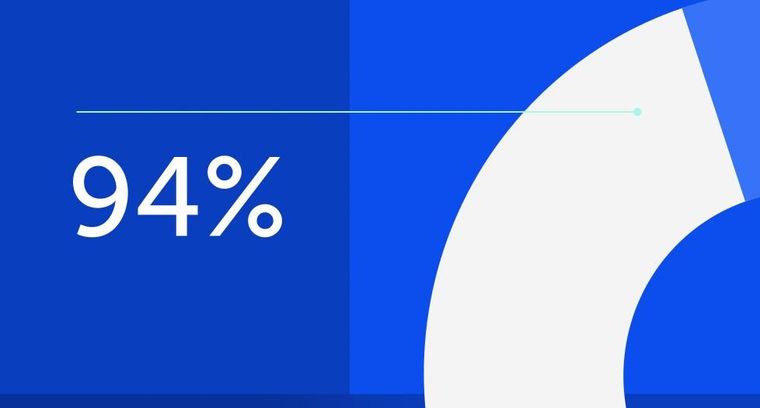
94% of researchers rate our articles as excellent or good
Learn more about the work of our research integrity team to safeguard the quality of each article we publish.
Find out more
ORIGINAL RESEARCH article
Front. Energy Res., 12 July 2022
Sec. Process and Energy Systems Engineering
Volume 10 - 2022 | https://doi.org/10.3389/fenrg.2022.929693
This article is part of the Research TopicAdvanced Technologies for Planning and Operation of Prosumer Energy SystemsView all 111 articles
Energy use differences between day and night have been a key point in the efficient use of utilities. The battery energy storage system (BESS) is an attractive solution to level the grid load and has been introduced independently into many communities, although with high costs. Battery sharing presents the possibility of integrating independent energy storage systems to save money and improve energy use. Battery sharing highlights the interactions between a smart grid, smart buildings, and distributed energy storage to produce better energy management practices. In this work, we provide an analysis of battery sharing by establishing a coordinated control model for a distributed battery system. In our case study design, we selected 39 buildings with different capacities of energy storage systems as a battery-sharing community to optimize sharing schedules and the load-leveling performance. The results indicate that battery sharing could achieve a 13.2% reduction in building battery capacity compared with independent operation. We further investigate the impact of a building’s load profile patterns on the battery capacity in a battery-sharing community. It is economical to introduce a larger capacity battery system into buildings with no closing days and higher electricity consumption throughout the year. The optimal BESS capacity in commercial buildings depends on the lowest daytime power consumption. Commercial buildings with closing days have limitations regarding BESS deployment. On closing days, buildings can only use BESS by sharing. Buildings with two closing days a week lose 14.3% more energy than those with one closing day.
Buildings are now responsible for around 55% of total electricity use (Global, 2020). Electricity consumption in the building sector shows irregular characteristics and is reflected in two main aspects. The first aspect is the valleys of low energy demand and low prices. Conversely, periods with high energy demand create peaks or ceilings in the load profile (Mehrjerdi, 2019). This irregularity in consumption leads to inefficiencies in energy supply as well as uncertainty (because conventional electricity suppliers were designed and created for average electricity demand rather than maximum demand). Compounding this situation, energy consumption in the building sector continues to grow due to population growth and increasing per capita income (Al Shawa, 2022). In Japan, after the Fukushima Crisis in 2011 (Li et al., 2018), the safety of nuclear energy has been seriously questioned; thus, Japan remains heavily dependent on imported fossil fuels that provided 88% of the total primary energy supply (TPES) in 2019 (Cozzi et al., 2020; Global, 2020). Large-scale power outages and year-round electricity bill hikes are also plaguing Japan due to insufficient conventional power-generation capacity and changes to the international situation. As a result, Japan is urgently upgrading its energy system. Integrating energy storage systems (ESSs) into the power system is one of the solutions being proposed to improve the grid’s reliability and performance (Child et al., 2018; Zhang et al., 2018). Distribution networks can utilize ESSs for a number of grid applications, including mitigating renewable resource uncertainties (Zhao et al., 2015), microgrid applications (Awad et al., 2015; Comodi et al., 2015), and risk mitigation in the electricity market (Zheng et al., 2013). Another significant benefit on the distribution side is that ESSs can contribute in the time shifting of energy outputs (Wong et al., 2019); for example, excess generated energy is stored energy at off-peak or low-price periods and the stored energy is dispatched during high-demand, high-price, or low-generation periods. This method also smooths the load pattern by decreasing the on-peak and increasing the off-peak loads in a day, known as load leveling (Mehrjerdi, 2019). By installing large-scale electricity storage facilities, planners would need to build only sufficient generating capacity to meet the average rather than the peak electrical demand (Van der Linden, 2006; Wu et al., 2018). Consequently, ESSs can be a critical element in helping to regulate a city’s peak demand by implementing load leveling. Among the various available ESS technology types, the battery energy storage system (BESS) has attracted considerable attention with clear advantages, such as fast response, controllability, and geographical independence (Divya and Østergaard, 2009; Dunn et al., 2011; Mahlia et al., 2014; Christiansen, 2015).
In spite of the fact that the use of BESSs for load leveling can bring significant economic advantages, the high initial investment remains an issue (Sparacino, 2012). It is therefore crucial that BESSs are optimally sized. The application of BESSs for load leveling can be broadly categorized as centralized battery storage and distributed battery storage. The majority of existing studies propose optimal manipulation and sizing for BESSs. Some researchers have examined the optimal policy for charging and discharging power based on two different optimization objectives (Lu et al., 2014). The first is to minimize the difference between the peak and valley demand, and the second is to minimize the daily variance in load. The storage devices have been used to reduce the peak of the load profile and therefore lessen the planning and operational costs. The allocation of the ESS has been incorporated into the network planning problem (Saboori et al., 2015a). A mixed integer nonlinear programming (MINLP) model was proposed using a particle swarm optimization algorithm (PSO) (Saboori et al., 2015b). As a result of the simulation, both the cost and technical performance of the network were improved. To increase the reliability of the distribution network, Saboori et al. (2015b) proposed as an investment plan for ESSs. By using the energy not supplied (ENS) index, the level of system reliability is elevated and system failures are minimized through optimal ESS planning. To perform load leveling and improve voltage curves in networks, a bi-objective optimization model was proposed by Mehrjerdi(2019). For economic assessment, Trivedi et al. (2020) proposed the stochastic cost–benefit analysis framework for allocating centralized ESSs to achieve load leveling in networks. An algorithm was presented to solve the issues of load leveling and loss minimization in networks impacted by temporary service restoration activities but without considering optimal allocation (Duerr et al., 2020). Jankowiak et al. (2020) introduced five indexes to evaluate the technical performances of load peak shaving for a test house in Northern Ireland but did not consider optimal operation. In addition, mobile BESS technology can provide services and economic benefits by connecting to the grid (such as a vehicle-to-grid system). However, some researchers have pointed out that the increased integration of electric vehicles is expected to have a negative impact on power quality, as well as incurring investment costs for microgrids. Hence, a decentralized energy management system, based on multiagent systems, has been developed for the efficient charging of electric vehicles, achieving approximately 17% peak load reduction and 29% load variance reduction (Boglou et al., 2022).
