- 1School of Economics and Management, Tibet University, Lhasa, Tibet, China
- 2School of Culture and Communication, Central University of Finance and Economics, Beijing, China
- 3School of Mathematics, Renmin University of China, Beijing, China
Energy storage solutions are strategically important for achieving carbon neutrality and carbon peaking goals. However, high installation costs, demand mismatch, and low equipment utilization have prevented the large-scale commercialization of traditional energy storage. The shared energy storage mode that relies on sharing economy can effectively overcome these problems and has recently attracted widespread attention. In this mini-review, firstly, the concept of shared energy storage is discussed and its application in different countries is illustrated. Second, two core issues in the shared energy storage research—optimal energy scheduling and rational profit distribution—are sorted out and the common modeling approaches and solving algorithms are summarized. Additionally, the dilemma of balancing energy efficiency with distribution fairness faced by the practical application of shared energy storage is pointed out. On this basis, blockchain technology is pointed out to solve the above dilemma of shared energy storage and key directions are given for future research.
1 Introduction
As the timeline for targets of reaching the carbon peak and carbon neutrality is nearing, the global energy structure is becoming cleaner and more diversified (Yang et al., 2016; Hou et al., 2021). The global consensus is that active renewable energy development is one of the main ways to transform the current energy industry to a clean and low-carbon type (Yang et al., 2018). To accelerate the development of renewable energy, countries have developed their action plans, stipulating the development goals of renewable energy (Energy research Centre of the Netherlands, 2011). In May 2021, the European Union promulgated the “European Climate Law,” raising the target of renewable energy in primary energy by 2030 from 32% to 40% (Council of the European Union, 2021). In October 2021, the Japanese government released “The Sixth Strategic Energy Plan,” to increase the share of renewable energy in electricity generation from 22%–24% to 36%–38% by 2030 (Ministry of Economy, Trade and Industry, 2021). In February 2022, the United States Department of Energy (DOE) released the “Offshore Wind Energy Strategy,” proposing that the installed capacity of offshore wind power should reach 30 GW by 2030 in the United States, achieving a CO2 emission reduction of 78 million tons (United States Department of Energy, 2022). In May 2022, China’s National Energy Administration released the “Implementation Plan on Promoting the High-Quality Development of New Energy in the New Era,” which sets the target for the total installed capacity of wind and solar power to reach more than 1.2 billion kilowatts by 2030, accelerating the construction of a clean, low-carbon, safe, and efficient energy system (National Development and Reform Commission et al., 2022b). In August 2022, South Korea’s Ministry of Trade, Industry, and Energy (MOTIE) released a draft long-term energy plan, which sets a goal for renewable energy to account for 21.5% of the country’s electricity generation by 2030 (South Korea’s Ministry of Trade, Industry, and Energy, 2022).
As one of the key areas to help achieve the goal of “carbon neutralization and carbon peaking”, the primary task is to promote the transformation of low-carbon energy (Parthan et al., 2010; Andrews-Speed, 2016) and to build a new power system with new energy as the main body (Li et al., 2022c). In recent years, new energy represented by wind power or photovoltaic power has attracted a wide range of attention worldwide (Chen et al., 2007; Li et al., 2013). According to the estimation from International Energy Agency (IEA), the annual global wind-power production will reach 1282 TW·h by 2020, a nearly 371% increase from 2009. By 2030, that figure will reach 2182 TW·h, almost doubling the year 2020 production (International Energy Agency, 2021). However, the volatility and inconsistency of wind and photovoltaic power generation cause many problems, such as supply and demand imbalance, insufficient absorption capacity, and frequent abandonment of wind and light (Tang et al., 2018; Erdiwansyah et al., 2021). These issues can be solved with battery energy storage, which is widely used in various scenarios, such as peak and frequency regulation of power systems, mitigation of renewable energy output fluctuations, demand-side response, and auxiliary services (Yang et al., 2022).
However, traditional battery energy storage has shortcomings, such as high individual installation costs, difficulty matching demand capacity (Zhao et al., 2020), and low equipment utilization (Lai et al., 2022). As a result, an effective energy storage profit mode has not yet been established, and there is a serious issue of storage system idleness (Song et al., 2021), indicating the urgent need for the commercialization of battery storage. In September 2020, the Federal Energy Regulatory Commission issued Order 2222, which allows energy storage companies to participate in the wholesale electricity market, laying the foundation for the deployment of energy storage business models (Federal Energy Regulatory Commission, 2020). In March 2022, China’s National Development and Reform Commission released the “14th Five-Year Plan” New Energy Storage Development Implementation Plan,” stating that by 2025, the new energy storage will begin its large-scale development from the initial stage of commercialization and have the conditions for large-scale commercial application (National Development and Reform Commission et al., 2022a). The support of national policies provides a solid foundation for the commercialization of energy storage.
The sharing economy is the phenomenon of peer-to-peer sharing of underutilized goods and services, placing utilization and availability above ownership (Cheng, 2016). As a new paradigm for improving resource utilization efficiency, the sharing economy provides ideas for the commercialization of traditional energy storage models (Lombardi and Schwabe, 2017; Jaeyeon and Jinkyoo, 2020; Henni et al., 2021). The shared energy storage model uses cost-sharing and economies of scale to solve the cost inefficiency of the original model. Shared energy storage enables all users to share its benefits by sharing the costs and making full use of power load complementarity. At the same time, because there is no need to build energy storage power stations independently, the original capital investment is reduced.
In recent years, the combination of the sharing economy and the energy Internet has led to a dramatic increase in the number of studies on shared energy storage, involving many fields such as economy, energy, and efficiencies (Hu et al., 2019; Dabbous and Tarhini, 2020). Dai et al. (2021) reviewed in detail the research related to the concept of shared energy storage, including the composition forms and application scenarios of shared energy storage. Yan and Chen. (2022) focused on the business model and pricing mechanism of shared energy storage, but there is no literature yet to systematically sort out the contradictory relationship between shared energy storage in energy coordination and fair pricing mechanism. The marginal contribution of this study is to review the common methods and models in the process of energy dispatch operation and individual benefit distribution, point out that the core contradiction of shared energy storage research is the efficiency of energy utilization and economic fairness, propose that the decentralized peer-to-peer transaction model based on blockchain technology can effectively alleviate this contradiction, and suggest the key research directions for the future.
