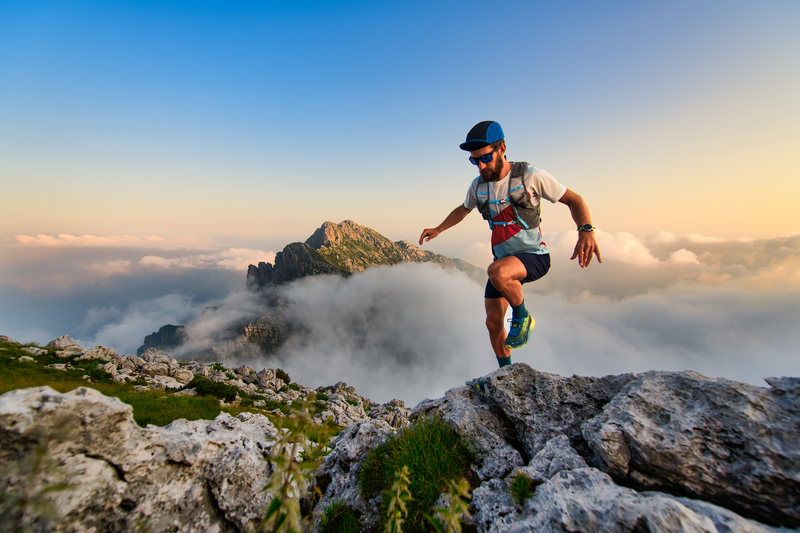
94% of researchers rate our articles as excellent or good
Learn more about the work of our research integrity team to safeguard the quality of each article we publish.
Find out more
ORIGINAL RESEARCH article
Front. Energy Res. , 15 September 2022
Sec. Sustainable Energy Systems
Volume 10 - 2022 | https://doi.org/10.3389/fenrg.2022.981097
In order to actively respond to the global climate and environmental challenges, and to help achieve the goal of carbon peaking and carbon neutrality, China aims to achieve carbon peaking by 2030. As the main contributor of energy consumption, construction industry transformation is imminent. This paper focuses on the development trend of carbon emissions in Anhui construction industry in the next 20 years, and how to help Anhui construction industry achieve the carbon peak target. The research process and conclusions are as follows: 1) Through literature review and gray correlation analysis, seven important factors affecting the construction industry are identified. 2) The gray prediction EGM(1,1) model was adopted to forecast the carbon emissions of the construction industry in Anhui Province from 2021 to 2040. The research results show that carbon emissions will increase year by year in the next 20 years. 3) The EGM-BP neural network model was used to further predict the carbon emissions from the construction industry in 2021–2040. It is expected that the carbon emissions from construction industry in Anhui Province will show an “inverted U″ trend in the next 20 years, and is expected to reach its peak in 2031, after which it will start to decline. Based on the results of the above study, we provide support paths for achieving the carbon peak in the construction industry from the perspective of improving and developing the construction system, and provide policy suggestions for relevant departments to accelerate the construction industry and carbon peak construction in Anhui Province.
Since the industrial revolution, the human ability to develop and utilize natural resources has been increasing, underground mineral deposits have been mined and smelted in large quantities, fuel consumption has increased dramatically, and carbon dioxide emissions have increased significantly with the large-scale development and use of fossil fuels such as coal, oil and natural gas. At the same time, the industrial revolution has also accelerated the urbanization process, large-scale urban construction has destroyed the original land environment, and the industrial “three wastes” brought about by urban production and living have dried up the environmental ecology of the region directly threatening human health. In response the United Nations Intergovernmental Panel on Climate Change (IPCC) released the “IPCC Special Report on Global Warming of 1.5 °C″, which emphasized that industrialization has made the global temperature increase by about 1.0 °C than before, and if not controlled, the global warming will be 1.5 °C in 2030–2052. To limit warming to 1.5°C, global carbon emissions need to be halved by 2030 and reach net zero by the middle of the century (IPCC., 2020).
At present, 49 countries in the world have reached the carbon peak, and more than 120 governments have pledged to achieve carbon neutrality. As the world’s largest developing country in terms of carbon emissions, the Chinese government attaches great importance to and has adopted a series of policy measures. In 2016, China signed the Paris Agreement, promising to work with other countries in the world to face the global climate change that mankind is facing (Zhang et al., 2022). On 22 September 2020, the Chinese government promised at the United Nations General Assembly that China would strive to achieve carbon peak by 2030 and carbon neutrality by 2060. In March 2021, the government work report of the State Council pointed out that we should do a solid job on carbon peaking and carbon neutralization, formulate an action plan for reaching the peak of carbon emissions by 2030, and optimize the industrial structure and energy structure.
