- 1Economic and Technological Research Institute, State Grid Fujian Electric Power Co., Ltd., Fuzhou, China
- 2China Center for Energy Economics Research, School of Economics, Xiamen University, Xiamen, China
To achieve carbon peak and carbon neutrality targets, it has become a common choice for all countries to introduce the carbon emissions trading market to foster low carbon sustainable development. The construction of national carbon emissions trading market in China is still in its initial stage. However, the carbon market in Fujian province has already accumulated certain experience, and its unique energy mix of “higher share of the clean energy and low share of fossil fuels consumption” can provide guidance to China’s future development. Therefore, an accurate forecast of the carbon price in Fujian province not only provides conducive suggestions for the further optimization of the carbon market in Fujian province, but also offers a significant reference for the development of China’s carbon trading market. By adopting the effective daily data from 2017.01 to 2022.02, this paper predicts the carbon emissions trading price in Fujian province based on the BP neural network model and analyzes the mechanism of different influencing factors on carbon price from six dimensions. The results show that the BP neural network model works well in predicting carbon price in Fujian province and in the impact mechanism analysis. This paper also puts forward corresponding policy recommendations, which provide theoretical support for the sound development of the carbon market in Fujian province.
1 Introduction
With rapid economic growth and the surge in energy consumption, climate change caused by excessive emissions of greenhouse gases has become a global concern. Since 2020, the Chinese government has put forward “dual carbon” goals of “peaking its carbon emissions before 2030 and achieving carbon neutrality by 2060” to ease the pressure on global carbon emissions. As one of the largest economies on the southeast coast of China, Fujian province actively promotes the implementation of the country’s “dual carbon” goals. The province makes joint efforts from both the government control and the market regulation, it not only accelerates the green-oriented transition of energy, but also vigorously develops the Fujian carbon emissions trading market.
In terms of the evolution of the energy system in Fujian province, the energy mix of Fujian province has a distinctive feature of “limited coal reserves, no oil and gas”. However, as a major coastal province, Fujian province is abundant in clean energy, such as wind energy, hydro energy, and solar energy, to name a few. With the increasing pressure on greenhouse gas emissions reduction, the country exercises greater regulation in carbon emissions reduction. In response to the call for energy conservation and emission reduction and based on its actual conditions, Fujian province optimized the energy mix by implementing measures including cutting coal overcapacity, closing inefficient coal mines, and actively developing clean energy like nuclear power and wind power to ensure energy supply. From the perspective of energy production, before 2011, the proportion of coal production in Fujian province was basically higher than 50%. After long-term governance, in 2019, the proportion of coal production was only 14.8%. and the clean energy production represented by hydropower, wind power and nuclear power accounted for 78.6%, which shows that Fujian province has made remarkable achievements in optimizing the energy production structure. At the same time, in order to further consolidate the achievements of green development, the provincial government issued the “Implementation Plan for Accelerating the Establishment and Improvement of a Green, Low-Carbon and Circular Development Economic System in Fujian Province” in 2021, indicating that the government encourages green and low-carbon transformation of the energy system, vigorously promotes the development of nuclear power, wind power, photovoltaic and other projects, promotes the deep integration of distributed energy technology, smart grid technology, energy storage technology, etc., and improves the ability to clean energy consumption and regulation. It can be noticed that under the guidance of the government, the energy mix of Fujian province is gradually transforming to a green and low-carbon direction, which will reduce the carbon emissions and carbon emissions intensity.
From the perspective of the development of the carbon emissions trading market in Fujian province, Fujian province, as one of the eight provincial-level pilot programs of China’s carbon market, has accumulated experience. Since 2013, the eight major carbon markets in China began operating one after another. The Fujian carbon market officially went into operation at the end of 2016, and operated in parallel with the national carbon market in 2021. The launching of the Fujian carbon market introduces market forces to reduce carbon emissions. To achieve emission reduction, the market measures the cost of carbon emissions in real time through changes in the carbon price, and turns emissions into a production factor that can be bought or sold.
Although there is a gap in the launching and experience between China’s and developed countries’ carbon markets, and the current coverage of carbon emissions in China’s carbon market is slightly lower than that of the EU carbon trading system, China’s carbon market and the eight pilot carbon markets have covered the majority of regions including the East, Mid and West regions. Taking the carbon market in Fujian Province as an example, the carbon market in Fujian Province has the characteristics of wide coverage and complete trading varieties. Since the formal trading in january 2017, the trading volume of the carbon emission right market in Fujian Province has been increasing year by year. By February 2022, the cumulative trading volume has reached 12.4935 million tons, which indicates that the carbon trading market in Fujian has been expanding and has played a role in controlling the total amount of carbon emission reduction. The increase of carbon trading volume is bound to reflect the carbon price. The average monthly trading price is shown in Figure 1.