2 Shared energy storage: Definition and application
Shared energy storage uses the power grid as a link; energy resources from independent and decentralized grid-side, power-side, and user-side energy storage in certain areas are optimized for the entire network. The power grid performs unified coordination to promote the full release of energy storage capabilities at all ends of the source, grid, and load. The direct realization of shared energy storage is a service provided by a public energy storage device for multiple users (Tascıkaraoglu, 2018; Terlouw et al., 2019). Public energy storage equipment can be jointly invested in and operated by all users (Chakraborty et al., 2019) or by a third party (Kang et al., 2017). The indirect realization of shared energy storage refers to the installation of a separate energy storage device for each user, who can only access their energy storage and conduct energy transactions or share with other users (Rahbar et al., 2018; Wang and Huang, 2018; Kong et al., 2020).
The separation of ownership and rights to use energy storage is the core idea of shared energy storage, that is, users of energy storage facilities lease the right to use idle energy storage resources to service providers at a certain price. In sharing energy storage, the utilization rate of the energy storage resources is improved. Consequently, the owner gains added benefits, thereby shortening the cost recovery cycle.
The United Kingdom, European Union, China, and United States have successively carried out the pilot and application of shared energy storage. The UK deployed shared energy storage on the user side in 2012, the EU deployed shared energy storage platforms in communities in 2016, and the US planned to connect batteries in Massachusetts by providing incentives to customers in 2019 Yan and Chen. (2022). The same year, China organized new energy power generation providers in Qinghai Province to conduct pilot transactions for shared energy storage peak-shaving auxiliary services (Dong et al., 2020).
With the application of shared energy storage in various scenarios and countries, shared energy storage to absorb renewable energy (Liu et al., 2021; Tercan et al., 2022), shared energy storage auxiliary services (Ma et al., 2022; Nagpal et al., 2022), and evaluation systems (Qiu et al., 2021; Shi et al., 2021) are all hot topics in research. However, we believe that the core issue is how to ensure the efficiency of resource allocation and the fairness of income distribution under the sharing economy. Many scholars have been concerned about the trade-off between efficiency and fairness in resource-sharing (Bertsimas et al., 2012; Joe-Wong et al., 2013). Here, we have described the main models and methods for the coordinated optimization of shared energy storage.
3 Efficiency and fairness of shared energy storage
The operation mode of shared energy storage is a coupling of the energy system and economic system, involving the issues of energy allocation efficiency and fair distribution of economic benefits among the participating subjects. The optimal scheduling of energy storage modules among multiple entities in a microgrid is key to the efficient operation of a power system. At the same time, users and shared energy storage operators, as independent participants, have conflicts in the distribution of benefits when they pursue the maximization of interests. The key to the efficient operation of a sharing economy is the design of a reasonable distribution mechanism.
3.1 Optimal scheduling of energy storage
Optimal scheduling of energy storage is also often referred to as the capacity sharing problem (Lombardi and Schwabe, 2017), mainly focusing on the allocation and scheduling of energy storage among multiple participants.
3.1.1 Single-stage problems
The single-stage model only considers the scheduling problem of energy storage without accounting for the initial capacity allocation (Wu et al., 2021). Early research was dominated by heuristic algorithms, Arghandeh et al. (2014) investigated optimal community energy storage schemes with real-time and day-ahead scheduling using a gradient-based heuristic optimization algorithm, Sardi et al. (2017a), Sardi et al. (2017b) examined a threshold control strategy for a shared energy storage operating system based on a genetic algorithm. The drawback of heuristic methods is that they can only state the relative optimum and cannot give the global optimum. In recent years, optimization and simulation-based approaches have become popular. Xie et al. (2019) used an online convex optimization algorithm to solve the problem of allocating energy storage resources to multiple household users. Schram et al. (2020) explored the trade-offs of shared energy storage operation plans based on the optimization of economic and environmental benefits through simulations. Walker and Kwon. (2021) used Mixed Integer Linear Programming (MILP) to discover potential patterns in the optimal operation of shared energy storage, providing insights for efficient control of shared energy storage. The use of optimization algorithms allows operators of shared energy storage to dispatch energy most efficiently.
3.1.2 Multi-stage problems
The multi-stage problems consider energy storage capacity installation along with energy allocation and dispatch (Wu et al., 2021). Common multi-stage problems can be summarized in a two-layer model, where the upper layer considers the capacity installation in the grid and the lower layer optimizes the allocation of energy storage (Long et al., 2018; Cui et al., 2019; Li et al., 2021; Wu et al., 2021; Ma et al., 2022; Wang et al., 2022), and such problems are characterized by trade-offs between the upper and lower layer models choices, where higher installation costs reduce the dispatch cost, and conversely, an insufficient initial capacity installation makes the allocation cost increase. The disadvantage of the model is that it treats the shared energy storage problem exclusively as an energy problem and ignores its economic properties. Another multi-stage problem regarding shared energy storage is to study the minimization of energy storage cost in the first stage and benefits allocation in the second stage (Li et al., 2021; Shuai et al., 2021; Li et al., 2022; Li et al., 2022b), and such model omits the energy deployment process and focuses only on the total energy use efficiency and the individual benefit allocation problem. The drawback is that the model results are only suitable for macroscopic analysis and not for specific applications because the details of dispatching are ignored. The common algorithms for solving multi-stage problems and the advantages of the algorithms are summarized in Table 1. The linear optimization algorithm (e.g., MILP) has high modeling requirements, requiring the model and constraints to all meet linearity, limiting the degree of freedom of the model, but the solution is faster and can be used to deal with larger-scale or long-duration scheduling problems. Heuristic algorithms (e.g., genetic algorithm, water filling algorithm) do not have linear limitations but the solving speed increases exponentially with the problem size. NSGA-II is an improvement of the genetic algorithm and can handle multi-objective optimization problems, but the solution speed is slower when the problem size is larger.
