- 1Institute of Modern Physics, Chinese Academy of Sciences, Lanzhou, China
- 2School of Nuclear Science and Technology, University of Chinese Academy of Sciences, Beijing, China
- 3School of Nuclear Science and Technology, Lanzhou University, Lanzhou, China
Compared to assuming a constant turbulent Prandtl number model, an advanced four-equation model has the potential to improve the numerical heat transfer calculation accuracy of low–Prandtl number
1 Introduction
Nuclear energy is playing an increasingly irreplaceable role in the future energy structure as the demand for energy increases rapidly (Gu and Su, 2021). Lead-cooled fast reactor (LFR) is one of the six types of innovative nuclear power systems proposed by the Generation IV International Forum (Abram and Ion, 2008; Pacio et al., 2015). Benefiting from the excellent performance in chemical inertness, neutron economy, and thermohydraulic properties, lead–bismuth eutectic (LBE) is considered as one of most promising coolants for LFR. It is indispensable to research the thermohydraulic behaviors of the LBE inner fuel assembly, which influences the security and economic performance of LFRs but is poorly understood (Martelli et al., 2017; Pacio et al., 2017).
Since it is expensive, parlous, and complicated to conduct an experiment with LBE under a high-temperature state, computational fluid dynamics (CFD) methods are widely employed to study the thermohydraulic characteristics of LBE. The CFD methods can be subdivided into three categories: direct numerical simulation (DNS), large eddy simulation (LES), and Reynolds-averaged Navier–Stokes simulation (RANS). Despite the high calculation accuracy of DNS and LES, they have a high demand for computational resources, and as a result, they are only suitable for some specific and straightforward geometric models (Kawamura et al., 1999). Since the computational cost of the RANS approach is much lower than that of the DNS and LES, the RANS approach is the most widely adopted CFD method in engineering calculation. In the RANS method, the linear eddy-viscosity
In the past four decades, to improve the calculation accuracy of heat transfer for low-
1.1 Differential Heat Flux Model
DHFM is a full second-moment differential model for the transport of Reynolds heat fluxes. Compared with the constant
1.2 Algebraic Heat Flux Model
AHFM is a simplified second-moment form of DHFM, which transports Reynolds heat flux by establishing algebraic equations. Hanjalić et al. (1996), Kenjereš et al. (2005), Otić et al. (2005), and Otić and Grotzbach. (2007) developed and analyzed an implicit algebraic transport equation for the Reynolds heat flux term to close the energy equation. Evaluations and calibrations of AHFM for low-
1.3 A two-equation model for Reynolds heat flux
The
In recent years, the interest in reliable CFD methods used to investigate the turbulent heat transfer of low-
Thus, in the present study, an improved CFD solver buoyant2eqnFoam, which introduces a two-equation
2 Mathematical Model
2.1 Physical Model
Thermal-hydraulic phenomena in a 19-rod bundle geometry are an essential research topic. In the past decades, numerous experimental and simulation researches have been conducted to precisely obtain flow characteristics and heat transfer correlations of coolant (Pacio et al., 2014; Martelli et al., 2017). In the present study, a bare 19-rod bundle with a fully developed turbulent LBE flow is considered. Figure 1A displays the cross section of the bare 19-rod bundle. Since the cross-flow in the bare 19-rod bundle is negligible and its construction is symmetrical, one-twelfth of the whole bundle is selected to carry out simulation for the sake of economizing computational cost. The computational domain is sketched in Figure 1B, together with the definitions of sub-channels and boundary regions.
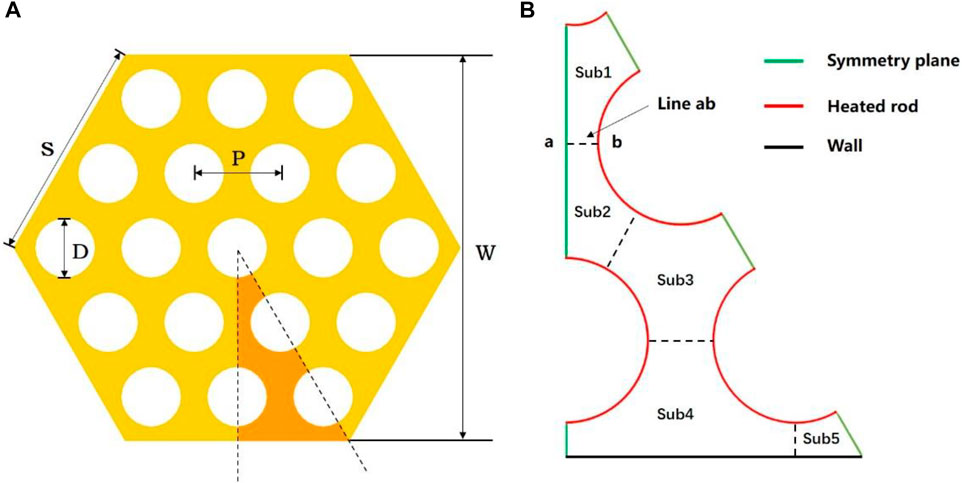
FIGURE 1. Diagrammatic sketch of the bare 19-rod bundle. (A) Cross section and (B) computational domain.
