- 1College of Electrical Engineering, Zhejiang University, Hangzhou, China
- 2School of Electric Power Engineering, South China University of Technology, Guangzhou, China
- 3National Power Dispatching and Control Center, State Grid Corporation of China, Beijing, China
- 4State Grid Zhejiang Electric Power Co., Ltd., Hangzhou, China
After proposing the carbon peaking and carbon neutrality target, China further proposed a series of specific carbon emission growth limit sub-targets. How to decarbonize the energy system to ensure the realization of the carbon growth limit sub-targets is a meaningful topic. At present, generation expansion planning of renewable energy in integrated energy systems has been well studied. However, few of the existing studies consider specific carbon emission growth targets. To address this research gap, a two-stage robust generation expansion planning framework for regional integrated energy systems with carbon growth constraints is proposed in this paper, which takes into account multiple uncertainties. In this framework, the objective function is to minimize the total operation cost and wind turbine investment cost. The first stage is the decision-making level of the wind turbine capacity configuration scheme. The second stage is the optimal economic dispatching in the worst-case scenario, which is a bi-level problem of max-min form. Thus, the two-stage robust optimization framework constitutes a problem of min-max-min form, which is pretty hard to solve directly with a commercial solver. Therefore, a nested column-and-constraint generation algorithm is adopted and nested iterations are performed to solve the complex problem. Finally, case studies are carried out on a regional electric-gas integrated energy system. The MATLAB/YALMIP simulation platform with the Gurobi solver is used to verify the effectiveness and superiority of the proposed framework. Compared with other four cases, 5,000 Monte Carlo scheduling tests demonstrate that the proposed framework can ensure the system carbon emission to be controlled within a certain limit even in the worst scenario. Due to the consideration of multiple uncertainties, the proposed framework planning results are both robust and economical for investment. This study can provide theoretical support for the actual regional integrated energy system to achieve a certain carbon growth target.
1 Introduction
Low carbon is the common theme of development in the world today. After the Paris Agreement was put forward, countries around the world have attached great importance to carbon reduction (Höhne et al., 2021; Liu Y. et al., 2022). Since China announced the carbon peak and carbon neutrality goals, it has concreted the macro-strategic goals into various regional development indicators (Zhang et al., 2021; Sun et al., 2022). The energy system is a key area of carbon emissions in a region (Huang et al., 2020). Therefore, it is of great practical significance to study the low-carbon optimization of regional integrated energy systems (Xie et al., 2022).
Renewable energy power generation is an essential path to decarbonizing the regional integrated energy system. Therefore, renewable energy generation expansion planning has attracted the attention of many scholars. A group of scholars focused on power generation expansion planning for different objects (Toloo et al., 2022) considered both centralized and distributed solar, wind, hydro, geothermal and biomass power generation expansion plans (Fang et al., 2021) studied the joint planning of renewable energy generation and energy storage batteries in renewable energy integrated microgrids (Gonzalez-Romero et al., 2020) presented a review of the state-of-the-art on the coordination of generation and transmission expansion planning (Zhang et al., 2020) conducted a coordination planning study of wind farms, energy storage, and transmission network.
On the other hand, many scholars have studied the power generation expansion planning of renewable energy considering different factors or scenarios, and proposed various new models. The continuous penetration of renewable energy has an impact on the optimization model construction (Deng and Lv, 2020) screened out 34 studies of power system planning considering increasing variable renewable energy, and then the models of which were further deconstructed and compared. A new hierarchical modeling framework that integrates two multi-period and multi-regional generation expansion planning models was studied in (Lyrio de Oliveira et al., 2022). While the objective function of most power generation expansion research is the total cost, (Li et al., 2020) focused on increasing the utilization of renewable energy and reducing the renewable energy curtailment., and proposed a new objective function to maximize the accommodation of renewable energy during the planning horizon (Jabarnejad, 2021) integrated dynamic-line rating and transmission switching into the renewable sources generation-expansion planning problem.
Two factors, the uncertainties and carbon emissions, are usually considered in the planning problems of integrated energy systems. The uncertainty is an unavoidable consideration when studying power generation expansion planning (Yang et al., 2022) summarized various uncertainty modeling methods and discussed the application of uncertainty modeling in power system planning and operation. For the integrated energy system, the uncertainties of renewable energy and energy demand are usually considered (Fang et al., 2021) considered the uncertainties of wind power and solar power in the planning of renewable energy generation and storage batteries in a renewable energy integrated microgrid (Shen et al., 2020) considered the uncertainties of load and wind power output at the same time when they studied the power generation expansion planning of the energy system (Ahmadi et al., 2020) proposed a dynamic, robust generation-transmission expansion planning in the presence of wind farms under long-term and short-term uncertainties of wind power (Lei et al., 2020) proposed a multi-objective stochastic planning method based on the multi-dimensional correlation scenario generation method for the regional integrated energy system integrated renewable energy considering the uncertainties of renewable energies and loads of multiple energy types. The above studies have fully considered various uncertainties of sources and loads of various energy types. However, under the current turbulent international situation and the swaying global economy, the impact of natural gas price uncertainty on the regional integrated energy system has not been paid enough attention in the power generation expansion planning.
