- 1New Energy (Photovoltaic) Industry Research Center, Qinghai University, Xining, China
- 2Qinghai Key Laboratory of Efficient Utilization of Clean Energy, Qinghai University, Xining, China
- 3Department of Electrical Engineering, Tsinghua University, Beijing, China
To improve the utilization of flexible resources in microgrids and meet the energy storage requirements of the microgrids in different scenarios, a centralized shared energy storage capacity optimization configuration model for microgrids based on bi-level optimization is proposed. First, the response characteristics of the shared energy storage and controllable load in the resilience microgrid are analyzed, and the centralized shared energy storage operation mode meeting the regulatory demand of multi-scenarios is designed. Then, a bi-level optimal allocation model is constructed, which takes the maximum net income of centralized shared energy storage as the upper layer and the minimum payment cost of load in the microgrid as the lower layer. Furthermore, the multi-objective whale optimization algorithm is used to solve the bi-level optimization model. The results show that the shared energy storage can jointly meet the regulation demand of multi-scenarios by coordinating the transferable load and cuttable load in the microgrid and improving the utilization rate of shared energy storage.
1 Introduction
The new energy industry has ushered in rapid development, resulting in the permeability of new energy in the microgrid continuing to improve, with the implementation of the strategic goal of carbon peak and carbon neutrality in China (Chen et al., 2017). Therefore, the reliability and security of the power system have attracted much attention. For example, the microgrid has an impact on the grid when it is connected to the grid due to the fluctuation of load and source. The randomness of new energy power generation in the microgrid will cause a power mismatch. Traditional energy storage has been unable to meet the adjustment above the demand of microgrids. Therefore, new modes and methods to improve the economy and flexibility of energy storage to meet the needs of multi-scenario regulation in a microgrid are urgently proposed. Therefore, it is of great significance to study the capacity allocation of centralized shared energy storage in the microgrid.
At present, a great deal of research has been done on the planning and configuration of energy storage in microgrids at home and abroad. Li et al., 2022 established an energy storage model based on the presence of load shortages in the microgrid and other indicators by considering the volatility of energy storage output. Li et al., 2020a proposed a hybrid energy storage system with hydrogen storage and batteries by tracking the output in the microgrid, which improved the economy and energy utilization of the microgrid. Wu et al., 2021 considered the interest decisions between energy storage operators and multi-microgrids and established a two-layer planning model with energy storage capacity configuration and multi-microgrid system optimized operation to configure energy storage. Fei et al., 2019 proposed a planning model for a hybrid energy storage system considering the economy of the microgrid during operation. In addition, some references study the flexible resources on the load side by considering the controllable load in the resilience microgrid to improve the resilience of the microgrid. Zhao et al., 2017 effectively improved the reliability of power supply and demand in the resilience microgrid by analyzing various types of loads in the microgrid. Hafiz et al., 2019 unified the load side response and distributed power generation to further improve the resilience of the microgrid. Amirioun et al., 2018 improved the resilience of microgrids by aggregating controllable loads and constructing them as continuous variables with upper and lower bounds. Gong et al., 2020 proposed that the load side elastic load resource in the power system is an effective resource to track the new energy output plan and adjust the power fluctuation balance.
However, the energy storage in the aforementioned studies has the disadvantage of low utilization and high cost. Shared energy storage is widely used in the energy storage planning of the microgrid. Xuanyue et al., 2022 configured shared energy storage in user groups and microgrids to meet users’ power access and access to auxiliary services. Li et al., 2021a considered the energy complementarity between multiple microgrids and multi-microgrids jointly built shared energy storage to meet their goals and needs. Wang et al., 2018 reduced its own energy cost by storing excess electric energy in new energy power stations in shared energy storage and using it during peak electricity consumption periods. Sun et al., 2020 configured energy storage by integrating different scales of electricity, heat, and natural gas storage resources to build a flexible multi-energy complementary sharing platform. Li et al., 2021b proposed a bi-level operation system for new energy and shared energy storage based on the goals of minimum total operating cost and maximum power comfort for virtual residential microgrids.
The aforementioned research is limited to the planning and optimization of traditional energy storage resources, and the regulation potential of controllable load in the resilience microgrid is rarely considered. There is less research on shared energy storage, transferable load, and switchable load in the microgrid to participate in the regulation demand of new energy power systems. Furthermore, conventional energy storage planning mostly serves a single subject or a single scene, which makes it difficult to meet the multi-scenario regulation demand of the new energy power systems in the future power grid development form under the sharing mode.