As shown in Figure 1, the average monthly carbon price of Fujian province shows a significant downward trend of fluctuation, from 38.02 yuan/ton in January 2017 to 12.57/ton yuan in February 2022, indicating that the carbon cost of Fujian province has decreased and the economic structure, industrial structure and energy mix of the Province is steadily shifting to green and low-carbon; Comparing the carbon price of Fujian Province with that of other provinces, it can be found that the cost of carbon emission in Fujian Province was second only to Beijing in the early stage. With the passage of time, the carbon price of Fujian Province showed a downward trend, ranking last among the pilot provinces and cities from March 2019. In terms of the comparison between Fujian province and the national monthly average carbon price, as the national carbon market was officially launched in 2021, compared with the average monthly carbon price of Fujian province and the national market in 2021 and onwards, the national average monthly carbon price fluctuates in the range from 40.78 yuan/ton to 57.72 yuan/ton, which is significantly higher than the average monthly carbon price in Fujian province. In addition, the lowest national average monthly carbon price is still higher than the highest average monthly carbon price in Fujian province, showing that the carbon emission cost in Fujian province is much lower than that of the whole country. Therefore, Fujian province has laid a solid foundation in green and low-carbon transformation, and the changes in the carbon price can effectively evaluate its carbon emissions process in real time.
According to the above analysis, the promotion of energy transformation and the efficient operation of the carbon market in Fujian province can both provide sufficient impetus for the realization of the “carbon peaking and carbon neutrality goals”. It should be noted that although the energy mix of Fujian province has been optimized to a certain extent, there are still some practical problems that are difficult to solve in the short term. Take nuclear power as an example, despite the fact that the installed capacity of nuclear power has increased rapidly in the short run, overcapacity will become an issue that needs to be addressed due to the constraints of current nuclear power storage technology and the limited actual increase in power demand. So the optimization of energy mix in Fujian province plays an important role in realizing the “dual carbon” goals. But due to practical and technical constraints, it is difficult to achieve the goal of substantial emission reduction in the short term only by relying on the optimization of the energy mix. Therefore, the key to realizing the “dual carbon” goals as scheduled is to depend on the Fujian carbon market to adjust carbon costs in real time, thereby improving the efficiency of market resource allocation so as to minimize carbon emissions on the basis of ensuring economic development.
As a key parameter of the carbon market, carbon price volatility will have a direct impact on market changes. Considering that China’s carbon market has just been launched with price fluctuations, which is not conducive for investors and market regulators to make reasonable predictions, thereby reducing the effectiveness of the carbon market and affecting investment and regulatory decision-making, this paper will first sort out the key factors that affect the carbon price, and then make a reasonable prediction of the carbon price, and finally determine an effective prediction model to provide theoretical support for the further optimization of the carbon market in Fujian province.
2 Literature Review
As a key parameter in the carbon market, effective forecasting of carbon price can improve the operating efficiency of the carbon market. Since the operation of the carbon market, the related research on the carbon emissions trading price has always attracted the attention of domestic and foreign scholars with rich results. The literature review will focus on three parts: the status quo of China’s carbon market, the influencing factors of the carbon price, and carbon price prediction, which will provide a solid theoretical foundation for this study.
First, the status quo of China’s carbon market. After several years of development, China’s carbon market has achieved remarkable results and accumulated lots of experience (Zhang et al., 2014; Karplus and Zhang, 2017; Surendra and Rajesh, 2020), but it still faced serious problems. Xu and Salem (2021) pointed out that although China’s allowance market and certified emission reductions (CERs) laid a certain foundation for development, practical problems such as the low proportion of auction quota and uncertainty of CERs would reduce the effectiveness of the carbon market; Wang et al. (2021) showed that as China’s carbon market development was still in the initial stage, combined by the pressure of achieving “dual carbon” goals, the emission reduction in the short term would face greater pressure and higher cost. At the same time, the impediments including inadequate technical guarantee, imperfect mechanism construction and unbalanced regional development also restricted the development of the market. From the perspective of external influencing factors, Wu et al. (2022) also indicated that the current legal system of China’s carbon market was incomplete, making it difficult to integrate with the international carbon market. In addition, Jia et al. (2021) focused on the impact of COVID-19 on China’s carbon market, and believed that the epidemic would widen the gap between regional development and affected the international carbon price, and the global economic downturn would also have a certain impact on China’s carbon market. Generally speaking, the current problems faced by China’s carbon market have been in line with the law in the early stages of development (Munnings et al., 2016; Pang and Duan, 2015; Du et al., 2021). Zhu et al. (2021) stated that it was still an effective way to reduce emissions despite problems existing in the market. Zhao et al. (2016) demonstrated that a staged carbon market should be launched with continuous improvement, which should be refined according to the actual situation in different periods. For example, during the “14th Five Year Plan” period, the carbon market should cover energy-intensive as well as emission-intensive industries, and determine the emission benchmark. During the “15th Five Year Plan” period, the allowance distribution of competitive auction and fixed price sale can be gradually expanded to achieve “stability reducing emissions”. Atsalakis (2016) showed that forecasting the carbon price in the short term can improve the market function and forced other areas to optimize. Therefore, predicting the carbon price plays an important role in the optimization of the carbon market.