The United Nations Environment Programme points out that the construction industry accounts for 36% of global final energy consumption and 37% of energy-related carbon dioxide emissions in 2020 (Song et al., 2019). In order to cope with the global climate change problem and promote carbon emission reduction, the National Plan to Address Climate Change proposes that a sound basic statistical system for greenhouse gas emissions should be established for greenhouse gas emission standards in key industries such as the construction industry, and the issue of carbon emissions from the construction industry has become a strategic concern for China. In recent years, the output value of China’s construction industry has been climbing year by year, and by the end of 2021 it was close to 300,000 billion yuan, an increase of 11.04% year on year. As a major carbon emitter, the construction industry has always had the problem of high resource consumption and high pollution emissions, and as China’s urbanization continues to increase, carbon emissions from the construction production process are also climbing. The China Building Energy Conservation Society issued the China Building Energy Consumption Research Report 2020, which pointed out that carbon dioxide emissions accounted for 51.2% of the national energy carbon emissions in the whole life cycle of buildings in 2018 (China Association of Building Energy Efficiency, 2021). This shows that buildings have become a major contributor to energy consumption in China and worldwide. The construction industry is an important area of end-use energy consumption and CO2 emissions. With the increasing urbanization, the new construction area in China is increasing year by year, which poses a great challenge to achieve the double carbon target. Therefore, it is urgent for the construction industry to actively respond to the challenges of global climate environment and help achieve the goal of carbon peak and carbon neutrality.
In recent years, the academic community has done a lot of research on the carbon emission reduction of the construction industry, involving the calculation of the carbon emission of the construction industry, the analysis of the influencing factors of carbon emission, the prediction of the peak neutralization of carbon in the construction industry and the research on the path of carbon emission reduction.
Zhang and Liu. (2013) divided the carbon emissions of the construction industry into direct carbon emissions and indirect carbon emissions, introduced the input-output method into the calculation of carbon emissions of the construction industry to calculate the indirect carbon emissions, and put forward the concept of correlation carbon emission coefficient and carbon emission pull coefficient. Feng et al. (2014) established a model according to the IPCC carbon emission calculation method, calculated the construction carbon emissions of each province, and analyzed the decoupling status of the construction industry in each province. Zhou J. et al. (2019) used the GVAR model to study the driving factors of carbon emission efficiency of the construction industry. The empirical results show that technological progress and energy structure adjustment can promote the improvement of carbon emission efficiency of the construction industry, but the expansion of economic scale is of great significance to the improvement of carbon emission efficiency of the construction industry. Li D et al. (2020) used the LMDI decomposition method to analyze the drivers of carbon emissions in the construction industry in Jiangsu Province, and the results show that the area factor and the output intensity factor have the greatest impact on carbon emissions. Chi et al. (2021) Used the STIRPAT model to study the factors influencing carbon emissions in the construction industry in China, the study shows that floor area and indirect emission intensity have the greatest influence on carbon emissions in the construction industry. There are also more studies on carbon emission forecasting in the construction industry. Li B et al. (2020) used scenario analysis to predict the peak carbon emissions of China’s construction industry under three different scenarios from 2018–2045 and examines the key factors affecting carbon emissions. The results show that GDP has the highest cumulative contribution to carbon emissions from China’s construction industry, labor productivity and output carbon intensity have a suppressive effect on carbon emissions from the construction industry, followed by a peak in 2045 under the baseline scenario, a peak in 2030 under the low-carbon scenario, and a peak in 2020 under the technological breakthrough scenario. Chi et al. (2021) constructed a provincial CO2 emission measurement model for China’s construction industry, and after the study, he concluded that CO2 emissions from China’s construction industry show a trend of increasing and then decreasing, and it is expected that carbon emissions from the construction industry will reach a peak in 2034. Wang G et al. (2022) predicted the peak carbon emission of public buildings in China. The research shows that under the basic scenario, the carbon emission of public buildings will reach the peak in 2041, and under the low-carbon scenario, it will reach the peak in 2030. Jiang L et al. (2022) studied the energy related carbon emissions of China’s construction industry from 2007 to 2017. The study found that optimizing the energy consumption structure of the construction industry has a significant emission reduction effect on the growth of energy carbon emissions of China’s construction industry, and provided suggestions for China’s construction industry from the aspects of supply side energy varieties and demand side industries.
