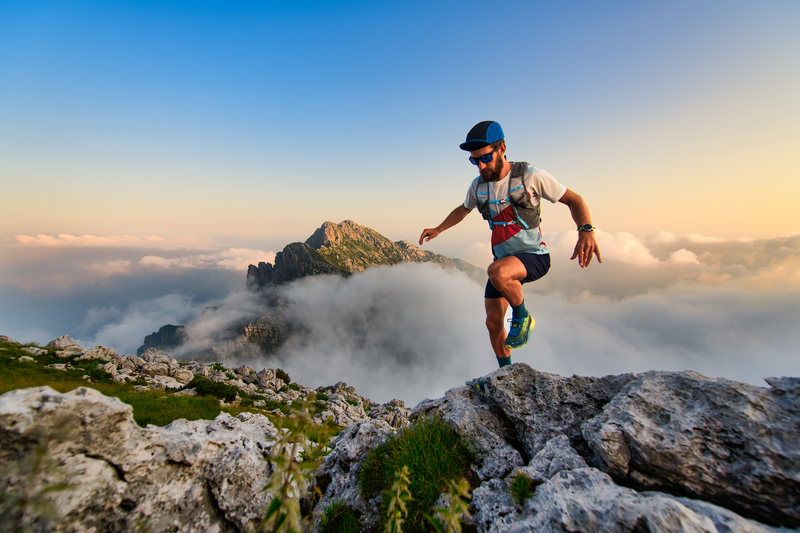
95% of researchers rate our articles as excellent or good
Learn more about the work of our research integrity team to safeguard the quality of each article we publish.
Find out more
ORIGINAL RESEARCH article
Front. Energy Res. , 22 July 2022
Sec. Smart Grids
Volume 10 - 2022 | https://doi.org/10.3389/fenrg.2022.952396
This article is part of the Research Topic AI-Driven Zero Carbon Cyber-Energy System View all 11 articles
In order to reduce port pollution and carbon emissions and improve the utilization rate of clean energy, a port microgrid based on we-energies (WEs) and its polymorphic distributed low-carbon energy management method is proposed. First, this study considers a variety of heterogeneous Wes, such as ship we-energies (SWEs), to establish a polymorphic energy management system for port microgrids and to achieve reliable information exchange between WEs under different communication networks. Second, considering the bidirectional energy transmission characteristic of the port WEs, the operating cost function of heterogeneous WEs is established. In addition, with the objective of economic and low-carbon operation of port microgrids, the energy management model of a port microgrid is constructed, and the optimal solution is obtained based on distributed optimization theory. Finally, simulation cases are performed to verify the effectiveness of the proposed method.
With the development of the shipping industry, maritime transport is responsible for nearly 90% of global trade in goods. As important transportation hubs for sea and land transport, ports consume huge amounts of energy (Coppola et al., 2016; Huang et al., 2022). In addition, ships and large port equipment emit a lot of air pollutants, causing poor air quality in the port and the surrounding environment (Tang et al., 2018; Alamoush et al., 2020). Therefore, in order to solve the problem of port energy consumption and environmental pollution, it is urgent to build a green and low-carbon port microgrid with clean energy as the main energy (Nikolaos and Theocharis, 2021). In order to ensure the reliability of operation of the port microgrid, it is crucial to study its energy management issues (Çağatay and Jasmine, 2019).
