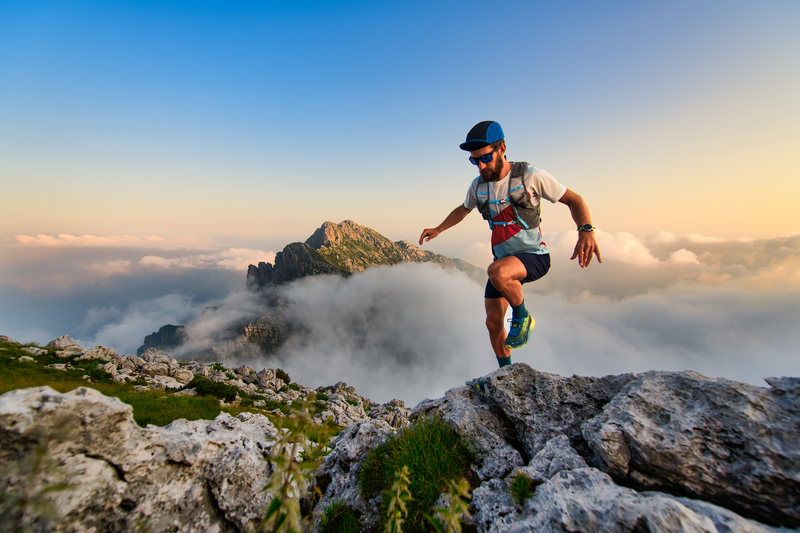
95% of researchers rate our articles as excellent or good
Learn more about the work of our research integrity team to safeguard the quality of each article we publish.
Find out more
ORIGINAL RESEARCH article
Front. Energy Res. , 03 June 2022
Sec. Smart Grids
Volume 10 - 2022 | https://doi.org/10.3389/fenrg.2022.911985
This article is part of the Research Topic Energy Hubs in Modern Energy Systems with Renewables and Energy Storage View all 7 articles
Electrical power communication networks undertake complicated power services. They interact with physical power grids all the time, which will result in a high risk to power grids when their electric power communication network is attacked. Therefore, to improve the reliability of power systems, it is of great significance to identify the key links of communication networks. Existing research studies focus more on the risk of power communication services undertaken by communication links, which ignores the frequent interaction between power grids and communications networks. In this paper, from a multi-layer network perspective, an assessment method of communication equipment is proposed in view of the operation characteristics of nodes in different systems as well as the dynamic fault propagation between homogeneous communication nodes. Firstly, we build a model of cyber-physical power interdependent network. Then, for communication nodes, a dynamic model is adopted for cascading failure among the nodes based on the load-capacity model. Afterward, we define an index to evaluate the node importance considering characteristics of the operation of both communication networks and power grids. Finally, a local power grid is taken as an example for verification by comparing the effects of different indexes. The simulation results demonstrate the validity of the evaluation method proposed.
The cyber side of the smart grid is the information infrastructure to support the primary equipment in the physical side and the development of electric power enterprises (Chen et al., 2021). In addition, it is the path to improve the digital and the intelligent level of the power system (Al-Saud et al., 2019). However, the global energy transition brings several new elements, such as the multi-task voltage and current measurement device with high accuracy proposed by Li et al. (2021), the decision-making systems of scheduling plan of thermal units, hydropower units, and wind turbines proposed by Yang et al. (2021), and other intelligent devices. New elements are likely to add uncertainty to the stable operation of power grids (Ma et al., 2020; Zhang et al., 2021). Meanwhile, this makes the structure of the cyber side of smart grids, which is called the electric power communication network, and the physical side (physical power grids consisting of primary equipment) more sophisticated and vulnerable (Li et al., 2021). When a power communication network suffers an attack (including equipment aging, network attacks, and equipment damage caused by extreme weather), fault spread across the two systems, threatening the operation of power grids (Wu et al., 2021). Consequently, it is necessary to identify the vulnerable links or important nodes in electric power communication networks, to maintain the stability of power systems and to draw up the long-term plan of the architecture of electric power communication network (Avatefipour et al., 2019; Mohamed, et al., 2021).
At present, the key communication nodes are mainly studied in two aspects. On the one hand, many objects of research studies are the single side of smart grids. Researchers have established the model of electric power communication networks and identified the key nodes mainly according to the communication links undertaking power services. To evaluate important nodes, some research studies considered the average risk degree of business and the balance degree of business risk as evaluation indicators (Shao et al., 2016). Moreover, link capacity (Constantinou and Ellinas, 2016) and the extent of power business importance (Du et al., 2021) were used to evaluate network reliability. These studies mainly evaluated the reliability of the network or the importance of nodes from the perspective of undertaking power communication services, so the model of the communication network can be relatively accurate. However, changes in communications network will have a significant impact on not only the power services but also the structure of both itself and the power grid it controls.