The various works mentioned here discuss strategies for the placement of BESSs to improve the load-leveling performance of the building sector and propose solutions in terms of optimal location and size. Most importantly, previous research has demonstrated the feasibility of BESS participation in grid management in terms of economics. As the prices of centralized or distributed battery systems (explained in detail in Section 3.3) decrease, they are expected to become an attractive application for the building sector. Centralized energy storage not only requires operational and size optimization considerations but also requires battery siting and distribution system upgrade considerations due to large transient branch circuit current changes over long distances. The conventional designs of distributed systems are based on single-building energy allocation for sizing the distributed batteries, so they neglect the interaction (that we call “energy sharing” in this article) between the smart grid, smart buildings, and distributed energy storage to achieve better energy management practices.
With the rapid growth of the sharing economy around the world, a new proprietary idea for community-centric sharing has recently emerged in the energy market (Roberts et al., 2019; Kang et al., 2022). Unlike traditional individually owned BESSs and large utility-level BESSs, customers in a community can provide optimal energy management services through energy sharing (for example, using remaining power to match the power needs in the same community) and storage sharing (for example, using the battery to take remaining power to or out of the building community) (Huang et al., 2020). A peer-to-peer (P2P) energy-sharing paradigm involving hybrid solar-wind renewable energy systems, battery storage, and grid-connected commercial prosumers (a high-rise office and hotel) has been proposed (Zheng et al., 2021). Zheng et al. (2021) found that the proposed P2P sharing operation with storage sharing can promote self-consumption from 0.591 to 0.795 and reduce the net cost. Henni et al. (2021) established a shared economy model that enabled residential communities to share solar power generation and storage capacity. The model saves an average of €615 per year compared to operating alone. A P2P energy trading framework was proposed by He et al. (2021), enabling distributed photovoltaic (PV) consumers and prosumers to participate in a community sharing market created by a stakeholder. Dai and Charkhgard (2018) proposed a bi-objective mixed-integer linear programming (MINLP) approach for managing clusters of buildings equipped with shared electrical energy storage. Therefore, the uneconomical use case for batteries may become profitable when individual EES assets are aggregated into a large portfolio to provide grid-scale ancillary services (Rappaport and Miles, 2017).
Currently, large-scale batteries are mainly used for load leveling in electricity generating plants by supplying power. The power generated by the electricity suppliers at times of low demand (i.e. at dawn or other off-peak hours) or during periods of overgeneration is charged to the battery and discharged to buildings during times of high need and electricity shortage (i.e. peak hours). Economic viability is the major critical element in large battery implementations because of the high initial costs. However, the utilization rate of large BESSs for load leveling can be low due to fluctuating demand. In addition, large BESSs also have high transmission loss due to size and distance limitations. Conversely, the ability to perform energy arbitrage is the biggest motivation for individual users to install BESSs. Therefore, through energy sharing, private BESSs can participate in load leveling to reduce the peak stress of the power supply, saving costs for peak power generators. Electricity suppliers are willing to pay a fee to cooperate with individuals by sharing information and optimizing energy use. Stakeholder-based information exchange is essential to enable energy sharing between electricity suppliers and buildings where load leveling is performed. Hence, a wide-area monitoring system (WAMS) based on real-time measurement and monitoring is becoming a key solution for online stability, situational awareness, and grid planning. The possibility of monitoring and controlling the operation of the whole power system to achieve real-time, dynamic power control has been proven (Karavas et al., 2021). Mekikis et al. (2016) studied the performance of communication technology in dense networks with wireless energy harvesting (WEH)-enabled sensor nodes. Li et al. (2021) proposed an information-sharing strategy based on linked data for the improved management of net-zero communities. This strategy systematically integrates stakeholders’ engagement by using energy performance indicators as information carriers and linked data as engagement channels.
With the development and application of technologies such as blockchain and the Internet of Things (IoT), the outstanding flexibility and rapid response of BESSs has been proven. With the increasing impact of distributed energy on the grid, BESSs and the sharing strategy have gradually become the most effective means to improve distributed energy use in the community. Most of the literature mentioned in Section 1.2 focuses on this area. Co-investment by producers and consumers can effectively reduce energy costs, avoid unnecessary investments, and increase BESS use. This trading model enhances community resilience by reducing the community’s reliance on the main grid and increasing the ability to participate in demand response (DR) to achieve better load leveling. Many studies have also discussed the role of appropriate pricing models in sharing (through the auction model, blockchain, and bilateral contracts) (Wang et al., 2021), as this directly affects the incentives of P2P participants for participating in energy sharing. However, there is a lack of understanding of the impact of sharing strategies on battery sizing across multiple buildings (especially non-residential buildings), and it is unclear whether community or grid-level battery sharing will provide the best solution for load leveling performance. To address the knowledge gaps identified above, we aim to achieve load leveling by grouping individual BESSs based on the information sharing and energy sharing strategy of linked data.
The main achievements of this work are as follows:
• We develop an energy-sharing framework between the distribution network and the individual batteries for the allocation of distributed ESSs for community load-leveling applications.
• Representative load profiles (RLPs) for non-residential buildings are extracted by clustering and feature analysis methods, the optimal deployment of BESSs in the RLP with sharing framework are discussed, and the results provide promising insights into battery sharing.
