotional signal (so-called stress). Then controller parameters are updated so that the critic’s stress is reduced. For system simulation and ANN training, a 1P non-linear model including the 135Xe dynamics is used, and a single control rod is used to regulate the reactor power. The inputs to both the neuro-fuzzy controller and the critic are the power error and its derivative. The neuro-fuzzy controller is based on the Takagi-Sugeno fuzzy system with Gaussian and sigmoidal membership functions, an inference table of the fuzzy rules whose outputs are different linear combinations of the inputs, and the weighted-average defuzzification method. The output of the controller, multiplied by a constant gain, is applied to the control rod actuator. The alternative interpretation of the fuzzy system as a four-layer ANN allows training of the controller from input-output data using optimization algorithms, here the steepest descent method. For the fuzzy critic, a fuzzy system with the singleton fuzzifier, the product fuzzy inference engine and the center average defuzzifier is used. The neuro-fuzzy controller appears to be used in the sense of direct inverse control, and the issue of non-minimum-phase 135Xe dynamics is not discussed.
• Liu et al. (2016) used fuzzy model-based non-linear MPC for control of the reactor core power in a PWR. A Takagi-Sugeno type fuzzy model is used to approximate the 1P non-linear plant (not considering the 135Xe dynamics). The non-linear MPC controller is devised via a parallel distributed compensation (PDC) scheme: for each local model, a local finite-horizon state-space MPC controller is designed. The overall controller is constructed as a weighted sum of the local controllers via fuzzy inference.
• Ejigu and Liu (2022) proposed a deep-ANN-based controller for power control in a PWR, represented with an 1P non-linear model without considering the 135Xe dynamics. The deep neural network is trained using an optimisation algorithm that combines the gradient descent and the particle swarm optimisation. The stability of the system is analyzed using an energy-like Lyapunov function.
• Zeng et al. (2021) described the design of a flexible switching controller for core power control in a small PWR based on a fuzzy model. Firstly, a core fuzzy multi-model suitable for full power range is established, based on a 1P non-linear model without 135Xe dynamics. The model is built for a two-input two-output system, where the outputs are the core relative power deviation and the average coolant temperature deviation, and the inputs are the reactivity and the core coolant inlet temperature. A transfer function matrix is identified in operating points at 5 power levels. The flexible switching controller comprises two modes: a fuzzy controller is used when the power tracking error is relatively large, while a multi-model LQG/LTR controller is used when the error is relatively small.
Hui and Yuan (2022d) described an ANN-based adaptive fault-tolerant controller for load-following of a MHTGR described with a 1P non-linear model without considering the 135Xe dynamics. Fault-tolerant control design was used with the aim of the resilience to the control rod drive mechanism (CRDM) faults. The unknown values of the CRDM fault effects and the lumped model uncertainties were approximated with a radial-basis ANN, which has online-learning capability. A fault-tolerant controller able to provide efficient load-following PWR power control despite the expected CRDM faults was designed using Lyapunov functions.
3.4.1 Control based on models with distributed parameters
Methods in the previous subsections design their control strategies based on the assumption that the control system may be designed using a simplified model with concentrated parameters, in case of PWR control using models with a single node, or two or more axially distributed nodes. However, there is a range of control methods that do not make use of such assumption (at least not in the initial control design stage). For designing PWR control it may be important to consider the detailed distribution of the power along the main reactor axis, rather than just the axial offset between the top and the bottom half of the reactor.
• Winokur et al. (1979) formulated the problem of controlling the spatial flux distribution in a reactor as an optimization problem, with the aim of overcoming the 135Xe oscillations in a load-following operation. A nodal representation of the 3D distributed parameter system that results in a set of non-linear ordinary differential equations is used. A cost functional which numerically integrates deviations of the neutron flux from its required level and the deviations of the 135Xe and 135I concentrations from their respective final values over model nodes and time is introduced. This non-linear optimal control problem is solved in an iterative way by using the Differential Dynamic Programming (DDP) optimisation method, where the neutron flux is assumed to be the virtual control variable. In Winokur and Tepper (1984), the formulation and the results of applying DDP using a 2-node model to optimally follow a daily load-following curve towards nuclear fuel end-of-lifecycle are presented. Full-length control rods, part-length control rods, and boric acid concentration are used as manipulated variables. Axial power distribution control is addressed by controlling the core axial power offset by using the part-length (AO) rods. The purpose of applying the optimal control is to stretch the load-following capability as far as possible beyond where the required boron dilution speed is larger than the one available.
• Cho and Grossman (1983) presented an approach for the control of 1D axial power distribution, addressing 135Xe spatial oscillations in load following operations, via the LQ-optimal state-feedback control. The control is via the full-length and part-length control rod banks, the soluble boron, and the coolant inlet temperature. The control model is derived from a 3D spatial PWR reactor model using the one-group diffusion equation with temperature and 135Xe feedbacks, the 135I-135Xe dynamics equations, and an energy balance relation for the reactor core. The system equations are linearised around an equilibrium state, which is an eigensolution of the non-linear static equations with feedback. The non-linear eigenvalue problem is shown to have a unique positive solution under certain conditions by using the bifurcation theory, the solution being obtained by an iteration based on the use of monotone operators. A modal expansion reduces the linearised equations to a lumped parameter system. A quadratic objective functional that expresses tracking the 1D axial power profile with small control effort is chosen as the performance index. Minimisation of the functional leads to a stiff two-point boundary value problem with boundary layers at both initial and final times, which is solved numerically by the techniques of the initial value methods.
• Ukai et al. (1990) presented an approach for control of the 135Xe axial oscillations during the load-following operating mode of a PWR using a robust servo system. The approach is based on an 1D distributed-parameter model, comprising a one-group diffusion equation with 135Xe and power feedbacks and 135I-135Xe dynamic equations. The model design is based upon finite-dimensional systems constructed by linearising around steady states (with 5 base functions for the simulation model and 2 for the controller design, respectively), and reducing dimensions via the singular perturbation method where derivatives of the fast dynamic modes are set equal to zero. The controlled system has two control inputs, full-length and part-length control rods, and two controlled outputs, the total thermal power and the axial offset. A servo system is designed so that the controlled outputs track their respective demand values, that depend on the load. The feedback gains are determined by either pole placement or the LQ optimal approach, and a state observer is used. A servo compensator with four integrators is used for the purpose of offset-free tracking in the presence of the disturbances and the model uncertainty. The simulations show time evolution of the axial flux distribution. The approach is shown to be robust against the modelling errors due to the linearisation and the modal truncation.
• Ye and Turinsky (1998) described an operating strategy generator (OSG) for automatically determining the optimal control strategies for PWR core maneuvering using optimisation-based control. It is designed for the use with a 3D PWR core simulator NESTLE, but it uses a 1D core model finite-difference code NATO for control optimization to reduce the computation time. The OSG determines optimal rod insertions and boration/dilution operations versus time for the studied manoeuvres by optimising a performance index with various operational objectives and enforcing axial neutron flux difference constraints. The resulting non-linear two-point boundary-value optimisation problem is solved via an iterative approach based on the first-order gradient method. The performance of OSG in various maneuvers at the beginning-of-cycle and the end-of-cycle is compared to the strategies produced using heuristic rules.
• Gondal and Axford (1986) presented a 2D diffusion model in plane geometry, developed for optimal control analysis with 135Xe and 149Sm feedback. The resulting space-time neutron balance equations are reduced to a single space-time algebraic equation by using the 2D Fourier transform. The system equations are linearised around an equilibrium operating point, determined with the steady-state form of the original non-linear system. To determine the optimal spacing between the fuel rods and to see the effect of including the control rods, a poisonless criticality analysis is used. A performance criterion is defined for the 135Xe-induced spatial neutron flux oscillations control problem in the form of a functional to be minimised. The problem is formulated as a LQ regulator problem.
3.5 Experimental studies
In this section, application studies of the load-following strategies to specific PWRs are listed. These studies focus on the operational configuration of the PWRs and maintaining the performance requirements throughout the fuel lifecycle based on detailed models, some including experimental validation, mostly without discussing the control algorithms or using basic industrial control methods.