Based on the aforementioned analysis, this article takes the power regulation demand and frequency regulation demand in the microgrid as the scenario. The response characteristics of various controllable loads and shared energy storage in the resilience microgrid are analyzed. The multi-scenario and multi-regulation demand by sharing energy storage and coordinating the controllable loads in the microgrid are met. A bi-level optimization model including shared energy storage and the microgrid is established, which considers the different interest needs and objectives of participants. Finally, taking a regional microgrid as an example, the results of shared energy storage in the microgrid under multi-scenario regulation demand are discussed. The outputs of controllable load and shared energy storage are analyzed through response characteristics. The study provides a decision reference for the development of centralized shared energy storage in the microgrid.
2 Microgrid with shared energy storage and controllable load
2.1 The operation mode of shared energy storage
The proposed centralized shared energy storage operation mode is described as follows: the power supply, energy storage, and load are combined to build a system architecture including a microgrid, shared energy storage, and power grid (Kang et al., 2017). On one hand, the centralized shared energy storage combines with the controllable load in the resilience microgrid to jointly coordinate the output plan on the power side of the microgrid, solve the power mismatch problem in the microgrid, ensure the balance of power supply and demand, and meet the regulation demand on the power side. On the other hand, the centralized shared energy storage coordinates its charge and discharge power to stabilize the net load fluctuation on the grid side, solve the frequency fluctuation caused by the impact of the microgrid on the grid, ensure the stability of power supply and demand, and meet the regulation demand on the grid side. Therefore, this study designs a centralized shared energy storage operation mode to meet the regulation demand of the multi-scenario in the microgrid. The operation mode of centralized shared energy storage in the microgrid constructed in this study is shown in Figure 1.
2.1.1 Coordination of power fluctuation of the power supply side in the microgrid
where
2.1.2 Stabilization of the frequency fluctuation of the microgrid to the grid side
where
2.1.3 Shared energy storage
The shared energy storage meets the regulation demand of a microgrid by charging and discharging power. Therefore, by compensating the charge and discharge power of shared energy storage (Ma et al., 2019), it can mobilize its enthusiasm to participate in the multi-scenario regulation demand in the microgrid
where
2.2 The response characteristics of controllable load
In this study, the new energy power station (unregulated), traditional load (unregulated), transferable load and cuttable load (controllable), and shared energy storage (controllable) in the microgrid in the same region are aggregated into a whole (Li et al., 2020). According to the behavior characteristics and actual situation, the controllable load of resilience microgrids is described as follows:
2.2.1 Transferable load
This kind of load is flexible and can be removed or interrupted according to the user’s comfort and response. The compensation price coefficient of the cuttable load is high, and the power of the cut load is always positive.
The load shedding power is equivalent to the charging power of shared energy storage in this period
where
2.2.2 Cuttable load
This kind of load can be reasonably allocated in different periods according to the actual situation. At the same time, considering the load incentive cost provided for the transferred load, the transfer incentive price coefficient is low, and the total load before and after the transfer is unchanged.
When the power difference between the transferable loads after transfer and before the transfer is positive, it is equivalent to the discharge power of shared energy storage; when the power difference between the transferable loads after transfer and before the transfer is negative, it is equivalent to the charging power of shared energy storage
where
3 Microgrid with shared energy storage and controllable load
3.1 Elements of the bi-level optimization model
1) Collection of players. The players in the optimal allocation model of shared energy storage capacity include shared energy storage and multiple microgrids, and the player’s set is expressed as
2) Players’ strategy. In the optimal allocation model of shared energy storage capacity, the strategy of player 1 sharing energy storage is its capacity
3) Players’ payment. In this study, the capacity allocation problem of centralized shared energy storage in a microgrid is studied. The payment includes the net income of shared energy storage and the payment cost of the microgrid. The players’ payment’ is expressed as follows:
3.2 Payments for capacity allocation models
In this study, there is interaction and influence of energy between shared energy storage and the microgrid. First, the capacity construction of shared energy storage affects the payment cost of the microgrid. Second, the controllable load in the resilience microgrid determines the power and construction capacity of shared energy storage participating in different regulation demands (Zhang et al., 2022). Therefore, a bi-level optimization model is used to optimize the allocation of shared energy storage capacity.