The power generation expansion planning considering the carbon emissions has been well researched. In the context of low-carbon development, some power generation expansion planning studies have considered carbon trading. A two-layer low-carbon expansion generation planning model considering carbon trading and carbon capture technology was established in (Mi et al., 2021). An innovative carbon emission trading scheme was formulated and applied to the co-planning of distributed renewable energy generation units, energy storage systems, and capacitor banks in (Melgar-Dominguez et al., 2020). (Liu J. et al., 2022) established a multi-flexibility resource planning model with a ladder-type carbon trading. The power generation expansion planning considering carbon trading can effectively promote the allocation of renewable energy power generation and mitigate system carbon emissions but cannot limit the amount of the total carbon emissions. Although there are few studies of expansion planning considering the carbon emission constraints (LU; Zhigang et al., 2022), a certain macro-target of carbon emissions isn’t considered in the regional development planning.
All in all, the existing research on power generation expansion planning in the regional integrated energy system has been well studied, but there is still a lack of integrating the actual regional carbon emission control targets and the uncertainty of natural gas price into the planning. To fill the research gap, this paper mainly makes the following contributions.
(1) Based on regional macro development goals, e.g., GDP (Gross Domestic Product) growth rate, energy consumption per unit of GDP, and carbon emission per unit of GDP, a regional integrated energy system carbon growth constraint model is constructed.
(2) In the power generation expansion planning of the regional integrated energy system, this paper considers multiple uncertainties in the process of robust optimization. In addition to the conventional wind power output and load uncertainties, the impact of natural gas price uncertainty on regional integrated energy system planning is also considered in light of the international situation. The influence mechanism of the three kinds of uncertainties on planning results is also briefly analyzed.
(3) A two-stage robust generation expansion planning framework for regional integrated energy systems with carbon growth constraints is proposed to obtain the wind power capacity that ensures the system meets carbon growth targets even in the worst-case scenarios. Based on the nested column-and-constraint generation (C&CG) algorithm, the proposed framework can be transformed into several mixed integer quadratic programming models that can be solved by commercial solvers.
The remainder of the paper is organized as follows: Section 2 describes the multiple uncertainty modeling and the proposed carbon growth constraints modeling. In Section 3, the proposed robust generation expansion planning framework and solution procedure are constructed. In Section 4, case studies are demonstrated. Section 5 concludes the work.
2 Methodology
2.1 Uncertainty modeling
Robust optimization does not require the probability distribution of uncertain parameters to be given in advance, but describes the fluctuation of parameters through a certain bounded set, which is called an uncertain set. As long as the value of the parameter is within the range of the uncertain set, the solution of the robust optimization model must be feasible. Commonly used uncertain sets include box uncertain sets, polyhedral uncertain sets, ellipsoid uncertain sets, and N-k uncertain sets (Zhu et al., 2017). The first three sets are suitable for continuous uncertain parameters such as bus injected power and electricity price, and the third set is suitable for discrete uncertain parameters such as fault states.
2.1.1 Uncertainty modeling of power system
Common uncertainties in power system include uncertainty of injected power, uncertainty of electricity price, uncertainty of power load and uncertainty of N-k fault (Zhu et al., 2017). This study mainly focuses on the uncertainty of wind power and power load. Since the optimization results of the box uncertainty set are too conservative, polyhedral uncertainty sets are adopted to model uncertainties of wind power and power load. The polyhedral uncertainty set of power load are shown as Eq. 1.
where
To better characterize the fluctuation of wind power, the relative value of wind power deviation is used to generate the base uncertainty set, which is a special polyhedron uncertainty set. The base uncertainty set of wind power are shown as Eq. 2.
where
2.1.2 Uncertainty modeling of the gas system
Since natural gas can be compressed, the uncertainty of natural gas load can be mitigated by sufficient storage capacity in the transmission pipeline (Guo et al., 2020; Yi et al., 2022). Therefore, the gas load uncertainty can usually not be considered in the research. However, as stated in the Section 1, the gas price will even increase several times in a short period of time because there are many uncertain factors affecting it, and its uncertainty is strong. Therefore, this study mainly focuses on the uncertainty of gas price and a polyhedral uncertainty set are adopted to model uncertainties of gas price. The polyhedral uncertainty set of gas price are shown as Eq. 3.
where
2.2 Carbon growth constraint modeling
A regional integrated energy system plan should be incorporated into the regional macro development plan. After putting forward the goal of achieving peak carbon neutrality, the Chinese government further put forward the target of reducing carbon emission per unit of GDP, reducing energy consumption per unit of GDP. Considering these macroscopic development goals, a specific constraint for regional integrated energy system planning can be proposed. The following takes China’s 14th Five-Year Plan as an example to illustrate the modeling of carbon growth constraints.