Therefore, to consider the deep coupling of services and structure between the physical power grid and the communication network, a growing number of studies pay attention to the cyber-physical power system (CPPS) (Zhao et al., 2010; Liu et al., 2015; Ji et al., 2016; Qing and Liu, 2020). Generally, the CPPS has been divided into three layers: equipment layer, topology layer, and functional layer (Shao et al., 2016; Mohamed, et al., 2019; Xu et al., 2021). Shao et al. (2016) discussed the relationship between the three layers and network business risk, came up with a risk assessment method of power business, and concluded that network risk was mainly related to the key nodes and the links of the networks. Additionally, power network operation and maintenance personnel are in direct contact with communication equipment, so the specific equipment evaluation of the communication network can make the key links and nodes of the communication network more oriented and targeted. Parandehgheibi and Modiano (2013) put forward the view that infrastructures were strongly interdependent and proposed the importance of assessment methods of social infrastructures from qualitative to semi-quantitative in general scenarios, which were of certain guiding significance but could not be directly applied to specific scenarios like CPSs. Guo et al. (2016) established a fine-grained model at the equipment level from the perspective of physical energy loss. Furthermore, its link with the physical power grid was reflected in energy loss rather than topological structure change. Nevertheless, the above studies have not considered the dynamic influence between network devices on the same side (i.e., the interaction between communication devices) while considering the topological interdependence between physical power grids.
In this paper, to deal with the above problems, a communication equipment evaluation method is proposed based on node dynamic failure. The primary contributions of this paper can be summarized as follows:
1) Considering the dynamic failure mechanism of nodes from the same network on the basis of node-to-edge interdependent networks.
2) Defining an evaluation index of communication equipment importance consisting of network efficiency of the power grid and the largest connected subgraph (LCS) of communication network.
3) Proposing a communication equipment evaluation method based on “point-to-edge” interdependent networks.
In Section 2, we explain the theory of a node-to-edge interdependent network. In Section 3, an evaluation method based on dynamic failure mechanism and node-to-edge interdependent network model is put forward. In Section 4, results from a case of real power system reveal the effectiveness of the indexes and the assessment method.
Various approaches for coupling two unilateral networks into the interdependent network have been proposed by different researchers. Among them, Ji (2016) summarized and classified them as follows: “one-to-one” interdependent network (Buldyrev et al., 2010), “partially interdependent” network (Zhou et al., 2013), “multiple interdependent” network (Shao et al., 2011), “one-to-many” interdependent network (Zhen et al., 2013), “many-to-many” interdependent network (Huang et al., 2013), and cyber-physical power interdependent network considering heterogeneity of nodes. These models completely summarized the “node-to-node” interdependence between two networks.
The above models completely summarize “node-to-node” dependence relationships between two networks and are suitable for the cases where nodes are equivalent to plants or stations. However, when specific communication equipment needs to be evaluated, it is not always through nodes in which communication networks and power grids are coupled to each other. For example, there is a “device–power line” relationship between a circuit breaker and its corresponding power line. A device is equivalent to a node, while a power line is equivalent to an edge. The traditional theory cannot accurately involve the situation of coupling between “node” and “edge.”
The node-to-edge interdependency model can describe the coupling relationship between the power communication network and the physical power grid.
For the node-to-edge dependent network, the following hypotheses are made:
Hypothesis 1: For a one-side network, a node will only fail if all of its connecting edges stop functioning.
Hypothesis 2: When the node fails, all its connecting edges and dependent edges stop functioning (“disappear”).
Hypothesis 3: If a node becomes an outlier, it will disappear.
Hypothesis 4: In the initial network, if a node is connected to a dependent edge, the node will fail when its dependent edge is removed.
Hypothesis 5: In the initial network, if a connecting edge is connected to the dependent edge, the connecting edge will stop functioning when the dependency edge fails.
As is shown in Figure 1A, the black edges are dependent edges, the blue nodes and edges belong to a communication network, and the green nodes and the edges belong to a physical power grid. Set the dark green node as a failed node of the communication network without considering the cascading failure of nodes belonging to the same network, and its topology is shown in Figure 1A. First, the connecting edge connected to the broken node disappears, and the dependent edge of the connecting edge also fails as shown in Figure 1B. Then, due to the failure of the dependent edge, the other end of the dependent edge, namely, the corresponding node of the physical grid, also stops functioning. And the ultimate LCS is shown in Figure 1C.