• Sipush et al. (1976) reported a comprehensive load-follow demonstrations conducted on Consolidated Edison’s Indian Point, Unit 2 NPP. The purpose was to examine the load-following procedures for the PWR developed by Westinghouse. These procedures are based on a constant axial offset (or target neutron flux difference) power-distribution control (CAOC). To demonstrate PWR licensability at the rated power level, it is necessary to show the margins to four limits: loss-of-coolant accident limits during normal operation, departure from nucleate boiling limits in anticipated transients, local power change limits during accident situations, and fuel melting limits in anticipated transients. Operating flexibility demands that the NPP: has the capability to make load changes consistent with the utility system’s needs, should not have unnecessarily complex operating instructions and technical specifications, and has an assured margin between the monitored safety limits and the expected operating conditions. The basic principle is the minimisation of the local power density values by controlling the power distribution during load swings. This is done by maintaining the core axial offset (AO) within a band about a reference target value. Load-follow capability using CAOC was demonstrated for power swings between 100% and 50% of full power with ramp power change rates between 0.25%/min and 5%/min. The power distribution along the axis of the core is not uniform and depends on the state of the fuel. Insertion of the control rods at a constant power level makes this power distribution peak toward the bottom of the core. If fuel depletion is made with relatively deep control rod insertion, the top part of the fuel depletes even less in relation to the bottom part than it would with the rods withdrawn. A skewed power distribution also causes the 135Xe distribution to skew after several hours of operation. The 135Xe distribution at any time is a result of the operating history of the previous ∼ 40 h. The target AO chosen is that AO that would occur at conditions of full power, equilibrium 135Xe, and all rods out. Both the manual and automatic mode of control rod operation were used. In automatic operation, the control rods were adjusted so that the coolant average temperature tracks its set-point (which is a function of power). The control algorithm of the automatic mode is not specified. This automatic mode does not automate the implementation of the CAOC strategy. The latter is implemented by pre-planned manual commands of the operators. Prior to the experimental tests, the CAOC procedures were simulated in Westinghouse design codes such as PANDA, mainly with 1D models and were validated with 3D models. In Mode A of the load-follow operation, four daily load cycles of operation without the use of part-length rods were performed. In this mode, the full-length rods are mainly used for AO correction, and the balance of the reactivity change is then controlled by the operator through changes in the boron concentration. In Mode B, three daily load cycles of operation with the use of part-length rods were carried out. In this mode, the full-length rods are used for the reactivity control, and the part-length rods are used for AO control. The reactivity change due to 135Xe buildup/depletion is followed by the boron concentration. A problematic issue may arise in this mode when the insertion of the full-length rod is shallow, the compensation of the AO demands that the part-length rods are to be moved towards the bottom of the core. This may result in a high peak in the axial power distribution in the centre of the reactor, which cannot be detected with the standard two-section ex-core detector. Such power distributions are not acceptable during full-power operation. Hence, a part-length rod insertion limit curve is used.
• Meyer et al. (1978) proposed a control bank redesign and a modified control strategy to improve the daily load-following capabilities of the Westinghouse PWRs. The use of controlled moderator temperature reductions adds to both the load following and the return-to-power capability through an inherently negative moderator temperature coefficient. The strategy relies on the use of the full-length control rods and also adjusts the boron concentration. The first change involves a redesign of the main control rod bank, decreasing its reactivity worth. The second change involves a widening of the CAOC band and also a skewing of the wider band to the negative side of the target value. This allows using the control rods to the maximum possible extent and a more skewed power distribution (which offsets the reduced peaking exhibited by the reduced worth control bank). At maximum power, the AO is kept near the positive edge of the CAOC band, but at reduced power levels as it approaches the negative edge.
• Onoue et al. (2003) described a new core control strategy for the Westinghouse AP1000 PWR, in which two independently moving rod cluster control assembly (RCCA) groups are utilized for the reactor core control; one group (M-banks) for reactivity/temperature control, the other (AO-bank) for the axial power distribution (axial offset) control. This control procedure eliminates the need for chemical shim adjustments of the boric acid concentration during the load-following maneuvers, and is designated as MSHIM (mechanical shim). The M-banks consists of six control rod banks, moving with a fixed overlap. Reduced-reactivity-worth RCCAs, termed “gray rod”, are utilised for the first four rod groups of the M-banks, while the last two are regular “black rods”. Before any anticipated load-following operation, the first two of the M-bank will have been fully inserted into the core, so that power changing maneuvers in both directions are possible. The AO-bank consists of so called “black rods” and is used during the load-following manoeuvres for AO correction in such way that it always operates in the top region of the PWR and has a monotonic relation between the bank position and the AO. The operation follows the CAOC strategy; the control algorithms are not specified. This core control strategy has been evaluated via computer simulations to provide appropriate margins to core and fuel design limits during normal operation maneuvers and also during anticipated accident transients. Load-follow simulations for 9 daily power-adjustment patterns are presented. It is possible to perform totally automated MSHIM load-following maneuvers for up to 95% of the core cycle life without changing the boron concentration in the moderator.
• Franceschini and Petrovic (2008) applied the CAOC strategy using MSHIM to the International Reactor Innovative and Secure (IRIS) PWR. IRIS has a large inventory of primary coolant, hence a dilution/boration strategy is expensive for short time changes. Therefore, a control bank suitable for the MSHIM strategy has been designed, and the performance was evaluated in nine load-following scenarios covering a wide range of possible operating requirements. The control algorithms are not specified.
• Wei and Zhao (2015) described the power control scheme used for MSHIM control in the Westinghouse AP1000 PWR, and proposed a modified control scheme which uses the error between the reactor coolant average temperature and its reference value as the unique control signal with a P-controller added. The modified MSHIM control strategy is verified by simulations of three typical working conditions using a 14-node non-linear model.
• Zhang et al. (2015) described an improvement of the reactor core control strategy for the CPR1000 PWR for load following operation without changing the soluble boron concentration, inspired by the MSHIM strategy of Westinghouse. The rod cluster control assemblies (RCCAs) are reconfigured with modified reactivity worths with their number and location unchanged. The neutron flux difference between the top and the bottom region of the PWR is kept within the specified target band. More negative values than in regular base-load operation are permitted. For reactor core simulation, a 1D non-linear model is used, which is matched to a 3D model. Compared to the typical MODE-G control strategy (AREVA), the full load-following capability is extended from 80% to more than 90% of the full cycle life, and there is a significant reduction in the daily coolant effluent to be processed. The control algorithms are not discussed.
• Park et al. (2022) presented an approach for control automation in the heat-up mode (hot shutdown to hot standby) of a PWR-based NPP using deep reinforcement learning (DRL) with an actor-critic structure. The compact nuclear simulator (CNS) of the NPP, which models a three-loop Westinghouse PWR, was expanded to enable reinforcement learning. The aim of the heat-up mode is to increase the reactor coolant system temperature and the pressurizer pressure while maintaining the pressurizer level, three steam generator levels, and the pressure difference between the primary system and the secondary steam generators within the prescribed limits. Under manual control, the operator is required to manipulate the spray flow valve and the flow control valve frequently. For the reinforcement learning approach, the simulator was connected to the DRL in (simulated) real-time, and a reward function was defined. The deep neural network was trained from simulated experiments in 3,000 episodes, through which the value of the reward function tended to increase gradually (but not monotonically due to the random actions introduced by the learning process for exploration). The operation testing of the trained DRL showed that the resulting reward rate depended on the stability of the starting state of each initial condition. Lee et al. (2020) describes a prior DRL approach for control automation of a later phase of the NPP startup, for increasing the reactor power from 2% to 100% at a specified rate of power increase. Lee et al. (2022) discussed a DRL approach for automatic cold shutdown operation.
3.5.1 Control for pressurized heavy water reactor (PHWR)
The control design for PHWRs is based on very similar local non-linear dynamic models as the control design for PWRs. Due to the different geometry, the differences in the power profile in the direction of the coolant flow are not so pronounced, and axial offset control is not practised. However, multiple model/control nodes with spatial interaction are used along the plane perpendicular to the coolant flow, demanding a multiple-input multiple-output control system. The power in the nodes is controlled by adjusting their coolant flow control valves. The control rods are perpendicular to the coolant flow axis and are not used for power control in the listed references.