The reduction target of carbon emission per unit GDP during the 14th Five-Year Plan period can be expressed as Eq. 4.
where
The target of reducing energy consumption per unit of GDP during the 14th Five-Year Plan period can be expressed as Eq. 5.
where
The GDP growth target during the 14th Five-Year Plan period can be expressed as Eq. 6.
where
According to Eqs 4, 6, the average annual carbon emission growth rate
According to Eqs 5, 6, the annual growth rate of energy consumption
3 The proposed robust generation expansion planning framework and solution procedure
Planning problems that consider uncertainty can usually be solved by fuzzy optimization, stochastic optimization, or robust optimization. Compared with fuzzy optimization and stochastic optimization, robust optimization does not require artificially given membership function or probability distribution function of uncertain parameters, and can strictly guarantee the feasibility of the solution (Du et al., 2022). Achieving carbon reduction targets is a daunting and important task, and this study aims to explore how power generation expansion planning can be done under various uncertainties to achieve carbon reduction targets. Therefore, a robust generation expansion planning framework are proposed in this paper.
3.1 The proposed two-stage robust planning framework
According to the number of decision-making stages, robust optimization can be divided into static robust optimization and two-stage robust optimization. Static robust optimization, also known as single-stage robust optimization, is characterized by making decisions before the specific realization of uncertain parameters is known, so the results are usually too conservative and inconsistent with the actual situation. Because the decision of the two-stage robust optimization is carried out in stages, the conservativeness of the model is weaker than that of the static robust optimization model (Zhu et al., 2017). Therefore, two-stage robust optimization are adopted in this study to solve the generation expansion planning considering the carbon emission growth limits. The schematic diagram of proposed two-stage robust generation expansion planning framework is shown as Figure 1.
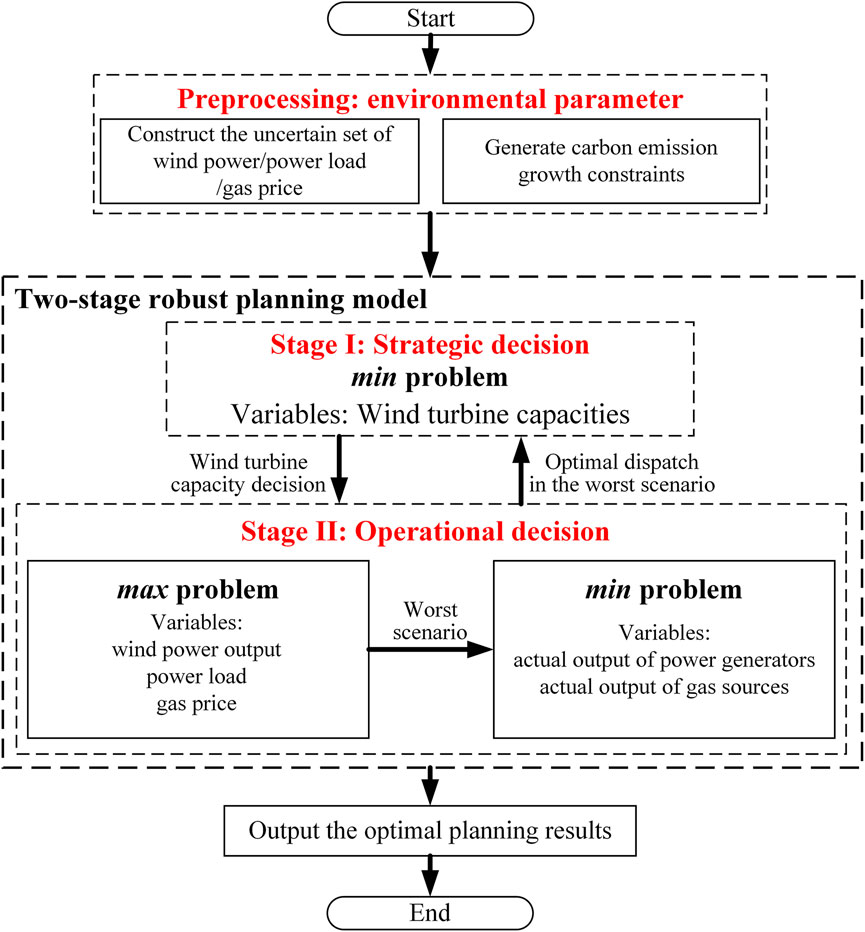
FIGURE 1. The schematic diagram of the proposed two-stage robust generation expansion planning framework.