3.2 Benefit distribution mechanism
The scheduling problem of shared energy storage addresses the question of how a limited amount of energy should be used among multiple participants but does not address the distribution of sharing the consequential benefits and costs. Therefore, there is a further need to solve the problem of the optimal distribution of benefits, since a reasonable mechanism can ensure that participants do not leave the system. This kind of problem is known as the pricing mechanism for shared energy storage (Yan and Chen, 2022).
3.2.1 Allocation based on cooperative games
Cooperative games use negotiations and alliances to achieve efficient benefit distribution at a lower total cost. Common mechanisms are the Shapley value allocation approach (Li et al., 2021; Gao et al., 2022; Jiang et al., 2022), and the Nash bargaining method (Cui et al., 2021b; Chen et al., 2022; Huang et al., 2022; Liu et al., 2022). The Shapley method values the marginal contribution of the individual, whereas Nash bargaining focuses on maximizing individual returns. Jiang et al. (2022) proposed a cooperative game model under the framework of energy cells, using the Shapley value to allocate benefits and costs. Li et al. (2021) considered the Shapley values and individual differences to design the optimal allocation. The advantage of this method is fairness but the drawback is that the alliance is unstable and the participants may leave the large alliance and form a small alliance. Huang et al. (2022) constructed a multi-entity cooperative operation model based on the Nash negotiation theory and proved its effectiveness. Liu et al. (2022) used the generalized Nash bargaining method to establish a cooperation model that can overcome the “benefits increase” problem under the general method. The strength of this method is stability but the weakness is the need to know the negotiation rupture point of each participant. The common modeling approaches and solving methods are listed in Table 2. Since the revenue allocation problem involves multiple participants and usually has a large model size, studies often use the Alternating Direction Method of Multipliers (ADMM) algorithm in addition to the linear and heuristic optimization algorithms mentioned above. The characteristic of this algorithm is that the problem can be split into multiple subproblems for solving, so it is often chosen to improve the solution efficiency when facing large-scale solution problems. However, the problem is that the convergence speed is slow and the parameters in the convergence condition are difficult to determine.
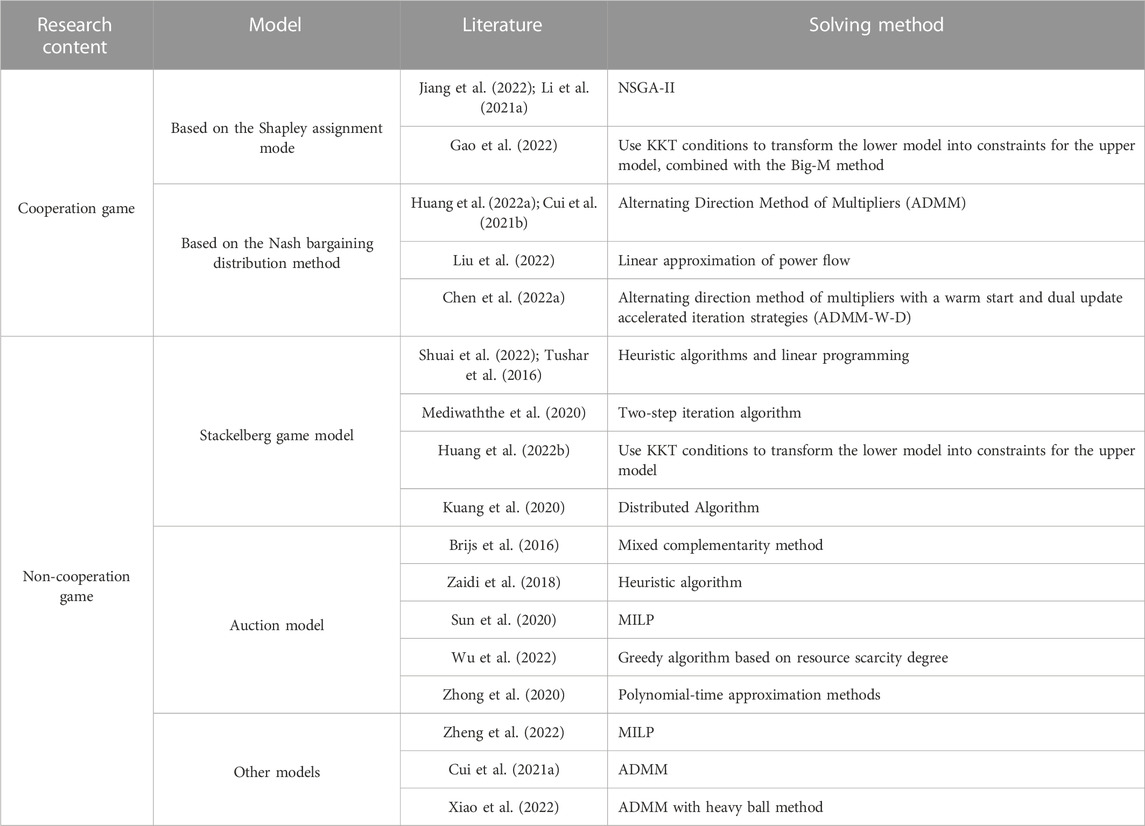
TABLE 2. Common solution methods for the optimal benefit distribution of the shared energy storage systems.