2.2 Conservation Equations
For forced convection, the incompressible RANS equations with constant physical properties and no gravity are considered
where
where
It should be noted that, in OpenFOAM, the energy conservation equation can be expressed in terms of enthalpy (Darwish and Moukalled, 2021):
where
2.3 Turbulence Model for Momentum Field
Benefiting from replacing the friction velocity
where
where
The model constants utilized in the Abe
2.4 Two-Equation Model for Thermal Field
In the current work, the
where
with the appropriate functions set as follows:
where
The constant empirical coefficients used in the Manservisi
3 Solver and Boundary Conditions
To calculate the thermal-hydraulic characteristics of LBE, a CFD solver named buoyant2eqnFoam was developed on the OpenFOAM platform having user-friendly programming language features based on the turbulence model and the aforementioned turbulent heat transfer model. The SIMPLE algorithm is adopted to handle pressure–velocity coupling equations and the coupled multigrid iterations technique is utilized for matrix solutions. All calculations were performed using double precision on OpenFOAM and the convergence conditions of residual error are set as follows:
where
In the computational domain, periodic boundary conditions are set on the region of inlet and outlet, considering the fully developed turbulent inner flow in the bundle. It is worth noting that the energy source term needs to be added to the energy Eq. 4 in order to apply periodic boundary conditions to temperature variables. The calculation method of energy source term refers to this literature (Ge et al., 2017). For
4 Results and Discussions
4.1 Mesh Independence Analysis
In this section, the buoyant2eqnFoam, which utilizes the Abe
Three sets of mesh with different mesh numbers of 2.05 million, 2.64 million, and 3.11 million were adopted to analyze the mesh sensitivity. The dimensionless coolant temperature
where
4.2 Solver Verification
The fully developed turbulent heat transfer characteristics of LBE inner flow in the bare 19-rod bundle were studied by Chierici et al. (2019), using a four-equation model in logarithmic specific dissipation form
Because these reported correlations were developed for triangular lattices, the Nusselt number of inner sub-channel
where
4.3 Flow and Heat Transfer Analysis
4.3.1 Velocity Field
The profiles of velocity magnitude on the computational domain of the bare 19-rod bundle are reported in Figure 6, with
4.3.2 Dimensionless Temperature and Hot Spot Factor Distributions
Figure 7 shows the distribution of dimensionless temperature from where it can be seen that the maximum temperature is located in the corner sub-channel
The dimensionless hot spot factor characterizing the inhomogeneity of wall temperature is defined as follows:
where
4.3.3 Dimensionless Thermal Diffusivity Distribution
To analyze the dependence of heat transfer on
4.3.4 Turbulent Prandtl Number Distribution
The distribution of
The mean turbulent Prandtl number
In order to investigate the influence of
4.4 Assessment of Different Turbulence Models and Turbulent Heat Transfer Models
To analyze the effect of the turbulence model on the simulation of heat transfer, in this sub-section, various turbulence models of OpenFOAM are also employed in buoyant2eqnFoam, including standard
Moreover, the
5 Conclusion
In the current study, the Abe
1) The Nusselt numbers obtained by the self-compiled solver buoyant2eqnFoam are in good agreement with experimental correlations and Chierici simulation research, indicating the validity and reliability of the self-compiled solver.
2) In the bare 19-rod bundle with
3) Although the turbulent Prandtl number of LBE inner flow in the bare 19-rod bundle will decrease as the Peclet number increases, the overall turbulent Prandtl number is higher than 0.85, revealing that the Reynolds-analogy hypothesis about
4) The turbulence model has a considerable influence on the calculation of turbulent heat transfer of low–
The applicability of the solver developed in the present study for the more complicated geometry like fuel assembly with grid spacer or wire-wrapped configurations requires further verification.