3.1.1 Objective function
The two-stage robust optimization model is adopted to handle the uncertainties of wind power, power loads, and gas prices. The objective function of stage I consists of wind turbine investment costs and its strategic decision variables are the capacities of wind turbines. Stage II is to carry out the economic dispatch in the worst scenario of the whole uncertain set under the situation of the first-stage decision. Its operational decision variables are the actual outputs of power generators and gas sources. The objective function of stage II consists of the energy supply costs of the electric-gas integrated energy system. The complete formulation of the objective function in the proposed two-stage robust optimization model is shown as Eq. 9.
where
3.1.2 Constraints
Since carbon emissions in the power system are closely related to active power flow, the DC power flow modeling is adopted in this paper. Constraints Eqs 10–16 represent the power system operational constraints. Constraint Eq. 17 represents the wind turbine capacity constraint. Constraints of uncertain sets of power load, wind power and gas price are shown in Eqs 1–3.
where
The steady-state modeling of gas system based on the Weymouth function (De Wolf and Smeers, 2000) is adopted in this paper. Constraints Eqs 18–22 represent the gas system operational constraints. Constraints Eqs 23, 24 represent operational constraints of coupling units. Constraints Eq. 25 denotes the carbon emission growth constraints.
where
3.2 Solution procedure based on the nested C&CG algorithm
Even the easiest two-stage robust optimization (TRO), composed of three levels, can be an NP-hard problem. To overcome the computational burden, the Benders-dual cutting plane algorithm and column-and-constraint generation algorithm are commonly used to solve the two-stage robust optimization. Compared to the Benders-dual cutting plane algorithm, the C&CG algorithm performs an order of magnitude faster and therefore is commonly used to solve the TRO (Zeng and Zhao, 2013). Specifically in this study, the Weymouth function in constraints Eq. 19 is quadratic and can be piecewise linearized by introducing 0–1 variables. Since stage II in the proposed planning framework is a bi-level mixed integer programming problem that cannot be solved by the traditional C&CG algorithm, a nested C&CG algorithm proposed in (Long and Bo, 2012) was adopted to the TRO with integer variables in the second stage. According to the nested C&CG algorithm, the proposed TRO problem is divided into the master problem (MP) and the subproblem (SP) which is divided into the master problem of SP (MPS) and the subproblem of SP (SPS). Specifically, MPS is a single-level max problem converted from a bi-level max-min problem by KKT conditions and given values of binary variables
where
Algorithm 1. The nested C and CG algorithm.
Outer loop: Solve the MP
Step 1: Set the loop index, upper bound, and lower bound of the outer loop, and artificially give an initial scenario of uncertain sets:
Step 2: Solve the MP and obtain the optimal wind turbine capacities
Step 3: Jump to the inner loop, solve the SP and obtain the worst scenario of the uncertain set
Step 4: Determine whether it is convergent. 1) If
Inner loop: Solve the SP
Step 1: Set the loop index, upper bound, and lower bound of the inner loop, and give initial values of binary variables
Step 2: Solve the MPS, obtain the current worst scenario of the uncertain set
Step 3: Solve the SPS, obtain the binary variables
Step 4: Determine whether it is convergent. 1) If
4 Case studies
To verify the validity and superiority of the proposed planning framework, case studies were conducted on a regional integrated energy system formed by the coupling of IEEE 24-bus power system and 7-node natural gas system. All emulators run on a desktop computer with an Intel Core i7-8700 CPU and 32 GB memory. The case models were constructed based on the MATLAB/YALMIP platform and solved by Gurobi solver.
4.1 System parameters
The regional electric-gas integrated energy system adopted in this section is shown in Figure 2 (Zimmerman et al., 2011). The power system consists of five coal-fired units and two gas-fired units, as well as three wind turbines to be planned. The relevant parameters of the generator set are shown in Table 1 (Jamie McIntyre et al., 2011; Zimmerman et al., 2011). The natural gas system consists of two gas sources, five branches and one compressor. The parameters of the gas source are shown in Table 2. The parameters of the natural gas pipeline are shown in Table 3. Compressor ratios in natural gas systems range from 1.1 to 1.5. There are 12 electrical loads and three gas loads in the integrated energy system. The reference values of electrical load and gas load are shown in Table 4 and Table 5. The prediction value of the 24-h power load and gas load is shown in Figure 3. As described in Section 2, the uncertainty of natural gas load can be ignored. The maximum prediction error of power load is 20% (Guo et al., 2020). The uncertainty parameters of electric load, wind power and natural gas prices are shown in Table 6. The electric power system and natural gas system are coupled by two gas turbine units and one power-to-gas (P2G) unit. The installed capacity of the P2G unit is 150 MW. The maximum wind power capacity planned for each node in the system is 500 MW. During the 14th Five-Year Plan period, the carbon emission per unit GDP in this region is targeted to be reduced by 19%, the energy consumption per unit GDP is targeted to be reduced by 14.5%, and the average annual growth rate of GDP is 7%. The fixed investment cost of wind power is 115 USD/(MW∙day).