For the construction industry, in order to achieve the strategic goal of carbon peak and carbon neutralization, it not only depends on the national macro-control, but also makes targeted carbon reduction countermeasures according to the local actual situation. At present, there are few studies on the dual carbon related to the provincial construction industry. This paper starts with the actual situation of the construction industry in Anhui Province, and uses EGM (1.1) model and BP neural network to predict the carbon emission trend of the construction industry in Anhui Province from 2021 to 2040. Explore the key factors that affect the carbon emission of construction industry in Anhui Province, and provide practical suggestions for the carbon reduction work in Anhui Province.
Anhui Province is located in the Yangtze River Delta in East China, with a total area of 140100 square kilometers. By the end of 2021, Anhui has a permanent population of 61.13 million. With the development of economy, by 2020, there are 5878 construction enterprises in Anhui Province, with 2037300 employees and a total output value of 93651164 yuan. The total output value of the construction industry accounts for 25% of the GDP of Anhui Province, and the scale of the construction industry continues to expand. The large proportion of the construction industry in the economy shows the importance of the construction industry for the economic development of Anhui Province. The development of the construction industry has also brought many impacts on the environment, and the economic development has also increased the difficulty of carbon emission reduction. This paper selects Anhui Province as the research object, as shown in Figure 1. To study the development trend of carbon emissions from the construction industry in Anhui Province, explore the path to reach the peak of carbon emissions in the construction industry in Anhui Province, and put forward policy suggestions for achieving the goal of reaching the peak of carbon emissions in Anhui Province.
The data involved in this paper are from the statistical yearbook and Anhui construction industry statistical yearbook on the website of Anhui Provincial Bureau of statistics or the National Bureau of statistics. According to the research object of the article, the basic data of Anhui Province from 2006 to 2020 are selected as the research data, and some index data are obtained through grey correlation analysis.
The carbon emission of the construction industry mainly comes from two parts. The first is direct carbon emission (
Grey system theory is a system science theory initiated by Professor Deng Julong in 1982. It is one of the important achievements in the field of uncertain system research. The grey correlation analysis in the grey system theory refers to the quantitative description and comparison of the development and change trend of a system. It is to judge whether the relationship is close by determining the geometric similarity of the reference series and several comparison series. It reflects the correlation degree between curves (Yi and Liu, 2011). Relevance is a measure of the relevance between things and factors. It quantitatively describes the mutual changes between things or factors, that is, the relativity of the magnitude, direction and speed of changes. If the change situation of things or factors is basically the same, it can be considered that the degree of correlation between them is large, on the contrary, the degree of correlation is small. For carbon emission related research, Chinese scholars often use regression, correlation and other statistical analysis methods, but the above methods often require large enough data and obvious distribution characteristics. For the data with small amount of data and less obvious sample law, grey correlation analysis can be used to process the data. Relatively speaking, grey correlation analysis requires less data, has low requirements for data, has simple principle, is easy to understand and master, and overcomes and makes up for the above shortcomings. Therefore, this paper uses grey correlation analysis to screen the influencing factors of carbon emissions from the construction industry and get the key factors. The steps of grey correlation analysis method are as follows (Liu et al., 2013):
First step, set reference sequence.
Comparison sequence.
Second step, normalization of data. The calculation formula is:
Third step, the absolute difference is obtained by subtracting the reference series from the comparison series. The calculation formula is as follows:
Fourth step, calculate the number of gray correlation coefficients. The calculation formula is as follows:
Fifth step, calculate the gray correlation degree. The calculation formula is as follows:
After professor Deng Julong announced the birth of grey system theory in 1982, after decades of development, it has become a new discipline and is increasingly widely used. Grey system theory is a set of theories and methods to solve grey system, which extends the viewpoints and methods of general system theory, information theory and Cybernetics to abstract systems such as society, economy and ecology, combined with mathematical methods. Grey system theory has been successfully applied to many fields such as industry, agriculture, society and economy, and has solved a large number of practical problems in production, life and scientific research. The grey system theory takes small data, poor information and uncertain systems with some known information and some unknown information as the research object. It mainly extracts valuable information through mining some known information, so as to realize the correct description and effective monitoring of the system operation behavior and evolution law. Among them, the grey prediction model is one of the most active and widely used grey system models. It has a good prediction effect for this kind of data with few sample years.