Second, the research on the influencing factors of carbon price. The carbon market is an important way to introduce a market mechanism in carbon emissions trading (Wang et al., 2022), and carbon price is an effective indicator that can effectively measure the changes in supply and demand in the carbon market (Fleschutz et al., 2021). Therefore, it is of great significance to investigate the influencing factors of carbon price for the efficient operation of carbon market. Jiao et al. (2018) showed that the carbon market has financial characteristics, which closely related to the macroeconomic situation. Batten et al. (2021) believed that energy prices were the factor affecting carbon price. The carbon market is vulnerable to climate impacts, mostly manifested in extreme weather, that is, when the temperature exceeds or falls below a certain threshold, energy demand will increase, thereby increasing carbon emissions. With the deepening of study, the study on energy prices has been refined to specific fossil energy prices. Ding et al. (2020) found that coal price was the best predictor of carbon price. Wang and Guo (2018) stated that oil market changes should have a stronger influence on carbon markets than other types of energy markets. Han et al. (2019) argued that the carbon emissions generated by natural gas were much lower than other types of fossil fuels. Chu et al. (2020) found that natural gas prices have a stronger effect on carbon prices as quantiles increased in the Beijing and Hubei ETS pilots. With regard to the research on China’s carbon market, Zhou and Li (2019) discovered that energy prices and macroeconomic indicators had an important impact on the carbon price like other foreign carbon markets. Kim and Bae (2022) found that in addition to conventional factors, the industrial production had an important impact on carbon prices. Pan et al. (2020) believed that China’s carbon market prices are affected by foreign economies such as exchange rates and foreign carbon market prices.
Third, the study on carbon price forecast. From the perspective of prediction methods, Fu (2018) predicted the thermal coal price of Qinhuangdao port based on the quadratic exponential smoothing method, showing that it is applicable to use the prediction model with an accuracy of over 90%. Zhao et al. (2021) used multi-factor integrated model to simulate the evolution of carbon prices. The study discovered that the mechanism conversion model provides more effective forecasting. In order to improve the prediction accuracy, Zou and Li (2022) used the Monte Carlo method to test the fitting of multiple coal price models under the background of carbon peaks, indicating that the prediction results of the geometric Brown model and the MR model in the short term were consistent while showing differences for medium and long-term forecasts. In order to improve the prediction accuracy, Naser (2016) introduced time-varying characteristics into the factors affecting oil price based on the dynamic model averaging theory and predicted the pork price. It is found that the oil price prediction model based on the dynamic model averaging can effectively improve the prediction accuracy. Although the above prediction methods can effectively fit the linear changes in the price, they cannot capture the nonlinear components in the price changes. To compare the prediction difference between the linear and nonlinear models, Matyjaszek et al. (2019) used the ARIMA model and the generalized regression neural network model to predict the coking coal price, and found that the prediction model of the generalized regression neural network was better than the linear ARIMA model. Some scholars applied linear research methods to domestic carbon price forecasts. For example, use ARCH model clusters to investigate the characteristics of carbon price fluctuations while most scholars adopted machine learning models to investigate carbon prices.
Based on the above analysis, it can be seen that the carbon market in Fujian Province, as an important starting point to promote the “double carbon” goal, is in the early stage of development and faces many challenges, but the carbon price, as a means of “marketization” to solve environmental problems, is an important battlefield to help reduce emissions. Transmitting a reasonable carbon price to enterprises and society will promote the flow of social capital to low-carbon fields and provide impetus for cultivating and innovating low-carbon economy. Therefore, the reasonable prediction of carbon price can promote the gradual optimization of carbon market in Fujian Province and force the development of relevant fields. Moreover, the carbon price is affected by multiple factors. In the process of carbon price prediction, it is necessary to take into account the multi-dimensional factors such as energy price, macroeconomic impact, industrial structure, climate change, foreign carbon price and international financial market. At the same time, the prediction accuracy of machine learning model is better than that of linear model. Based on this, this paper will use BP neural network model to predict the carbon price in Fujian Province, so as to improve the prediction accuracy.
The remainder of this paper is organized as follows. Section 3 is mechanism analysis and model description, mainly explaining the mechanism of influencing factors and introducing the BP neural network model; Section 4 presents data description and simulation prediction, which introduces the indicators and data sources, and simulation prediction analysis is carried out; Section 5 concludes with some policy suggestions.
3 Mechanism Analysis and Model Description
In forecasting analysis, the multi-factor factor analytic approach that takes into account different dimensions is more accurate than the single factor analytic approach. Therefore, this section first describes the mechanism of each influencing factor on carbon price based on different dimensions, then briefly explains the BP neural network model.