The essence of port microgrid energy management is an optimization problem with constraints, which requires meeting the conditions of reliable operation for port microgrid and minimizing port operating costs by using suitable optimization strategies (Hein et al., 2021). Research results on port microgrids have mainly considered the uncertainty of renewable energy supply on the energy supply side (Çağatay and Jasmine, 2021) and the reliability of supplying flexible loads such as ships on the demand side (Parise et al., 2016; Fang et al., 2020). Considering the presence of a large number of flexible loads in the port power system, an energy management model with multiple decision variables and constraints has been proposed to vary the flexible loads’ power demand at high loads or high electricity prices in order to optimize the operating costs of the port power system (Kanellos et al., 2019). Studies on energy management in port microgrids are often solved by using centralized optimization methods (Olivares et al., 2014; Kermani et al., 2020). The centralized methods rely on the design of the centralized controller, which will need to be redesigned if the structure of the port microgrid changes. At the same time, although the centralized controller is capable of handling huge amounts of data in the microgrid, its failure would cause huge losses to the port. To sum up, the centralized methods have problems such as difficult network expansion and single point of failure. Moreover, port microgrids with large-scale clean energy present a distributed structure, so the distributed methods are attracting extensive attention of researchers (Li et al., 2020; Li et al., 2021). In the distributed methods, each agent needs to obtain information about its neighbors with the help of a communication network and performs local calculations based on the exchange of information between itself and its neighbors to achieve distributed energy management (Yang et al., 2019). For port power systems with flexible loads, such as ships, a distributed hierarchical control method has been proposed to solve the problem of reducing the operating costs of the port power system (Gennitsaris and Kanellos, 2019). A multiobjective operation scheduling method based on an innovative virtual fuzzy electricity price has been proposed to address the problem of carbon emissions for large ports in a short period of time (Kanellos, 2019). A distributed alternating direction method of multipliers algorithm has been proposed for the existence of energy entities with different energy forms in the port energy system, in which the energy bodies only share local information with their neighbors to complete the information update, solving the problem of optimal operating costs of the port energy system (Zhang et al., 2020). A distributed hierarchical topology reconfiguration approach has been proposed for port power systems with false data attacks, solving the problem of energy management in port power systems with an unknown and arbitrarily large number of attacked nodes (Shan et al., 2022).
The high level of clean energy connected to the port microgrid increases the flexibility of the energy supply and gives rise to a manufacturing and marketing integration of energy main body – WEs, which contains at least one type of energy production equipment or energy consumption equipment (Sun et al., 2017; Sun et al., 2019). According to the type of energy consumption of the power generating equipment, the port microgrid includes traditional energy generating equipment WEs (TWEs) and clean energy generating equipment WEs (CWEs). However, as more and more all-electric ships fueled by clean energy come into service (Wen et al., 2021; Zhang et al., 2021), they can either be connected directly to the shore power plant with generators switched off and powered by the port microgrid for the purpose of reducing pollution (Fang et al., 2020) or they can be used as power generators to supply electricity to the shore loads, giving them manufacturing and marketing integration of behavior. Therefore, all-electric ships fueled by clean energy can also be considered a dynamic type of WE in port, namely ship WEs (SWEs). The bidirectional energy transmission characteristic of WEs makes them both energy suppliers and energy consumers (Sun et al., 2019), to the extent that the operating costs of WEs are no longer just the cost of the power generating equipment. This not only increases the complexity of the WE operating cost function but also creates difficulties in modelling the energy management of the port microgrid. In addition, the distributed energy management methods for the considered port microgrid rely on the information interaction between WEs, which is based on the premise that all WEs need to be in the same communication network. However, the SWEs and various heterogeneous WEs ashore are always in different traditional communication networks. Due to the problems of closed network element structure and single communication mode in the traditional communication network, the network convergence has a low support capability, with the result that the information interaction between the WEs based on the traditional communication network cannot be realized, thus, the distributed energy management of the port microgrid cannot be realized. In recent years, the polymorphic network is a newly emerging type of smart network in different communication networks, which uses a dynamic combination of resources and network reconfiguration to enhance the functions, performance, and other needs of the network and fundamentally meet the service needs of network intelligence, diversity, personality, high tenacity, and high performance (Hu et al., 2019). Polymorphic network breaks the traditional network structure to achieve flexible interconnection of heterogeneous networks, and new networks with hybrid addressing based on polymorphic identification can achieve efficient interaction of different data in space (Hu et al., 2020). The implementation of distributed energy management for port microgrids based on WEs can be guaranteed under a polymorphic network.
In summary, this study is dedicated to solving the energy management problem of port microgrids containing WEs and proposes a distributed method under a polymorphic network, with the following main contributions:
1) A polymorphic energy management system was established for a port microgrid based on WEs. Considering various WEs in different communication networks, such as SWEs and CWEs in the port microgrid, in order to guarantee the information interaction between neighbor WEs, the port microgrid energy management system was established based on a polymorphic network to realize distributed energy management.