• We propose a generic sharing model that takes into account the interaction between various hierarchies in the city, and the application of BESSs is expanded from the individual building to the community level and finally to the city, providing a basis for efficient energy sharing for city hierarchies in the future.
The remainder of this article is organized as follows. The basic idea of cloud-based battery sharing is presented in Section 2. The general process and proposed methodology are presented in Section 3. The results are presented and discussed in Section 4. Future directions for BESSs are discussed in Section 5, and the conclusions are presented in Section 6.
The proposed energy-sharing network allows potential users to benefit from operating the BESS and to participate in energy trading. Real-time energy monitoring and physiographic data from multiple perspectives will help to build a database in the cloud-based battery-sharing community (Supplementary Figure S1). All energy participants, including power producers and customers and all energy components (including distributed generation systems and consumers) can be interlinked in a decentralized battery system within a community to influence each component.
Raw power demand data are collected via meters from a smart community in Japan. A 1-h time-step is used for monitoring the active power and reactive power. In this study, only active power is considered. Raw electricity demand data are provided in a single-column format, with each row corresponding to a selected time range. Data formatting is performed by extracting the daily load profile (DLP) of the annual data set. It is challenging for utilities to analyze a large number of DLPs from different customers, especially when the volume of customer data is very large or when the study time is very long. A common solution is to assign a representative load profile (RLP) to each customer. The RLP is usually calculated by averaging DLPs over a period of time. Therefore, each customer is represented by one RLP rather than many DLPs. There are various clustering algorithm methods, of which three of the most common are self-organizing maps (SOMs) (Zhang et al., 2011), hierarchical clustering (Chicco, 2012), and K-means (Richard et al., 2017). Clustering algorithms always assign DLPs with the same consumption pattern to the same group. Commercial buildings, for example, do not undergo significant changes in DLP due to stable commercial activities. The K-means algorithm stands out for its high-quality clustering effect, strong interpretability, fast convergence, and other advantages (Rajabi et al., 2019; Bourdeau et al., 2021) when applied to non-residential building performance analysis (Miller et al., 2018). Conversely, the application of clustering algorithms is a challenge when dealing with highly variable data sets (e.g. residential customers, who do not use specific appliances or electrical equipment at the same time every day). At this point, if clustering is performed using the K-means algorithm and distance metrics such as Euclidean distance, a customer’s DLP may be assigned to many different clusters. Therefore, the SOM approach has been proven to be the most suitable clustering algorithm for residential customers (McLoughlin et al., 2015). The strategy of hierarchical clustering is to first treat each object as a cluster, and then merge these atomic clusters into larger clusters until all objects are in a cluster or some end condition is satisfied (Wang et al., 2020). Once two clusters are merged, they are not undone, so that the computational storage is costly. Therefore, its disadvantages are obvious: 1) the computational complexity is too high; and 2) the singular values can have a significant impact (e.g. caused by maintenance or temporary closures). As a result, we adopt the K-means clustering algorithm to reduce the calculation sophistication while retaining high accuracy in this study. The specific steps are as follows:
• First, the annual data set is processed using principal component analysis (PCA) to extract the most important features of each day. Feature extraction finds meaningful information within a confusing data set. In addition, feature extraction can reduce complex data sets to a lower dimension while retaining the variation to eliminate noise and reveal hidden structures (Yilmaz et al., 2019).
• After reconstructing the total load curves using PCA, the daily load-demand curves are clustered into independent nonoverlapping clusters (Richard et al., 2017).
• The feature-based DLPs are used as inputs to search the optimal set of BESS deployments. Meanwhile, feature extraction is used to provide the physical meaning for identifying the results (Supplementary Figure S2).
In the past few decades, BESSs have become significantly more attractive because of the rapidly decreasing price. Additionally, BESSs have the ability to transform the production–consumption energy paradigm into a new production–storage–consumption paradigm. In our case study design, we select sodium–sulfur (NaS) technology as the electricity power storage tool because of its high energy efficiencies (Divya and Østergaard, 2009). The state of the battery changes during the input and output of power. The state of charge (SOC) and power rating of the battery are used to develop a realistic model of the storage system. During the charging and discharging process, the SOCs of the battery can be described by Eqs. 1, 2, respectively, as:
where SOC(t) is the state of charge of the BESS, and
where
where
Load-transfer management is the main work of load leveling. The purpose is to optimize the allocation and rational use of power resources, improve the economic operation of the power grid by changing the time and method of power consumption, increase facility use, and simultaneously benefit customers.
The community’s buildings are equipped with a single centralized battery, which is usually mounted on the outside of the structure. The capacity and power of the aggregated BESS are defined by the load demand of all buildings in the community. The aggregated BESS is charged when the load demand is low (e.g. at night) and discharged when the load demand is high to relieve the instantaneous supply pressure on the power plant and to improve the energy efficiency. This is a conventional application of a large BESS on the distribution network side (Trivedi et al., 2020). Because all processes take place outside the building, the overall peak-shaving performance is not affected by the individual building. Hence, there is no need to consider the load of a single consumer; it easily reaches a stable state both in on-peak and off-peak periods (see the red line in Supplementary Figure S3A).
Each building has its own individual battery, and energy exchange is based on single building energy allocation. The common solution is to deploy the BESS inside the building to achieve load leveling individually according to load demand. For example, Mair et al. (2021) took a demand-driven approach to determine the residential battery capacity of individual household demand both for load smoothing and peak shaving. The results show that the BESS can successfully achieve load smoothing and keep the cell size within the design specification. However, due to the complexity of building types (e.g. shopping malls and office buildings have highly variable electricity consumption habits), when the number of buildings in a community reaches dozens or even hundreds of buildings, such a solution will become inefficient. When each BESS is installed individually, there is no interchange of information between them and the whole system becomes unpredictable. Hence, although it is possible to maintain a smooth load profile at an individual level, the final balance performance is difficult to predict and control due to the involvement of multiple users (Supplementary Figure S3B). The innovation introduced by Mair et al. (2021) was that they also compared the battery capacity required for a single house with the battery capacity required for the total needs of a group of households (20 households). Aggregation reduces battery demand per house by 50% for load smoothing and 90% for peaking. This means that allowing battery resources to be shared among users may result in smaller battery deployments per house to achieve the same level of load smoothing (Bayliss et al., 2012). Consequently, under the cloud-based energy sharing framework, the individual BESS has the capability to manage the energy of the other buildings in the community. The relationship between the distribution network and the individual batteries is developed so that the individual consumer’s management system can adjust the charging and discharging power by means of the peak information of the distribution network. However, the disadvantage is that the capacity and charging/discharging power of the distributed battery are limited by its own load demand. Therefore, when the load demand is compensated by its own battery at a certain moment, the remaining power is supplied by the other buildings in the community (battery storage sharing).