3.2.2 Allocation based on non-cooperative games
The theoretical basis of non-cooperative games is individual rationality, where the interests of the alliance cannot constrain individual decisions. The main goal of non-cooperative games is to solve the Nash equilibrium, and common modeling methods include master-slave games (Tushar et al., 2016; Mediwaththe et al., 2020; Kuang et al., 2020; Huang et al., 2022; Shuai et al., 2022), auctions (Brijs et al., 2016; Zaidi et al., 2018; Sun et al., 2020; Wu et al., 2021; Wu et al., 2022), and other models (Cui et al., 2021a; Xiao et al., 2022; Zheng et al., 2022). The master-slave game describes the optimal strategy between the shared energy storage operator (master) and participant (slave). Shuai et al. (2021) proved that the master-slave game model can achieve a win-win situation between microgrid operators and user aggregators. Huang et al. (2022) gave the energy framework for the shared energy storage trader based on the master-slave game and showed that everyone can benefit from it. Auction depicts competitive bidding among multiple users. Brijs et al. (2016) proposed a periodic auction mechanism for shared energy storage and demonstrated that the mechanism can reduce the overall risk. Sun et al. (2020) proposed a two-way auction mechanism that can break the monopoly drawbacks of one-way auctions and formed a mechanism where buyers and sellers share social benefits equally. The common modeling approaches and relevant solution methods are presented in Table 2. The distributed algorithm proposed by Kuang et al. (2020) can protect the privacy of participants, but is not scalable and cannot handle large-scale problems. The greedy algorithm based on resource scarcity used by Wu et al. (2022) allows for fast solving in polynomial time on large-scale problems, but with a slight loss in economic allocation efficiency. Polynomial time approximation methods proposed by Zhong et al. (2020) can solve the NP problem in the auction but may violate the resource supply constraint by allocating more resources to users than are sold in the auction. The non-cooperative game satisfies the maximization of individual interests, but the final equilibrium result will deviate from the social optimum and fail to maximize social welfare because of the conflict of interests between each other.
4 Discussion
The above analysis shows that existing research on shared energy storage faces a dilemma between efficiency in resource scheduling and fairness in revenue distribution. More information disclosed by the participants would improve the efficiency of resource dispatching, but this would affect the interests of individuals. Conversely, a fair distribution among individuals would affect the overall energy dispatching efficiency because everyone does not want to suffer losses.
Blockchain, as a decentralized and de-trusted computer technology, can provide effective support for building an efficient, multi-party trusted, transparent, and open trading platform, which can reasonably allocate revenue under the premise of guaranteeing that each entity has sufficient energy storage resources. Existing studies on blockchain and shared energy storage mostly focus on how to improve the efficiency of energy distribution under the P2P model (Long et al., 2018; Cao et al., 2021; Zheng et al., 2022), and how to design mechanisms to enhance the service capacity in the field of ancillary services (Luo and Shen, 2022; van Leeuwen et al., 2020; Xie et al., 2022; Zhang and Lu, 2022). The existing literature has still not fully exploited the advantages of blockchain-enabled technology in shared energy storage. Future research directions should make full use of the advantages of decentralization, information disclosure, automatic execution of smart contracts, and traceability of transaction records, and consider more details of energy deployment and benefit distribution in the actual process in the model. Specific feasible research directions include the following topics.
(1) Whether existing participants of shared energy storage should accept blockchain. Although a decentralized transaction model can reduce transaction costs and avoid the moral hazard of central operators, it also reduces transaction efficiency due to counterparty matching problems. Smart contracts in blockchain can solve this shortcoming, then how to design reasonable smart contracts to improve the deployment efficiency and benefit distribution so that the participants can accept the decentralized model is an important research content.
(2) The shared energy storage platform based on blockchain technology can record and disclose every transaction between users, making it possible to model as a dynamic game under incomplete information. Users can update their beliefs about the remaining nodes and adjust their optimal decisions based on the historical transaction information and the reputation of the transaction nodes.
(3) The manager of the shared energy storage platform can evaluate the creditworthiness of the nodes in the network based on the traceability of transaction information in the blockchain, and punish the nodes with a lower reputation in the future transaction process, such as lowering the transaction priority or increasing the transaction rate. What kind of regulation can reduce the default rate and improve the overall service quality is worthy of in-depth study.
5 Conclusion
Shared energy storage use can promote the consumption of renewable energy, improve the stability of power grid operation, reduce user installation costs, and achieve carbon neutrality and peaking. This study presents the concept and summarizes the current application scenarios for shared energy storage. From the discussion of the efficiency and fairness of shared energy storage, we conclude that methods that can effectively provide the optimization of the system to incentivize its use are lacking. Based on this finding, we propose that research should focus on the incorporation of blockchain technology within shared energy systems, owing to its valuable characteristics, including decentralization, data traceability, and transaction transparency.
Shared energy storage can promote the consumption of renewable energy, improve the stability of power grid operation, reduce user installation costs, and achieve carbon neutrality and peaking. This study presents the concept of shared energy storage, summarizes the current application scenarios, discusses the efficiency and fairness of shared energy storage through two themes-energy dispatch and benefit distribution, and concludes the following: 1) Energy dispatch emphasizes overall energy utilization efficiency while ignoring individual utility, and benefit distribution emphasizes economic efficiency among individuals while ignoring energy efficiency, with few articles jointly considering both economic and energy aspects. 2) The cooperative game in benefit distribution is fair and efficient but lacks stability, while the non-cooperative game is stable but often fails to achieve Pareto optimality. 3) The centralized role of the energy storage regulator in shared energy storage will improve the overall operational efficiency but increase the cost of each participant, and the decentralized operation mode can effectively solve this problem. Based on these, we point out that the features of blockchain technology can be used to solve the dilemma of energy efficiency and benefit distribution and give three perspectives from designing rational transaction mechanisms, adjusting gaming behavior under information disclosure, tracking nodes’ reputation, and providing feedback in transactions that can continue to be studied in depth.
Author contributions
SK proposed a topic and ideas for the minireview. DX and YW collected the relevant papers and sorted out the models and algorithms used in the study. DX participated in the whole discussion and gave professional advice. SK and YW assisted in polishing the manuscript.