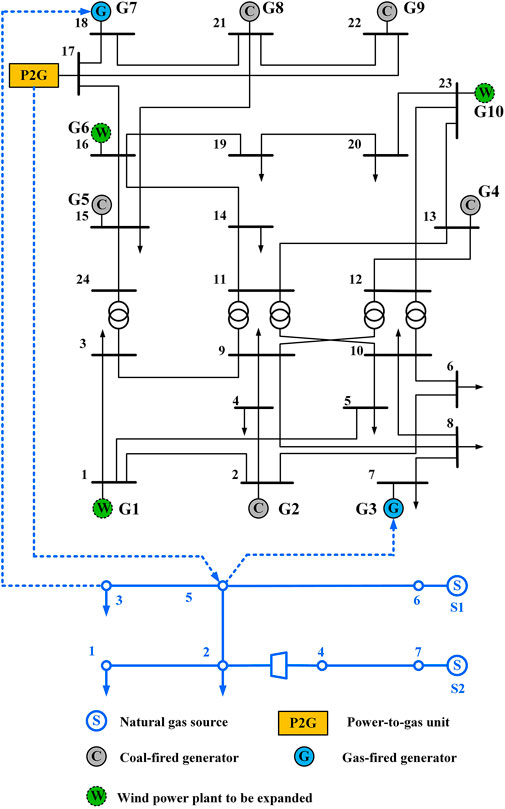
FIGURE 2. Integrated energy system coupled with IEEE 24-bus power system and 7-node natural gas system.
4.2 Optimized results of the proposed two-stage robust planning framework
The nested C&CG algorithm is used to solve the proposed two-stage robust programming framework. The convergence condition is the percentage deviation between the objective function values of the MP and SP. In the process of solving the model in this paper, the convergence condition is set to 0.8%. Figure 4 illustrates the convergence process for solving the proposed two-stage robust planning framework and the solution time of proposed nsted C&CG algorithm is 1864.2 s.
Before adopting the proposed framework, the total carbon emission of the test system is 52,513 tCO2. According to Eqs 7, 8, the annual energy demand of the test system increases by 3.70% brought by regional economic development, and the growth of carbon emissions should be controlled within 2.58%. It can be seen that after the planning of wind power in the regional integrated energy system, the total carbon emissions of the system should be controlled within 53,868 tCO2 in any scenario.
Figure 5 shows the wind power planning capacity of the three buses under the proposed planning framework. To verify whether the planning results can ensure carbon emissions meet the requirements, this paper conducts a robust scheduling test on the planning results. The results show that the planning results can indeed make the system meet the carbon growth constraints in the worst scenario, as shown in Figure 5. Therefore, it can be verified that the proposed two-stage robust planning framework can effectively promote wind power configuration. In addition, it can ensure that the carbon emissions of the regional integrated energy system will not exceed the limited target during the dispatch operation even in the worst scenario.
4.3 Effect analysis of carbon emission constraints
The planning framework proposed in this paper embeds carbon growth constraints based on regional macro-development goals. To study the impact of carbon growth constraints on the planning results, the following two cases are set for comparison as shown in Table 7. Case 1 is the planning framework proposed in this paper, and Case 2 is the control group.
The results of wind turbine capacity planned in Case 1 are shown in Figure 5 above. In Case 2, the optimal planning capacity of the wind turbines at the three buses is all 0. Since the carbon growth constraints are not considered in Case 2, while relatively cheap and sufficient thermal power can meet the growing energy demand, wind turbines with fixed investment costs will not be planned. In Case 1, in the two-stage robust optimization, the system will be forced to plan the wind turbines to meet the carbon growth constraints.
To compare the effect of planning results on system carbon emissions in the two cases, this paper conducts 5,000 Monte Carlo simulations on the planning results of Case 1 and Case 2 in the dispatch model with carbon growth constraints and multiple inferior uncertainty sets. The simulation results are shown in Table 8. It is obvious that the planning results of Case 2 can hardly meet the carbon emission growth constraints when dispatching in inferior scenarios. Thus, the necessity of considering carbon growth constraints in the proposed framework is further highlighted.
The wind power planning capacity of Case 1 is obtained under the worst scenario in robust optimization. In order to further study the carbon reduction potential of the system in Case 1, the wind turbine capacity of Case 1 is tested in the system economic dispatch. Figure 6 comparatively presents the carbon emissions of the system under economic dispatch and robust dispatch with the wind turbine capacity of Case 1. It can be seen that the wind power capacity obtained by the proposed planning framework cann’t only enable the system to meet the carbon growth targets in the worst scenario, but also have a huge space for carbon reduction in actual dispatch.