3.1 Description of the Influence Mechanism of Carbon Price
Combined with the above analysis, this paper will investigate the influence mechanism of the carbon price from six dimensions: fossil fuels price, macroeconomic development, industrial structure, climate change, foreign carbon price, and international financial market. Details are as follows:
3.1.1 Prices of Fossil Fuels
Changes in fossil fuel prices have a direct impact on carbon prices. Overall, fossil fuel consumption produces a large amount of carbon dioxide, which is also the main source of carbon emissions. When the price of fossil fuels is relatively high, energy consumption will decrease to a certain extent, which will lead to a decrease in the overall carbon emissions level. The reduction in carbon emissions level will reduce the demand for carbon emissions allowances, which will in turn reduce carbon price. On the contrary, when the price of fossil fuels is relatively low, the price of carbon will show an upward trend. From the perspective of price changes of different types of fossil fuels, the carbon dioxide emissions of coal and oil consumption are much higher than that of natural gas, and all kinds of fossil fuels are equal to each other. For industries that can use all types of fossil fuels, under the premise of generating the same energy, when the price of natural gas is lower than the price of other fossil fuels, the use of natural gas can reduce carbon emissions and the demand for carbon emissions allowances, thereby reducing carbon price. It also demonstrates that increasing the proportion of cleaner energy use can reduce carbon prices. Therefore, an increase in the price of fossil fuels can reduce the carbon price, and a decrease in the relative price of natural gas can also lead to a decrease in the price of carbon.
3.1.2 Macroeconomic Environment
Macro-environment has a profound impact on the carbon market. The macroeconomic structure will have a direct impact on carbon price. The energy-intensive and emission-intensive industries will greatly impact the energy efficiency and generate a large amount of carbon dioxide, thereby increasing the demand for carbon emissions allowances and carbon price. The low-carbon, green and intensive economic structure will improve energy efficiency, and significantly reduce carbon emissions, thus reducing the demand for carbon emissions allowances and driving down the carbon price. Macroeconomic development is a dynamic process. Under the current requirements of the “dual carbon” goals, the transformation of the economic structure to a green, low-carbon, and intensive type is an inevitable trend. However, considering that the macroeconomic transformation presents fluctuating changes instead of a simple linear process, the carbon emissions generated in this process will also fluctuate, resulting in volatile carbon prices. In addition, in the process of macroeconomic development, along with technological upgrades and production changes, more advanced production technologies will greatly improve the efficiency of production factors, thereby reducing energy consumption and reducing carbon emissions, leading to lower carbon prices. Therefore, the macroeconomic dynamics will have a direct impact on carbon prices.
3.1.3 Industrial Structure
The impact of industrial structure on carbon price is reflected at the industrial level and enterprise level. As for the industrial level, different industrial structures mean different levels of development. Regional development will inevitably go through the process of industrialization. The period of rapid industrial development, especially the rapid development of heavy industry, must be accompanied by a large amount of energy consumption and a large number of emissions. Industrial pollution is the culprit behind environmental degradation and climate issues. Large industrial emissions will increase the demand for carbon emissions allowances and increase the carbon price. Therefore, to reduce industrial pollution, speeding up the adjustment of industrial structure and the development of the tertiary industry can be introduced in Fujian province. From the enterprise level, compared with other enterprises, the impact of the carbon price on industrial enterprises is the most obvious. The energy consumption and carbon emissions of industrial enterprises are much higher than other enterprises. The high carbon price will directly increase the production costs of industrial enterprises and reduce the profit of industrial enterprises. Therefore, an increase in the carbon price will force industrial enterprises to choose clean energy, and prompt enterprises to accelerate the development of green and efficient production technologies, thereby reducing carbon emissions and reducing the demand for carbon allowances, which will lead to a decline in carbon prices. So the industrial structure, especially the level of industrial development, will have an important impact on the carbon price.
3.1.4 Climate Change
Climate change will affect human production and lifestyle, which will further lead to carbon price change. Specifically, from the perspective of residents’ lifestyles, as greenhouse gases emit excessively, the extreme weather issues caused by rising temperature have become increasingly prominent. When the temperature is too high or too low, residents will use devices for cooling or heating, which will result in a short-term surge in energy consumption and carbon dioxide emissions, thereby increasing the demand for carbon emissions allowances and carbon price. Generally speaking, compared with spring and autumn, the impact of extreme weather on carbon price mostly occurs in summer and winter. Meanwhile, the residential electricity consumption in summer and winter is also higher than the annual average, which is a main manifestation of the increase in energy consumption. From the perspective of production, some equipment requires certain temperature when producing. Therefore, if extreme weather occurs, enterprises need to pay extra energy costs to support the operation of equipment, which will add additional carbon emissions, thereby increasing the demand for carbon emissions allowances and the carbon price. So climate change, especially when extreme weather occurs, will increase the carbon price.
3.1.5 Impact of Foreign Carbon Prices
Foreign carbon prices have some influence on China’s carbon price. On the one hand, the development of China’s national carbon market is in the initial stage. However, foreign carbon markets have developed for a long time and have relatively mature market. Moreover, carbon emissions is a global concern. Consequently, when setting carbon emissions allowances and carbon price, China’s carbon market will refer to foreign carbon markets to some extent. On the other hand, the gap between China’s economic development and other countries’ will lead to pricing differences in carbon market. If the carbon price in China’s carbon market is lower, some multinational companies will speculate and purchase a large amount of carbon emissions allowances in China’s carbon market, therefore increasing the demand for carbon emissions allowances in the China’s market and prompting an increase in China’s carbon price until it is on a par with the international carbon price. At present, China’s carbon market has not yet integrated with the international carbon market, so the number of speculators is relatively small. But when the China’s carbon market is fully developed and integrated with the international carbon market, the problem of speculation will become more serious. Therefore, foreign carbon prices will not only provide a reference for the setting of China’s carbon price, but also increase China’s carbon price due to speculation.