FIGURE 1. Cascading process of cyber node failure (A–C). (A) The first step of the cascading failure. (B) The second step of the cascading failure (C) is the third step of the cascading failure.
Virtual nodes are those that have no actual meaning. They are set up to satisfy the traditional model because present structures of the interdependent network are both ends of dependent edges, which are nodes.
In the traditional model, as shown in Figure 2A, virtual nodes are added at the junction of all dependent edges and physical grid connecting edges. When the same faulty node occurs in the power grid, the dark green node with only a connecting edge will not cause cascading failure due to the traditional interdependent theory. Therefore, the fault stops at the virtual node near the faulty node. Its final LCS is shown in Figure 2B. It can be found that when a fault occurs on the power grid, the results are not equivalent in terms of cascading faults between networks, where the “node-to-node” model adds nodes to the physical power grid. Based on the above analysis, the “node-to-edge” model includes scenes different from traditional models.
FIGURE 2. Cascading process with virtual nodes added to cyber node failure (A,B). (A) The first step of the cascading failure. (B) The second step of the cascading failure.
In the undirected dependent edge model of “node-to-edge,”
where
To accurately evaluate the equipment inside the substation, it is fundamental to model the substation monitoring system. On the basis of the main power business undertaken by secondary equipment, the intelligent substation secondary equipment can be divided into three layers: station layer, bay layer, and process layer. Then, according to the business chain of substation equipment (Liu et al., 2019), the communication network model of substation is established as shown in Figure 3.
To simplify the model, a device-level topology model is established and the following assumptions are made:
Hypothesis 1: The automation systems of control centers at all levels are abstracted as nodes of control centers.
Hypothesis 2: Each device in communication networks of each substation is abstracted as a node, and the physical and cyber connection between two devices is abstracted as an edge.
Here, we define new types of nodes, critical nodes. Critical nodes are those that are connected to nodes outside a substation. And the nodes inside substations are divided into two categories: internal nodes and critical nodes. As is shown in Figure 3, the control node means the control center. And the nodes from the telecontrol workstation to circuit breakers and mutual inductors all belong to the substation. The telecontrol workstation is connected to the control node outside the station and the switch at the station control layer inside. Thus, it is a critical node. Meanwhile, the other nodes in the substation are defined as internal nodes.
The site connection graph of power communication network can be expressed as follows:
where
Secondary equipment of a power system belongs to the cyber side of a power grid, but it has a direct or indirect effect on the physical side. Thus, it is difficult to reflect the reality of power systems to evaluate the secondary equipment from single side alone. In consideration of the cyber-physical coupling relationship, circuit breakers are considered the interactive operation point between communication systems and physical systems (Guo et al., 2016). On the one hand, it controls the on–off state of the power line. On the other hand, it is an analog signal collected by the information monitoring system in communication networks. Therefore, we control the connecting edge of physical networks through the state of breaker nodes of a communication network. Then, a undirected “node-to-edge” interdependent network model can be established. Based on the definition of critical nodes in Section 2.1, breakers and mutual inductors also belong to critical nodes because they are externally connected to dependency edges and internally connected to intelligent terminals and merging units.
The model obtained from the above description can be described as follows:
where
The adjacency matrix could be obtained from the graph
To sum up, the steps of the CPPS model establishment of general power systems are as follows:
Step 1: Establish a model based on the service chain for all substations.
Step 2: Input topology of the communication network and the physical power grid.
Step 3: Determine the dependencies and connect the critical nodes of the substation to the outside.
For a single-layer complex network with N nodes,
When the network becomes disconnected due to node failure,
The difference between network efficiency and LCS is whether remaining connected branches are considered. As is known to all, for main networks with a mass of power generation and substation nodes, more than nodes from the largest connected branches can work when the networks evolve into an unconnected graph.
After node i is deleted, we get the remaining network efficiency represented as
However, for the “node-to-edge” interdependent network established, the network efficiency of the original single-layer complex network is not enough to measure the node importance. It needs to integrate the characteristics of both sides of an interdependent network. To investigate the influence of nodes in the communication network on the efficiency of power grid, the importance degree of nodes of the interdependent network built in this paper is defined as
where
In terms of the definition of node importance, this paper considers the impact of node failure on communication network and physical network topology, respectively.