This study takes the shared energy storage as the upper layer, and its objective function is the maximum net income
where
This study takes the microgrid as the lower layer, and its objective function is the minimum payment cost
where
The payment of the aforementioned players should also meet the following constraints:
1) The constraints of shared energy storage capacity. The real-time capacity
where
To ensure the long-term stable operation of shared energy storage and prolong the service life of shared energy storage, the initial and final capacity of shared energy storage should remain unchanged in each cycle, and the real-time capacity of shared energy storage should be within the safe range of shared energy storage capacity. The specific expressions are as follows:
In this study, it is assumed that the relationship between the rated capacity of centralized shared energy storage and the maximum charge-discharge power is linear (Xuanyue et al., 2021), which is expressed as follows:
where
2) The constraint of sharing the charging and discharging power of the energy storage is shown in Equation 3.
3) The constraint of the controllable load power in the microgrid is shown in Equations 4, 5.
3.3 Capacity configuration model solution process
In this study, a bi-level optimization model of centralized shared energy storage capacity allocation in the resilience microgrid is established (Ma et al., 2021). Shared energy storage needs to coordinate the controllable loads in the microgrid to meet the regulatory demand of power fluctuations on the power supply side and the frequency on the grid side. The solution flow chart of the shared energy storage capacity configuration model is shown in Figure 2, and the specific expressions are as follows:
Phase 1: The initial capacity value of N groups of shared energy storage is randomly generated and transmitted to the lower microgrid. The microgrid optimizes its controllable load according to the capacity of the shared energy storage and returns it to the shared energy storage. The returned controllable load power is used to calculate the net income corresponding to the N groups of capacity values under the current situation, and the maximum net income
Phase 2: MOWOA is used to update the capacity value of N groups of shared energy storage (Li et al., 2021), and the steps of stage 1 to solve the net income of N groups of shared energy storage in the new case are repeated, and the net income
Phase 3: The net income of shared energy storage
4 Case study
4.1 Parameter setting
This study selects two adjacent microgrids in an area for example analysis, in which the installed capacities of the wind farm are 70 and 120 Kw. Figure 3A shows the predicted power and actual power of the wind farm of the two microgrids under typical days, and Figure 3B shows the predicted load and actual load.
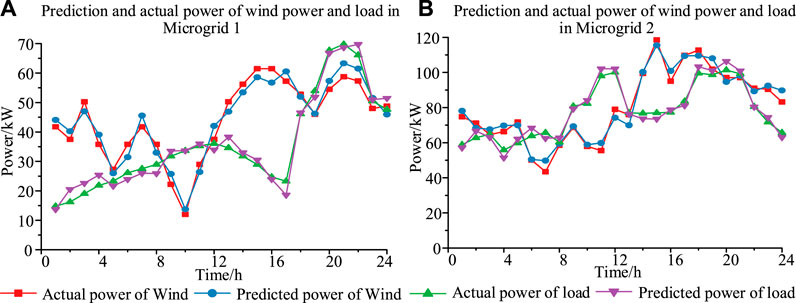
FIGURE 3. Actual power and predicted power of wind field and load in MG. Sizing of shared energy storage in MG.
In this study, the relevant parameters of the calculation example are set according to the change of load in the microgrid and the actual situation. The time of allowable change of controllable load in the microgrid and the setting of other relevant parameters are the main parameters with reference to Lin et al., 2019. It is assumed that the allowable reduction period of controllable load in the microgrid is 10:00–14:00 and 19:00–22:00, and the allowable increase period of the load is 0:00–6:00; the detailed setting of the cuttable load and transferable load is shown in Table 1. In addition, the relevant parameters of centralized shared energy storage are mainly referred to Xuanyue et al., 2021, and the detailed settings are shown in Table 2. The setting of the time-of-use price of the load is mainly referred to Li et al., 2021c, and the detailed setting is shown in Table 3.
4.2 Sizing of shared energy storage in MG
This section uses the parameters and data given in Section 3.1 to solve the optimal configuration model. The configuration results of shared energy storage are shown in Table 4.
As can be seen from Table 4, the revenue of centralized shared energy storage under the regulation demand scenario on the power side of the microgrid is 121,160 $, and the revenue under the regulation demand scenario on the power grid side is 2,147 $. In this case, the capacity of shared energy storage is 46 kW, and the net revenue of shared energy storage is 112,567 $. Multi-scenario regulation demand in the microgrid is the main source of revenue for shared energy storage.
The payment cost of the microgrid is 351,531 $, of which the cost of the traditional load is 390,684 $, and the benefits of the transferable load and the cuttable load in controllable compliance are 21,356 $ are 17,797 $, respectively. The resilience microgrid can benefit itself by coordinating controllable load to jointly coordinate the power fluctuation on the power side and reduce the payment cost of the microgrid without affecting the comfort of use.