Funding
This work was funded by the Research on energy storage safety monitoring and early warning system on the plateau user side from the perspective of the economy (Number: XZ202201YD0002C).
Conflict of interest
The authors declare that the research was conducted in the absence of any commercial or financial relationships that could be construed as a potential conflict of interest.
Publisher’s note
All claims expressed in this article are solely those of the authors and do not necessarily represent those of their affiliated organizations, or those of the publisher, the editors and the reviewers. Any product that may be evaluated in this article, or claim that may be made by its manufacturer, is not guaranteed or endorsed by the publisher.
References
Andrews-Speed, P. (2016). Applying institutional theory to the low-carbon energy transition. Energy Res. Soc. Sci. 13, 216–225. doi:10.1016/j.erss.2015.12.011
Arghandeh, R., Woyak, J., Onen, A., Jung, J., and Broadwater, R. P. (2014). Economic optimal operation of Community Energy Storage systems in competitive energy markets. Appl. Energy 135, 71–80. doi:10.1016/j.apenergy.2014.08.066
Bertsimas, D., Farias, V. F., and Trichakis, N. (2012). On the efficiency-fairness trade-off. Manag. Sci. 58, 2234–2250. doi:10.1287/mnsc.1120.1549
Brijs, T., Huppmann, D., Siddiqui, S., and Belmans, R. (2016). Auction-based allocation of shared electricity storage resources through physical storage rights. J. Energy Storage 7, 82–92. doi:10.1016/j.est.2016.05.009
Cao, S., Zhang, H., Cao, K., Chen, M., Wu, Y., and Zhou, S. (2021). Day-ahead economic optimal dispatch of microgrid cluster considering shared energy storage system and P2P transaction. Front. Energy Res. 9, 645017. doi:10.3389/FENRG.2021.645017
Chakraborty, P., Baeyens, E., Poolla, K., Khargonekar, P. P., and Varaiya, P. (2019). Sharing storage in a smart grid: A coalitional game approach. IEEE Trans. Smart Grid 10, 4379–4390. doi:10.1109/TSG.2018.2858206
Chen, C., Li, Y., Qiu, W., Liu, C., Zhang, Q., Li, Z., et al. (2022a). Cooperative-game-based day-ahead scheduling of local integrated energy systems with shared energy storage. IEEE Trans. Sustain. Energy 13, 1994–2011. doi:10.1109/TSTE.2022.3176613
Chen, J., Li, L., Cui, Y., Shen, F., Qiu, M., and Wei, T. (2022b). Utility-driven renewable energy sharing systems for community microgrid. J. Syst. Archit. 126, 102492. doi:10.1016/J.SYSARC.2022.102492
Chen, Y., Liu, Y., Hung, S., and Cheng, C. (2007). Multi-input inverter for grid-connected hybrid PV/wind power system. IEEE Trans. Power Electron. 22 (3), 1070–1077. doi:10.1109/tpel.2007.897117
Cheng, M. (2016). Sharing economy: A review and agenda for future research. Int. J. Hosp. Manag. 57, 60–70. doi:10.1016/j.ijhm.2016.06.003
Council of the European Union (2021). European climate Law. Avaliable At: https://data.consilium.europa.eu/doc/document/ST-8440-2021-INIT/en/pdf.
Cui, S., Wang, Y., Liu, X., Wang, Z., and Xiao, J. (2021a). Economic storage sharing framework: Asymmetric bargaining-based energy cooperation. IEEE Trans. Ind. Inf. 17, 7489–7500. doi:10.1109/TII.2021.3053296
Cui, S., Wang, Y., Shi, Y., and Xiao, J. (2021b). Community energy cooperation with the presence of cheating behaviors. IEEE Trans. Smart Grid 12, 561–573. doi:10.1109/TSG.2020.3022792
Cui, S., Wang, Y., Xiao, J. W., and Liu, N. (2019). A two-stage robust energy sharing management for prosumer microgrid. IEEE Trans. Ind. Inf. 15, 2741–2752. doi:10.1109/TII.2018.2867878
Dabbous, A., and Tarhini, A. (2021). Does sharing economy promote sustainable economic development and energy efficiency? Evidence from OECD countries. J. Innovation Knowl. 6, 58–68. doi:10.1016/J.JIK.2020.11.001
Dai, R., Esmaeilbeigi, R., and Charkhgard, H. (2021). The utilization of shared energy storage in energy systems: A comprehensive review. IEEE Trans. Smart Grid 12, 3163–3174. doi:10.1109/TSG.2021.3061619
Dong, L., Nian, H., Fan, Y., Zhao, J., Zhang, Z., and Lin, Z. (2020). Exploration and practice of business model of shared energy Storagein energy Internet. Electr. Power Constr. 04, 38–44. doi:10.3969/j.issn.1000–7229.2020.04.005
Energy research Centre of the Netherlands (2011). Renewable energy projections as published in the national renewable energy action plans of the European member states. Avaliable At: https://www.ecn.nl/publications/ECN-E–10-069.
Erdiwansyah, M., Mahidin, F., Husin, H., Nasaruddin, F., Zaki, M., and Muhibbuddin, N. (2021). A critical review of the integration of renewable energy sources with various technologiesAcritical review of the integration of renewable energy sources with various technologies. Prot. Control Mod. Power Syst. 6, 3. doi:10.1186/S41601-021-00181-3
Federal Energy Regulatory Commission (2020). Order 2222. Avaliable At: https://www.sohu.com/a/420013827_120597874.