The reasons for selecting different indexes to measure the importance of nodes to the two networks are as follows: 1) The communication nodes separated from the inside of the station and the control center cannot convey information, so the importance of nodes relative to the communication network is examined by the relative size of the largest connected branch. 2) In the case of isolated island operation of physical power grid, nodes other than the largest connected branch may still operate; hence, the influence of cyber nodes on the power network is considered based on the efficiency of interdependent network. With the influence of the two aspects integrated, the above two indicators are weighted to obtain the final node importance.
Provided that information is always exchanged along the shortest path between nodes in an electric power communication network, the removal of nodes with relatively large betweenness will have a more prominent impact on other nodes and be more likely to lead to cascading faults (Motter and Lai. 2003). In the model, when a node fails, the load it carries is passed to the next node along the shortest path, and the load of the next node changes accordingly.
Based on the cascading failure model proposed by Motter, we take the initial network betweenness of nodes as the initial load value. Betweenness is the proportion of the shortest path passing through the node in all shortest paths, as shown by
where
In view of cascade failures that nodes with high betweenness are prone to, a node should have a maximum allowable value of information load that it can bear. In the cascading failure model (Motter and Lai. 2003), the maximum allowable value of information load of a node is proportional to the initial information load of the node, as is shown by
where
The evaluation process is as follows and is shown in Figure 4:
Step 1: Establish a cyber-physical power coupling model based on the grid topology data.
Step 2: Calculate the initial load of the nodes, when the nodes and value
Step 3: Calculate the maximum allowable value of information load of all nodes in the communication network.
Step 4: Start to attack the cyber node which is to be evaluated.
Step 5: Recalculate the load of all cyber nodes. This is because the load of nodes under attack and faulted nodes changes, and the network topology may also change after removing nodes whose load exceeds the node capacity.
Step 6: Judge whether a new faulty node appears. If yes, perform step 7. If no, go to step 8.
Step 7: Judge whether there is a circuit breaker node. If yes, the corresponding connecting edge of the physical power grid stops functioning. At this time, the physical power grid topology changes. Calculate all evaluation indexes at this moment. And if no, perform step 5.
Step 8: Terminate the cycle and calculate the evaluation indexes. The evaluation process is completed.
In the evaluation process, the successive faults of the communication networks are coupled with the connecting edge of the physical power grid (power line) through circuit breakers. And the load-capacity cascading failure theory is introduced to attack the nodes of the communication network. Finally, the importance of different nodes is reflected by the topological changes of the two-layer network. The assessment process includes both the fault propagation process of the communication network and the structural importance of communication nodes in the overall network topology.
In this evaluation process, the successive faults of the communication network are coupled with the connecting edge of the physical power grid through circuit breakers. And the load-capacity cascading failure theory is introduced to attack the nodes of the communication network. Finally, the importance of different nodes is reflected by the topological changes of the two-layer network. The assessment process includes both the fault propagation mechanism of the communication network and the interaction process between two networks.
A local power grid is taken as an example for simulation analysis. The structure of the power grid is shown in Figure 5. The dependency among substations and their subordinate control center is shown in Table 1. Letters “a” to “m” are power nodes including substations and power plants. Letters “x” to “z” are the numbers of three control centers.
According to the modeling method described in this paper, we established the corresponding substation communication model for each substation node in the communication network. Then, the critical nodes in the model were related to the outside according to the topology of dispatching communication network. The final model contains 200 communication nodes and 15 power nodes, and the dependent node–edge set contains 25 dependent “node–edge” pairs. The sets of power nodes, communication network edges, and interdependent “node-to-edge” pairs are obtained from the constructed network, and the adjacency matrix of the physical power grid and the communication network is, respectively, generated. Then, we can calculate the importance of all nodes.
We take the case when
In most CPPS models established from the perspective of complex network, the proportion of the largest connected branch to the initial nodes was taken as an index to measure network vulnerability and node importance. As is shown in Figure 6, two curves were obtained by calculating the importance of all nodes according to the method in this paper and the above method. Figure 6A is the node importance obtained by the index which is shown in expression (13), based on the method of node deletion. Figure 6B is the node importance obtained by considering the number of nodes of LCS of the communication network based on the method of node deletion. The difference between those two indexes lies in that the former takes into account the existence of isolated power grids, while the latter directly selects the power grid with the largest scale after decompression.
FIGURE 6. Importance of nodes. (A) is double-weighted importance of nodes (B) is the propotion of the nodes of the largest connected branches.