In addition, to verify the rationality of centralized shared energy storage configuration results and the effectiveness of the combination of shared energy storage and controllable loads in the microgrid, assumption Case 1 is the model constructed in this study; Case 2 is that controllable loads in the resilience microgrid do not participate in multi-scenario regulation demand. The results of centralized shared energy storage capacity optimization under different conditions are shown in Figure 4.
Figure 4 shows the configuration results of centralized shared energy storage under different conditions. When the controllable loads in the microgrid do not participate in the regulation demand of the multi-scenario (case 2), the centralized shared energy storage, as the only flexible resource, participates in the regulation of the microgrid. Compared with case 1, its capacity increases by 3 KW, the payment cost of the microgrid increases by 134,105$, and the net income of shared energy storage decreases by 7,038 $. This is because the capacity of centralized shared energy storage increases, increasing its investment cost, and the capacity utilization rate of shared energy storage is low, resulting in a decline in the net income of shared energy storage. In conclusion, the capacity allocation model proposed in this study can scientifically balance the benefits of centralized shared energy storage and the microgrid.
4.3 The result analysis of controllable load in MG
The change of controllable load in the resilience microgrid is shown in Figure 5. Comparing Figure 5A and Figure 5C, it can be seen that before the controllable load does not participate in the wind power regulation demand of the resilience microgrid, the load of microgrid 1 has two peak periods from 9:00 to 13:00 and from 18:00 to 22:00, and microgrid 2 has two peak periods from 11:00 to 12:00 and from 18:00 to 22:00.
Combined with the allowable change period of controllable load in Section 3.1, compared with Figures 5A,B, the transferable load in microgrid 1 is transferred from 10:00–13:00 and 19:00–22:00 to 1:00–6:00, the total transferred power is 79.43 kW, and the power before and after the transfer has not changed. The cuttable load is also reduced in this period, and the total reduced power is 39.72 kW. Comparing Figures 5C,D, the transferable load of microgrid 2 is transferred from 11:00–12:00 and 19:00–22:00 to 1:00–6:00. The total transferred power is 115.59kW, and there is no change before and after the transfer. The cuttable load is reduced in this period, and the total reduced power is 57.80 kW. In addition, the peak point of the microgrid at 18:00 does not change before and after participating in the multi-scenario regulation demand. This is because 18:00 does not belong to the changeable period of controllable load in the resilience microgrid, so it does not participate in the multi-scenario regulation demand in the microgrid. According to the aforementioned analysis, it can be seen that the change of controllable load in the microgrid is sufficient to meet its response characteristics, and the regulation capacity of the transferable load is greater than that of the cuttable load.
It should be noted that after the controllable load participates in the power side regulation demand of the microgrid together with the shared energy storage, the changing trend of the two microgrid loads tends to be gentle. It should be noted that the peak load of microgrid 2 decreased by 18% and that of microgrid 1 decreased by 29%. The initiative of controllable load in the microgrid has been improved, which has played a role in cutting peaks and filling valleys for power supply in the microgrid.
4.4 The result analysis of controllable load in MG
In this section, according to the output characteristics of centralized shared energy storage and combined with different regulatory demands in the microgrid, the operation of centralized shared energy storage in the microgrid is shown in Figure 6.
Figure 6A shows the change of charge and discharge power of shared energy storage on typical days, where the shared energy storage power greater than 0 indicates charging and that less than 0 indicates discharging. Combined with Figure 3, it can be seen that the charging and discharging powers of shared energy storage are consistent with the fluctuation trend of frequency and power in the microgrid. It should be pointed out that in combination with the response characteristic analysis in Section 1.2 and Figure 5, the shared energy storage is charged during the period of 1:00–6:00. This is because the transferable load in the microgrid makes the load in this period greater than the load before transfer. The shared energy storage performs the charging operation. The shared energy storage is discharged from 9:00 to 12:00 because the controllable load after the change in the resilience microgrid is less than the controllable load before the change, and the shared energy storage performs the discharge operation.
It can be seen from Figure 6B that the utilization rate of centralized shared energy storage in the microgrid has been improved under the regulation demand of multiple scenarios. On a typical day, the cumulative output of shared energy storage in the coordinated power fluctuation of the wind station in the microgrid is 629.17 kW, and the cumulative output of shared energy storage in stabilizing microgrid frequency fluctuation on the grid side is 117.62 kW. To sum up, shared energy storage can meet the multi-scenario regulation demand in the microgrid.