• Talange et al. (2006) described the spatial control of a large pressurized heavy water reactor (PHWR) by decentralized periodic output feedback control. The spatial model of a 540 MWe PHWR with 14 zones comprising 5 state equations results in a 70th-order non-linear system with 14 inputs and outputs. With the introduction of the state variables corresponding to the fuel and the coolant temperatures, the system order increases to 72. Using linearisation and an aggregation technique, a 26th-order linear model with unstable dynamics is obtained. For this model, a stabilizing state-feedback controller is designed. A state observer is designed for the estimation of the unavailable states from the available outputs. As the stability is not guaranteed by static output feedback, the spatial controller is designed by periodically time-varying piecewise constant output feedback which is static in nature and at the same time guarantees complete closed-loop pole assignability. Thus, state feedback controls are designed separately for the slow subsystem and the fast subsystem and then a composite state feedback control is obtained. With conventional design, the periodic output feedback controller yields a gain matrix with a large magnitude. By imposing suitable design constraints and employing a linear-matrix-inequality-based technique, a lower matrix gain is obtained.
• Liu et al. (2016) described a decentralized fuzzy MPC control scheme for spatial power control of a large pressurized heavy water reactor (PHWR). In the PHWR, six zone control compartments (ZCC) are placed at the centre of each corresponding zone and are divided into 14 liquid zone control (LZC) assemblies. The spatial power control of PHWR maintained by adjusting of the control valves of LZCs. A decentralized MPC (DMPC), which decentralizes the decision-making tasks for the systematic handling of constraints, is particularly suitable for controlling this interconnected high-order nodal system. As the state evolution of each nodal system is coupled with the neighboring nodal system, these multiple locally designed controllers must collaborate to stabilise the PHWR. Due to the load dependent dynamics of the nuclear power plant, fuzzy modeling is used to approximate the non-linear process. A fuzzy Lyapunov function and “quasi-min-max” strategy is utilised in designing the DFMPC. The plant-wide stability is achieved by the asymptotically positive realness constraint (APRC) for this decentralized MPC. The solving optimisation problem is based on a receding horizon scheme involving the efficient linear matrix inequalities (LMIs) technique.
4 Open questions and possible future work
In the following subsection, two proposed reference scenarios for load-following operations of nuclear rectors are explicitly defined.
In addition, possibilities for future work include, but are not limited to.
• Taking into account the weather and climate. Definition of more detailed reference scenario(s), which would allow for a flexible production from the renewable energy sources and a flexible consumption, as well as a limited energy storage capacity.
• Modelling of the secondary system response of the NPPs.
• Uncertainties due to nuclear data. However, due to large modelling uncertainties and variations in reactor design, this is a minor factor.
• Development and testing of new control algorithms and strategies, based on modern methods such as e.g. machine learning.
• Bridging the gap between theory and practice. Development and testing of new control algorithms and strategies should be made more relevant to actual reactor operation.
4.1 Reference scenarios for load-following operations
Most of the currently operating Generation II nuclear reactors were designed to have strong manoeuvring capabilities. Some nuclear power plants already operate in load-following mode. They participate in the primary and the secondary frequency control, and some units follow a variable load programme with one or two large power changes per day. However, the minimum requirements for modern Generation III/III + reactors are defined based on the requirements of the grid operators. For example, according to the current version of the European Utility Requirements (EU, 2016), the all newly licensed NPPs must be able to.
• Change the rate of the electrical power up to 3% of the nominal maximum power per minute.
• Operate in the power range from 100% of the maximum nominal power to the minimum operating level of the unit.
• Go through the following number of load scheduled variations, with each change defined as a transient from the full power to the minimum load and back to full power at least:
– 2 times per day;
– 5 times per week; and
– cumulatively 200 times per year.
• Perform scheduled load following and unscheduled load variations during 90% of the whole fuel cycle.
In addition, the following plant-specific requirements are in place.
• The above should be achieved for PWRs without immediate adjustment of the soluble boron concentration during the manoeuvre.
• For evolutionary BWRs, the load following should be achieved as much as possible by controlling the re-circulation flow, i.e. minimising the control rod movements.
• The core design shall provide the flexibility to extend the operation beyond the natural cycle length.
• The designer should also evaluate the technical and the economic impact of shortening the natural length of the fuel cycle.
Based on these requirements, a reference scenario is proposed to test the limitations of the load-following capabilities, i.e. mostly the ability of the system to compensate for build-up of 135Xe. In addition, the scenario is defined to be in accordance with the European Utility Requirements (EU, 2016), i.e. to be capable of changes of up to 3% of nominal power per minute at power levels within the interval (30, 100)% for at least 90% of the operating cycle. The normalised required electric power produced by NPP(s) within a 7-day period is given in Figure 1. Numerical details are provided as Supplementary Material (test_scenario.txt).
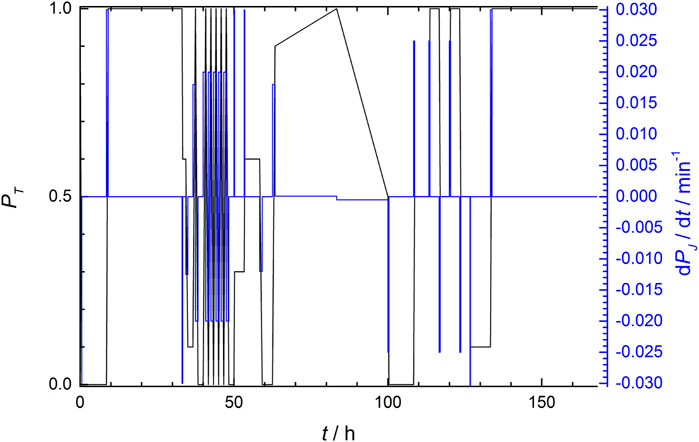
FIGURE 1. Time dependence of the relative power PT for a scenario to test the ability of the system to follow the European Utility Requirements. In blue, the derivative of the power with respect to time is presented.
A second, quasi-realistic scenario with variable shares of solar and wind production, with NPPs trying to follow a production pattern to fit a typical daily electricity demand cycle. On one hand, it is based on realistic data, however, on the other hand, it includes some assumptions that are not necessarily fully reflecting reality, with an aim to be conservative in the sense to demand a harsher than expected flexibility of NPP(s) for load-following. The normalised required electric power produced by NPP(s) within a 14-day period is given in Figure 2 based on the following assumptions.
• Electricity demand is based on typical consumption in the United Kingdom.
• Electricity storage capacity is neglected.
• Electricity produced from solar energy is based on a typical production in November at 45° latitude and peaks at 35% peak demand.
• Electricity produced from wind energy is based on a typical production in November at a windy location in Slovenia and peaks at 35% peak demand.
• Electricity produced by NPP(s) needs to follow the difference between the consumption and the production from other sources at any time.
• Electricity production from energy sources other than solar, wind and nuclear is excluded.
• Both demand and supply are averaged over 10-min time intervals assuming that NPPs would be used for the tertiary grid frequency regulation only.
More details are provided in Supplementary Appendix S1A and as supplementary material (ref_nuclear_norm_035.txt).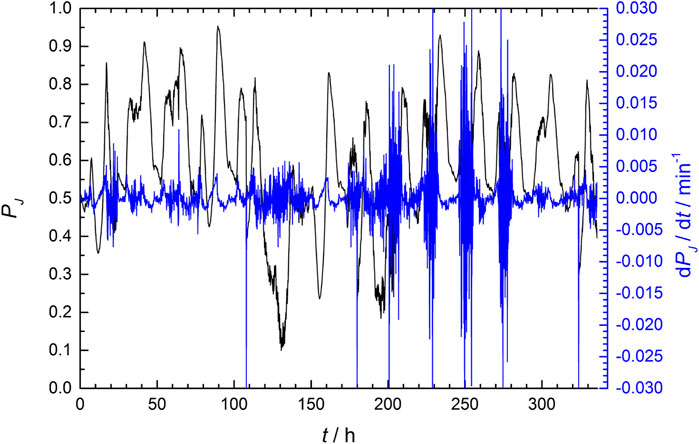
FIGURE 2. Time dependence of the relative power from NPP(s) PJ for the quasi-realistic reference scenario. In blue, the derivative of the power with respect to time is presented.
5 Summary and conclusions
In literature, models and methods with very different degrees of detail and sophistication were used to describe the ability of a nuclear reactor to follow the changes in electric power grid demand. From some aspects, such as e.g., rapid power transients, the limitations for load-following operation are the same as for general nuclear safety and operation requirements. From other aspects, such as e.g., the 135Xe “poisoning”, additional restrictions may result from the load-following operations.