3.1.6 International Financial Markets
The international financial market will affect China’s carbon price through exchange rate changes. As countries deepen their economic ties, the import and export of energy has become the basis for the economic development of every country. For energy import enterprises, when the exchange rate rises, the relative price of foreign energy will decrease, so will the energy cost of the enterprises. So enterprises will expand their production and increase energy consumption, resulting in excessive carbon dioxide emissions and increased demand for carbon emissions allowances, causing carbon price to rise. When the exchange rate falls, the carbon price will also decrease. For energy export enterprises, when the exchange rate rises, the price of exporting energy will increase, which will in turn reduce the sales of products, resulting in a slowdown in the production rate of related enterprises and reduction in CO2 emissions and carbon emissions allowances, leading to carbon price decrease. It can be discovered that an increase in the exchange rate will promote the production of energy imported enterprises, leading to an increase in carbon price, also it can lower the carbon price by reducing the production of energy export enterprises. Therefore, the impact of exchange rate on carbon price depends on the net effect of energy import enterprises and energy export enterprises.
3.2 Model Description
BP (Back Propagation) neural network model is a representative of the machine learning models, and it is the most mature and widely used machine learning model. The core idea of this model is to take the square of the network error as the objective function, and use gradient descent to calculate the minimum value of the objective function. The algorithm of this model is mainly divided into signal forward propagation and error back propagation, and it does not consider the mapping equation between input and output, but explores the potential learning rules through continuously training the data itself. Therefore, the algorithm can discover the linear and nonlinear characteristics between input and output. At the same time, the BP neural network model is highly adaptive, which can effectively improve prediction accuracy. The algorithm of the BP neural network model is mainly divided into the following steps:
Step 1. Network initialization, determine the number of nodes at each layer of the network and the weights of connections, and initialize the threshold of the hidden layer and the output layer, and set the learning rate and the neuron excitation function;
Step 2. Computing hidden layer output, and by utilizing the input vector, the weights of connections and the hidden layer threshold to calculate the hidden layer output;
Step 3. Computing output layer output, by using weights of connections, output layer threshold and hidden layer output to calculate the output layer output;
Step 4. Using the output layer output and the expected output to conduct error calculation, weight update and threshold update successively.
Step 5. If the iteration is not over, go back to Step 2; otherwise, end the calculation.
4 Data Description and Simulation Analysis
Based on the mechanism analysis of carbon price and the introduction of the BP model in the previous section, this section first describes the indicators and data, and then a simulation analysis is conducted to predict the carbon price in Fujian province.
4.1 Data Description
Since the establishment of carbon market in Fujian province was relatively late, with the carbon price first disclosed on 9 January 2017, the sample period of in this paper ranges from 9 January 2017 to 25 February 2022. Among them, 640 days’ data is collected. The data adopted in this paper are all obtained from the Wind database and CSMAR database.
For forecasting data, this paper selects the average daily strike price of carbon price in the carbon emissions trading market in Fujian province. From the perspective of influencing factors, combined with the previous analysis, this paper selects fossil fuel prices, macroeconomic development, industrial structure, climate change, foreign carbon prices, and international financial markets as indicators. Given the influence of different fossil fuel prices on carbon price, coal price, oil price, and natural gas price are used to represent the price of fossil fuel prices. Because Fujian province lacks coal resources and most of the coal are imported from other provinces, the coal price is represented by the National Thermal Coal Futures Closing Price, the retail price of No. 0 diesel in Fujian province is the oil price, and the market price of Fuzhou industrial pipeline gas refers to the natural gas price. In order to effectively measure the development level and market level of macroeconomic, the CSI 300 index is adopted to represent the macroeconomic development level, and the 1-year treasury bond interest rate is utilized to represent the macroeconomic market level. As for the industrial structure, the above analysis points out that industrial development plays a key role in affecting carbon emissions, and the manufacturing industry is an important component of the industry, so this paper uses the Shenzhen Stock Exchange Manufacturing Index Closing Price to represent the industrial structure. Considering that different degrees of climate change have different impacts on carbon price, therefore the absolute value between daily average temperature and the suitable temperature denotes climate change, of which the suitable temperature is 25°C. Considering that the EU has the most mature carbon market, the EUA futures settlement price is selected to represent the impact of foreign carbon prices. In regard to the impact of the international financial market, this paper selects the exchange rate of RMB against the US dollar and the exchange rate of RMB against the euro respectively to reveal the influence of the international financial market. The details are shown in Table 1.