The energy system configuration may vary depending on the grid and the components of the energy flow. As an example, the following equation can be used to represent the load balance between components of the system:
where
• Charging state: The individual BESS is in a charging state during the night when electricity is at the off-peak price. The charging power of the private BESS can be defined as follows:
• Discharging state: The BESS is in a discharging state during the day, when the building has insufficient power (i.e.
Currently, the definition of load leveling is not clear. The purpose of load leveling is to obtain a more stabilized and balanced load curve, as well as to avoid frequent transitions between peaks and troughs to reduce the peak-supply pressure on the electricity supplier. Therefore, load leveling can be interpreted as a small fluctuation of electricity consumption data. Xu et al. (2017) proposed using the load standard deviation (LSD) to measure the fluctuation statistically. The LSD is defined as:
where
Energy sharing requires reliance on the distribution network to share surplus battery capacity to other buildings within the community. Generally, a smaller power network will incur relatively high transmission losses because even though the electricity travels only a few miles, the low-voltage distribution lines cause high losses in the community-level network. Meanwhile, the different operation of individual batteries due to their locations and capacities will cause different energy losses. Therefore, energy loss cannot be ignored in shared energy communities. Additional losses are incurred during the charging or discharging of the BESS. The total energy loss can be described as:
where
The net present value (NPV) of an investment is the difference between the present value of cash inflows and the present value of cash outflows over time. The NPV is a tool used in capital budgeting and investment planning to determine whether a planned investment or project will be profitable. Ignoring the operation and maintenance costs, the NPV of the BESS can be determined as:
where NPVBESS donates the net present value of the BESS, Pr(t) is the electricity price at time t, i is the discount rate, y is the lifecycle of the project, and Cinv is the investment price, which includes the inverters, equipment costs, installation costs, and initial conservation costs.
This section describes the design and optimization methods of a distributed BESS within an energy sharing community. The first step is to obtain the electrical load profile of the entire community by evaluating each building. In the second step, the battery capacity at the aggregation level is optimized using a genetic algorithm (GA) using the aggregation level power/demand as input parameters, with the goal of maximizing the load leveling performance of the community. The objective function of this step can be described as:
The output of this step is considered to be the minimum capacity required to achieve the required energy performance (load leveling) for the entire community. In step 3, nonlinear programming (NLP) is used to optimize the capacity of the distributed batteries installed in each building to minimize storage sharing (i.e. power exchange with other batteries) and thus reduce the associated energy losses. The objective function of NLP is represented by Eq. 17.
A step-by-step method is described below. Supplementary Figure S4 presents a flow chart depicting the method.
The first step is the data fetching. Features of the BESS that are collected consist of the design range of the BESS dimensions (kWh), module dimensions of the BESS (kWh), module dimensions of the power rating (kW), and the planning optimization objective function. The parameters necessary for the GA are also imported in this step.
The load-demand curves for a standard year are approached using PCA and clustered using the K-means method. After alteration according to the chronology, representative load-demand profiles are computed.
An initial population of individuals is the starting point of the process. Each individual is represented using an alphabetical string (string of 1 and 0 s). Every individual represents the capacity of the BESS. The parent groups are initialized stochastically in this step.
The fitness function determines how fit an individual is (the fitness function is the LSD). MATLAB’s fmincon solver is used to determine the optimal BESS operation for each population to correspond with the minimum LSD. This step is performed for each individual member of the population in turn and results in a fitness score. Based on the fitness score of an individual, we determine whether it will be selected for breeding.
Each individual progeny member of a population is evaluated for fitness in step 4 of the evaluation of a generation. Selective breeding, cross-breeding, and mutation are used within the population to generate the offspring population. An elite algorithm is used to blend the parent and offspring populations together to form the next generation. Step 6 should be followed if the current generation is the final generation; otherwise, update the number of generations, reset the year to 1, and go to step 4.
An optimal capacity of the aggregated battery, which has the minimum LSD, is the result of the GA search.
In this step, GA is used to optimize the capacity of distributed batteries (kWh) installed in individual buildings based on the aggregated battery capacity, which aims to minimize load limitations.
In the eighth step, the battery capacity of all batteries to be tested is calculated. We can use NLP and combine other factors to assign the battery capacity.
Supplementary Table S1 provides an overview of the input variables used in the study; the investment price of the BESS is 25,000 Yen/kWh. The discount rate is set to 4.5% by the Bank of Fukuoka. The power rating of each BESS is limited to 16% of its capacity. The upper limit of the discharging depth is 90%. The power transmission loss rate is set as 8%. The parameters of the GA are also summarized in Supplementary Table S1.
We collected the load profile data from a typical commercial community in Japan. The measurement occurred from April 2013 to March 2014. The data set contains annual load data for 39 buildings that form a load matrix (365 days × 24 data points/day). The 39 buildings provide common activities of a commercial community, including a supermarket, hotel, factory, convenience store, gas station, shopping mall, stadium, exhibition hall, clinic, station, service center, office, and restaurant. The daily electricity consumption curves of the whole community are shown in Supplementary Figure S5. Due to the chronological nature of commercial activities, the electricity consumption is much higher in the daytime than in the nighttime. The peak annual electricity consumption is concentrated in the summer (June, July, and August).
Supplementary Figure S6 shows the curve of load-leveling performance with BESS capacity at the aggregation level. In the initial part of the curve, the LSD decreases rapidly as the aggregated capacity increases, while near the lowest point (98,120 kWh/3,960) the rate of decrease slows. Then, with increasing BESS size, the load-leveling performance worsens. Energy losses are plotted in green in Supplementary Figure S6. Energy losses increase with increasing BESS sizes because the frequency of energy exchanges is increasing. Therefore, the optimal BESS aggregate capacity is 98,120 kWh.