Data Availability Statement
The original contributions presented in the study are included in the article/supplementary material, further inquiries can be directed to the corresponding authors.
Author Contributions
XL: concept, research, writing, editing, code, and data processing; XS: modification, concept, research, and code; LG: fund, project management, concept, and research; LZ: editing and research; and XS: editing and research.
Funding
This study was supported by the Research on key technology and safety verification of primary circuit, Grant No. 2020YFB1902104; the Experimental study on thermal hydraulics of fuel rod bundle, Grant No. Y828020XZ0; and the National Natural Science Foundation of China, Grant No.12122512.
Conflict of Interest
The authors declare that the research was conducted in the absence of any commercial or financial relationships that could be construed as a potential conflict of interest.
Publisher’s Note
All claims expressed in this article are solely those of the authors and do not necessarily represent those of their affiliated organizations, or those of the publisher, the editors, and the reviewers. Any product that may be evaluated in this article, or claim that may be made by its manufacturer, is not guaranteed or endorsed by the publisher.
References
Abe, K., Kondoh, T., and Nagano, Y. (1994). A New Turbulence Model for Predicting Fluid Flow and Heat Transfer in Separating and Reattaching Flows-I. Flow Field Calculations. Int. J. Heat Mass Transf. 37 (1), 139–151. doi:10.1016/0017-9310(94)90168-6
Abram, T., and Ion, S. (2008). Generation-IV Nuclear Power: A Review of the State of the Science. Energy Policy 36 (12), 4323–4330. doi:10.1016/j.enpol.2008.09.059
Carteciano, L., Dorr, B., Grötzbach, G., Olbrich, W., and Jin, X. J. F. (2001). Two-and Three-Dimensional Thermal and Fluid-Dynamical Analysis of the Complete MEGAPIE-Module with the Computer Code. FLUTAN 6653, 443–458.
Carteciano, L. J. H. (1995). Analysis of a Turbulence Model for Buoyant Flows Implemented in the 3d Thermal-Hydraulic Computer Code FLUTAN and Comparison with the Standard Kε-Σt Model. Turbulence 1.
Carteciano, L. N., and Grötzbach, G. (2003). Validation of Turbulence Models in the Computer Code FLUTAN for a Free Hot Sodium Jet in Different Buoyancy Flow Regimes. ETDEWEB.
Carteciano, L., Weinberg, D., and Müller, U. (1997). “Development and Analysis of a Turbulence Model for Buoyant Flows,” in Proc. of the 4th World Conf. on Experimental Heat Transfer, Fluid Mechanics and Thermodynamics, Bruxelles, Belgium, 2–6. June: Citeseer).
Cerroni, D., Da Via, R., Manservisi, S., Menghini, F., Pozzetti, G., and Scardovelli, R. (2015). Numerical Validation of a κ-ω-κθ-ωθ Heat Transfer Turbulence Model for Heavy Liquid Metals. J. Phys. Conf. Ser. 655 (1), 012046. IOP Publishing). doi:10.1088/1742-6596/655/1/012046
Cervone, A., Chierici, A., Chirco, L., Da Vià, R., Giovacchini, V., and Manservisi, S. (2020). CFD Simulation of Turbulent Flows over Wire-Wrapped Nuclear Reactor Bundles Using Immersed Boundary Method. J. Phys. Conf. Ser. 1599 (1), 012022. IOP Publishing). doi:10.1088/1742-6596/1599/1/012022
Cheng, X., and Tak, N.-I. (2006). Investigation on Turbulent Heat Transfer to Lead-Bismuth Eutectic Flows in Circular Tubes for Nuclear Applications. Nucl. Eng. Des. 236 (4), 385–393. doi:10.1016/j.nucengdes.2005.09.006
Chierici, A., Chirco, L., Da Vià, R., and Manservisi, S. (2019). Numerical Simulation of a Turbulent Lead Bismuth Eutectic Flow inside a 19 Pin Nuclear Reactor Bundle with a Four Logarithmic Parameter Turbulence Model. J. Phys. Conf. Ser. 1224 (1), 012030. IOP Publishing). doi:10.1088/1742-6596/1224/1/012030
Da Vià, R., Giovacchini, V., and Manservisi, S. (2020). A Logarithmic Turbulent Heat Transfer Model in Applications with Liquid Metals for Pr= 0.01–0.025. Appl. Sci. 10 (12), 4337. doi:10.3390/app10124337
Da Vià, R., Manservisi, S., and Menghini, F. (2016). A K-Ω-Kθ-Ωθ Four Parameter Logarithmic Turbulence Model for Liquid Metals. Int. J. Heat Mass Transf. 101, 1030–1041. doi:10.1016/j.ijheatmasstransfer.2016.05.084
Da Vià, R., and Manservisi, S. (2019). Numerical Simulation of Forced and Mixed Convection Turbulent Liquid Sodium Flow over a Vertical Backward Facing Step with a Four Parameter Turbulence Model. Int. J. Heat Mass Transf. 135, 591–603. doi:10.1016/j.ijheatmasstransfer.2019.01.129
Darwish, M., and Moukalled, F. (2021). The Finite Volume Method in Computational Fluid Dynamics: An Advanced Introduction with OpenFOAM® and Matlab®. Springer.