Shaheen et al. (2020) studied the impact of Turkey’s economic growth on environmental degradation through GM (1.1) model. The research results show that power utilization and economic growth have accelerated carbon dioxide emissions. In the long run, agriculture, forestry and fisheries have reduced Turkey’s environmental pollution. Jiang et al. (2021) established a GM (1.1) prediction model to predict the carbon emissions and added value of various industries in China. The results confirm that the carbon emissions of various industries in China are on the rise and will be significantly reduced by 2030. Wang G et al. (2022) used GM (1.1) and BP neural network to study the trend of China’s carbon emission reduction in the next 40 years under the influence of the development of green finance. The research results show that China’s carbon dioxide emissions are expected to show an “inverted V″ trend in the next 40 years. It is expected that China’s carbon dioxide emissions will reach the carbon peak in 2032 and reach carbon neutrality in 2063. Therefore, this paper establishes a EGM (1.1) grey prediction model to simulate the carbon emission data of Anhui construction industry from 2011 to 2020, and then predicts the carbon emission data from 2021 to 2040. The method of constructing EGM (1.1) model is as follows (Wang Y et al., 2022):
First step, set the original sequence:
Generate a new series by accumulating once:
Convert to mean form:
Second step, establishing the whitening differential equation. That is the EGM(1,1) model,
Combine (12) with (13):
Third step, averaging the accumulated data to generate B and the constant term vector Yn,
Use the least square method to get
Fourth step, the time response equation is obtained as follows:
BP neural network was proposed by a research team led by Rumelhart and McClellan in 1986. It is a multilayer feedforward network trained based on error back propagation algorithm. It is one of the most widely used neural network models at present. BP neural network has a strong nonlinear learning ability. In fact, it is a parallel distributed processor composed of multiple neurons. It can learn the data, master the ability to classify and judge the data, and store the experience gained through learning. Neural network can get some skills from the outside through the learning and training process. After the learning process, it will respond to the external stimuli encountered in the learning process according to the learned rules. BP neural network model has input layer, hidden layer and output layer. The basic principle is shown in Figure 2.
The number of input layer nodes is n, and the number of hidden layer nodes is m,
The data is calculated and corrected by forward propagation and error back propagation. This process is repeated until the error is less than the previously set target error. Zhou et al. (2018) used the improved BP neural network to predict the carbon emissions under different scenarios in Beijing, Tianjin and Hebei. The results show that under the low-carbon scenario, the carbon emissions will be in the declining stage from 2015 to 2018, and will be in the rising stage from 2019 to 2030. Under the basic and high carbon scenarios, carbon emissions will peak in 2025 and 2028 respectively. Chai et al. (2022) used BP neural network to predict the carbon emission trend in Xinjiang. The research shows that the peak time of low-carbon scenario is the earliest, between 2029 and 2033. From 2032 to 2037, the peak time of the baseline scenario is later than that of the low-carbon scenario, while the peak time of the high-carbon scenario is the latest, which is unlikely to arrive before 2050.
In recent years, many scholars have conducted relevant research on the influencing factors of carbon emissions from the construction industry. Through literature review, the influencing factors of carbon emissions from the construction industry are identified, and the identification results are shown in Table 2.
Through the data collection of the statistical yearbook of Anhui Province and the statistical yearbook of China’s energy from 2006 to 2020, the relevant data are substituted into the carbon emission calculation formula of the construction industry 1) to obtain the carbon emission of the construction industry in Anhui Province. Basic data are shown in Table 3.
Take the carbon emission of construction industry in Anhui Province as the reference sequence and the other 10 influencing factors as the comparison sequence, the correlation degree between the factors can be obtained by substituting into the correlation degree calculation formula, as shown in Table 4.
According to the correlation degree of each factor, the factors that affect the carbon emission of construction industry in Anhui Province from large to small are: per capita GDP of construction industry, labor productivity, energy consumption of construction industry, building area, total output value of construction industry, number of construction industry employees, urbanization rate, industrial structure, total population and energy consumption intensity of construction industry.
In this paper, we use MATLAB software to establish a gray prediction model to simulate and forecast the carbon emissions of construction industry in Anhui Province from 2021 to 2040 based on the relevant data of Anhui Province from 2006 to 2020, and the simulation and forecast results are shown in Figure 3. The accuracy table of the prediction model calculation is shown in Table 5. After calculation, the variance ratio of this prediction model is C = 0.35, and the probability of small error p = 1. Comparing with the accuracy table of the prediction model calculation, it can be concluded that the accuracy grade of this prediction model is good.
Through the grey prediction model, the prediction of the carbon emissions of the construction industry in Anhui Province from 2006 to 2040 can be seen that the actual value from 2006 to 2020 is basically consistent with the predicted value, and the error is small, indicating that the prediction fitting is good. However, due to the weak trend change of grey prediction model, it can only show a monotonic increasing or decreasing trend. Therefore, it is necessary to further improve the prediction of carbon emissions.