We select the four most representative days by season across the whole year to verify the validity of the BESS for load leveling. The load curve for the whole community with and without BESS operation is plotted in Supplementary Figure S7. The battery capacity is the optimal size of 98,120 kWh as discussed in Section 4.2. The SOC of the virtual aggregated BESS is also shown in Supplementary Figure S7 (green line), which represents the comprehensive operation capability of the distributed batteries. A large fluctuation in the community load without BESS can be seen in Supplementary Figure S7 (black line). The peak demand is 44,300 kWh at noon while the valley demand is 13,123 kWh at 4:00 a.m. in summer. The difference between the peak and valley is more than 30,000 kWh. Optimal BESS operation results in the BESS charging from 0 to 8 a.m. and discharging from 10 a.m. to 8 p.m. The green part represents charging, and the blue part represents discharging. Therefore, the difference between the peak and valley is greatly reduced and the peak becomes significantly flatter (red line). In winter, although the peak demand is 28,390 kW, the optimal BESS operation also reduces the difference between the peak and the valley. Compared with the case of no BESS, the peak demand is reduced by 26.6%. These results show that optimal BESS operation can effectively implement load leveling.
Supplementary Table S2 compares the results and performances of the battery-sharing design method and the individual design method. From the perspective of load-leveling performance, the LSD value of the individual design is 4,113, while that of the battery-sharing method is 3,960. Compared with the situation without BESS (Scenario 1), these values are reduced by more than half, indicating that both design methods offer good performance. The aggregated capacity of the distributed batteries is 113,056 kWh under the individual design and operation scenario (Scenario 2). The aggregated capacity of the distributed batteries to achieve better load-leveling performance is 98,120 kWh in Scenario 3. The aggregated battery capacity is significantly reduced (i.e. a 13.2% decrease) compared with Scenario 2. Correspondingly, the initial investment in the BESS also is significantly reduced in Scenario 3.
Due to the energy-sharing framework benefits, private BESSs manage all energy flows within the community. The limitations of deploying batteries inside buildings are greatly reduced. The surplus capacity can be consumed inside other buildings, providing significant flexibility in the BESS deployment. However, the variety of curves for individual buildings shows the different features of BESS operation. The flexibility of BESS deployment in a single building is presented in Supplementary Figure S8 as the optimal range of BESS capacities. BESS deployment flexibility varies greatly between buildings. We selected three scenarios that have the same BESS capacity at the aggregation level (98,120 kWh) but with different capacities for each building. It is noted that in the case of Scenario a, all the buildings deploy the batteries under the optimal sizes. The energy flows of the three scenarios are plotted in Supplementary Figure S9. In the case of Scenario a, all energy flows of batteries occur within the individual buildings so there is no energy loss to exchange between buildings. In the case of Scenario b, some buildings (such as buildings 1, 2, 21, and 22) first discharge within the buildings, but they still have remaining capacity to share with other building to achieve the load-leveling objective. Therefore, 333,697 kWh of energy losses occurred in the energy-sharing process. In the case of Scenario c, there are much higher remaining battery capacities in the buildings so the energy losses also are much higher.
A comparison of Scenarios a, b, and c indicates that different-sized buildings will have different energy losses. Scenario a achieves 0 kWh energy losses, because all buildings consume their own batteries’ charged power so that there is no energy exchange between buildings. In the cases of Scenarios b and c, surplus power always remains charged in the batteries. Some buildings do not require electric power for much time and have installed oversized batteries. Therefore, building load features and battery sizes are highly dependent. Due to the characteristics and operations of the BESS, power from the grid is charged to the battery at night and discharged to the building in the daytime. Supplementary Figure S10 illustrates the relationship between daytime electricity consumption (from 10:00 a.m. to 10:00 p.m. in this case) and optimal battery capacity for all buildings. Daytime electricity consumption is shown as a box plot, in which the dots represent the four most representative sets of data points, and the red boxes are 95% confidence intervals. The battery capacity of the building is always below the minimum value of daytime electricity consumption; when the capacity is greater than the minimum value, the battery capacity remains surplus power. The optimal capacity depends on the lowest daytime electricity consumption.
The optimal capacity depends on the lowest daytime electricity consumption. Commercial activities are the determinants of electricity consumption in commercial buildings. On working days, buildings have high electricity demand to cope with busy commercial activities (e.g. air conditioning, lights, operation of factory production equipment), but on closing days, buildings have little demand for electricity consumption and require only a small quantity of power to keep equipment on standby (e.g. network, security, and some storage equipment). In Supplementary Figure S11, buildings 2, 8, and 22 have relatively high consumption, but the optimal BESS capacities are at a low level. Therefore, rest days can have a significant impact on battery sharing as well as battery capacity in a shared framework. We selected four buildings with similar annual electricity consumption as a case study. Two of the buildings (B9 and B11) have no closing days, and electricity consumption is relatively stable throughout the year. One building (B35) closes on Sunday. One building (B22) closes on Saturdays and Sundays. The daily electricity consumption curves are plotted in Supplementary Figure S11. There is a clear difference between business days and closing days for buildings B22 and B35.
Batteries with a capacity of 2,000 kWh were installed in all four buildings. The operation of the batteries between buildings can be clearly seen in Supplementary Figure S12. On weekdays, all buildings have high power consumption during the day, so all batteries have to be discharged within their own buildings and there is no energy exchange. On Saturdays, the batteries in B22 will be shared between B9 and B11 because B22 is closed and there is almost no power consumption during the daytime. On Sundays, the remaining battery capacity in B22 and B35 will be shared between B9 and B1, as B22 and B35 are closed. Buildings with two closing days in 1 week contribute 14.3% more energy loss than those with one day.
Based on the literature review of battery sharing and on our work, the effectiveness of BESS for better energy planning at the individual as well as community level can be demonstrated. However, when moving up to the city level of service recipients, there is still room for improvement in data utilization, interoperability, and the integrated management of the technologies currently used in BESS applications. This section will discuss the upgrading of BESS service targets and future directions for application. It is well known that cities are pagoda-shaped, from many single buildings to fewer communities to the city as a whole, and the higher levels have exponentially more individuals or systems involved. The current BESS applications are used only for specific buildings or groups within cities and do not take into account the city as a whole. As a result, we have taken an important step forward in our work. We have expanded the application of BESS from individual buildings to the community level and are connecting customers scattered across a community through battery sharing. Accordingly, the BESS will improve the connectivity between systems to fully integrate the various factors that affect energy generation and consumption in cities. BESS application strategy still faces some challenges in city-level interaction. First, the BESS requires the analysis, utilization, and interaction of data. Therefore, energy data need to be collected and shared at all levels of the city through an integrated IoT. Geospatial information and automation-based technologies need to be shared as well in order to reflect the changing spatial and environmental characteristics of the city.