5 Conclusion
To the urgent demand for energy storage flexibility and economy of microgrids under the demand of multi-scenario regulation, a bi-level optimization allocation model of centralized shared energy storage in resilience microgrids is proposed. The main contributions are as follows:
1) A shared energy storage operation mode is proposed for the resilience microgrid with controllable loads. In the mode, the response characteristics of a variety of controllable loads are comprehensively considered and the regulatory demand of different scenarios by coordinating the controllable loads and shared energy storage in the resilience microgrid are met. The cumulative transferable load of the microgrid is 195.02 kW, the cumulative cuttable load is 97.51 kW, and the cumulative output of shared energy storage is 746.78 kW, which improves the utilization rate of shared energy storage and flexible resources in the microgrid.
2) A bi-level optimization allocation model of shared energy storage is established. In the model, the payment cost of the microgrid is effectively reduced, and the net income of shared energy storage is improved. Under this model, before and after the controllable load in the microgrid participates in the multi-scenario regulation demand, the capacity of centralized shared energy storage is reduced by 3 KW, and the net income is increased by 7,038$. The result shows that the benefits of shared energy storage and microgrids can be balanced by the bi-level optimization model, and the validity of the combination of shared energy storage and controllable load and the rationality of capacity allocation results are verified.
The follow-up studies will focus on the optimal allocation of shared energy storage on the load side like the industrial park with the energy interaction between different loads.
Data availability statement
The original contributions presented in the study are included in the article/Supplementary Material; further inquiries can be directed to the corresponding author.
Author contributions
XD, XL, YH, and LC contributed to conception and design of the study. YH summarized existing studies. XL performed the statistical analysis. LC adjusted the overall structure of the paper. XD wrote the first draft of the manuscript. All authors contributed to manuscript revision, read, and approved the submitted version.
Funding
This work was supported by the National Natural Science Foundation of China (52077109).
Conflict of interest
The authors declare that the research was conducted in the absence of any commercial or financial relationships that could be construed as a potential conflict of interest.
Publisher’s note
All claims expressed in this article are solely those of the authors and do not necessarily represent those of their affiliated organizations or those of the publisher, the editors, and the reviewers. Any product that may be evaluated in this article or claim that may be made by its manufacturer is not guaranteed or endorsed by the publisher.
References
Amirioun, M. H., Aminifar, F., and Lesani, H. (2018). Towards proactive scheduling of microgrids against extreme floods. IEEE Trans. Smart Grid 9 (4), 3900–3902. doi:10.1109/tsg.2017.2762906
Chen, L., Mu, L., and Xu, X., (2017). Influences of energy storage operational strategy and characteristic on microgrid reliability. Electr. Power Autom. Equip. 37 (7), 70–76. doi:10.16081/j.issn.1006-6047.2017.07.011
Fei, L., Li, C., and Sun, K., (2019). Integrated planning and operation method of hybrid energy storage system in microgrid. Control Theory & Appl. 36 (3), 453–460. doi:10.7641/CTA.2019.80523
Gong, X. M., M, Y., and Du, P. L. (2020). Renewable energy accommodation potential evaluation of distribution network: A hybrid decision making framework under interval type- 2 fuzzy environment. J. Clean. Prod. 286 (8), 124918. doi:10.1016/j.jclepro.2020.124918
Hafiz, F., Chen, B., Chen, C., Rodrigo de Queiroz, A., and Husain, I. (2019). Utilising demand response for distribution service restoration to achieve grid resiliency against natural disasters. IET Gener. Transm. Distrib. 13 (14), 2942–2950. doi:10.1049/iet-gtd.2018.6866
Kang, C., Liu, J., and Zhang, N.(2017). A new form of energy storage in future power system: Cloud energy storage. Automation Electr. Power Syst. 41 (21), 2–8. doi:10.7500/AEPS20170601011
Li, H., Lv, M., and Hu, L., (2020). Joint planning of microgrid considering generalized energy storage. Electr. Power Autom. Equip. 40 (07), 149–160. doi:10.16081/j.epae.202007006
Li, J., Tan, Y., and Wang, H., (2022). Configuration optimization strategy of distribution network and optical storage microgrid energy storage system. High. Volt. Technol. 48 (05), 1893–1902. doi:10.13336/j.1003-6520.hve.20201333
Li, S., Zhu, J., Chen, Z., and Luo, T. (2021). Double-layer energy management system based on energy sharing cloud for virtual residential microgrid. Appl. Energy 282, 116089. doi:10.1016/j.apenergy.2020.116089
Li, X., Xie, S., and Fang, Z., (2021). Optimal configuration and cost allocation of energy storage shared by multiple microgrids. Electr. Power Autom. Equip. 41 (10), 44–51.