Even as of 2022, a full 3D time-dependent coupled neutron transport, fuel depletion and thermal-hydraulic calculation of a power reactor is too computationally demanding, be it in form of a Monte Carlo simulation or as a deterministic solution of the neutron transport equation. Therefore, different levels of approximation are introduced. In most literature, there is a clear tendency to reduce the reactivity feedback effects, both originating from the thermal-hydraulics and from the fuel depletion, to a set of (linear) differential equations. Similarly, spatial modelling is mostly reduced to 1D, frequently to only 2 regions. On the other hand, the time variable is often treated explicitly taking into account different phenomena over many time scales. Due to crude approximations regarding the spatial variables and reactivity feedback effects, this approach has a limited practical validity for existing reactor systems. It can be useful, however, for comparison studies of performance of different control algorithms, as well as potential qualitative assessments of load-following capabilities of new reactor concepts.
When performing an extensive literature review, another widespread, i.e. not limited to this field of research, issue becomes apparent. Too frequently, openly published data does not meet one of the fundamental requirements of the scientific approach: reproducibility of the results. The lack of provided information can be on different levels. Rarely, the models and/or methods/algorithms are not explained precisely enough. In most cases, the models and algorithms are well explained, however not all input data required to perform calculations are provided. Finally, results published solely in graphical format require some reverse engineering to assign the corresponding numerical values, however essentially with reduced accuracy and fidelity. In order to avoid issues with reproducibility of the results, the following guidelines are strongly recommended for any future publications.
• All models and methods that are not considered general knowledge need to be described explicitly and clearly.
• All input parameters need to be defined. If not in the main text, then e.g., in appendix or as supplementary material.
• All graphically presented results are suggested to be additionally supplemented in a tabular format, e.g., in appendix or as supplementary material.
In order to facilitate and standardise the testing and comparison of different reactor models and control algorithms, two reference scenarios for load-following operations of NPPs are proposed.
• The first reference scenario is primarily intended to test the capability of the system to adhere to the current and future EU regulatory requirements.
• The second reference scenario, the so-called quasi-realistic scenario, is trying to emulate the requirement for the NPPs to follow a production pattern to fit a typical bi-weekly electricity demand cycle in a system with only solar, wind and nuclear electricity production and no storage capacity or flexibility on the side of the electricity consumers.
Within the field of NPP load following operating, many possibilities for future work are open. One direction is to try to study a further integration of the NPPs into the power grid system, taking into account all externally available options and to perform optimisation on the grid rather than the NPP level. This would require much more detailed load-following scenarios which would allow for a flexible production from the renewable energy sources and a flexible consumption, as well as some energy storage capacity. Additionally, modelling of the secondary system response of the NPPs, which was not performed in most study cases available in the literature, would be required. Further possibilities include the development and testing of new control algorithms and strategies based on modern methods, and an uncertainty study to input parameters, such as geometry, material composition, nuclear data, etc.
Author contributions
GŽ was the main contributor to Section 2, conclusions and references, and a major contributor to Section 4. DČ was the main contributor to the introduction and a major contributor to Section 4. SG was the main contributor to Section 3. MK and AT were major contributors to Section 2 and helping with advice and experience. JM was a major contributor with technicalities especially regarding references and giving scientific advice regarding methodology in Section 2. AM was the main contributor to Section 4. LS coordinated the project and writing of the review paper.
Funding
The work was funded by the Slovenian Research Agency (ARRS), project No. L2-2612, and by GEN energija, d.o.o.
Conflict of interest
The authors declare that the research was conducted in the absence of any commercial or financial relationships that could be construed as a potential conflict of interest.
Publisher’s note
All claims expressed in this article are solely those of the authors and do not necessarily represent those of their affiliated organizations, or those of the publisher, the editors and the reviewers. Any product that may be evaluated in this article, or claim that may be made by its manufacturer, is not guaranteed or endorsed by the publisher.
Supplementary material
The Supplementary Material for this article can be found online at: https://www.frontiersin.org/articles/10.3389/fenrg.2023.1111357/full#supplementary-material
Footnotes
1This issue is of regulatory rather than technical nature.
2In reactor designs, where the moderator is homogeneously mixed with fuel, such as in e.g. TRIGA reactors, the moderator temperature feedback effects are faster, i.e. on the same time scales as the fuel temperature feedback.
References
Aboanber, A. E., Nahla, A. A., and Al-Muhiameed, Z. I. (2014). A novel mathematical model for two-energy groups of the point kinetics reactor dynamics. Prog. Nucl. Energy 77, 160–166. ISSN 01491970. doi:10.1016/j.pnucene.2014.06.016
Alten, S., and Danofsky, R. A. (1993). Knowledge base expert system control of spatial xenon oscillations in pressurized water reactors. Ann. Nucl. Energy 20 (10), 691–700. doi:10.1016/0306-4549(93)90051-p
Ansarifar, G. R., and Saadatzi, S. (2015). Nonlinear control for core power of pressurized water nuclear reactors using constant axial offset strategy. Nucl. Eng. Technol. 47, 838–848. ISSN 17385733. doi:10.1016/j.net.2015.09.002
Arab-Alibeik, H., and Setayeshi, S. (2005). Adaptive control of a PWR core power using neural networks. Ann. Nucl. Energy 32, 588–605. ISSN 03064549. doi:10.1016/j.anucene.2004.11.004
Ayodeji, A., Amidu, M. A., Olatubosun, S. A., Addad, Y., and Ahmed, H. (2022). Deep learning for safety assessment of nuclear power reactors: Reliability, explainability, and research opportunities. Prog. Nucl. Energy 151, 104339. ISSN 0149-1970. doi:10.1016/j.pnucene.2022.104339
Bauer, D. C., and Poncelet, C. G. (1974). Practical xenon spatial control. Nucl. Technol. 21 (3), 165–189. doi:10.13182/NT74-A31388
Bell, G. I., and Glasstone, S. (1970). Nuclear reactor theory. New York: Van Nostrand Reinhold Company.
Ben-Abdennour, A., Edwards, R. M., and Lee, K. Y. (1992). LQG/LTR robust control of nuclear reactors with improved temperature performance. IEEE Trans. Nucl. Sci. 39, 2286–2294. doi:10.1109/23.211438
Berkan, R. C., Upadhyaya, B. R., Tsoukalas, L. H., and Reconstructive, R. A. Kisner. (1991). Reconstructive inverse dynamics control and application to xenon-induced power oscillations in pressurized water reactors. Nucl. Sci. Eng. 109 (2), 188–199. doi:10.13182/NSE91-A28517
Boroushaki, M., Ghofrani, M. B., Lucas, C., and Yazdanpanah, M. J. (2003). An intelligent nuclear reactor core controller for load following operations, using recurrent neural networks and fuzzy systems. Ann. Nucl. Energy 30, 63–80. doi:10.1016/s0306-4549(02)00047-6
Boroushaki, M., Ghofrani, M. B., Lucas, C., Yazdanpanah, M. J., and Sadati, N. (2004). Axial offset control of PWR nuclear reactor core using intelligent techniques. Nucl. Eng. Des. 227, 285–300. ISSN 00295493. doi:10.1016/j.nucengdes.2003.11.002
Borowiec, K., Wysocki, A., Shaner, S., Greenwood, M. S., and Ellis, M. (2019). Increasing revenue of nuclear power plants with thermal storage. J. Energy Resour. Technol. 142 (4). ISSN 0195-0738. doi:10.1115/1.4044800
Bragg-Sitton, S. M., Boardman, R., Rabiti, C., and O’Brien, J. (2020). Reimagining future energy systems: Overview of the US program to maximize energy utilization via integrated nuclear-renewable energy systems. Int. J. Energy Res. 44 (10), 8156–8169. doi:10.1002/er.5207
Brown, D., Chadwick, M., Capote, R., Kahler, A., Trkov, A., Herman, M., et al. (2018). ENDF/B-VIII.0: The 8 th major release of the nuclear reaction data library with CIELO-project cross sections, new standards and thermal scattering data. Nucl. Data Sheets 148, 1–142. ISSN 00903752. doi:10.1016/j.nds.2018.02.001
Ćalić, D., Trkov, A., Kromar, M., and Snoj, L. (2016). Use of effective diffusion homogenization method with the Monte Carlo code for light water reactor. Ann. Nucl. Energy 94, 392–398. ISSN 18732100. doi:10.1016/j.anucene.2016.04.008
Canosa, J., and Brooks, H. (1966). Xenon-induced oscillations. Nucl. Sci. Eng. 26 (2), 237–253. ISSN 0029-5639. doi:10.13182/nse66-a28166
Chae, S. K. (1979). Review of computational methods for space-time reactor kinetics. J. Korean Nucl. Soc. 11 (3), 219–229.