We number the nodes in accordance with the substation numbering order, and each substation’s internal nodes are continuous. Obviously, there is a highest value for every indefinite number of nodes, which means each substation has a communication device whose importance clearly distinguishes it from other communication devices. It reflects the high similarity of each substation’s internal modeling in the two curves in Figures 6A,B because the structure of communication equipment inside different substations is similar.
Figure 7 reveals the curve of degree (denoted by “D”), betweenness (denoted by “B”), C in this paper, and traditional indicator (the node proportion of LCS, which is denoted by “G”) in ascending order. It indicates that the smoothness of the black curve representing the importance of nodes in this paper is better than other indexes. The other three indexes all have more line segments parallel to the x-axis, which means that a lot of devices have the same index values, that is, it is difficult to distinguish the significance of those. Hence, the results of node evaluation of coupled networks have higher resolution and perform better.
We have drawn a separate graph of the black curve near the node ranking 182 in Figure 7. Before this point, the curve rises slowly, after which it begins to rise sharply. The slope of the black curve increases significantly in the last 19 values, that is, the slope of the curve corresponding to the top 19 devices in terms of coupling network loss is the largest. Then, the black curve slowly falls off with the decrease in the index value. Table 2 reveals the top 19 devices in importance. In Table 2, SS represents the switch at the station layer, TS represents the telecontrol workstation, SP represents the switch at the process layer, MU represents the merging unit, and B represents the breaker.
Table 2 indicates that switches at the station layer and switches at the process layer are the most noteworthy among all types of devices; especially switches at the station control layer account for 90% of the most important 10 devices. So, in the maintenance of communication network equipment, special attention should be paid to such equipment. In addition to the switch, the other three important pieces of equipment are No. 150 “telecontrol workstation,” No. 94 “merging unit,” and No. 100 “circuit breaker.” That is because the above devices belong to the site level nodes L and G, respectively. However, the two substations nodes L and G in Figure 5 have higher degrees and are the nodes in the core position in the power grid, which have a great influence on the physical power grid when the substations fail.
As is shown in Figure 8, the index C was arranged in ascending order and compared with the curve of D, B, and G corresponding to each communication node after the arrangement. It can be found that, in terms of the overall trend, the traditional index is relatively consistent with the node importance curve defined in this paper. The distribution interval of degree and betweenness curves is simpler, and the curve fluctuates a lot. Moreover, nodes with high degree overlap with nodes that have high betweenness. Accordingly, there are certain limitations in calculating the importance of communication equipment nodes only from the perspective of the centralization of network nodes: 1) many devices or nodes have the same value of D, B, and G and 2) the preceding indexes in 1) cannot reflect the topology characteristics of the physical power grid.
The blue dotted line in Figure 8 shows the four normalized index values of the node ranked 132, which is numbered 102. The values of each indicator of the No. 102 communication device are shown in Table 3.
As can be seen from Table 3 and Figure 8, the normalized D, B, and G values of No. 102 are obviously much larger than those of C. K, B, and G values of other devices whose C values are close to those of Device 102 are also significantly lower than those of Device 102. There are many similar nodes in Figure 8, and this node is used as an example to analyze why if calculated according to the other three indicators, this node is clearly a very important node. Through the analysis of this node, it is found that this node does not influence the network efficiency of the physical power grid. As a result, considering the role of the physical grid, the node is not that significant to the coupled network:
where
Thus, the traditional indexes are suitable for identifying the important nodes at the center of the single-layer network. Nevertheless, as for the two-layer network, the traditional indicators cannot reflect the coupling between the networks. The equipment importance assessment index defined in this paper solves this problem.
In this article, we investigated the importance of different types of devices in electric power communication networks by attacking them on the basis of an importance assessment method. From the above analysis, the following conclusions can be drawn:
1) The index proposed in this paper can distinguish the importance of equipment in the power communication network better than the traditional complex network index.
2) Considering the services in the model of this paper in the secondary equipment of the communication network, the top 10 pieces of equipment in importance rank are all the switches in the station control layer of each substation.
3) Considering all equipment in the grid, the position of the substation in the grid also affects the importance of equipment.
However, in the further work, more detailed propagation mechanisms of failure and more sophisticated power communication services should be taken into account.
The raw data supporting the conclusions of this article will be made available by the authors, without undue reservation.