De Santis, A., and Shams, A. (2018). Application of an Algebraic Turbulent Heat Flux Model to a Backward Facing Step Flow at Low Prandtl Number. Ann. Nucl. Energy 117, 32–44. doi:10.1016/j.anucene.2018.03.016
De Santis, D., De Santis, A., Shams, A., and Kwiatkowski, T. (2018). The Influence of Low Prandtl Numbers on the Turbulent Mixed Convection in an Horizontal Channel Flow: DNS and Assessment of RANS Turbulence Models. Int. J. Heat Mass Transf. 127, 345–358. doi:10.1016/j.ijheatmasstransfer.2018.07.150
Deng, B., Wu, W., and Xi, S. (2001). A Near-Wall Two-Equation Heat Transfer Model for Wall Turbulent Flows. Int. J. Heat Mass Transf. 44 (4), 691–698. doi:10.1016/s0017-9310(00)00131-9
Ge, Z., Liu, J., Zhao, P., Nie, X., and Ye, M. (2017). Investigation on the Applicability of Turbulent-Prandtl-Number Models in Bare Rod Bundles for Heavy Liquid Metals. Nucl. Eng. Des. 314, 198–206. doi:10.1016/j.nucengdes.2017.01.032
Gräber, H., and Rieger, M. J. A. (1972). Experimentelle Untersuchung des Wärmeübergangs an Flüssigmetalle (NaK) in parallel durchströmten Rohrbündeln bei konstanter und exponentieller Wärmeflussdichteverteilung. Atomkernenergie (ATKE) Bd. 19 23-40.
Gu, L., and Su, X. (2021). Latest Research Progress for LBE Coolant Reactor of China Initiative Accelerator Driven System Project. Front. Energy 15 (4), 810–831. doi:10.1007/s11708-021-0760-1
Hanjalić, K., Kenjereš, S., and Durst, F. (1996). Natural Convection in Partitioned Two-Dimensional Enclosures at Higher Rayleigh Numbers. Int. J. Heat Mass Transf. 39 (7), 1407–1427.
Hattori, H., Nagano, Y., and Tagawa, M. (1993). Analysis of Turbulent Heat Transfer under Various Thermal Conditions with Two-Equation Models. In Engineering Turbulence Modelling and Experiments, 43–52. Elsevier
He, S., Wang, M., Zhang, J., Tian, W., Qiu, S., Su, G. H., et al. (2021). Numerical Simulation of Three-Dimensional Flow and Heat Transfer Characteristics of Liquid Lead-Bismuth. Nucl. Eng. Technol. 53 (6), 1834–1845. doi:10.1016/j.net.2020.12.025
Hellsten, A. (2012). “Some Improvements in Menter's K-Omega SST Turbulence Model,” in 29th AIAA, Fluid Dynamics Conference, 2554.
Hwang, C. B., and Lin, C. A. (1999). A Low Reynolds Number Two-Equation Model to Predict Thermal Fields. Int. J. Heat Mass Transf. 42 (17), 3217–3230. doi:10.1016/s0017-9310(98)00382-2
Karcz, M., and Badur, J. (2005). An Alternative Two-Equation Turbulent Heat Diffusivity Closure. Int. J. Heat Mass Transf. 48 (10), 2013–2022. doi:10.1016/j.ijheatmasstransfer.2004.12.006
Kawamura, H., Abe, H., Matsuo, Y., and Flow, F. (1999). DNS of Turbulent Heat Transfer in Channel Flow with Respect to Reynolds and Prandtl Number Effects. Int. J. Heat Fluid Flow 20 (3), 196–207. doi:10.1016/s0142-727x(99)00014-4
Kenjereš, S., Gunarjo, S., and Hanjalić, K. (2005). Contribution to Elliptic Relaxation Modelling of Turbulent Natural and Mixed Convection. Int. J. Heat Fluid Flow 26 (4), 569–586.