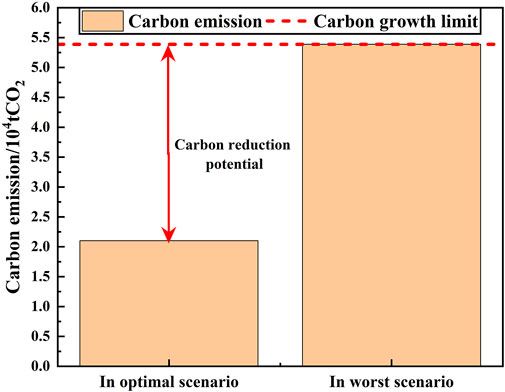
FIGURE 6. System carbon emissions of economic dispatch and robust dispatch with wind turbine capacity of Case 1.
4.4 Robustness analysis
The proposed two-stage robust planning framework takes into account the three uncertainties of power load, wind power and natural gas prices. To test the advantages of the proposed model in terms of robustness, several sets of cases as shown in Table 9 are set up for comparison.
Figure 7 presents the capacity of wind turbines obtained by two-stage robust optimization of Case 1, Case 3, Case 4, and Case 5. It can be found that the total wind power capacities planned in Case 3 and Case 5 are significantly lower than those in Case 1 and Case 4. Case 3 doesn’t consider the uncertainty of power load, so in the two-stage robust optimization, there will be lower load requirements compared with Case 1, which directly leads to lower wind turbine capacity requirements. Case 5 doesn’t consider the uncertainty of natural gas prices. The optimal operation of coupling equipment depends on the relative price of electricity and natural gas. Therefore, in the robust optimization, Case 1 will generate higher gas prices than Case 5. As a result, the P2G is promoted to operate and consume electric power, as shown in Figure 8, which is equivalent to increasing the power load. Therefore, natural gas price uncertainty and power load uncertainty have similar effects. In addition, it can be noticed that the total capacity of wind turbines in Case 4 is slightly larger than that in Case 1, which is affected by the uncertainty of wind power. Since Case 1 considers the uncertainty of wind power compared with Case 4, Case 1 has a worse wind power scenario in the two-stage robust planning. In this way, considering the fixed investment cost of wind turbines, the equivalent cost of wind power generation in Case 1 is higher than that in Case 4. Therefore, the installed capacity of wind turbines in Case 4 will be irrationally configured as much as possible. Overall, in the two-stage robust optimization, the uncertainties of power load and natural gas price will promote the capacity planning of wind turbines in the regional integrated energy system, while the uncertainty of wind power will inhibit the capacity planning of wind turbines.
To demonstrate the superiority of the proposed model, 5,000 times of Monte Carlo robust dispatching simulations are performed on the planned wind turbine capacity of the above four cases. The simulation results are shown in Table 10.
According to the results in Table 10, it can be seen that the planning results of Case 1 and Case 4 have good robustness, while the planning results of Case 3 and Case 5 are less robust. Although the robustness of Case 4 is good, the wind power uncertainty is not considered in the robust optimization, which will lead to irrational configuration of wind turbine capacities and waste of wind turbine investment. The proposed Case 1 considers more comprehensive uncertainties, and the planning results are both robust and economical in investment, which is a better planning scheme.
5 Conclusion
In this paper, a two-stage generation expansion planning framework for a regional integrated energy system is proposed considering carbon emission constraints. The uncertainties of wind power, power load, and natural gas price are also considered in the proposed framework. The nested C&CG algorithm is used to solve the two-stage robust planning model. Based on the IEEE 24-bus power system and 7-node natural gas system integrated energy system, the effectiveness and superiority of the proposed framework are verified. The following conclusions can be obtained through numerical simulation.
(1) The proposed two-stage robust generation expansion planning framework with carbon growth constraints can effectively promote the configuration of wind turbines and ensure that the carbon emissions of the regional integrated energy system will not exceed the defined target even in the worst scenario.
(2) The wind plant planning result of the proposed model is the configuration result in the worst scenario. Therefore, in actual operation, the wind power capacity obtained by the proposed planning framework can not only enable the system to meet the carbon growth targets in the worst scenario but also have a huge space for carbon reduction.
(3) In the two-stage robust optimization, both electricity load uncertainty and natural gas price uncertainty will promote the capacity planning of wind power, while wind power uncertainty will inhibit the capacity planning of wind turbines.
(4) By comparing the model planning results with different uncertainty sets, it can be found that the proposed model has good robustness, and at the same time, it takes into account the economy of wind turbine planning investment.
This article focuses on the wind turbine capacity planning, so the operating cost of P2G isn’t considered. P2G isn’t only a coupling channel for integrating energy systems, but also an effective way to introduce low-carbon natural gas into gas systems. In future studies, the proposed model can further consider the coordinated planning of wind turbines and P2G units.
Data availability statement
The original contributions presented in the study are included in the article/supplementary material, further inquiries can be directed to the corresponding author.
Author contributions
JN, JF, and HZ conceptualized the study, performed the methodology, and validated the results. JN and JF prepared the original draft. XD contributed to the improvement of the study design. LG and KS reviewed and edited the draft. HZ supervised the study. All authors listed have made a substantial, direct, and intellectual contribution to the work and approved it for publication.