2) Considering the bidirectional energy transmission characteristic of WEs, the operating cost function for WEs was constructed. The manufacturing and marketing integration of the behavior of WEs meant that the operating cost for WEs not only included the cost of WE’s power generation but also the cost or benefit of trading with neighbor WEs or the main grid. We constructed operating cost functions for WEs with incremental cost as a variable, simplified the cost functions based on rotation symmetry, and analyzed the convexity of the operating cost functions.
3) Considering the cost of carbon emissions, a port microgrid energy management model was constructed, and a distributed solution method was proposed. With the objective of minimizing the operating cost of all WEs and the carbon emissions of the port, taking into account the constraints on the reliable operation of the port microgrid, the energy management model of port microgrid was constructed, and a distributed energy management strategy was proposed based on a multi-agents consensus algorithm.
The structure of the rest of the article is as follows. In Section 2, a polymorphic energy management system for WE-based port microgrid is established. In Section 3, the port microgrid energy management model is established, and a distributed solution method is proposed. In Section 4, the proposed method is validated by simulation using MATLAB. Finally, the results of the study are summarized.
The port microgrid considered in this study is composed of a large number of WEs, which can be classified according to the type of power generating equipment: TWEs and CWEs. In particular, CWEs include wind turbine WEs (WWEs) and photovoltaic generating equipment WEs (PWEs). Unlike traditional ships that use shore power after berthing, all-electric ships fueled by clean energy are also dynamic WEs for port microgrids – SWEs. The widespread presence of WEs gives the port microgrid a distributed characteristic and requires a distributed method based on a multi-agents system to solve the port microgrid energy management problem. Distributed energy management relies on the interaction of information between agents corresponding to each WEs, provided that all the agents are in the same communication network and that their communication topology meets certain constraints. However, the shore-based WEs and berthing SWEs are in different communication networks, making information interaction between neighbor agents impossible. Polymorphic network supports polymorphic identification, such as content identification and identity identification. Identification based on polymorphic addressing can realize flexible networking and provide a channel for information interaction between WEs in different communication networks. Therefore, a polymorphic energy management system for a port microgrid was constructed, and its structure is shown in Figure 1.
As can be seen in Figure 1, the port microgrid polymorphic energy management system consists of a data layer, a control layer, and a service layer from the bottom up. The function of the data layer is fitting the routing and resources of a variety of heterogeneous infrastructure platforms, providing basic data support and security for the construction of port microgrid network elements, receiving operational data, and broadcasting the scheduling instructions. The control layer is used for polymorphic addressing for heterogeneous WEs based on different identities and forming a dynamic communication topology network following certain constraints so as to enable peer-to-peer information interaction between neighbor WEs. The function of the service layer is to enable the energy management of the port microgrid. First, the generating cost functions of different WEs are obtained, and various WEs’ operating cost functions are established, considering the bidirectional energy transmission characteristic. Second, the energy management model of the port microgrid with the economic and low-carbon objective is constructed. Finally, based on the communication topology of the WEs given by the control layer, a distributed solution method is designed to realize the distributed energy management of the port microgrid.
In summary, the polymorphic energy management system of the port microgrid is based on a polymorphic network to achieve the compatibility of heterogeneous traditional communication networks, which enables the interaction of information between WEs in different modes and lays the foundation for the subsequent implementation of distributed energy management in the port microgrid.