Chakraborty, A., Singh, S., and Fernando, M. (2018). A novel approach for bifurcation analysis of out of phase xenon oscillations using multipoint reactor kinetics. Nucl. Eng. Des. 328, 333–344. ISSN 00295493. doi:10.1016/j.nucengdes.2017.12.037
Chakraborty, A., Singh, S., and Fernando, M. P. (2019). An improved reduced order model for nonlinear stability analysis of spatial xenon oscillations. Prog. Nucl. Energy 116, 62–75. ISSN 01491970. doi:10.1016/j.pnucene.2019.03.04310.1016/j.pnucene.2019.03.043
Chang, G. S. (2016). Advanced CANDU reactors axial xenon oscillation controllability validation. Ann. Nucl. Energy 96, 441–445. ISSN 18732100. doi:10.1016/j.anucene.2016.06.03910.1016/j.anucene.2016.06.039
Che, Y., Yurko, J., Seurin, P., and Shirvan, K. (2022). Machine learning-assisted surrogate construction for full-core fuel performance analysis. Ann. Nucl. Energy 168, 108905108905. ISSN 0306-4549. doi:10.1016/j.anucene.2021.108905
Cho, B. O., Joo, H. G., Cho, J. Y., and Zee, S. Q. (2002). “Master: Reactor core design and analysis code,” in Proc. PHYSOR 2002 int. Conf. New Frontiers of nuclear Technology: Reactor physics, safety and high-performance computing (American Nuclear Society).
Cho, N. Z., and Grossman, L. M. (1983). Optimal control for xenon spatial oscillations in load follow of a nuclear reactor. Nucl. Sci. Eng. 83 (1), 136–148. doi:10.13182/NSE83-A17995
Christie, A. M., and Poncelet, C. G. (1973). On the control of spatial xenon oscillations. Nucl. Sci. Eng. 51 (1), 10–24. doi:10.13182/NSE73-A23253
Demazière, Christophe, Sanchez-Espinoza, V. H., Hugo, Victor, and Chanaron, Bruno (2020). Advanced numerical simulation and modelling for reactor safety - contributions from the CORTEX, HPMC, McSAFE and NURESAFE projects. EPJ Nucl. Sci. Technol. 6, 42. . doi:10.1051/epjn/201900610.1051/epjn/2019006
Denholm, P., King, J. C., Kutcher, C. F., and Wilson, P. P. (2012). Decarbonizing the electric sector: Combining renewable and nuclear energy using thermal storage. Energy Policy 44, 301–311. ISSN 0301-4215. doi:10.1016/j.enpol.2012.01.055
Domingos, R. P., Caldas, G. H., Pereira, C. M., and Schirru, R. (2003). PWR’s xenon oscillation control through a fuzzy expert system automatically designed by means of genetic programming. Appl. Soft Comput. J. 3, 317–323. ISSN 15684946. doi:10.1016/j.asoc.2003.05.002
Duderstadt, J. J., and Hamilton, L. J. (1976). Nuclear reactor analysis. John Wiley and Sons. 0-471-22363-8.
Dzianisau, S., Lee, H. C., and Lee. Development of Rast-Ai, D. (2022). A hybrid nodal diffusion/artificial neural network code for reactor analysis. SSRN. ISSN 1556-5068. doi:10.2139/ssrn.4207485
Edwards, R. M., Lee, K. Y., and Schultz, M. A. (1990). State feedback assisted classical control. an incremental approach to control modernization of existing and future nuclear reactors and power plants. Nucl. Technol. 92, 167–185. ISSN 00295450. doi:10.13182/NT90-A34468
Ejigu, D. A., and Liu, X. (2022). Gradient descent-particle swarm optimization based deep neural network predictive control of pressurized water reactor power. Prog. Nucl. Energy 145, 104108. ISSN 0149-1970. doi:10.1016/j.pnucene.2021.104108
El-Bassioni, A. A., and Poncelet, C. G. (1974). Minimal time control of spatial xenon oscillations in nuclear power reactors. Nucl. Sci. Eng. 54 (2), 166–176. doi:10.13182/NSE74-A23404
Eliasi, H., Menhaj, M. B., and Davilu, H. (2011). Robust nonlinear model predictive control for nuclear power plants in load following operations with bounded xenon oscillations. Nucl. Eng. Des. 241, 533–543. ISSN 00295493. doi:10.1016/j.nucengdes.2010.12.004
Elsisi, M., and Abdelfattah, H. (2020). New design of variable structure control based on lightning search algorithm for nuclear reactor power system considering load-following operation. Nucl. Eng. Technol. 52, 544–551. ISSN 2234358X. doi:10.1016/j.net.2019.08.003
Evans, J. A., DeHart, M. D., Weaver, K. D., and Keiser, D. D. (2022). Burnable absorbers in nuclear reactors – a review. Nucl. Eng. Des. 391, 111726. ISSN 00295493 doi. doi:10.1016/j.nucengdes.2022.11172610.1016/j.nucengdes.2022.111726
Franceschini, F., and Petrovic, B. (2008). Advanced operational strategy for the iris reactor: Load follow through mechanical shim (mshim). Nucl. Eng. Des. 238 (12), 3240–3252. ISSN 00295493. doi:10.1016/j.nucengdes.2008.06.021
Gomez-Fernandez, M., Higley, K., Tokuhiro, A., Welter, K., Wong, W.-K., and Yang, H. (2020). Status of research and development of learning-based approaches in nuclear science and engineering: A review. Nucl. Eng. Des. 359, 110479. ISSN 0029-5493. doi:10.1016/j.nucengdes.2019.110479
Gondal, I. A., and Axford, R. A. (1986). Optimal xenon control in heterogeneous reactors. IEEE Trans. Nucl. Sci. 33 (6), 1722–1729. doi:10.1109/tns.1986.4334678
Gyorey, G. L. (1962). The effect of modal interaction in the xenon instability problem. Nucl. Sci. Eng. 13 (4), 338–344. ISSN 0029-5639. doi:10.13182/nse62-a26175
Hassan, S., Khan, A. H., Verma, R., Kumar, D., Kobayashi, K., Usman, S., et al. (2021). Machine Learning and Artificial Intelligence-Driven Multi-Scale Modeling for High Burnup Accident-Tolerant Fuels for Light Water-Based SMR Applications. Cham: Springer International Publishing, 1–24. 978-3-030-72322-4.
Hetrick, D. L. (1971). Dynamics of nuclear reactors. Chicago and London: University of Chicago Press. 978-0226331669.
Hu, G., Zhou, T., and Liu, Q. (2021). Data-driven machine learning for fault detection and diagnosis in nuclear power plants: A review. Front. Energy Res. 9. ISSN 2296-598X. doi:10.3389/fenrg.2021.663296
Hui, J., Ge, S., Ling, J., and Yuan, J. (2020). Extended state observer-based adaptive dynamic sliding mode control for power level of nuclear power plant. Ann. Nucl. Energy 143, 107417. ISSN 18732100. doi:10.1016/j.anucene.2020.107417
Hui, J., and Yuan, J. (2022b). Adaptive second-order nonsingular terminal sliding mode power-level control for nuclear power plants. Nucl. Eng. Technol. 54 (5), 1644–1651. 2022b. ISSN 2234358X. doi:10.1016/j.net.2021.10.041
Hui, J., and Yuan, J. (2021). Chattering-free higher order sliding mode controller with a high-gain observer for the load following of a pressurized water reactor. Energy 223, 12006603605442. doi:10.1016/j.energy.2021.120066
Hui, J., and Yuan, J. (2022c). High-gain disturbance observer-based double closed-loop sliding mode controller for the load following of a pressurized-water reactor. Prog. Nucl. Energy 150, 10432010432001491970. doi:10.1016/j.pnucene.2022.104320
Hui, J., and Yuan, J. (2022a). Load following control of a pressurized water reactor via finite-time super-twisting sliding mode and extended state observer techniques. Energy 241, 12283603605442. doi:10.1016/j.energy.2021.122836
Hui, J., and Yuan, J. (2022d). Neural network-based adaptive fault-tolerant control for load following of a MHTGR with prescribed performance and CRDM faults. Energy 257, 12466303605442. doi:10.1016/j.energy.2022.124663
IAEA (2018). IAEA nuclear energy series @ non-baseload operation in nuclear power plants: Load following and frequency control modes of flexible operation. IAEA. Technical report Available at: http://www.iaea.org/Publications/index.html.