According to the calculation of grey correlation degree, seven factors such as per capita GDP of construction industry, labor productivity, energy consumption of construction industry, building area, total output value of construction industry, number of construction industry employees, urbanization rate and industrial structure are selected as the input values of BP neural network model. The carbon emission of Anhui construction industry from 2006 to 2020 is taken as the output variable of BP neural network. Then the input layer is seven and the output layer is 1. According to the empirical formula, the number of neurons in the hidden layer is
It can be seen from Figure 4 that the correlation coefficient between the output value of the training sample and the expected value is 0.93503, the correlation coefficient between the validation sample and the test sample is 0.99999, and the correlation coefficient of the whole sample is 0.94014. The data regression is good. The fitting diagram between the actual value and the predicted value of carbon emissions from the construction industry in Anhui Province from 2006 to 2020 as shown in Figure 5A. According to the fitting curve, the deviation between the actual data and the expected data is small, and the generalization ability of the training network is strong. This model is suitable for the prediction of carbon emissions from construction industry in Anhui Province.
FIGURE 5. Prediction results of BP neural network model (A) Fitting diagram of actual and predicted carbon emissions of construction industry in Anhui Province from 2006 to 2020 (B) Predicted value of carbon emission from construction industry in Anhui Province from 2021 to 2040.
Use MATLAB software to predict the carbon emission of construction industry in Anhui Province from 2021 to 2040. The results are shown in Figure 5B.
According to the gray prediction results, the carbon emissions of construction industry in Anhui Province will continue to grow in the next 20 years and fail to reach the peak of carbon emissions by 2030. However, the gray prediction model tends to show only a single trend of change, with the development and innovation of building technology and the state’s attention to the dual carbon strategic goal, energy consumption will not increase all the time. China plans to achieve carbon peak in 2030 and carbon neutralization in 2060. At present, all industries and regions are actively responding to the national call for energy conservation and carbon reduction, and have achieved some good results. Therefore, it can be expected that the carbon emissions of the construction industry in Anhui Province will fluctuate or gradually decrease in the future.
According to the simulation results of BP neural network, the carbon emission of Anhui province construction industry will show an “inverted U″ trend. Until 2031, the carbon dioxide emissions have been rising without decreasing trend, mainly because the construction industry accounts for a large proportion of the national economy and is a pillar industry in China, and it is difficult to change the existing industrial structure and energy consumption mode in a short time, so it does not reach the peak of carbon emissions before 2030. After the macro policy regulation and control as well as technological innovation, the carbon emission of construction industry in Anhui Province has been continuously reduced from 2031. This proves the effectiveness of a series of policy measures adopted in Anhui Province. However, there is still a gap between the predicted results and China’s dual carbon strategy goals, indicating the need for Anhui Province to make efforts in many aspects. Aiming at the impact of the development of the construction industry on the realization of the carbon peak goal, this paper puts forward relevant policy suggestions for the development and improvement of the construction industry system, so as to promote the realization of the carbon peak goal in China. In this paper, the factors affecting the carbon peak of the construction industry are screened according to previous studies, and other factors affecting carbon emissions are not considered. Therefore, the best prediction ability of BP neural network may not be achieved when establishing BP neural network model.
This paper analyzes the influencing factors affecting the carbon emissions of the construction industry, predicts the carbon emissions of the construction industry in Anhui Province, and puts forward the following suggestions about the construction industry in view of the prediction results and China’s carbon peak carbon neutrality target (Figure 6).
Firstly, the green and low-carbon requirements are integrated into urban and rural planning and management. In urban and rural planning, the selection of building addresses, environmental planning and design, settlement traffic planning, and regional energy planning will have a significant impact on carbon emissions in the later use phase, so the building energy consumption in the whole life cycle must be considered in the building planning and design phase. The relevant departments should according to the urban development and population size to plan the residential areas, reduce unnecessary human and material resources consumption. Government should control the construction of high energy consumption public buildings, promote the development of urban clusters, and build urban ecological ventilation pipes Reduce urban heat island effect, thus reducing building energy consumption.
Secondly, reduce the energy consumption of building material production. Currently, China’s carbon emissions from construction materials account for 9–12% of the whole-life carbon emissions of buildings (Liu et al., 2009). In the global reduction trend, the work of CO2 removal of construction materials will also become an essential part of China’s overall CO2 reduction plan. In the whole life cycle construction of buildings, indirect carbon emissions account for a large proportion of total carbon emissions, the consumption of building materials is increasing, the CO2 generated during the production and use of building materials continues to grow, which means that must innovate the production process of building materials and develop low-carbon building materials. In the production process of building materials, clean energy and renewable energy are used instead of traditional coal, oil and other energy sources to reduce energy consumption in building materials. At present, the building materials industry has begun to transform and develop towards more environmentally new energy such as photovoltaic and wind power. It has driven the development of solar energy, glass and other industrial chains and become a supporting industry for the new energy industry.