In this work, we have proposed an energy-sharing framework to optimize the control strategy and allocation method for a distributed battery system. The framework achieves the optimization of load leveling by investigating interactions between buildings, the BESS, and the distribution network. First, we have proposed the advantages of energy sharing to analyze the differences between centralized and distributed energy storage in dealing with load-leveling problems. We have established a strategy to reduce the peak-to-valley difference for the battery management system. Then, we have capitalized on the advantages of centralized energy storage to propose a size allocation method for distributed energy storage to optimize the load-leveling performance and reduce the battery capacity at the aggregation level. The proposed distributed battery system was modeled in a typical community with 39 buildings in Japan to analyze the load-leveling performance.
The major conclusions of this study are as follows:
1) The optimal solution obtained with the energy-sharing structure performs load leveling effectively. It achieved a peak-shaving rate of 26.6%.
2) Compared with individual operation, the energy-sharing model achieves better load-leveling performance for the public grid, while it reduces the battery capacity by 13.2% at the aggregation level.
3) Energy sharing is a generic framework for decomposing the optimal BESS allocation problem into individual optimal BESS sizes within buildings. This approach obtains optimal solutions by reducing unnecessary energy exchange between buildings. Conversely, the approach also obtains near-optimal solutions for the whole system (with energy losses) due to the different investment budgets and preferences for the buildings. This gives flexibility in the placement of the BESS, and investors can choose a solution according to their budget, other available investment opportunities, and their preferences.
4) Commercial buildings have great potential for BESS placement because of their fixed power-consumption patterns. The optimal BESS capacity in commercial buildings depends on the lowest daytime power consumption. Commercial buildings with closing days have limitations on BESS deployment. On closing days, buildings can only use a BESS by sharing. Buildings with two closing days a week lose 14.3% more energy than those with one day.
5) The proposed method highlights the possibility of cooperation between consumers. It provides a blueprint for the implementation of regional battery sharing.
Many buildings are now producing their own electricity from local PV panels and selling it to the grid. However, PV penetration and its intermittent output place additional management burdens on the public grid. There is no consideration of the impact of PV penetration or power trading on the design of individual BESSs in this study. Thus, future work should consider the techno-economic case of a PV–battery system for building-energy-sharing communities to identify interactions between coordinated ESSs and a smart grid.
The original contributions presented in the study are included in the Supplementary Materials; further inquiries can be directed to the corresponding author.
YL: methodology, formal analysis, visualization and writing—original draft, and writing—review and editing; YW: software, writing—original draft, and writing—review and editing; HF: writing—review and editing; WG: writing—review and editing and resources; FQ: methodology and writing—review and editing.
The authors declare that the research was conducted in the absence of any commercial or financial relationships that could be construed as a potential conflict of interest.
All claims expressed in this article are solely those of the authors and do not necessarily represent those of their affiliated organizations, or those of the publisher, the editors and the reviewers. Any product that may be evaluated in this article, or claim that may be made by its manufacturer, is not guaranteed or endorsed by the publisher.
The Supplementary Material for this article can be found online at: https://www.frontiersin.org/articles/10.3389/fenrg.2022.929693/full#supplementary-material
Al Shawa, B. (2022). The Ability of Building Stock Energy Models (BSEMs) to Facilitate the Sector's Climate Change Target in the Face of Socioeconomic Uncertainties: A Review. Energy Build. 254, 111634. doi:10.1016/j.enbuild.2021.111634
Awad, A. S., El-Fouly, T. H., and Salama, M. M. (2015). Optimal ESS Allocation for Benefit Maximization in Distribution Networks. IEEE Trans. Smart Grid 8 (4), 1668–1678. doi:10.1109/tsg.2015.2499264
Bayliss, C. R., Bayliss, C., and Hardy, B. (2012). Transmission and Distribution Electrical Engineering. Elsevier.
Boglou, V., Karavas, C. S., Karlis, A., and Arvanitis, K. (2022). An Intelligent Decentralized Energy Management Strategy for the Optimal Electric Vehicles' Charging in Low-Voltage Islanded Microgrids. Intl J Energy Res. 46 (3), 2988–3016. doi:10.1002/er.7358
Bourdeau, M., Basset, P., Beauchêne, S., Da Silva, D., Guiot, T., Werner, D., et al. (2021). Classification of Daily Electric Load Profiles of Non-residential Buildings. Energy Build. 233, 110670. doi:10.1016/j.enbuild.2020.110670
Chicco, G. (2012). Overview and Performance Assessment of the Clustering Methods for Electrical Load Pattern Grouping. Energy 42 (1), 68–80. doi:10.1016/j.energy.2011.12.031
Child, M., Bogdanov, D., and Breyer, C. (2018). The Role of Storage Technologies for the Transition to a 100% Renewable Energy System in Europe. Energy Procedia 155, 44–60. doi:10.1016/j.egypro.2018.11.067
Christiansen, C., Murray, B., and Conway, G. (2015). Energy Storage Study-Funding and Knowledge Sharing Priorities. Dallas, Texas, US: AECOM.
Comodi, G., Giantomassi, A., Severini, M., Squartini, S., Ferracuti, F., Fonti, A., et al. (2015). Multi-apartment Residential Microgrid with Electrical and Thermal Storage Devices: Experimental Analysis and Simulation of Energy Management Strategies. Appl. Energy 137, 854–866. doi:10.1016/j.apenergy.2014.07.068
Cozzi, L., Gould, T., Bouckart, S., Crow, D., Kim, T., McGlade, C., et al. (2020). World Energy Outlook 2020, 2050. Paris, France: IEA, 1–461.