Kastin, N., Meron, E., Kolin, A., and Kinast, S. (2019). Nonlinear stability and limit cycles in xenon-induced reactor oscillations. Prog. Nucl. Energy 116, 168–179. ISSN 01491970 doi. doi:10.1016/j.pnucene.2019.03.02310.1016/j.pnucene.2019.03.023
Keepin, G. (1965). Physics of nuclear kinetics. Reading, Palo Alto, London: Addison-Wesley Publishing Company, Inc.
Khajavi, M. N., Menhaj, M. B., and Suratgar, A. A. (2002). A neural network controller for load following operation of nuclear reactors. Ann. Nucl. Energy 29, 751–760. doi:10.1016/s0306-4549(01)00075-5
Khorramabadi, S. S., Boroushaki, M., and Lucas, C. (2008). Emotional learning based intelligent controller for a PWR nuclear reactor core during load following operation. Ann. Nucl. Energy 35, 2051–2058. ISSN 03064549. doi:10.1016/j.anucene.2008.05.014
Kim, J. H., and Alameri, S. A. (2020). Harmonizing nuclear and renewable energy: Case studies. Int. J. Energy Res. 44 (10), 8053–8061. doi:10.1002/er.4987
Kobayashi, K., and Yoshikuni, M. (1982). Analysis of xenon oscillation by coupled reactor model. J. Nucl. Sci. Technol. 19 (2), 107–118. ISSN 00223131. doi:10.1080/18811248.1982.9734123
Kollias, S., Yu, M., Wingate, J., Durrant, A., Leontidis, G., Alexandridis, G., et al. (2022). Machine learning for analysis of real nuclear plant data in the frequency domain. Ann. Nucl. Energy 177, 109293109293. ISSN 0306-4549. doi:10.1016/j.anucene.2022.109293
Lee, D., Arigi, A. M., and Kim, J. (2020). Algorithm for autonomous power-increase operation using deep reinforcement learning and a rule-based system. IEEE Access 8, 196727–196746. doi:10.1109/ACCESS.2020.3034218
Lee, D., Koo, S., Jang, I., and Kim, J. (2022). Comparison of deep reinforcement learning and PID controllers for automatic cold shutdown operation. Energies 15 (8), 2834. ISSN 1996-1073. doi:10.3390/en15082834
Lee, S. W., Kim, J. H., Na, M. G., Kim, D. S., Yu, K. J., and Kim, H. G. (2012). Design of a load following controller for APR+ nuclear plants. Nucl. Eng. Technol. 44, 369–378. ISSN 17385733. doi:10.5516/NET.04.2012.509
Lellouche, G. S. (1962). Space dependent xenon oscillations. Nucl. Sci. Eng. 12 (4), 482–489. ISSN 0029-5639. doi:10.13182/nse62-a26095
Li, G. (2014a). Extension for load-follow operation of PWR core by using single- or multi-variable control of state-feedback. Ann. Nucl. Energy 68, 183–192. ISSN 03064549. doi:10.1016/j.anucene.2014.01.023
Li, G. (2014b). Modeling and LQG/LTR control for power and axial power difference of load-follow PWR core. Ann. Nucl. Energy 68, 193–203. ISSN 03064549. doi:10.1016/j.anucene.2014.01.022
Li, G., Wan, J., and Zhao, F. (2014a). New strategies with multi-model, state-feedback control and stability analysis for load-follow PWR core. Prog. Nucl. Energy 75, 168–179. ISSN 01491970. doi:10.1016/j.pnucene.2014.04.019
Li, G., Wan, J., and Zhao, F. (2014b). New strategies with multi-model, state-feedback control and stability analysis for load-follow PWR core. Prog. Nucl. Energy 75, 168–179. ISSN 01491970. doi:10.1016/j.pnucene.2014.04.019
Li, G., and Zhao, F. (2013b). Flexibility control and simulation with multi-model and LQG/LTR design for PWR core load following operation. Ann. Nucl. Energy 56, 179–188. ISSN 03064549. doi:10.1016/j.anucene.2013.01.035
Li, G., and Zhao, F. (2013a). Load following control and global stability analysis for PWR core based on multi-model, LQG, IAGA and flexibility idea. Prog. Nucl. Energy 66, 80–89. ISSN 01491970. doi:10.1016/j.pnucene.2013.03.015
Li, G., and Zhao, F. (2014). Multimodel-based power-level control with state-feedback and observer for load-follow PWR core. Ann. Nucl. Energy 63, 696–710. ISSN 03064549. doi:10.1016/j.anucene.2013.09.021
Lin, C., and Shen, C.-m. (2000). Neurocontrol of pressurized water reactors in load-follow operations. Nucl. Technol. 132 (3), 389–402. doi:10.13182/NT00-4
Liu, X., Jiang, D., and LeeDecentralized, K. Y. (2016). Decentralized fuzzy MPC on spatial power control of a large PHWR. IEEE Trans. Nucl. Sci. 63, 2343–2351. ISSN 00189499. doi:10.1109/TNS.2016.2580558
Liu, X., and Wang, M. (2014). Nonlinear fuzzy model predictive control for a PWR nuclear power plant. Math. Problems Eng. 2014, 1–10. ISSN 15635147. doi:10.1155/2014/908526
Marseguerra, M., Zio, E., and Torri, G. (2003). Power density axial oscillations induced by xenon dynamics: Parameter identification via genetic algorithms. Prog. Nucl. Energy 43 (1-4), 365–372. ISSN 01491970. doi:10.1016/S0149-1970(03)00049-0
Mascolino, V. (2021). “Development and benchmarking of advanced FM-based particle transport algorithms for steady-state and transient conditions,” in RAPID and its VRS web-application. PhD thesis. Blacksburg: University of Virginia.
Mascolino, V., and Haghighat, A. (2019). Validation of the transient fission matrix code tRAPID against the Flattop-Pu benchmark. Int. Conf. M&C 2019.
Merljak, V., Kromar, M., and Trkov, A. (2017). Verification of kinetic capabilities of the GNOMER neutron diffusion code. Ann. Nucl. Energy 109, 431–439. ISSN 18732100. doi:10.1016/j.anucene.2017.04.037
Meyer, C. E., Bennett, C. L., Hill, D. J., and Dzikowski, K. J. (1978). Improved load follow strategy for return-to-power capability. Nucl. Technol. 41, 27–35. ISSN 00295450. doi:10.13182/NT78-A32130
Moon, H. J., and Han, K. I. (1982). PWR core stability against xenon-induced spatial power oscillation. J. Korean Nucl. Soc. 14 (2), 51–63.
Mousakazemi, S. M. H. (2019). Control of a PWR nuclear reactor core power using scheduled PID controller with GA, based on two-point kinetics model and adaptive disturbance rejection system. Ann. Nucl. Energy 129, 487–502. ISSN 18732100. doi:10.1016/j.anucene.2019.02.019
Na, M. G. (2001). Design of a receding horizon control system for nuclear reactor power distribution. Nucl. Sci. Eng. 138 (3), 305–314. doi:10.13182/NSE01-A2216
Na, M. G., Jung, D. W., Shin, S. H., Jang, J. W., Lee, K. B., and Lee, Y. J. (2005). A model predictive controller for load-following operation of PWR reactors. IEEE Trans. Nucl. Sci. 52 (4), 1009–1020. ISSN 00189499. doi:10.1109/TNS.2005.852651
Na, M. G., Jung, D. W., Shin, S. H., Lee, S. M., Lee, Y. J., Jang, J. W., et al. (2004). Design of a nuclear reactor controller using a model predictive control method. KSME Int. J. 18 (12), 2080–2094. doi:10.1007/bf02990213
Na, M. G., Upadhyaya, B. R., and Choi, J. I. (1998a). Adaptive control for axial power distribution in nuclear reactors. Nucl. Sci. Eng. 129 (3), 283–293. doi:10.13182/NSE98-A1982
Na, M. G., Upadhyaya, B. R., and Member, S. (1998b). A neuro-fuzzy controller for axial power distribution in nuclear reactors. IEEE Trans. Nucl. Sci. 45 (59).