Launder, B. E., and Sharma, B. I. (1974). Application of the Energy-Dissipation Model of Turbulence to the Calculation of Flow Near a Spinning Disc. Lett. Heat Mass Transf. 1 (2), 131–137. doi:10.1016/0094-4548(74)90150-7
Launder, B. E., and Spalding, D. B. (1972). Lectures in Mathematical Models of Turbulence. Academic Press.
Launder, B. E., and Spalding, D. B. (1983). “The Numerical Computation of Turbulent Flows,” in Numerical Prediction of Flow, Heat Transfer, Turbulence and Combustion (Elsevier), 96–116. doi:10.1016/b978-0-08-030937-8.50016-7
Manservisi, S., and Menghini, F. (2014a). A CFD Four Parameter Heat Transfer Turbulence Model for Engineering Applications in Heavy Liquid Metals. Int. J. Heat Mass Transf. 69, 312–326. doi:10.1016/j.ijheatmasstransfer.2013.10.017
Manservisi, S., and Menghini, F. (2015). CFD Simulations in Heavy Liquid Metal Flows for Square Lattice Bare Rod Bundle Geometries with a Four Parameter Heat Transfer Turbulence Model. Nucl. Eng. Des. 295, 251–260. doi:10.1016/j.nucengdes.2015.10.006
Manservisi, S., and Menghini, F. (2014b). Triangular Rod Bundle Simulations of a CFD κ-ϵ-κ-ϵ Heat Transfer Turbulence Model for Heavy Liquid Metals. Nucl. Eng. Des. 273, 251–270. doi:10.1016/j.nucengdes.2014.03.022
Mareska, M. W., and Dwyer, O. E. (1964). Heat Transfer in a Mercury Flow along Bundles of Cylindrical Rods. J. Heat. Transf. 86 (2), 180–186.)
Martelli, D., Marinari, R., Barone, G., Di Piazza, I., and Tarantino, M. (2017). CFD Thermo-Hydraulic Analysis of the CIRCE Fuel Bundle. Ann. Nucl. Energy 103, 294–305. doi:10.1016/j.anucene.2017.01.031
Menter, F., and Esch, T. (2001). “Elements of Industrial Heat Transfer Predictions,” in 16th Brazilian Congress of Mechanical Engineering (COBEM), 650.
Menter, F. R., Kuntz, M., and Langtry, R. J. T. (2003). Ten Years of Industrial Experience with the SST Turbulence Model. Heat Mass Transf. 4 (1), 625–632.
Mikityuk, K. (2009). Heat Transfer to Liquid Metal: Review of Data and Correlations for Tube Bundles. Nucl. Eng. Des. 239 (4), 680–687. doi:10.1016/j.nucengdes.2008.12.014
Nagano, Y., Hattori, H., and Abe, K. (1997). Modeling the Turbulent Heat and Momentum Transfer in Flows under Different Thermal Conditions. Fluid Dyn. Res. 20 (1-6), 127–142. doi:10.1016/s0169-5983(96)00049-4
Nagano, Y., and Kim, C. (1988). A Two-Equation Model for Heat Transport in Wall Turbulent Shear Flows. J. Heat. Transf. 110 (3), 583–589. doi:10.1115/1.3250532
Nagano, Y., and Shimada, M. (1996). Development of a Two‐equation Heat Transfer Model Based on Direct Simulations of Turbulent Flows with Different Prandtl Numbers. Phys. Fluids 8 (12), 3379–3402. doi:10.1063/1.869124
Otić, I., and Grötzbach, G. (2007). Turbulent Heat Flux and Temperature Variance Dissipation Rate in Natural Convection in Lead-Bismuth. Nucl. Sci. Eng. 155 (3), 489–496.
Otić, I., Grötzbach, G., and Wörner, M. (2005). Analysis and Modelling of the Temperature Variance Equation in Turbulent Natural Convection for Low-Prandtl-Number Fluids. J. Fluid Mech. 525, 237–261.