Dai, R., and Charkhgard, H. (2018). Bi-objective Mixed Integer Linear Programming for Managing Building Clusters with a Shared Electrical Energy Storage. Comput. Operations Res. 96, 173–187. doi:10.1016/j.cor.2018.01.002
Divya, K. C., and Østergaard, J. (2009). Battery Energy Storage Technology for Power Systems-An Overview. Electr. Power Syst. Res. 79 (4), 511–520. doi:10.1016/j.epsr.2008.09.017
Duerr, S., Ababei, C., and Ionel, D. M. (2020). A Case for Using Distributed Energy Storage for Load Balancing and Power Loss Minimization in Distribution Networks. Electr. Power Components Syst. 48 (9-10), 1063–1076. doi:10.1080/15325008.2020.1825556
Dunn, B. K., Haresh, , and Tarascon, J-M. (2011). Electrical Energy Storage for the Grid: A Battery of Choices, Science, 334, (6058) 928–935. Materials for grid energy. doi:10.1126/science.1212741
Global, A. (2020). Global Status Report for Buildings and Construction. Global Alliance for Buildings and Construction.
He, L., Liu, Y., and Zhang, J. (2021). Peer-to-peer Energy Sharing with Battery Storage: Energy Pawn in the Smart Grid. Appl. Energy 297, 117129.
Henni, S., Staudt, P., and Weinhardt, C. (2021). A Sharing Economy for Residential Communities with PV-Coupled Battery Storage: Benefits, Pricing and Participant Matching. Appl. Energy 301, 117351. doi:10.1016/j.apenergy.2021.117351
Huang, P., Lovati, M., Zhang, X., and Bales, C. (2020). A Coordinated Control to Improve Performance for a Building Cluster with Energy Storage, Electric Vehicles, and Energy Sharing Considered. Appl. Energy 268, 114983. doi:10.1016/j.apenergy.2020.114983
Jankowiak, C., Zacharopoulos, A., Brandoni, C., Keatley, P., MacArtain, P., and Hewitt, N. (2020). Assessing the Benefits of Decentralised Residential Batteries for Load Peak Shaving. J. Energy Storage 32, 101779. doi:10.1016/j.est.2020.101779
Kang, H., Jung, S., Lee, M., and Hong, T. (2022). How to Better Share Energy towards a Carbon-Neutral City? A Review on Application Strategies of Battery Energy Storage System in City. Renew. Sustain. Energy Rev. 157, 112113. doi:10.1016/j.rser.2022.112113
Karavas, C.-S. G., Plakas, K. A., Krommydas, K. F., Kurashvili, A. S., Dikaiakos, C. N., and Papaioannou, G. P. (2021). A Review of Wide-Area Monitoring and Damping Control Systems in Europe. IEEE Madr. PowerTech, 1–6.
Kerestes, R. J., Reed, G. F., and Sparacino, A. R. (2012). Economic Analysis of Grid Level Energy Storage for the Application of Load Leveling IEEE Power and Energy Society General Meeting. doi:10.1109/PESGM.2012.6345072
Li, Y., Gao, W., and Ruan, Y. (2018). Performance Investigation of Grid-Connected Residential PV-Battery System Focusing on Enhancing Self-Consumption and Peak Shaving in Kyushu, Japan. Renew. Energy 127, 514–523. doi:10.1016/j.renene.2018.04.074
Li, Y., Hu, S., Hoare, C., O'Donnell, J., García-Castro, R., Vega-Sánchez, S., et al. (2021). An Information Sharing Strategy Based on Linked Data for Net Zero Energy Buildings and Clusters. Automation Constr. 124, 103592. doi:10.1016/j.autcon.2021.103592
Lu, C., Xu, H., Pan, X., and Song, J. (2014). Optimal Sizing and Control of Battery Energy Storage System for Peak Load Shaving. Energies 7 (12), 8396–8410. doi:10.3390/en7128396
Mahlia, T. M. I., Saktisahdan, T. J., Jannifar, A., Hasan, M. H., and Matseelar, H. S. C. (2014). A Review of Available Methods and Development on Energy Storage; Technology Update. Renew. Sustain. Energy Rev. 33, 532–545. doi:10.1016/j.rser.2014.01.068
Mair, J., Suomalainen, K., Eyers, D. M., and Jack, M. W. (2021). Sizing Domestic Batteries for Load Smoothing and Peak Shaving Based on Real-World Demand Data. Energy Build. 247, 111109. doi:10.1016/j.enbuild.2021.111109
McLoughlin, F., Duffy, A., and Conlon, M. (2015). A Clustering Approach to Domestic Electricity Load Profile Characterisation Using Smart Metering Data. Appl. energy 141, 190–199. doi:10.1016/j.apenergy.2014.12.039
Mehrjerdi, H. (2019). Simultaneous Load Leveling and Voltage Profile Improvement in Distribution Networks by Optimal Battery Storage Planning. Energy 181, 916–926. doi:10.1016/j.energy.2019.06.021
Mekikis, P.-V., Antonopoulos, A., Kartsakli, E., Lalos, A. S., Alonso, L., and Verikoukis, C. (2016). Information Exchange in Randomly Deployed Dense WSNs with Wireless Energy Harvesting Capabilities. IEEE Trans. Wirel. Commun. 15 (4), 3008–3018. doi:10.1109/twc.2016.2514419
Miller, C., Nagy, Z., and Schlueter, A. (2018). A Review of Unsupervised Statistical Learning and Visual Analytics Techniques Applied to Performance Analysis of Non-residential Buildings. Renew. Sustain. Energy Rev. 81, 1365–1377. doi:10.1016/j.rser.2017.05.124
Rajabi, A., Eskandari, M., Jabbari Ghadi, M., Ghavidel, S., Li, L., Zhang, J., et al. (2019). A Pattern Recognition Methodology for Analyzing Residential Customers Load Data and Targeting Demand Response Applications. Energy Build. 203, 109455. doi:10.1016/j.enbuild.2019.109455
Rappaport, R. D., and Miles, J. (2017). Cloud Energy Storage for Grid Scale Applications in the UK. Energy Policy 109, 609–622. doi:10.1016/j.enpol.2017.07.044
Richard, M.-A., Fortin, H., Poulin, A., Leduc, M.-A., and Fournier, M. (2017). “Daily Load Profiles Clustering: a Powerful Tool for Demand Side Management in Medium-Sized Industries,” in Proceedings of the ACEEE Summer Study on Energy Efficiency in Industry (Denver, CO, USA, 15–18.