Henni, S., Staudt, P., and Weinhardt, C. (2021). A sharing economy for residential communities with PV-coupled battery storage: Benefits, pricing and participant matching. Appl. Energy 301, 117351. doi:10.1016/J.APENERGY.2021.117351
Hu, J., Li, Q., Huang, B., and Feng, K. (2019). Business model research of energy storage on grid side adapted to application scenarios and policy environment in China. Glob. Energy Interconnect. 04, 367–375. doi:10.19705/j.cnki.issn2096-5125.2019.04.007
Huang, C., Liu, H., Ma, B., and Lu, H. (2022a). Research on optimal operation of shared energy-storage power station applying Nash negotiation. Electr. Power Constr. 02, 1–9. doi:10.12204/j.issn.1000.7229.2022.02.001
Huang, S., Gao, X., Chen, J., Chen, R., Su, Z., and Bao, J. (2022b). An optimal hierarchical pricing strategy for shared energy storage services. Front. Energy Res. 10. 967998, doi:10.3389/fenrg.2022.967998
International Energy Agency (2021). World energy outlook 2021. Avaliable At: https://www.iea.org/reports/world-energy-outlook-2021.
Jaeyeon, J., and Jinkyoo, P. (2020). Demand-side management with shared energy storage system in smart grid. IEEE Trans. Smart Grid. 5, 2980318. doi:10.1109/tsg.2020.2980318
Jianchao, J., Ruoyu, R., Pingkuo, P., and Lyuyang, L. (2021). A review and comparative analysis on energy transition in major industrialized countries. Int. J. Energy Res. 45, 1246–1268. doi:10.1002/er.5866
Jiang, C., Ou, Q., Wu, Z., Zhang, J., Yang, S., Zhu, J., et al. (2022). Joint configuration and utility analysis of multi-microgrid shared energy storagebased on alliance game. Electr. Power 55 (12), 11–21. Avaliable At: https://kns.cnki.net/kcms/detail/11.3265.tm.20220712.1417.002.html.
Jianwei, J., Fangjie, F., Yu, Y., Haoyu, H., Yi, Y., and Pengcheng, P. (2022). Configuration optimization and benefit allocation model of multi-park integrated energy systems considering electric vehicle charging station to assist services of shared energy storage power station. J. Clean. Prod. 336, 130381. doi:10.1016/J.JCLEPRO.2022.130381
Joe-Wong, C., Sen, S., Lan, T., and Chiang, M. (2013). Multiresource allocation: Fairness-efficiency tradeoffs in a unifying framework. IEEE/ACM Trans. Netw. 21, 1785–1798. doi:10.1109/TNET.2012.2233213
Kang, C., Liu, J., and Zhang, N. (2017). A new form of energy storage in future power system: Cloud energy storage. Autom. Electr. Power Syst. 21, 2–8. doi:10.7500/AEPS20170601011
Kong, X., Liu, D., Wang, C., Sun, F., and Li, S. (2020). Optimal operation strategy for interconnected microgrids in market environment considering uncertainty. Appl. Energy 275, 115336. doi:10.1016/j.apenergy.2020.115336
Kuang, Y., Wang, X., Wang, J., Peng, Q., Zhao, H., and Wang, X. (2020). Virtual power plant energy sharing mechanism based on Stackelberg game. Power Syst. Technol 12, 4556–4564. doi:10.13335/j.1000-3673.pst.2020.0683
Lai, S., Qiu, J., and Tao, Y. (2022). Individualized pricing of energy storage sharing based on discount sensitivity. IEEE Trans. Ind. Inf. 18, 4642–4653. doi:10.1109/TII.2021.3119953
Li, F., Li, X., Fang, Z., and Zhang, L. (2022a). A game optimization scheduling strategy of active distribution network with multi-microgrid sharing energy storage. Front. Energy Res. 10, 906406. doi:10.3389/FENRG.2022.906406
Li, S., Zhu, J., Chen, Z., and Luo, T. (2021b). Double-layer energy management system based on energy sharing cloud for virtual residential microgrid. Appl. Energy 282, 116089. doi:10.1016/j.apenergy.2020.116089
Li, X., Fang, Z., Li, F., Xie, S., and Cheng, S. (2022b). Game-based optimal dispatching strategy for distribution network with multiple microgrids leasing shared energy storage. Proc. CSEE 18, 6611–6625. doi:10.13334/j.0258-8013.pcsee.211424
Li, X., Hui, D., and Lai, X. (2013). Battery energy storage station (BESS)-Based smoothing control of photovoltaic (PV) and wind power generation fluctuations. IEEE Trans. Sustain. Energy 4 (2), 464–473. doi:10.1109/tste.2013.2247428
Li, X., Ma, H., and Jiang, Q. (2022c). Review of energy storage configuration technology on renewable energy side. Electr. Power 55 (01), 13–25. doi:10.11930/j.issn.1004-9649.202109032
Li, X., Xie, S., Fang, Z., Li, F., and Cheng, S. (2021a). Optimal configuration of shared energy storage for multi-microgrid and its cost allocation. Electr. Power Autom. Equip. 10, 44–51. doi:10.16081/j.epae.202110019
Liu, D., Zhao, N., Li, P., Liu, M., Xu, X., and Shao, P. (2021). Market-oriented consumption model based on the joint tracking of renewable energy generation curve of “shared energy storage and demand side resources”. Power Syst. Technol. 07, 2791–2802. doi:10.13335/j.1000-3673.pst.2020.1109
Liu, Y., Xu, Q., Yang, Y., Xia, Y., Fang, J., and Tang, L. (2022). Distribution network power flow constrained shared energy storage configuration for industrial consumers based on generalized NashBargaining theory. Power Syst. Technol. 38 (1), 1–14. doi:10.13335/j.1000-3673.pst.2022.1035
Lombardi, P., and Schwabe, F. (2017). Sharing economy as a new business model for energy storage systems. Appl. Energy 188, 485–496. doi:10.1016/j.apenergy.2016.12.016
Long, C., Wu, J., Zhou, Y., and Jenkins, N. (2018). Peer-to-peer energy sharing through a two-stage aggregated battery control in a community microgrid. Appl. Energy 226, 261–276. doi:10.1016/j.apenergy.2018.05.097
Luo, F., and Shen, X. (2022). Bidding model and trading mechanism of shared energy storage joint market based on blockchain. Electr. Meas. Instrum. 59 (8), 30–38. doi:10.19753/j.issn1001-1390.2022.08.004
Ma, M., Huang, H., Song, X., Peña-Mora, F., Zhang, Z., and Chen, J. (2022a). Zhang, Z.,and chen, JOptimal sizing and operations of shared energy storage systems in distribution networks: A bi-level programming approach. Appl. Energy 307, 118170. doi:10.1016/J.APENERGY.2021.118170
Ma, Y., Hu, Z., and Diao, R. (2022b). Day-ahead optimization strategy for shared energy storage of renewable energy power stations to provide frequency regulation service. Power Syst. Technol. 10, 3857–3868. doi:10.13335/j.1000-3673.pst.2021.1787
Mediwaththe, C. P., Shaw, M., Halgamuge, S., Smith, D. B., and Scott, P. (2020). An incentive-compatible energy trading framework for neighborhood area networks with shared energy storage. IEEE Trans. Sustain. Energy 11, 467–476. doi:10.1109/tste.2019.2895387
Ministry of Economy Trade, and Industry (2021). The Sixth strategic energy plan. Avaliable At: https://www.meti.go.jp/press/2021/10/20211022005/20211022005.html.