Port microgrid energy management relies on the exchange of information between neighbor WEs, and its communication topology is represented as
The bidirectional energy transmission of WEs in port microgrids complicates their operating cost, which includes not only the cost of generating equipment but also the cost associated with energy interaction between WEs. As the energy consumed by the load in the WE can be supplied by the WE itself, neighbor WEs via the microgrid, or even the main grid. Therefore, the operating cost of individual WE in the port microgrid in this study includes the following: the generating electricity cost of the WEs, the cost of purchasing electricity from neighbors and the main grid, and the cost of selling electricity to neighbors and the main grid. Based on the above-stated analysis, the operating cost of individual WE in the port microgrid is expressed as follows:
where
Remark 1. The unit price of electricity trading between WEs is the incremental cost of each WE’s generation equipment.Assuming a port microgrid containing
where
According to Eq. 3, it can be seen that the cost of energy interaction between WEs in the port microgrid offsets each other. Therefore, the operating cost function of port microgrid based on WEs can be expressed as
Remark 2. Due to the manufacturing and marketing integration of WEs, the energy supply–demand balance of the WE is ensured by considering the electricity trading between WEs and the electricity trading between the WE and the main grid. Furthermore, the relationship between the incremental cost
For CWEs and TWEs, the cost function
As the process of analyzing the operating cost function of WEs requires modeling with the incremental cost
The character of the port microgrid operating cost function is closely related to the design of subsequent distributed energy management methods for the port microgrid, so it is necessary to prove whether the constructed port microgrid operating cost function is convex. As
Lemma 1. It is assumed that the cost function of electricity generation
Then, the cost function
Proof. The cost function
From Lemma 1, we know that
In order to build a green, low-carbon, and economic port microgrid, the objective of energy management for port microgrid is to minimize the operating cost and carbon emissions. Based on the operating cost function for port microgrid and considering the cost of port carbon emissions, the energy management model for port microgrid is constructed as follows:
where
Remark 3. The first term in Eq. 9, namely the operating cost of the port microgrid based on WE, is a convex function. The second item is the cost of carbon emissions for WE. As CWEs do not produce carbon emissions, that is,
Considering the distributed structure presented by the port microgrid, the convex optimization problem (9) with inequality constraints can be solved based on the distributed optimization method (10).
where
According to Eq. 10, a distributed solution method for the energy management problem for the port microgrid is proposed. The method is expressed in the following iterative way:
Since model (9) is differentiable and its gradient is continuous as a convex function, and there exists an auxiliary variable z satisfying condition (10) (Liu et al., 2017), the incremental cost of WEs can converge to an optimal solution, and then a distributed optimal solution to the energy management problem for the port microgrid is obtained.
In this subsection, we used MATLAB as an experimental tool to verify the effectiveness of the distributed energy management method for the port microgrid proposed in this article. The considered port microgrid model based on WEs is shown in Figure 2.
The port microgrid model contains 10 WEs, including 6 CWEs (2 WWEs and 1 PWE), 2 TWEs, and 2 SWEs. The port microgrid has a total of 41555W of load and line losses. The electricity required is provided by both the main grid and the WEs, at the cost of 5 Yuan per unit of carbon emissions. It is assumed that there are no energy losses in the generating equipment during the simulations for the heterogeneous WEs. The parameters related to the operation of the generating equipment in the heterogeneous WE during regular operation of the microgrid are shown in Table 1 (Huang et al., 2016).
An energy management model with the objective of minimizing the operating cost and carbon emissions of the port microgrid, without considering the trading of electricity between WEs and the trading of electricity between the WE and the main grid, is as follows:
It is assumed that the total energy losses remain constant during the regular operation of the port microgrid. It was solved by using the centralized method and the distributed method based on the leader-following consensus algorithm (Huang et al., 2016), respectively. The results of the operation of each generating equipment in the port microgrid are shown in Table 2.
According to Table 2, the centralized method and the leader-following consensus method result in different operating costs for the port microgrid, namely 30,821 Yuan and 30,801 Yuan, respectively.
In this study case, the load demand, transmission line energy losses, and main grid electricity price are kept constant in the port microgrid, and a distributed method (Liu et al., 2017) is used to solve the port microgrid energy management problem (13). The parameter
As can be seen from Figure 3, the incremental cost of WEs is ultimately 1.0 Yuan per kWh. In the port microgrid, TWEs are operating, and there is not only the operating cost of the WE but also carbon emissions cost. According to Table 3, the operating cost of the port microgrid during regular operation of the heterogeneous WEs is 30,381 Yuan. This case has a lower operating cost compared with Case 1 without carbon emissions cost.
In this case, the distributed method (11) proposed in this article is used to solve the energy management problem (9) for the port microgrid. It is assumed that load demand, transmission line energy losses, and main grid electricity price in the port microgrid remain constant.