It can be discovered from the above table that there is a large gap between each indicator and the dimension. In order to avoid the influence, the min-max standardization method is adopted to normalize each index, so that the value range of each index is mapped between [0,1]. For any index X, the specific formula is as follows:
4.2 Simulation Analysis
Among the 640 samples, this paper uses 619 samples (about 97%) from 2017 to 2021 as the training data set, and 21 samples (about 3%) in 2022 as the test data set for prediction. Matlab 2017bis used to conduct simulation analysis. In order to avoid overfitting, newff function is selected, and the calculation formula for the number of neurons is
From Figure 2, the goodness of fit of all the samples is 0.9237, while the goodness of fit of the training data set is 0.9270, and the goodness of fit of the validation data set is 0.9301, the goodness of fit of the test data set is 0.8974. Moreover, the average goodness of fit is basically around 0.9, demonstrating a sound fitting effect. In addition, the mean square error (MSE) of this prediction is 7.7569, and the root mean square error (RMSE) is 2.7851, which also shows that the prediction effect is relatively good.
From Figure 3, it can be discovered that the predicted value of carbon price is obviously lower than the real value in the early stage of prediction. However, as time prolongs, the gap between the real value and the predicted value gradually decreases until it is basically fitted. This indicates that the BP neural network model has a good effect on the prediction of carbon price in Fujian province.
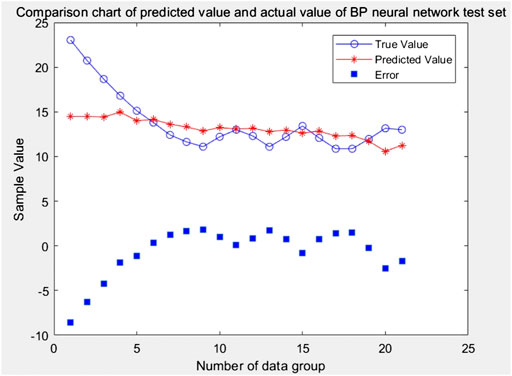
FIGURE 3. Comparison between the true value and the predicted value of the carbon price in Fujian province.
It can be seen from Table 2 that in the early stage of the forecast, the predicted value of carbon price is 8.57 lower than the real value. But in the fourth forecast period (i.e., 6 January 2022), the forecast error is controlled within 2, and the error of some forecast periods is within 1.5, which shows a sound prediction effect of the BP neural network model on carbon price in Fujian province, and also proves that the influencing factors selected in this paper have a significant impact on the carbon price.
5 Conclusion and Policy Recommendations
Based on the BP neural network model, this paper uses the available daily data from January 2017 to February 2022 to predict the carbon emission trading price in Fujian province and analyze the mechanism of different influencing factors on carbon prices. It can be discovered that the BP neural network model works well in predicting the carbon price in Fujian province, which indicates that in the era of big data, a machine learning model with nonlinear and non-parametric characteristics is applicable for high-dimensional system prediction. At the same time, the good prediction effect also shows that the mechanism analysis of carbon price influencing factors in this paper is effective. The optimization of the carbon market in Fujian province can be carried out from six dimensions: fossil fuel prices, macroeconomic development, industrial structure, climate change, foreign carbon prices, and international financial markets. Based on the above conclusions, the policy recommendations are as follows:
Firstly, machine learning models represented by the BP neural network model should be used more widely in economic forecasting. With the advent of the big data era, it is difficult for linear models represented by the time series model to accurately predict the current economy. The setting limit of the linear equation cannot present the nonlinear components in economic development, but the coordinated development of multi-domain markets in the context of the market economy means that the uncertainty and nonlinear components in economic development will increase. Therefore, using a machine learning model with nonlinear and non-parametric features can discover the hidden relationships by training data itself, thereby predicting the economic development accurately.
Secondly, from the perspective of domestic influencing factors, the development of the carbon market in Fujian province needs to focus on fossil fuel prices, macroeconomic development, industrial structure and climate change. Specifically, the development of clean energy in Fujian province enjoys a solid foundation, so it should continue to enhance the development of clean energy, reduce the use of fossil fuel and the demand for carbon emissions allowances. Ensuring the stable economic development of Fujian province so as to provide an enabling business environment for the carbon market operation. Fujian needs to comprehensively upgrade its traditional industries, accelerate the development of the three leading industries, promote the large-scale development of strategic emerging industries, promote the industry to move towards the middle and high end, and build a large advanced manufacturing province. To overcome challenges of the climate change, response mechanisms should be designed to reduce the impact of extreme weather on the surge in carbon emissions. In conclusion, Fujian province should take carbon price as the reference standard to vigorously develop green economy so that the dual carbon goals can be achieved.
Thirdly, from the perspective of foreign influencing factors, the stable development of Fujian province’s carbon market needs to attach importance to foreign carbon prices and international financial markets. Although Fujian province’s carbon market has not yet integrated with the international carbon markets, the speculation caused by the carbon price gap still exists. For Fujian province, China’s carbon price is already lower than the international carbon price and the carbon price in Fujian province is about 30% of the price in China’s carbon market. The huge arbitrage space will bring unfavorable factors into Fujian province’s carbon market. So, the market should establish a system to avoid the adverse impact. Meanwhile, as a major energy importer, the exchange rate between different countries will also exert great impact on Fujian province’s carbon market. This indicates that Fujian province should predict its energy demand accurately and vigorously develop clean energy storage projects to reduce reliance on importing energy, thereby reducing the impact of the international financial market on the carbon market in Fujian province.