Nagpal, H., Avramidis, I. I., Capitanescu, F., and Madureira, A. G. (2022). Local energy communities in service of sustainability and grid flexibility provision: Hierarchical management of shared energy storage. IEEE Trans. Sustain. Energy 13, 1523–1535. doi:10.1109/TSTE.2022.3157193
National Development and Reform Commission (2022a). 14th five-year plan’ new energy storage development implementation plan. Avaliable At: www.gov.cn/zhengce/zhengceku/2022–03/22/content_5680417.htm.
National Development and Reform Commission (2022b). Implementation plan on promoting the high-quality development of new energy in the New Era. Avaliable At: www.gov.cn/zhengce/content/2022–05/30/content_5693013.htm.
Parthan, B., Osterkorn, M., Kennedy, M., Hoskyns, S. J., Bazilian, M., and Monga, P. (2010). Lessons for low-carbon energy transition: Experience from the renewable energy and energy efficiency partnership (REEEP). Energy Sustain. Dev. 14 (2), 83–93. doi:10.1016/j.esd.2010.04.003
Qiu, W., Wang, M., Lin, Z., Yang, L., Wang, L., and Sun, J. (2021). Comprehensive evaluation of shared energy storage towards new energy accommodation scenario under targets of carbon emission peak and carbon neutrality. Electr. Power Autom. Equip. 10, 244–255. doi:10.16081/j.epae.202110017
Rahbar, K., Chai, C. C., and Zhang, R. (2018). Energy cooperation optimization in microgrids with renewable energy integration. IEEE Trans. Smart Grid 9, 1482–1493. doi:10.1109/TSG.2016.2600863
Sardi, J., Mithulananthan, N., Gallagher, M., and Hung, D. Q. (2017a). Multiple community energy storage planning in distribution networks using a cost-benefit analysis. Appl. Energy 190, 453–463. doi:10.1016/j.apenergy.2016.12.144
Sardi, J., Mithulananthan, N., and Hung, D. Q. (2017b). Strategic allocation of community energy storage in a residential system with rooftop PV units. Appl. Energy 206, 159–171. doi:10.1016/j.apenergy.2017.08.186
Schram, W. L., AlSkaif, T., Lampropoulos, I., Henein, S., and van Sark, W. G. J. H. M. (2020). On the trade-off between environmental and economic objectives in community energy storage operational optimization. IEEE Trans. Sustain. Energy 11, 2653–2661. doi:10.1109/TSTE.2020.2969292
Shi, P., Shi, P., Wang, P., Wang, Y., and Che, S. (2021). Analysis and operation evaluation of power peak-shaving ancillary service market in North China. Autom. Electr. Power Syst. 20, 175–184. doi:10.7500/AEPS20201025003
Shuai, X., Ma, Z., Wang, X., Guo, H., and Zhang, H. (2022). Research on optimal operation of shared energy storage and integrated energy microgrid based on leader-follower game theory. Power Syst. Technol., 1–12. doi:10.13335/j.1000-3673.pst.2021.2191
Shuai, X., Wang, X., Wu, X., Zhang, H., Li, J., and Ma, Z. (2021). Shared energy storage capacity allocation and dynamic lease model considering electricity-heat demand response. Autom. Electr. Power Syst. 19, 24–32. doi:10.7500/AEPS20210518003
Song, T., Li, K., Han, X., Chen, W., Jia, Y., and Wen, F. (2021). Coordinated operation strategy of energy storage system participating in multiple application scenarios[J/OL]. Autom. Electr. Power Syst. 0530, 9002. doi:10.7500/AEPS20210129002
South Korea’s Ministry of Trade Industry, and Energy (2022). A draft long-term energy plan. Avaliable At: https://www.world-nuclear-news.org/Articles/Draft-Korean-energy-policy-reflects-expansion-of-n.
Sun, C., Zheng, T., Chen, L., Xie, Y., Gao, B., and Mei, S. (2020). Energy storage sharing mechanism based on combinatorial double auction. Power Syst. Technol. 05, 1732–1739. doi:10.13335/j.1000-3673.pst.2020.0186a
Tang, Y., Zhang, Q., Mclellan, B., and Li, H. (2018). Study on the impacts of sharing business models on economic performance of distributed PV-Battery systems. Energy 161, 544–558. doi:10.1016/j.energy.2018.07.096
Taşcıkaraoğlu, A. (2018). Economic and operational benefits of energy storage sharing for a neighborhood of prosumers in a dynamic pricing environment. Sustain. Cities Soc. 38, 219–229. doi:10.1016/j.scs.2018.01.002
Tercan, S. M., Demirci, A., Gokalp, E., and Cali, U. (2022). Maximizing self-consumption rates and power quality towards two-stage evaluation for solar energy and shared energy storage empowered microgrids. J. Energy Storage 51, 104561. doi:10.1016/J.EST.2022.104561
Terlouw, T., AlSkaif, T., Bauer, C., and van Sark, W. (2019). Multi-objective optimization of energy arbitrage in community energy storage systems using different battery technologies. Appl. Energy 239, 356–372. doi:10.1016/j.apenergy.2019.01.227
Tushar, W., Chai, B., Yuen, C., Huang, S., Smith, D. B., Poor, H. V., et al. (2016). Energy storage sharing in smart grid:A modified auction-based approach. IEEE Trans. Smart Grid 7, 1462–1475. doi:10.1109/TSG.2015.2512267
United States Department of Energy (2022). Offshore wind energy strategy. Avaliable At: https://www.energy.gov/eere/wind/offshore-wind-research-and-development.