A comparative analysis between Case 3 and Case 1 shows that the power output of heterogeneous WEs does not differ significantly under the three solution methods. Therefore, the distributed method proposed in this paper can enable port microgrid energy management. As can be seen from Figure 4, the incremental cost of WEs converges to 1.0 Yuan per kWh when the port microgrid is operating steadily. The output power and operating cost of the heterogeneous WEs in the port microgrid during regular operation can be seen in Table 3. The operating cost of the port microgrid is 30,792 Yuan, of which the cost of carbon emissions is 412.7 Yuan. In this study case, there are not only low operating costs but also low carbon emissions.
A comparison between Case 1 and Case 3 shows that the distributed solution method proposed in this article can solve the port microgrid energy management problem, indicating the effectiveness of the distributed solution method proposed in this article. In addition, Case 3 has lower operating costs compared with Case 1. The comparison between Case 2 and Case 3 shows that Case 3 is able to maximize the use of clean energy, which not only reduces the operating cost of the port microgrid but also reduces carbon emissions, contributing to the development of a green and low-carbon port.
The large-scale use of clean energy in port microgrids has given rise to WEs in the port, and the widespread presence of WEs has made the port microgrid a distributed structure. A distributed energy management strategy for WE-based port microgrid under a polymorphic network has been proposed in this article. First, this article has established a polymorphic energy management system for port microgrids based on WEs, ensuring reliable information interaction between heterogeneous WEs, including SWEs, and laying the foundation for the subsequent implementation of distributed energy management. Then, considering the characteristic of bidirectional energy transmission between WEs in the port, the operating cost function of WEs has been analyzed and established. Furthermore, an energy management model for the port microgrid has been constructed considering both the operating cost and the carbon emissions of WEs. Finally, this article has proposed a distributed method to solve the energy management problem of the port microgrid based on the multi-agents consensus method. Through the comparison and analysis of different simulation cases, it has been concluded that the method proposed in this article can not only reduce the operating cost of the port microgrid but also reduce carbon emissions, which can help the development and construction of the green low-carbon port.
The original contributions presented in the study are included in the article/Supplementary Material; further inquiries can be directed to the corresponding author.
HL constructed the polymorphic energy management system for the port microgrid. FT built the model, designed the method, and adapted the article. The experimental simulations, data processing, and draft writing were carried out by JW. QZ collated and revised the format of the references. CS participated in proofreading and organizational management. All authors have read and agreed to the publication of the manuscript.
This research was supported by the National Key R&D Program of China (2019YFB1802501), the Key Research Project of Zhejiang Lab (2021LE0AC02), the National Natural Science Foundation of China (under Grant Nos. 51939001, 61976033, 61751202, 61903092, and U1813203), the Science and Technology Innovation Funds of Dalian (under Grant No. 2018J11CY022), and the Liaoning Revitalization Talents Program (under Grant Nos. XLYC1908018 and XLYC1807046).
The authors declare that the research was conducted in the absence of any commercial or financial relationships that could be construed as a potential conflict of interest.
All claims expressed in this article are solely those of the authors and do not necessarily represent those of their affiliated organizations, or those of the publisher, the editors, and the reviewers. Any product that may be evaluated in this article, or claim that may be made by its manufacturer, is not guaranteed or endorsed by the publisher.