Naimi, A., Deng, J., Doney, P., Sheikh-Akbari, A., Shimjith, S. R., and Arul, A. J. (2022a). Machine learning-based fault diagnosis for a PWR nuclear power plant. IEEE Access 10, 126001–126010. 2022a. ISSN 2169-3536. doi:10.1109/ACCESS.2022.3225966
Naimi, A., Deng, J., Vajpayee, V., Becerra, V., Shimjith, S. R., and Arul, A. J. (2022b). Nonlinear model predictive control using feedback linearization for a pressurized water nuclear power plant. IEEE Access 10, 16544–16555. doi:10.1109/ACCESS.2022.3149790
Nair, P. P., and Gopal, M. (1987). Sensitivity-reduced design for a nuclear pressurized water reactor. IEEE Trans. Nucl. Sci. 34, 1834–1842. doi:10.1109/tns.1987.4334825
Obaidurrahman, K., Doshi, J. B., Jain, R. P., and Jagannathan, V. (2010). Development and validation of coupled dynamics code ’TRIKIN’ for VVER reactors. Nucl. Eng. Technol. 42, 259–270. doi:10.5516/net.2010.42.3.259
Obaidurrahman, K., and Doshi, J. B. (2011). Spatial instability analysis in pressurized water reactors. Ann. Nucl. Energy 38, 286–294. ISSN 03064549 doi. doi:10.1016/j.anucene.2010.10.01510.1016/j.anucene.2010.10.015
OECD (2011). Technical and economic aspects of load following with nuclear power plants. OECD. Technical report. Available at: www.oecd-nea.org.
Oka, Y., and Suzuki, K. (2013). Nuclear reactor kinetics and plant control. Springer. Available at: https://link.springer.com/book/10.1007/978-4-431-54195-0.
Onega, R. J., and Kisner, R. A. (1978). An axial xenon oscillation model. Ann. Nucl. Energy 5 (13–19), 13–19. doi:10.1016/0306-4549(78)90056-7
Onoue, M., Kawanishi, T., Carlson, W. R., and Morita, T. (2003). “Application of MSHIM core control strategy for Westinghouse AP1000 nuclear power plant,” in GENES4/ANP2003: Int. conf. on global environment and advanced nuclear power plants (Tokyo (Japan)Tokyo (Japan): Tokyo Univ.Tokyo Institute of Technology), Vol. 15, 16759.
Ott, K. O. K. O., and Neuhold, R. J. (1985). Introductory nuclear reactor dynamics. American Nuclear Society, 0894480294.
Parhizkari, H., Aghaie, M., Zolfaghari, A., and Minuchehr, A. (2015). An approach to stability analysis of spatial xenon oscillations in WWER-1000 reactors. Ann. Nucl. Energy 79, 125–132. ISSN 18732100. doi:10.1016/j.anucene.2015.01.026
Park, J., Kim, T., Seong, S., and Koo, S. (2022). Control automation in the heat-up mode of a nuclear power plant using reinforcement learning. Prog. Nucl. Energy 145, 104107. ISSN 0149-1970. doi:10.1016/j.pnucene.2021.104107
Park, M. G., and Cho, N. Z. (1992). Design of a nonlinear model-based controller with adaptive PI gains for robust control of a nuclear reactor. Prog. Nucl. Energy 27 (1), 37–49. doi:10.1016/0149-1970(92)90016-v
Park, M. G., and Cho, N. Z. (1993). Time-optimal control of nuclear reactor power with adaptive proportional-integral-feedforward gains. IEEE Trans. Nucl. Sci. 40, 266–270. doi:10.1109/23.221049
Plompen, A., Cabellos, O., De Saint Jean, C., Fleming, M., Bauge, E., Bersillon, O., et al. (2020). The joint evaluated fission and fusion nuclear data library, JEFF-3.3. EPJ Nucl. Sci. Technol. 56, 181. doi:10.1140/epja/s10050-020-00141-9
Pradhan, S. K., Obaidurrahman, K., Iyer, K. N., and Gaikwad. Development, A. J. (2016). Development, validation and application of multi-point kinetics model in RELAP5 for analysis of asymmetric nuclear transients. Nucl. Eng. Des. 300, 506–516. ISSN 00295493 doi. doi:10.1016/j.nucengdes.2016.01.02810.1016/j.nucengdes.2016.01.028
Racheal, S., Liu, Y., and Ayodeji, A. (2022). Evaluation of optimized machine learning models for nuclear reactor accident prediction. Prog. Nucl. Energy 149, 104263. ISSN 0149-1970. doi:10.1016/j.pnucene.2022.104263
Randall, D., and John, D. S. S. (1962). Xenon spatial oscillations. Nucl. Sci. Eng. 14 (2), 204–206. ISSN 0029-5639. doi:10.13182/nse62-a28124
Ronchi, C., Sheindlin, M., Staicu, D., and Kinoshita, M. (2004). Effect of burn-up on the thermal conductivity of uranium dioxide up to 100.000 mwdt-1. J. Nucl. Mater. 327, 58–76. ISSN 00223115. doi:10.1016/j.jnucmat.2004.01.018
Saadatzi, S., and Ansarifar, G. (2017). Robust observer-based non-linear control for PWR nuclear reactors with bounded xenon oscillations during load-following operation using two-point nuclear reactor model. Int. J. Nucl. Energy Sci. Technol. 11 (1), 22–55. doi:10.1504/ijnest.2017.085075
Schulz, E. J., and Lee, J. C. (1980). Time-optimal control of spatial xenon oscillations to a generalized target. Nucl. Sci. Eng. 73 (2), 140–152. doi:10.13182/NSE80-A18694
Shibata, K., Iwamoto, O., Nakagawa, T., Iwamoto, N., Ichihara, A., Kunieda, S., et al. (2011). JENDL-4.0: A new library for nuclear science and engineering. J. Nucl. Sci. Technol. 48 (1), 1–30. ISSN 00223131. doi:10.1080/18811248.2011.9711675
Shimazu, Y. (1995). Continuous guidance procedure for xenon oscillation control. J. Nucl. Sci. Technol. 32 (2), 95–100. ISSN 00223131. doi:10.1080/18811248.1995.9731678
Shimjith, S. R., Tiwari, A. P., Naskar, M., and Bandyopadhyay, B. (2010). Space-time kinetics modeling of advanced heavy water reactor for control studies. Ann. Nucl. Energy 37, 310–324. ISSN 03064549 doi. doi:10.1016/j.anucene.2009.12.01110.1016/j.anucene.2009.12.011
Shotkin, L. M., and Abernathy, F. H. (1963). Linear stability of the thermal flux in a reflected core containing xenon and temperature reactivity feedback. Nucl. Sci. Eng. 15 (2), 197–212. ISSN 0029-5639. doi:10.13182/nse63-a26419
Sipush, P. J., Kerr, R. A., Ginsberg, A. P., Morita, T., and Scherpereel, L. R. (1976). Load follow demonstrations employing constant axial offset power distribution control procedures. Nucl. Technol. 31, 12–31. ISSN 00295450 doi:10.13182/NT76-A31695
Smith, K. S. (2007). SIMULATE-3 advanced three-dimensional two-group reactor analysis code. Technical report. Nyköping: Studsvik Scandpower Inc.
Song, J. S., and Cho, N. Z. (1997). Two-group, flux-coupled xenon oscillation model with an equation system of axial difference parameters. Nucl. Technol. 119 (2), 105–111. ISSN 00295450. doi:10.13182/NT97-A35379
Song, J. S., Cho, N. Z., and Zee, S. Q. (1999). Analytic modeling of the xenon oscillation due to control rod movement. J. Korean Nucl. Soc. 31 (1), 80–87.