Li, X., Wang, W., and Wang, H. (2021). A novel bi-level robust game model to optimize a regionally integrated energy system with large-scale centralized renewable-energy sources in Western China. Energy 228, 120513. doi:10.1016/j.energy.2021.120513
Li, X., Wang, W., and Wang, H.(2021). Hybrid time-scale energy optimal scheduling strategy for integrated energy system with bilateral interaction with supply and demand. Appl. Energy 285, 285116458. doi:10.1016/j.apenergy.2021.116458
Li, Y., Guo, X., and Dong, H. (2020). Optimal capacity configuration of wind/PV/storage hybrid energy storage system in microgrid. Proc. CSU-EPSA 32 (6), 123–128. doi:10.19635/j.cnki.csu-epsa.000322
Lin, C., Qi, N., and Tian, L. (2019). Joint planning of generalized energy storage resource and distributed generator considersing opreation control strategy. Automation Electr. Power Syst. 43 (10), 27–35+43. doi:10.7500/AEPS20180814004
Ma, H., Liu, Z., Li, M., Wang, B., Yang, S., and Yang, Y. (2021). A two-stage optimal scheduling method for active distribution networks considering uncertainty risk. Energy Rep. 7, 4633–4641. doi:10.1016/j.egyr.2021.07.023
Ma, H., Wang, B., Gao, W., Zhou, Z., Yang, S., and Yang, Y. (2019). Operation optimization of energy storage equipment participating in auxiliary service in regional integrated energy system[J]. Power Syst. autom. 43 (08), 34–40+68. doi:10.7500/AEPS20180813007
Sun, L., Qiu, J., Han, X., Yin, X., and Dong, Z. Y. (2020). Capacity and energy sharing platform with hybrid energy storage system: An example of hospitality industry. Appl. Energy 280 (4), 115897. doi:10.1016/j.apenergy.2020.115897
Wang, S., Chang, P., and Xue, G. (2018). Research on collaborative optimization of community integrated energy system considering shared energy storage. China Power 51 (08), 77–84. doi:10.11930/j.issn.1004-9649.201805160
Wu, S., Li, Q., and Liu, J., (2021). Double-layer optimal configuration of cooling, heating and power multi-microgrid system based on energy storage power station services. Grid Technol. 45 (10), 3822–3832. doi:10.13335/j.1000-3673.pst.2020.1838
Xuanyue, S., Ma, Z., and Wang, X., (2022). Research on optimal operation of shared energy storage and integrated energy microgrid based on master-slave game theory. Grid Technol., 1–12. doi:10.13335/j.1000-3673.pst.2021.2191
Xuanyue, S., Wang, X., and Huang, J. (2021). Optimal configuration of shared energy storage capacity under multiple regional integrated energy systems interconnection. J. Glob. Energy Interconnect. 4 (04), 382–392. doi:10.19705/j.cnki.issn2096-5125.2021.04.007
Zhang, W., Zheng, B., Wei, W., Chen, L., and Mei, S. (2022). Peer-to-peer transactive mechanism for residential shared energy storage. Energy 246, 123204. doi:10.1016/j.energy.2022.123204
Keywords: centralized shared energy storage, controllable load, bi-level optimization, the resilience microgrid, multi-scenarios
Citation: Du X, Li X, Hao Y and Chen L (2022) Sizing of centralized shared energy storage for resilience microgrids with controllable load: A bi-level optimization approach. Front. Energy Res. 10:954833. doi: 10.3389/fenrg.2022.954833
Received: 27 May 2022; Accepted: 27 June 2022;
Published: 05 August 2022.
Edited by:
Tianyang Zhao, Jinan University, ChinaReviewed by:
Bo Wang, Wuhan University, ChinaYin Yao, Shanghai University of Electric Power, China
Wei Gao, University of Denver, United States
Copyright © 2022 Du, Li, Hao and Chen. This is an open-access article distributed under the terms of the Creative Commons Attribution License (CC BY). The use, distribution or reproduction in other forums is permitted, provided the original author(s) and the copyright owner(s) are credited and that the original publication in this journal is cited, in accordance with accepted academic practice. No use, distribution or reproduction is permitted which does not comply with these terms.
*Correspondence: Laijun Chen, Y2hlbmxhaWp1bkBxaHUuZWR1LmNu