Alamoush, A. S., Ballini, F., and Ölçer, A. I. (2020). Ports' technical and operational measures to reduce greenhouse gas emission and improve energy efficiency: a review. Mar. Pollut. Bull. 160 (2020), 111508. doi:10.1016/j.marpolbul.2020.111508
Çağatay, I., and Jasmine, S. L. L. (2019). A review of energy efficiency in ports: Operational strategies, technologies and energy management systems. Renew. Sustain. Energy Rev. 112, 170–182. doi:10.1016/j.rser.2019.04.069
Çağatay, I., and Jasmine, S. L. L. (2021). Optimal energy management and operations planning in seaports with smart grid while harnessing renewable energy under uncertainty. Omega 103 (2021), 102445. doi:10.1016/j.omega.2021.102445
Coppola, T., Fantauzzi, M., Lauria, D., Pisani, C., and Quaranta, F. (2016). A sustainable electrical interface to mitigate emissions Due to power supply in ports. Renew. Sustain. Energy Rev. 54, 816–823. doi:10.1016/j.rser.2015.10.107
Fang, S., Wang, Y., Gou, B., and Xu, Y. (2020). Toward future green maritime transportation: An overview of seaport microgrids and all-electric ships. IEEE Trans. Veh. Technol. 69 (1), 207–219. doi:10.1109/TVT.2019.2950538
Gennitsaris, S. G., and Kanellos, F. D. (2019). Emission-aware and cost-effective distributed demand response system for extensively electrified large ports. IEEE Trans. Power Syst. 34 (6), 4341–4351. doi:10.1109/TPWRS.2019.2919949
Hein, K., Xu, Y., Gary, W., and Gupta, A. K. (2021). Robustly coordinated operational scheduling of a grid-connected seaport microgrid under uncertainties. IET Gener. Transm. Distrib. 15 (2), 347–358. doi:10.1049/gtd2.12025
Hu, Y., Li, D., Sun, P., Yi, P., and Wu, J. (2020). Polymorphic smart network: An open, flexible and universal architecture for future heterogeneous networks. IEEE Trans. Netw. Sci. Eng. 7 (4), 2515–2525. doi:10.1109/TNSE.2020.3006249
Hu, Y., Yi, P., Sun, P., and Wu, J. (2019). Research on the full-dimensional defined polymorphic smart network. J. Commun. 40 (08), 1–12. doi:10.11959/j.issn.1000−436x.2019192
Huang, B., Li, Y., Zhang, H., and Sun, Q. (2016). Distributed optimal Co-multi-microgrids energy management for energy internet. IEEE/CAA J. Automatica Sinica 3 (4), 357–364. doi:10.1109/JAS.2016.7510073
Huang, Y., Huang, W., Wei, W., Tai, N., and Li, R. (2022). Logistics-energy collaborative optimization scheduling method for large seaport integrated energy system. Proc. CSEE, 1–12. doi:10.13334/j.0258-8013.pcsee.211093
Kanellos, F. D. (2019). Multiagent-system-based operation scheduling of large ports’ power systems with emissions limitation. IEEE Syst. J. 13 (2), 1831–1840. doi:10.1109/JSYST.2018.2850970
Kanellos, F. D., Volanis, E. M., and Hatziargyriou, N. D. (2019). Power management method for large ports with multi-agent systems. IEEE Trans. Smart Grid 10 (2), 1259–1268. doi:10.1109/TSG.2017.2762001
Kermani, M., Parise, G., Shirdare, E., and Martirano, L. (2020). “Transactive energy solution in a port's microgrid based on blockchain Technology,” in 2020 IEEE international conference on environment and electrical engineering and 2020 IEEE industrial and commercial power systems europe (EEEIC/I&CPS europe), Madrid, Spain, 09-12 June 2020, 1–6. doi:10.1109/EEEIC/ICPSEurope49358.2020.9160833
Li, Y., Gao, D. W., Gao, W., Zhang, H., and Zhou, J. (2021). A distributed double-Newton descent algorithm for cooperative energy management of multiple energy bodies in energy internet. IEEE Trans. Ind. Inf. 17 (9), 5993–6003. doi:10.1109/TII.2020.3029974
Li, Y., Gao, D. W., Gao, W., Zhang, H., and Zhou, J. (2020). Double-mode energy management for multi-energy system via distributed dynamic event-triggered Newton-raphson algorithm. IEEE Trans. Smart Grid 11 (6), 5339–5356. doi:10.1109/TSG.2020.3005179
Liu, Q., Yang, S., and Hong, Y. (2017). Constrained consensus algorithms with fixed step size for distributed convex optimization over multiagent networks. IEEE Trans. Autom. Contr. 62 (8), 4259–4265. doi:10.1109/TAC.2017.2681200