Thirdly, implement green construction during building construction. Development and promotion of prefabricated buildings. Prefabricated building is the development trend of construction industry in recent years. Parts of prefabricated buildings are produced in different places and then transported to the site for assembly. Using prefabricated buildings can reduce the workforce on the construction site, shorten the construction period and reduce the material loss. It is the representative of modern industrial green production mode (Chang et al., 2018). Promote and establish zero-carbon public buildings. Zero-carbon buildings can not consume coal, oil and other resources. The energy consumption of the whole year is provided by the renewable energy generated by the site. The zero-carbon pavilion at the Shanghai World Expo is the first zero-carbon building in China built with localized products, which fits the theme of the Shanghai World Expo: “Better City, Better Life".
Finally, adjust the energy consumption structure. Apply green energy to the whole life cycle of buildings, such as solar energy, wind energy and Hydraulic energy. The design of solar power generation and collection device shall be included in the design of the main structure of the building, and shall be installed, constructed and put into use simultaneously with the building. At present, China’s coal still accounts for more than half of energy consumption, from the experience of developed countries, control the total amount of energy consumption, optimize the consumption structure is the most important way to achieve carbon emission reduction. For the construction industry, it should strictly control the energy consumption structure in the production and processing of building materials as well as building construction and construction, use green energy instead of fossil energy, and implement the use of green energy into the whole life cycle of the building, accelerate technological innovation, develop green technology with green consciousness as the guide, reduce the amount of energy input and the output of polluting waste in the production process, improve energy utilization efficiency, and Reduce the intensity of energy consumption.
This paper uses gray correlation analysis to screen the key factors affecting carbon emissions in the construction industry, and seven factors are selected based on the analysis results: GDP per capita in the construction industry, labor productivity, energy consumption in the construction industry, construction area, total construction output, number of employees in the construction industry, urbanization rate and industrial structure to forecast the future carbon emissions in the construction industry of Anhui Province. A gray GM (1.1) model was used to predict the carbon emissions from construction industry in Anhui Province from 2021 to 2040, and the model accuracy was good and the prediction results were reliable. The data showed that the future carbon emissions from the construction industry in Anhui Province will show an increasing trend. Then, the BP neural network model was used to further predict the carbon emissions from the construction industry in Anhui Province, and the model fit was good. The conclusion shows that the carbon emissions from construction industry in Anhui province show an “inverted U″ trend, increasing year by year until 2031, reaching the peak in 2031, and decreasing year by year after 2031. Meanwhile, this paper makes suggestions for the future development of the construction industry in Anhui Province based on the prediction results and China’s carbon peak neutralization target.
The original contributions presented in the study are included in the article/supplementary material further inquiries can be directed to the corresponding author.
DD, Conceptualization, Methodology, Supervision. KL, Software, Formal analysis, Visualization, Writing—Original draft. SZ, Software, Validation, Writing—Reviewing and Editing. BZ, Data curation. All authors have read and agreed to the published version of the manuscript.
The paper is supported by the Special Topic of Spirit Research and Interpretation of the Sixth Plenary Session of the 19th CPC Central Committee of AUST (sjjlzqh 2021-15), the Postgraduate Innovation Fund Project of AUST (2021CX1013) and the Scientific Research Education Demonstration Project of AUST (KYX202123).
The authors declare that the research was conducted in the absence of any commercial or financial relationships that could be construed as a potential conflict of interest.
All claims expressed in this article are solely those of the authors and do not necessarily represent those of their affiliated organizations, or those of the publisher, the editors and the reviewers. Any product that may be evaluated in this article, or claim that may be made by its manufacturer, is not guaranteed or endorsed by the publisher.
The Supplementary Material for this article can be found online at: https://www.frontiersin.org/articles/10.3389/fenrg.2022.981097/full#supplementary-material
Cai, Y., Liu, Q., Chen, J., Tao, W. C., Shan, T. Y., and Hu, C. (2022). Research on carbon emission measurement and influencing factors construction industry in hubei province. J. Green Sci. Tcchnology 24 (06), 94–98. doi:10.16663/j.cnki.lskj.2022.06.018 |
Chai, Z. Y., Yan, Y. B., Simayi, Z., Yang, S. T., Abulimiti, M., and Wang, Y. Q. (2022). Carbon emissions index decomposition and carbon emissions prediction in Xinjiang from the perspective of population-related factors, based on the combination of STIRPAT model and neural network. Environ. Sci. Pollut. Res. 29, 31781–31796. doi:10.1007/s11356-021-17976-4 |
Chang, Y., Li, X. D., Masanet, E., Zhang, L. X., Huang, Z. Y., and Ries, R. (2018). Unlocking the green opportunity for prefabricated buildings and construction in China. Resour. Conserv. Recycl. 139, 259–261. doi:10.1016/j.resconrec.2018.08.025 |
Chi, Y. Y., Liu, Z. R., Wang, X., Zhang, Y. Y., and Wei, F. (2021). Provincial CO2 emission measurement and analysis of the construction industry under China's carbon neutrality target. Sustainability 13 (4), 1876–1915. doi:10.3390/su13041876 |
China Association of Building Energy Efficiency (2021). China building energy consumption annual report 2020. J. BEE 49, 2096–9422.