Roberts, M., Bruce, A., and MacGill, I. (2019). Impact of Shared Battery Energy Storage Systems on Photovoltaic Self-Consumption and Electricity Bills in Apart. Appl. Energy 245, 78–95. doi:10.1016/j.apenergy.2019.04.001
Saboori, H., Hemmati, R., and Abbasi, V. (2015a). Multistage Distribution Network Expansion Planning Considering the Emerging Energy Storage Systems. Energy Convers. Manag. 105, 938–945. doi:10.1016/j.enconman.2015.08.055
Saboori, H., Hemmati, R., and Jirdehi, M. A. (2015b). Reliability Improvement in Radial Electrical Distribution Network by Optimal Planning of Energy Storage Systems. Energy 93, 2299–2312. doi:10.1016/j.energy.2015.10.125
Sudworth, J., and Tiley, A. R. (1985). INSULATORS, N. in Sodium Sulfur Battery (Springer Netherlands). E.I.B. Group.
Trivedi, A., Chong Aih, H., and Srinivasan, D. (2020). A Stochastic Cost-Benefit Analysis Framework for Allocating Energy Storage System in Distribution Network for Load Leveling. Appl. Energy 280, 115944. doi:10.1016/j.apenergy.2020.115944
Van der Linden, S. (2006). Bulk Energy Storage Potential in the USA, Current Developments and Future Prospects. Energy 31 (15), 3446–3457. doi:10.1016/j.energy.2006.03.016
Wang, J., Xie, N., Ilea, V., Bovo, C., Xin, H., and Wang, Y. (2021). Cooperative Trading Mechanism and Satisfaction-Based Profit Distribution in a Multi-Energy Community. Front. Energy Res. 9. doi:10.3389/fenrg.2021.723192
Wang, S., Liu, H., Pu, H., and Yang, H. (2020). Spatial Disparity and Hierarchical Cluster Analysis of Final Energy Consumption in China. Energy 197, 117195. doi:10.1016/j.energy.2020.117195
Wong, L. A., Ramachandaramurthy, V. K., Taylor, P., Ekanayake, J. B., Walker, S. L., and Padmanaban, S. (2019). Review on the Optimal Placement, Sizing and Control of an Energy Storage System in the Distribution Network. J. Energy Storage 21, 489–504. doi:10.1016/j.est.2018.12.015
Wu, B., Maleki, A., Pourfayaz, F., and Rosen, M. A. (2018). Optimal Design of Stand-Alone Reverse Osmosis Desalination Driven by a Photovoltaic and Diesel Generator Hybrid System. Sol. Energy 163, 91–103. doi:10.1016/j.solener.2018.01.016
Yilmaz, S., Chambers, J., and Patel, M. K. (2019). Comparison of Clustering Approaches for Domestic Electricity Load Profile Characterisation-Implications for Demand Side Management. Energy 180, 665–677. doi:10.1016/j.energy.2019.05.124
Zhang, C., Wei, Y.-L., Cao, P.-F., and Lin, M.-C. (2018). Energy Storage System: Current Studies on Batteries and Power Condition System. Renew. Sustain. Energy Rev. 82, 3091–3106. doi:10.1016/j.rser.2017.10.030
Zhang, T., Zhang, G., Lu, J., Feng, X., and Yang, W. (2011). A New Index and Classification Approach for Load Pattern Analysis of Large Electricity Customers. IEEE Trans. Power Syst. 27 (1), 153–160. doi:10.1109/tpwrs.2011.2167524
Zhao, H., Wu, Q., Hu, S., Xu, H., and Rasmussen, C. N. (2015). Review of Energy Storage System for Wind Power Integration Support. Appl. energy 137, 545–553. doi:10.1016/j.apenergy.2014.04.103
Zheng, S., Huang, G., and Lai, A. C. (2021). Techno-economic Performance Analysis of Synergistic Energy Sharing Strategies for Grid-Connected Prosumers with Distributed Battery Storages. Renew. Energy 178, 1261–1278. doi:10.1016/j.renene.2021.06.100
Zheng, Y., Dong, Z. Y., Luo, F. J., Meng, K., Qiu, J., and Wong, K. P. (2013). Optimal Allocation of Energy Storage System for Risk Mitigation of DISCOs with High Renewable Penetrations. IEEE Trans. Power Syst. 29 (1), 212–220. doi:10.1109/tpwrs.2013.2278850
BESS Battery energy storage system
DLP Daily load profile
EL Energy loss
ESS Energy storage system
GA Genetic algorithm
LSD Load standard deviation
NAS Sodium–sulfur
NPV Net present value
PCA Principal component analysis
PV Photovoltaic
SOC State of charge
d Index for time (days), d = {1,2, … 365}
j Index for number of buildings
t Index for time (hours), t = {1,2, … 24}
yr Index for year
Cap Capacity of the BESS
E Energy (kW/kWh)
Pr Electricity price
pw Rated power of the BESS
Keywords: battery sharing, building community, load leveling, energy management, commercial building
Citation: Li Y, Wang Y, Fukuda H, Gao W and Qian F (2022) Analysis of Energy Sharing Impacts in a Commercial Community: A Case of Battery Energy Storage System Deployment for Load Leveling. Front. Energy Res. 10:929693. doi: 10.3389/fenrg.2022.929693
Received: 27 April 2022; Accepted: 06 June 2022;
Published: 12 July 2022.
Edited by:
Jian Zhao, Shanghai University of Electric Power, ChinaReviewed by:
Konstantinos G. Arvanitis, Agricultural University of Athens, GreeceCopyright © 2022 Li, Wang, Fukuda, Gao and Qian. This is an open-access article distributed under the terms of the Creative Commons Attribution License (CC BY). The use, distribution or reproduction in other forums is permitted, provided the original author(s) and the copyright owner(s) are credited and that the original publication in this journal is cited, in accordance with accepted academic practice. No use, distribution or reproduction is permitted which does not comply with these terms.
*Correspondence: Yafei Wang, eWFmZWl3YW5namFwYW5Ab3V0bG9vay5jb20=
Disclaimer: All claims expressed in this article are solely those of the authors and do not necessarily represent those of their affiliated organizations, or those of the publisher, the editors and the reviewers. Any product that may be evaluated in this article or claim that may be made by its manufacturer is not guaranteed or endorsed by the publisher.
Research integrity at Frontiers
Learn more about the work of our research integrity team to safeguard the quality of each article we publish.