The effective prediction of carbon price is conducive to the optimal development of carbon market in Fujian Province. Therefore, in the future, we can further explore the prediction methods of medium - and long-term carbon prices.
Data Availability Statement
The original contributions presented in the study are included in the article/Supplementary Material, further inquiries can be directed to the corresponding author.
Author Contributions
YD: Writing-original draft, Formal Analysis, Methodology, Software. KC: Writing-review and editing, Investigation, Software; SC: Writing-original draft, Conceptualization, Data curation. KY: Writing-review and editing, Supervision, Methodology, Validation.
Funding
This paper is supported by the National Natural Science Foundation of China (Grant Nos. 71874149, 71934001), National Social Science Fund of China (Grant No. 20ZDA084), and the State Grid Fujian Electric Power Company research project “Evaluation of the Effectiveness of Energy Transitions and Suggestions in Fujian Province”.
Conflict of Interest
YD, KC, and SC were employed by the Company Economic and Technological Research Institute, State Grid Fujian Electric Power Co., Ltd.
The remaining author declares that the research was conducted in the absence of any commercial or financial relationships that could be construed as a potential conflict of interest.
Publisher’s Note
All claims expressed in this article are solely those of the authors and do not necessarily represent those of their affiliated organizations, or those of the publisher, the editors and the reviewers. Any product that may be evaluated in this article, or claim that may be made by its manufacturer, is not guaranteed or endorsed by the publisher.
References
Atsalakis, G. S. (2016). Using Computational Intelligence to Forecast Carbon Prices. Appl. Soft Comput. 43, 107–116. doi:10.1016/j.asoc.2016.02.029
Batten, J. A., Maddox, G. E., and Young, M. R. (2021). Does Weather, or Energy Prices, Affect Carbon Prices? Energy Econ. 96, 105016. doi:10.1016/j.eneco.2020.105016
Chu, W., Chai, S., Chen, X., and Du, M. (2020). Does the Impact of Carbon Price Determinants Change with the Different Quantiles of Carbon Prices? Evidence from China ETS Pilots. Sustainability 12, 5581. doi:10.3390/su12145581
Ding, S., Xu, N., Ye, J., Zhou, W., and Zhang, X. (2020). Estimating Chinese Energy-Related CO2 Emissions by Employing a Novel Discrete Grey Prediction Model. J. Clean. Prod. 259, 120793. doi:10.1016/j.jclepro.2020.120793
Du, K., Cheng, Y., and Yao, X. (2021). Environmental Regulation, Green Technology Innovation, and Industrial Structure Upgrading: The Road to the Green Transformation of Chinese Cities. Energy Econ. 98, 105247. doi:10.1016/j.eneco.2021.105247
Fleschutz, M., Bohlayer, M., Braun, M., Henze, G., and Murphy, M. D. (2021). The Effect of Price-Based Demand Response on Carbon Emissions in European Electricity Markets: The Importance of Adequate Carbon Prices. Appl. Energy 295, 117040. doi:10.1016/j.apenergy.2021.117040
Fu, J. (2018). Research on Prediction of Thermal Coal Price Based on Exponential Smoothing Method. Coal Econ. Res. 38 (07), 6–12. doi:10.13202/j.cnki.cer.2018.07.001
Han, M., Ding, L., Zhao, X., and Kang, W. (2019). Forecasting Carbon Prices in the Shenzhen Market, China: The Role of Mixed-Frequency Factors. Energy 171, 69–76. doi:10.1016/j.energy.2019.01.009
Jia, Z., Wen, S., and Lin, B. (2021). The Effects and Reacts of COVID-19 Pandemic and International Oil Price on Energy, Economy, and Environment in China. Appl. Energy 302, 117612. doi:10.1016/j.apenergy.2021.117612
Jiao, L., Liao, Y., and Zhou, Q. (2018). Predicting Carbon Market Risk Using Information from Macroeconomic Fundamentals. Energy Econ. 73, 212–227. doi:10.1016/j.eneco.2018.05.008
Karplus, V. J., and Zhang, X. (2017). Incentivizing Firm Compliance with China's National Emissions Trading System. Eeep 6, 73–86. doi:10.5547/2160-5890.6.1.vkar
Kim, P., and Bae, H. (2022). Do firms Respond Differently to the Carbon Pricing by Industrial Sector? How and Why? A Comparison between Manufacturing and Electricity Generation Sectors Using Firm-Level Panel Data in Korea. Energy Policy 162, 112773. doi:10.1016/j.enpol.2021.112773