Ding, J., Chen, D. J., and Zhao, F. F. (2014). Carbon emissions prediction for construction industry in shandong province. J. Qingdao Univ. 27 (04), 65–68. doi:10.3969/j.issn.1006-1037.27.2014.11.14 |
Feng, B., Wang, X., and Liu, B. (2014). Provincial variation in energy efficiency across China's construction industry with carbon emission considered (in Chinese). Resour. Sci. 36 (06), 1256–1266.
Gao, S. H., Liu, Y. S., Li, X. T., and Yuan, J. B. (2019). Influencing factors and prediction of carbon emission in China's construction industry. Henan Sci. 37 (08), 1344–1350. doi:10.3969/j.issn.1004-3918.2019.08.024 |
IPCC (2020). Global warming of 1.5°C. Available online: https://www.ipcc.ch/sr15/.
Jia, J. S., Chen, Q., and Hu, L. L. (2014). Analysis on the carbon emission (CE) arising from the direct energy consumption (EC) of beijing's construction industry. Appl. Mech. Mater. 522-524, 1822–1825. doi:10.4028/www.scientific.net/amm.522-524.1822 |
Jiang, B. Y., Huang, B. L., and Zhang, H. (2021). Study on influencing factors of construction industry carbon emissions in Jiangsu Province based on LMDI model. Environ. Sci. Technol. 44 (10), 202–212. doi:10.19672/j.cnki.1003-6504.0425.21.338 |
Jiang, L., Sakhare, S. R., and Kaur, M. (2022). Impact of industrial 4.0 on environment along with correlation between economic growth and carbon emissions. Int. J. Syst. Assur. Eng. Manag. 13, 415–423. doi:10.1007/s13198-021-01456-6 |
Jiang, T. Y., Li, S. Q., Yu, Y., and Peng, Y. F. (2022). Energy-related carbon emissions and structural emissions reduction of China's construction industry: The perspective of input-output analysis. Environ. Sci. Pollut. Res. 29, 39515–39527. doi:10.1007/s11356-021-17604-1 |
Lai, X. D., Lu, C., and Liu, J. X. (2019). A synthesized factor analysis on energy consumption, economy growth, and carbon emission of construction industry in China. Environ. Sci. Pollut. Res. 26 (14), 13896–13905. doi:10.1007/s11356-019-04335-7 | |
Li, B., Han, S. W., Wang, Y. F., Li, J. Y., and Wang, Y. (2020). Feasibility assessment of the carbon emissions peak in China's construction industry: Factor decomposition and peak forecast. Sci. Total Environ. 706, 135716. doi:10.1016/j.scitotenv.2019.135716 | |
Li, D. Z., Huang, G. Y., Zhang, G. M., and Wang, J. B. (2020). Driving factors of total carbon emissions from the construction industry in Jiangsu Province, China. J. Clean. Prod. 276, 123179. doi:10.1016/j.jclepro.2020.123179 |
Liu, N. X., Wang, J., and Li, R. (2009). Computational method of CO_2 emissions in Chinese urban residential communities. J. Tsinghua Univ. 49 (09), 1433–1436. doi:10.16511/j.cnki.qhdxxb.2009.09.001 |
Liu, S. F., Cai, H., Yang, Y. J., and Cao, Y. (2013). Advance in grey incidence analysis modelling. Syst. Engineering-Theory Pract. 33 (08), 2041–2046. doi:10.3969/j.issn.1000-6788.2013.08.018 |
Qi, Z., Hao, S., and Xu, R. (2017). The influencing factors of carbon emissions in Chinese construction industry based on factor Analysis and improved STIRPAT model. Int. Conf. Constr. Real Estate Manag. 11, 148–155. doi:10.1061/9780784481066.015 |
Shaheen, A., Sheng, J. Y., Arshad, S., Defterli, O., Xie, X. Q., and Baleanu, D. (2020). A novel fractional grey model applied to the environmental assessment in Turkey. Int. J. Model. Simul. Sci. Comput. 11 (4), 2050039. doi:10.1142/S1793962320500397 |
Song, J. Z., Chen, C., Wang, X. P., and H, Z. (2019). Research on construction industry carbon emission efficiency based on three-stage super efficient DEA model. Environ. Sci. Technol. 42 (01), 28. doi:10.19672/j.cnki.1003-6504.2019.01.028 |