Conflict of interest
LG was employed by State Grid Corporation of China. KS was employed by State Grid Zhejiang Electric Power Co., Ltd.
The remaining authors declare that the research was conducted in the absence of any commercial or financial relationships that could be construed as a potential conflict of interest.
Publisher’s note
All claims expressed in this article are solely those of the authors and do not necessarily represent those of their affiliated organizations, or those of the publisher, the editors and the reviewers. Any product that may be evaluated in this article, or claim that may be made by its manufacturer, is not guaranteed or endorsed by the publisher.
References
Ahmadi, S., Mavalizadeh, H., Ghadimi, A. A., Miveh, M. R., and Ahmadi, A. (2020). Dynamic robust generation–transmission expansion planning in the presence of wind farms under long- and short-term uncertainties. IET Generation, Transm. Distribution 14 (23), 5418–5427. doi:10.1049/iet-gtd.2019.1838
De Wolf, D., and Smeers, Y. (2000). The gas transmission problem solved by an extension of the simplex algorithm. Manag. Sci. 46 (11), 1454–1465. doi:10.1287/mnsc.46.11.1454.12087
Deng, X., and Lv, T. (2020). Power system planning with increasing variable renewable energy: A review of optimization models. J. Clean. Prod. 246, 118962. doi:10.1016/j.jclepro.2019.118962
Du, G., Zhao, D., and Liu, X. (2022). Research review on optimal scheduling considering wind power uncertainty. Zhongguo Dianji Gongcheng Xuebao/Proceedings Chin. Soc. Electr. Eng. 1–21.
Fang, F., Zhu, Z., Jin, S., and Hu, S. (2021). Two-layer game theoretic microgrid capacity optimization considering uncertainty of renewable energy. IEEE Syst. J. 15 (3), 4260–4271. doi:10.1109/jsyst.2020.3008316
Gonzalez-Romero, I. C., Wogrin, S., and Gómez, T. (2020). Review on generation and transmission expansion co-planning models under a market environment. IET Generation, Transm. Distribution 14 (6), 931–944. doi:10.1049/iet-gtd.2019.0123
Guo, Z., Li, G., and Zhou, M. (2020). Fast and dynamic robust optimization of integrated electricity-gas system operation with carbon trading. Power Syst. Technol. 44 (4), 1220–1228.
Höhne, N., Gidden, M. J., den Elzen, M., Hans, F., Fyson, C., Geiges, A., et al. (2021). Wave of net zero emission targets opens window to meeting the Paris Agreement. Nat. Clim. Change 11 (10), 820–822. doi:10.1038/s41558-021-01142-2
Huang, W., Zhang, N., Cheng, Y., Yang, J., Wang, Y., and Kang, C. (2020). Multienergy networks analytics: Standardized modeling, optimization, and low carbon analysis. Proc. IEEE 108 (9), 1411–1436. doi:10.1109/jproc.2020.2993787
Jabarnejad, M. (2021). Linearized generation-expansion planning considering transmission planning, switching, and dynamic-line rating. J. Energy Eng. 147 (4). doi:10.1061/(asce)ey.1943-7897.0000769
Jamie McIntyre, B. B., Harvey, S., and Borchardt, S. (2011). Comparison of lifecycle greenhouse gas emissions of various electricity generation sources. World Nuclear Association, London.
Lei, Y., Wang, D., Jia, H., Chen, J., Li, J., Song, Y., et al. (2020). Multi-objective stochastic expansion planning based on multi-dimensional correlation scenario generation method for regional integrated energy system integrated renewable energy. Appl. Energy 276, 115395. doi:10.1016/j.apenergy.2020.115395
Li, Q., Wang, J., Zhang, Y., Fan, Y., Bao, G., and Wang, X. (2020). Multi-Period generation expansion planning for sustainable power systems to maximize the utilization of renewable energy sources. Sustainability 12 (3), 1083. doi:10.3390/su12031083
Liu, J., Liu, M., Wang, Z., Yang, J., and Lou, S. (2022a). Multi-flexibility resources planning for power system considering carbon trading. Sustainability 14 (20), 13296. doi:10.3390/su142013296
Liu, Y., Du, M., Cui, Q., Lin, J., Liu, Y., Liu, Q., et al. (2022b). Contrasting suitability and ambition in regional carbon mitigation. Nat. Commun. 13 (1), 4077. doi:10.1038/s41467-022-31729-y
Long, Z., and Bo, Z. (2012). An exact algorithm for two-stage robust optimization with mixed integer recourse problems. University of South Florida, Tampa.