Pacio, J., Daubner, M., Fellmoser, F., Litfin, K., Marocco, L., Stieglitz, R., et al. (2014). Heavy-liquid Metal Heat Transfer Experiment in a 19-rod Bundle with Grid Spacers. Nucl. Eng. Des. 273, 33–46. doi:10.1016/j.nucengdes.2014.02.020
Pacio, J., Litfin, K., Batta, A., Viellieber, M., Class, A., Doolaard, H., et al. (2015). Heat Transfer to Liquid Metals in a Hexagonal Rod Bundle with Grid Spacers: Experimental and Simulation Results. Nucl. Eng. Des. 290, 27–39. doi:10.1016/j.nucengdes.2014.11.001
Pacio, J., Wetzel, T., Doolaard, H., Roelofs, F., and Van Tichelen, K. (2017). Thermal-hydraulic Study of the LBE-Cooled Fuel Assembly in the MYRRHA Reactor: Experiments and Simulations. Nucl. Eng. Des. 312, 327–337. doi:10.1016/j.nucengdes.2016.08.023
Shams, A., Mikuž, B., and Roelofs, F. (2018). Numerical Prediction of Flow and Heat Transfer in a Loosely Spaced Bare Rod Bundle. Int. J. Heat Fluid Flow 73, 42–62. doi:10.1016/j.ijheatfluidflow.2018.07.006
Shams, A., Roelofs, F., Baglietto, E., Lardeau, S., and Kenjeres, S. (2014). Assessment and Calibration of an Algebraic Turbulent Heat Flux Model for Low-Prandtl Fluids. Int. J. Heat Mass Transf. 79, 589–601. doi:10.1016/j.ijheatmasstransfer.2014.08.018
Shams, A. (2018). Towards the Accurate Numerical Prediction of Thermal Hydraulic Phenomena in Corium Pools. Ann. Nucl. Energy 117, 234–246. doi:10.1016/j.anucene.2018.03.031
Shin, J. K., An, J. S., Choi, Y. D., Kim, Y. C., and Kim, M. S. (2008). Elliptic Relaxation Second Moment Closure for the Turbulent Heat Fluxes. J. Turbul. 9 (9), N3. doi:10.1080/14685240701823101
Sommer, T. P., So, R. M. C., and Lai, Y. G. (1992). A Near-Wall Two-Equation Model for Turbulent Heat Fluxes. Int. J. Heat Mass Transf. 35 (12), 3375–3387. doi:10.1016/0017-9310(92)90224-g
Su, X., Li, X., Wang, X., Liu, Y., Chen, Q., Shi, Q., et al. (2022). Development and Assessment of an Isotropic Four-Equation Model for Heat Transfer of Low Prandtl Number Fluids. Front. Energy Res. 57. doi:10.3389/fenrg.2022.816560
Subbotin, V., Ushakov, P., Kirillov, P., Ibragimov, M., Ivanovski, M., Nomofilov, E., et al. (1965). “Heat Transfer in Elements of Reactors with a Liquid Metal Coolant,” in Proceedings of the 3rd International Conference on Peaceful Use of Nuclear Energy, 192–200.
Youssef, M. S. (2006). A Two-Equation Heat Transfer Model for Wall Turbulent Shear Flows. JES. J. Eng. Sci. 34 (6), 1877–1903. doi:10.21608/jesaun.2006.111184
Keywords: low Pr fluid, liquid metal, turbulence model, turbulent heat transfer model, OpenFOAM
Citation: Li X, Su X, Gu L, Zhang L and Sheng X (2022) Numerical Study of Low Pr Flow in a Bare 19-Rod Bundle Based on an Advanced Turbulent Heat Transfer Model. Front. Energy Res. 10:922169. doi: 10.3389/fenrg.2022.922169
Received: 17 April 2022; Accepted: 06 May 2022;
Published: 20 June 2022.
Edited by:
Yixiang Liao, Helmholtz Association of German Research Centres (HZ), GermanyReviewed by:
Jinbiao Xiong, Shanghai Jiao Tong University, ChinaHui Cheng, Sun Yat-sen University, China
Copyright © 2022 Li, Su, Gu, Zhang and Sheng. This is an open-access article distributed under the terms of the Creative Commons Attribution License (CC BY). The use, distribution or reproduction in other forums is permitted, provided the original author(s) and the copyright owner(s) are credited and that the original publication in this journal is cited, in accordance with accepted academic practice. No use, distribution or reproduction is permitted which does not comply with these terms.
*Correspondence: Long Gu, Z3Vsb25nQGltcGNhcy5hYy5jbg==; Xingkang Su, c3V4aW5na2FuZ0BpbXBjYXMuYWMuY24=