Sutton, T. M., and Aviles, B. N. (1996). Diffusion theory methods for spatial kinetics calculations. Prog. Nucl. Energy 30 (2), 119–182. ISSN 01491970. doi:10.1016/0149-1970(95)00082-U
Talange, D. B., Bandyopadhyay, B., and Tiwari, A. P. (2006). Spatial control of a large PHWR by decentralized periodic output feedback and model reduction techniques. IEEE Trans. Nucl. Sci. 53 (4), 2308–2317. doi:10.1109/tns.2006.877154
Teachman, J. D., and Onega, R. J. (1983). The influence of energy group structure and nonlinearities on the calculation of xenon-induced flux oscillations. Nucl. Sci. Eng. 81 (1), 149–161. doi:10.13182/NSE83-A17996
Tiwari, A. P., Bandyopadhyay, B., and Govindarajan, G. (1996). Spatial control of a large pressurized heavy water reactor. IEEE Trans. Nucl. Sci. 43 (4), 2440–2453. ISSN 00189499. doi:10.1109/23.531794
Torabi, K., Safarzadeh, O., and Rahimi-Moghaddam, A. (2011). Robust control of the PWR core power using quantitative feedback theory. IEEE Trans. Nucl. Sci. 58, 258–266. ISSN 00189499. doi:10.1109/TNS.2010.2094207
Trkov, A. (1994). Gnomer – multigroup 3-dimensional neutron diffusion nodal code with thermohydraulic feedbacks. Ljubljana, Slovenia: Jožef Stefan Institute. Technical Report IJS-DP-6688, Rev. 1.
Trkov, A., and Kromar, M. (1998). Program LOADF_GNOMER za spremljanje sredice na PIS-NEK sistemu; izdelava DSFS dokumenta. Ljubljana, Slovenia: Jožef Stefan Institute. Technical Report IJS-DP-7854.
Trkov, A., Kromar, M., and žefran, B. (1998). “Load follow software development for the Krško NPP process computer,” in Nuclear energy in central europe ’98 (Ljubljana: Nuclear Society of Slovenia), 93–98.
Trkov, A., and Kurinčič, B. (2001). “On the use of Loadf code for optimisation of the Krško power plant operation,”. International conference nuclear energy in central europe 2001. Editors I. Jenčič, and B. Glumac (Portorož, Slovenia: Nuclear Society of Slovenia), 104.1–104.8.
Trkov, A. (1998). LOADF_GNOMER za spremljanje sredice na PIS-NEK sistemu. Ljubljana, Slovenia: Jožef Stefan Institute. izdelava ug dokumenta. Technical Report IJS-DP-7947.
Trkov, A. (1997). LOADF_GNOMER za spremljanje sredice na PIS-NEK sistemu; načrt izvedbe projekta. Ljubljana, Slovenia: Jožef Stefan Institute. Technical Report IJS-DP-7747.
Trkov, A., and Ravnik, M. (1994). Effective diffusion homogenization of cross sections for pressurized water reactor core calculations. Nucl. Sci. Eng. 116, 86–95. doi:10.13182/nse94-a21485
Trkov, A. (2012). Verifikacija loadf programa na tranzientu nek na 34 % moči. Ljubljana, Slovenia: Jožef Stefan Institute. Technical Report IJS-DP-11014.
Ukai, H., Morita, Y., Yada, Y., and Iwazumi, T. (1990). Control of xenon spatial oscillations during load follow of nuclear reactor via robust servo systems. J. Nucl. Sci. Technol. 27 (4), 307–319. doi:10.1080/18811248.1990.9731189
Walters, W., Roskoff, N., and Haghighat, A. (2015). A fission matrix approach to calculate pin-wise 3D fission density distribution. Int. Conf. M&C 2015.
Wang, G., Wu, J., Zeng, B., Xu, Z., and Ma, X. (2019). A nonlinear adaptive sliding mode control strategy for modular high-temperature gas-cooled reactors. Prog. Nucl. Energy 113 (381), 53–61. ISSN 01491970. doi:10.1016/j.pnucene.2019.01.006
Wang, G., Wu, J., Zeng, B., Xu, Z., Wu, W., and Ma, X. (2017). State-space model predictive control method for core power control in pressurized water reactor nuclear power stations. Nucl. Eng. Technol. 49, 134–140. ISSN 2234358X. doi:10.1016/j.net.2016.07.008
Wei, X., and Zhao, F. (2015). Study of the reactor control system of mshim in ap1000, 2015. Science and Technology of Nuclear Installations. ISSN 16876075. doi:10.1155/2015/620205
Winokur, M., Blank, D., and Tepper, L. (1979). A method of optimization of flux and xenon fluctuations in a nuclear reactor. IEEE Trans. Nucl. Sci. 26, 791–795. –795ISSN 15581578. doi:10.1109/TNS.1979.4329728
Winokur, M., and Tepper, L. (1984). Extension of load follow capability of a PWR reactor by optimal control. IEEE Trans. Nucl. Sci. 31, 932–939. ISSN 15581578. doi:10.1109/TNS.1984.4333401
Yadav, D. K., Gupta, A., and Munshi, P. (2018). Non linear dynamic inversion based controller design for load following operations in Pressurized Water Reactors with bounded xenon oscillations. Nucl. Eng. Des. 328, 241–254. ISSN 00295493. doi:10.1016/j.nucengdes.2018.01.012
Ye, J., and Turinsky, P. J. (1998). Pressurized water reactor core maneuvering utilizing optimal control theory. Nucl. Sci. Eng. 129 (2), 97–123. doi:10.13182/NSE98-A1967
Yim, M.-S., and Christenson, J. M. (1992). Application of optimal control theory to a load-following pressurized water reactor. Nucl. Technol. 100 (3), 361–377. doi:10.13182/NT92-A34731
Yoon, M. H., and No, H. C. (1985). Direct numerical technique of mathematical programming for optimal control of xenon oscillation in load following operation. Nucl. Sci. Eng. 90 (2), 203–209. doi:10.13182/NSE85-A17677
Zaidabadi nejad, M., and Ansarifar, G. R. (2017). Adaptive robust control for axial offset in the P.W.R nuclear reactors based on the multipoint reactor model during load-following operation. Ann. Nucl. Energy 103, 251–264. ISSN 18732100. doi:10.1016/j.anucene.2017.01.025
Zaidabadi nejad, M., and Ansarifar, G. R. (2018). Robust feedback-linearization control for axial power distribution in pressurized water reactors during load-following operation. Nucl. Eng. Technol. 50, 97–106. ISSN 2234358X. doi:10.1016/j.net.2017.10.013
Zarei, M., Ghaderi, R., and Minuchehr, A. (2016). Space independent xenon oscillations control in VVER reactor: A bifurcation analysis approach. Prog. Nucl. Energy 88, 19–27. ISSN 01491970. doi:10.1016/j.pnucene.2015.11.018
Zeng, W., Jiang, Q., Du, S., Hui, T., Liu, Y., and Li, S. (2021). Design of the flexible switching controller for small PWR core power control with the multi-model. Nucl. Eng. Technol. 53, 851–859. ISSN 2234358X. doi:10.1016/j.net.2020.07.037
Keywords: load-following, reactor kinetics, reactor control, reference scenarios, reactivity feedback
Citation: Žerovnik G, Čalič D, Gerkšič S, Kromar M, Malec J, Mihelčič A, Trkov A and Snoj L (2023) An overview of power reactor kinetics and control in load-following operation modes. Front. Energy Res. 11:1111357. doi: 10.3389/fenrg.2023.1111357
Received: 29 November 2022; Accepted: 31 January 2023;
Published: 23 February 2023.
Edited by:
Valerio Giusti, University of Pisa, ItalyReviewed by:
Jiuwu Hui, Hong Kong University of Science and Technology, Hong Kong SAR, ChinaMahmoud Elsisi, National Taiwan University of Science and Technology, Taiwan
Copyright © 2023 Žerovnik, Čalič, Gerkšič, Kromar, Malec, Mihelčič, Trkov and Snoj. This is an open-access article distributed under the terms of the Creative Commons Attribution License (CC BY). The use, distribution or reproduction in other forums is permitted, provided the original author(s) and the copyright owner(s) are credited and that the original publication in this journal is cited, in accordance with accepted academic practice. No use, distribution or reproduction is permitted which does not comply with these terms.
*Correspondence: Gašper Žerovnik, Z2FzcGVyLnplcm92bmlrQGlqcy5zaQ==