Wang, G. W. J., Li, S. S., and Yang, L. (2022). Research on the pathway of green financial system to implement the realization of China's carbon neutrality target. Int. J. Environ. Res. Public Health 19, 2451. doi:10.3390/ijerph19042451 | |
Wang, Y. K., Liang, Y., and Shao, L. S. (2022). Driving factors and peak forecasting of carbon emissions from public buildings based on LMDI-SD. Discrete Dyn. Nat. Soc. 2022, 1–10. doi:10.1155/2022/4958660 |
Yang, Y. F., Li, H. F., and Zheng, H. X. (2016). Research on factors of beijing's building carbon emissions. Ecol. Econ. 32 (01), 72–75. doi:10.3969/j.issn.1671-4407.2016.01.015 |
Yi, Y., and Liu, S. F. (2011). Grey systems: Theory and application. Grey Syst. Theory & Appl. 4883, 44–45. doi:10.1007/978-3-642-16158-2 |
Zhang, S. X., and Zhang, D. (2014). Study on the influence factors of energy consumption based on the STIRPAT model in shaanxi province. Constr. Econ. 2014 (03), 89–93. doi:10.14181/j.cnki.1002-851x.2014.03.009 |
Zhang, Z. H., and Liu, R. J. (2013). Carbon emissions in the construction sector based on input-output analyses. J. Tsinghua Univ. 53 (01), 53–57. doi:10.16511/j.cnki.qhdxxb.2013.01.007 |
Zhang, Z. X., Jia, J. S., Guo, Y. L., Wu, B., and Chen, C. D. (2022). Scenario of carbon dioxide (CO2) emission peaking and reduction path implication in five northwestern provinces of China by the low emissions analysis platform (LEAP) model. Front. Energy Res. 10, 983751. doi:10.3389/fenrg.2022.983751 |
Zhou, J. G., Jin, B. L., Du, S. J., and Zhang, P. (2018). Scenario analysis of carbon emissions of beijing-tianjin-hebei. Energies 11, 1489. doi:10.3390/en11061489 |
Zhou, J., Xu, X., Li, W., Guang, F., and Jin, B. L. (2019a). Forecasting CO 2 emissions in China's construction industry based on the weighted adaboost-ENN model and scenario analysis. J. Energy 2019 (11), 1–12. doi:10.1155/2019/8275491 |
Zhou, Y., Liu, W., Lv, X., Chen, X., and Shen, M. (2019b). Investigating interior driving factors and cross-industrial linkages of carbon emission efficiency in China's construction industry: Based on Super-SBM DEA and GVAR model. J. Clean. Prod. 241, 118322. doi:10.1016/j.jclepro.2019.118322 |
Keywords: carbon peak, construction industry, prediction of CO2 emission, grey correlation analysis, EGM(1,1)model, BP neural network model
Citation: Dai D, Li K, Zhao S and Zhou B (2022) Research on prediction and realization path of carbon peak of construction industry based on EGM-BP model. Front. Energy Res. 10:981097. doi: 10.3389/fenrg.2022.981097
Received: 30 June 2022; Accepted: 24 August 2022;
Published: 15 September 2022.
Edited by:
Yaolin Lin, University of Shanghai for Science and Technology, ChinaReviewed by:
Yun Guo, Shanghai University of Engineering Sciences, ChinaCopyright © 2022 Dai, Li, Zhao and Zhou. This is an open-access article distributed under the terms of the Creative Commons Attribution License (CC BY). The use, distribution or reproduction in other forums is permitted, provided the original author(s) and the copyright owner(s) are credited and that the original publication in this journal is cited, in accordance with accepted academic practice. No use, distribution or reproduction is permitted which does not comply with these terms.
*Correspondence: Shuhang Zhao, WlNIYW5nMTAwMUAxNjMuY29t
Disclaimer: All claims expressed in this article are solely those of the authors and do not necessarily represent those of their affiliated organizations, or those of the publisher, the editors and the reviewers. Any product that may be evaluated in this article or claim that may be made by its manufacturer is not guaranteed or endorsed by the publisher.
Research integrity at Frontiers
Learn more about the work of our research integrity team to safeguard the quality of each article we publish.