Nikolaos, S., and Theocharis, T. (2021). Planning zero-emissions ports through the nearly zero energy port concept. J. Clean. Prod. 286 (2021), 125448. doi:10.1016/j.jclepro.2020.125448
Olivares, D. E., Cañizares, C. A., and Kazerani, M. (2014). A centralized energy management system for isolated microgrids. IEEE Trans. Smart Grid 5 (4), 1864–1875. doi:10.1109/TSG.2013.2294187
Parise, G., Parise, L., Martirano, L., Chavdarian, P. B., Su, C., and Ferrante, A. (2016). Wise port and business energy management: port facilities, electrical power distribution. IEEE Trans. Ind. Appl. 52 (1), 18–24. doi:10.1109/TIA.2015.2461176
Pourakbari-Kasmaei, M., Lehtonen, M., Contreras, J., and Mantovani, J. R. S. (2020). Carbon footprint management: a pathway toward smart emission abatement. IEEE Trans. Ind. Inf. 16 (2), 935–948. doi:10.1109/TII.2019.2922394
Shan, Q., Zhang, X., Zhang, Q., Sun, Q., and Cai, N. (2022). Distributed energy management for port power system under false data injection attacks. Autom 102 (2019), 34–44. doi:10.1155/2022/5995281
Sun, Q., Fan, R., Li, Y., Huang, B., and Ma, D. (2019). A distributed double-consensus algorithm for residential we-energy. IEEE Trans. Ind. Inf. 15 (8), 4830–4842. doi:10.1109/TII.2019.2921431
Sun, Q., Wang, R., Ma, D., and Liu, Z. (2017). An islanding control strategy research of we-energy in energy internet. Proc. CSEE 37 (11), 3087–3098+3364. doi:10.13334/j.0258-8013.pcsee.161002
Tang, R., Li, X., and Lai, J. (2018). A novel optimal energy-management strategy for A maritime hybrid energy system based on large-scale global optimization. Appl. Energy 228 (2018), 254–264. doi:10.1016/j.apenergy.2018.06.092
Wen, S., Zhao, T., TangXu, Y, Y., Zhou, M., Zhu, M., Fang, S., et al. (2021). Coordinated optimal energy management and voyage scheduling for all-electric ships based on predicted shore-side electricity price. IEEE Trans. Ind. Appl. 57 (1), 139–148. doi:10.1109/TIA.2020.3034290
Yang, T., Yi, X., Wu, J., Yuan, Y., Wu, D., Meng, Z., et al. (2019). A survey of distributed optimization. Annu. Rev. Control 47 (2019), 278–305. doi:10.1016/j.arcontrol.2019.05.006
Zhang, Q., Shan, Q., and Li, T. (2020). “Large port energy management based on distributed optimization,” in 2020 7th international conference on information, cybernetics, and computational social systems (ICCSS), Guangzhou, China, November 13–15, 2020, 108–113. doi:10.1109/ICCSS52145.2020.9336919
Keywords: port microgrid, clean energy, low carbon, distributed energy management, we-energy, polymorphic network
Citation: Teng F, Wang J, Luo H, Zhang Q and Shen C (2022) Distributed low-carbon energy management method for port microgrid based on we-energies under polymorphic network. Front. Energy Res. 10:952396. doi: 10.3389/fenrg.2022.952396
Received: 25 May 2022; Accepted: 29 June 2022;
Published: 22 July 2022.
Edited by:
Bonan Huang, Northeastern University, ChinaReviewed by:
Yanfang Hou, Chongqing University of Technology, ChinaCopyright © 2022 Teng, Wang, Luo, Zhang and Shen. This is an open-access article distributed under the terms of the Creative Commons Attribution License (CC BY). The use, distribution or reproduction in other forums is permitted, provided the original author(s) and the copyright owner(s) are credited and that the original publication in this journal is cited, in accordance with accepted academic practice. No use, distribution or reproduction is permitted which does not comply with these terms.
*Correspondence: Hanguang Luo, bHVvaGdAemhlamlhbmdsYWIuY29t
Disclaimer: All claims expressed in this article are solely those of the authors and do not necessarily represent those of their affiliated organizations, or those of the publisher, the editors and the reviewers. Any product that may be evaluated in this article or claim that may be made by its manufacturer is not guaranteed or endorsed by the publisher.
Research integrity at Frontiers
Learn more about the work of our research integrity team to safeguard the quality of each article we publish.