Lyrio de Oliveira, L., de Oliveira Ribeiro, C., and Qadrdan, M. (2022). Analysis of electricity supply and demand intra-annual dynamics in Brazil: A multi-period and multi-regional generation expansion planning model. Int. J. Electr. Power and Energy Syst. 137, 107886. doi:10.1016/j.ijepes.2021.107886
Melgar-Dominguez, O. D., Pourakbari-Kasmaei, M., Lehtonen, M., and Sanches Mantovani, J. R. (2020). An economic-environmental asset planning in electric distribution networks considering carbon emission trading and demand response. Electr. Power Syst. Res. 181, 106202. doi:10.1016/j.epsr.2020.106202
Mi, Y., Liu, C., Yang, J., Zhang, H., and Wu, Q. (2021). Low-carbon generation expansion planning considering uncertainty of renewable energy at multi-time scales. Glob. Energy Interconnect. 4 (3), 261–272. doi:10.1016/j.gloei.2021.07.005
Shen, W., Qiu, J., Meng, K., Chen, X., and Dong, Z. Y. (2020). Low-carbon electricity network transition considering retirement of aging coal generators. IEEE Trans. Power Syst. 35 (6), 4193–4205. doi:10.1109/tpwrs.2020.2995753
Sun, L.-L., Cui, H.-J., and Ge, Q.-S. (2022). Will China achieve its 2060 carbon neutral commitment from the provincial perspective? Adv. Clim. Change Res. 13 (2), 169–178. doi:10.1016/j.accre.2022.02.002
Toloo, M., Taghizadeh-Yazdi, M., and Mohammadi-Balani, A. (2022). Multi-objective centralization-decentralization trade-off analysis for multi-source renewable electricity generation expansion planning: A case study of Iran. Comput. Industrial Eng. 164, 107870. doi:10.1016/j.cie.2021.107870
Xie, B.-C., Lu, L., and Duan, N. (2022). Environmental efficiency assessment of China’s integrated power system under the assumption of semi-disposability. Comput. Industrial Eng. 167, 108023. doi:10.1016/j.cie.2022.108023
Yang, C., Sun, W., Han, D., and Yin, X. (2022). Research on power system flexibility considering uncertainties. Front. Energy Res. 10. doi:10.3389/fenrg.2022.967220
Yi, W., Bu, Q., Lu, S., Qin, Y., and Li, P. (2022). Distributionally robust optimal dispatching of integrated energy system considering line pack effect of gas network. Electr. Power Autom. Equip. 42 (6), 53.
Zeng, B., and Zhao, L. (2013). Solving two-stage robust optimization problems using a column-and-constraint generation method. Operations Res. Lett. 41 (5), 457–461. doi:10.1016/j.orl.2013.05.003
Zhang, C., Cheng, H., Liu, L., Zhang, H., Zhang, X., and Li, G. (2020). Coordination planning of wind farm, energy storage and transmission network with high-penetration renewable energy. Int. J. Electr. Power and Energy Syst. 120, 105944. doi:10.1016/j.ijepes.2020.105944
Zhang, S., Li, M., Wang, C., An, K., Zhou, J., and Cai, B. (2021). Carbon emission trend analysis of China' s provinces and their differentiated peak strategy design. China Popul. Resour. Environ. 31 (9), 45–54.
Zhigang, L., Liu, Y., Dong, W., Li, Y., He, L., and Guo, X. (2022). Joint transformation planning of solar energy storage in carbon neutral area based on electricity-carbon coupling operation. High. Volt. Technol. 1–16. doi:10.13336/j.1003-6520.hve.20220852
Zhu, G., Lin, J., Luo, Z., Dai, S., Qin, L., and Liu, C. (2017). Review of robust optimization for generation scheduling in power systems. Zhongguo Dianji Gongcheng Xuebao/Proceedings Chin. Soc. Electr. Eng. 37 (20), 5881–5892. doi:10.13334/j.0258-8013.pcsee.161950
Keywords: carbon emission, carbon growth constraints, electric-gas integrated energy system, generation expansion planning, nested column-and-constraint generation algorithm, two-stage robust optimization
Citation: Nan J, Feng J, Deng X, Guan L, Sun K and Zhou H (2023) A two-stage robust generation expansion planning framework for regional integrated energy systems with carbon growth constraints. Front. Energy Res. 11:1106628. doi: 10.3389/fenrg.2023.1106628
Received: 24 November 2022; Accepted: 06 February 2023;
Published: 17 February 2023.
Edited by:
IMR Fattah, University of Technology Sydney, AustraliaCopyright © 2023 Nan, Feng, Deng, Guan, Sun and Zhou. This is an open-access article distributed under the terms of the Creative Commons Attribution License (CC BY). The use, distribution or reproduction in other forums is permitted, provided the original author(s) and the copyright owner(s) are credited and that the original publication in this journal is cited, in accordance with accepted academic practice. No use, distribution or reproduction is permitted which does not comply with these terms.
*Correspondence: Hao Zhou, emhvdWhhb19lZUB6anUuZWR1LmNu