van Leeuwen, G., AlSkaif, T., Gibescu, M., and van Sark, W. (2020). An integrated blockchain-based energy management platform with bilateral trading for microgrid communities. Appl. Energy 263, 114613. doi:10.1016/j.apenergy.2020.114613
Walker, A., and Kwon, S. (2021). Analysis on impact of shared energy storage in residential community: Individual versus shared energy storage. Appl. Energy 282, 116172. doi:10.1016/j.apenergy.2020.116172
Wang, H., and Huang, J. (2018). Incentivizing energy trading for interconnected microgrids. IEEE Trans. Smart Grid 9, 2647–2657. doi:10.1109/tsg.2016.2614988
Wang, Q., Zhang, X., Yi, C., Li, Z., and Xu, D. (2022). A novel shared energy storage planning method considering the correlation of renewable uncertainties on the supply side. IEEE Trans. Sustain. Energy 13, 2051–2063. doi:10.1109/TSTE.2022.3179837
Wu, S., Li, Q., Liu, J., Zhou, Q., and Wang, C. (2021). Bi-level optimal configuration for combined cooling heating and power multi-microgrids based on energy storage station service. Power Syst. Technol 10, 3822–3832. doi:10.13335/j.1000-3673.pst.2020.1838
Wu, Z., Zhou, M., Wang, J., Li, A., Chen, L., and Li, G. (2022). Operation method of shared energy storage for urban power energy based on combinatorial auction. Autom. Electr. Power Syst. 17, 83–90. doi:10.7500/AEPS20211229003
Xiao, J. W., Yang, Y. B., Cui, S., and Liu, X. K. (2022). A new energy storage sharing framework with regard to both storage capacity and power capacity. Appl. Energy 307, 118171. doi:10.1016/J.APENERGY.2021.118171
Xie, K., Zhong, W., Li, W., and Zhu, Y. (2019). Distributed capacity allocation of shared energy storage using online convex optimization. Energies 12, 1642. doi:10.3390/en12091642
Xie, Y., Yu-Lee, Y., Chang, X., Yin, X., and Zheng, H. (2022). Research on the transaction mode and mechanism of grid-side shared energy storage market based on blockchain. Energy Rep. 8, 224–229. doi:10.1016/j.egyr.2021.11.044
Yan, D., and Chen, Y. (2022). Review on business mode and pricing mechanism for shared energy storage. Autom. Electr. Power Syst. 46 (23), 178–191. doi:10.7500/AEPS20220219003
Yang, Y., Bremner, S., Menictas, C., and Kay, M. (2022). Modelling and optimal energy management for battery energy storage systems in renewable energy systems: A review. Renew. Sustain. Energy Rev. 167, 112671. doi:10.1016/J.RSER.2022.112671
Yang, Y. Q., Bremner, S., Menictas, C., and Kay, M. (2018). Battery energy storage system size determination in renewable energy systems: A review. Renew. Sustain. Energy Rev. 91, 109–125. doi:10.1016/j.rser.2018.03.047
Yang, Y., Solgaard, H. S., and Haider, W. (2016). Wind, hydro or mixed renewable energy source: Preference for electricity products when the share of renewable energy increases. Energy Policy 97, 521–531. doi:10.1016/j.enpol.2016.07.030
Zaidi, B. H., Bhatti, D. M. S., and Ullah, I. (2018). Combinatorial auctions for energy storage sharing amongst the households. J. Energy Storage 19, 291–301. doi:10.1016/j.est.2018.08.010
Zhang, W., and Lu, X. (2022). Sharing and self-operating multi-mode trading model of energy storage aggregators with peer-to-peer trade. Autom. Electr. Power Syst. 02, 15–23. doi:10.7500/AEPS20210304002
Zhao, D., Wang, H., Huang, J., and Lin, X. (2020). Virtual energy storage sharing and capacity allocation. IEEE Trans. Smart Grid 11, 1112–1123. doi:10.1109/tsg.2019.2932057
Zheng, B., Wei, W., Chen, Y., Wu, Q., and Mei, S. (2022). A peer-to-peer energy trading market embedded with residential shared energy storage units. Appl. Energy 308, 118400. doi:10.1016/J.APENERGY.2021.118400
Keywords: shared energy storage, energy scheduling, profit distribution, efficiency, fairness
Citation: Kong S, Wang Y and Xie D (2023) Battery energy scheduling and benefit distribution models under shared energy storage: A mini review. Front. Energy Res. 11:1100214. doi: 10.3389/fenrg.2023.1100214
Received: 16 November 2022; Accepted: 17 January 2023;
Published: 30 January 2023.
Edited by:
Lingna Liu, China University of Geosciences, ChinaReviewed by:
Jun Zhao, University of International Business and Economics, ChinaCopyright © 2023 Kong, Wang and Xie. This is an open-access article distributed under the terms of the Creative Commons Attribution License (CC BY). The use, distribution or reproduction in other forums is permitted, provided the original author(s) and the copyright owner(s) are credited and that the original publication in this journal is cited, in accordance with accepted academic practice. No use, distribution or reproduction is permitted which does not comply with these terms.
*Correspondence: Dongwei Xie, MjAyMDEwMzYxNUBydWMuZWR1LmNu