Matyjaszek, M., Riesgo Fernández, P., Krzemień, A., Wodarski, K., and Fidalgo Valverde, G. (2019). Forecasting Coking Coal Prices by Means of ARIMA Models and Neural Networks, Considering the Transgenic Time Series Theory. Resour. Policy 61, 283–292. doi:10.1016/j.resourpol.2019.02.017
Munnings, C., Morgenstern, R. D., Wang, Z., and Liu, X. (2016). Assessing the Design of Three Carbon Trading Pilot Programs in China. Energy Policy 96, 688–699. doi:10.1016/j.enpol.2016.06.015
Naser, H. (2016). Estimating and Forecasting the Real Prices of Crude Oil: A Data Rich Model Using a Dynamic Model Averaging (DMA) Approach. Energy Econ. 56, 75–87. doi:10.1016/j.eneco.2016.02.017
Pan, X., Li, M., Wang, M., Chu, J., and Bo, H. (2020). The Effects of Outward Foreign Direct Investment and Reverse Technology Spillover on China's Carbon Productivity. Energy Policy 145, 111730. doi:10.1016/j.enpol.2020.111730
Pang, T., and Duan, M. (2015). Cap Setting and Allowance Allocation in China's Emissions Trading Pilot Programmes: Special Issues and Innovative Solutions. Clim. Policy 16, 815–835. doi:10.1080/14693062.2015.1052956
Surendra, Singh Rajpurohit, and Rajesh, Sharma (2020). Impact of Economic and Financial Development on Carbon Emissions: Evidence from Emerging Asian Economies. Manag. Environ. Qual. doi:10.1108/MEQ-03-2020-0043
Wang, X.-Q., Su, C.-W., Lobonţ, O.-R., Li, H., and Nicoleta-Claudia, M. (2022). Is China's Carbon Trading Market Efficient? Evidence from Emissions Trading Scheme Pilots. Energy 245, 123240. doi:10.1016/j.energy.2022.123240
Wang, Y., Guo, C., Guo, C.-h., Du, C., Chen, X.-j., Jia, L.-q., et al. (2021). Carbon Peak and Carbon Neutrality in China: Goals, Implementation Path, and Prospects. China Geol. 4, 1–27. doi:10.31035/cg2021083
Wang, Y., and Guo, Z. (2018). The Dynamic Spillover between Carbon and Energy Markets: New Evidence. Energy 149, 24–33. doi:10.1016/j.energy.2018.01.145
Wu, M., Li, K. X., Xiao, Y., and Yuen, K. F. (2022). Carbon Emission Trading Scheme in the Shipping Sector: Drivers, Challenges, and Impacts. Mar. Policy 138, 104989. doi:10.1016/j.marpol.2022.104989
Xu, Y., and Salem, S. (2021). Explosive Behaviors in Chinese Carbon Markets: Are There Price Bubbles in Eight Pilots? Renew. Sustain. Energy Rev. 145, 111089. doi:10.1016/j.rser.2021.111089
Zhang, D., Karplus, V. J., Cassisa, C., and Zhang, X. (2014). Emissions Trading in China: Progress and Prospects. Energy Policy 75, 9–16. doi:10.1016/j.enpol.2014.01.022
Zhao, L.-T., Miao, J., Qu, S., and Chen, X.-H. (2021). A Multi-Factor Integrated Model for Carbon Price Forecasting: Market Interaction Promoting Carbon Emission Reduction. Sci. Total Environ. 796, 149110. doi:10.1016/j.scitotenv.2021.149110
Zhao, X.-g., Jiang, G.-w., Nie, D., and Chen, H. (2016). How to Improve the Market Efficiency of Carbon Trading: A Perspective of China. Renew. Sustain. Energy Rev. 59, 1229–1245. doi:10.1016/j.rser.2016.01.052
Zhou, K., and Li, Y. (2019). Influencing Factors and Fluctuation Characteristics of China's Carbon Emission Trading Price. Phys. A Stat. Mech. its Appl. 524, 459–474. doi:10.1016/j.physa.2019.04.249
Zhu, B., Tang, J., and Wang, P. (2021). Examining the Risk of China's Pilot Carbon Markets: A Novel Integrated Approach. J. Clean. Prod. 328, 129408. doi:10.1016/j.jclepro.2021.129408
Keywords: carbon market, carbon emissions trading price, mechanism analysis, BP neural network model, price forecast
Citation: Du Y, Chen K, Chen S and Yin K (2022) Prediction of Carbon Emissions Trading Price in Fujian Province: Based on BP Neural Network Model. Front. Energy Res. 10:939602. doi: 10.3389/fenrg.2022.939602
Received: 09 May 2022; Accepted: 20 June 2022;
Published: 08 July 2022.
Edited by:
Yongping Sun, Huazhong University of Science and Technology, ChinaCopyright © 2022 Du, Chen, Chen and Yin. This is an open-access article distributed under the terms of the Creative Commons Attribution License (CC BY). The use, distribution or reproduction in other forums is permitted, provided the original author(s) and the copyright owner(s) are credited and that the original publication in this journal is cited, in accordance with accepted academic practice. No use, distribution or reproduction is permitted which does not comply with these terms.
*Correspondence: Kai Yin, yinkai@stu.xmu.edu.cn