YL: Conceptualization, Methodology, Software, Validation, Visualization, Supervision, Investigation, Writing—Original Draft, Writing—Review and Editing. BW: Conceptualization, Methodology, Software, Validation, Visualization, Supervision, Investigation, Writing—Original Draft, Writing—Review and Editing. HW: Conceptualization, Methodology, Software, Validation, Visualization, Supervision, Investigation, Writing—Original Draft, Writing—Review and Editing. FM: Conceptualization, Methodology, Software, Validation, Visualization, Supervision, Investigation, Writing—Original Draft, Writing—Review and Editing. JZ: Conceptualization, Methodology, Software, Validation, Visualization, Supervision, Investigation, Writing—Original Draft, Writing—Review and Editing. HM: Conceptualization, Methodology, Software, Validation, Visualization, Supervision, Investigation, Writing—Original Draft, Writing—Review and Editing. YZ: Conceptualization, Methodology, Software, Validation, Visualization, Supervision, Investigation, Writing—Original Draft, Writing—Review and Editing. MM: Conceptualization, Methodology, Software, Validation, Visualization, Supervision, Investigation, Writing—Original Draft, Writing—Review and Editing.
This work was supported in part by the National Natural Science Foundation of China (No. 51907096).
The authors declare that the research was conducted in the absence of any commercial or financial relationships that could be construed as a potential conflict of interest.
All claims expressed in this article are solely those of the authors and do not necessarily represent those of their affiliated organizations, or those of the publisher, the editors, and the reviewers. Any product that may be evaluated in this article, or claim that may be made by its manufacturer, is not guaranteed or endorsed by the publisher.
Al-Saud, M., Eltamaly, A. M., Mohamed, M. A., and Kavousi-Fard, A. (2019). An Intelligent Data-Driven Model to Secure Intravehicle Communications Based on Machine Learning. IEEE Trans. Industrial Electron. 67 (6), 5112–5119. doi:10.1109/tie.2019.2924870
Avatefipour, O., Al-Sumaiti, A. S., El-Sherbeeny, A. M., Awwad, E. M., Elmeligy, M. A., Mohamed, M. A., et al. (2019). An Intelligent Secured Framework for Cyberattack Detection in Electric Vehicles' CAN Bus Using Machine Learning. IEEE Access 7, 127580–127592. doi:10.1109/access.2019.2937576
Buldyrev, S. V., Parshani, R., Paul, G., Stanley, H. E., and Havlin, S. (2010). Catastrophic Cascade of Failures in Interdependent Networks. Nature 464 (7291), 1025–1028. doi:10.1038/nature08932
Chen, J., Mohamed, M. A., Dampage, U., Rezaei, M., Salmen, S. H., Obaid, S. A., et al. (2021). A Multi-Layer Security Scheme for Mitigating Smart Grid Vulnerability against Faults and Cyber-Attacks. Appl. Sci. 11 (21), 9972. doi:10.3390/app11219972
Constantinou, C. K., and Ellinas, G. (2016). A Load Balancing Technique for Efficient Survivable Multicasting in Mesh Optical Networks. Opt. Switch. Netw. 22, 1–8. doi:10.1016/j.osn.2016.03.002
Du, Z., Chen, J., Zhang, Y., Jiang, X., and Lin, D. (2021). “Multi-objective Routing Optimization for Electric Power Communication Network Considering Business Importance,” in 2021 3rd Asia Energy and Electrical Engineering Symposium (Chengdu, China: AEEES), 959–963. doi:10.1109/AEEES51875.2021.9403042
Guo, J., He, Y. B., Han, Y. Q., Sun, J. B., Li, D. H., and Guo, C. X. (2016). Importance Assessment of Cyber Devices Considering Effective Distance Based Closeness Centrality. Automation Electr. Power Syst. 40 (23), 42–48. doi:10.7500/AEPS20160515021
Huang, Z., Wang, C., Stojmenovic, M., and Nayak, A. (2013). Balancing System Survivability and Cost of Smart Grid via Modeling Cascading Failures. IEEE Trans. Emerg. Top. Comput. 1 (1), 45–56. doi:10.1109/TETC.2013.2273079
Ji, X. P. (2016). Research on the Structural Vulnerability of Electrical Cyber-Physical System Based on Interdependent Networks Theory. [Wuhan (Hubei, China)]: Wuhan University. [Doctor’s thesis].
Ji, X. P., Wang, B., Liu, D. C., and Zhao, T. (2016). Review on Interdependent Networks Theory and its Applications in the Structural Vulnerability Analysis of Electrical Cyber-Physical System. Proceeding CSEE 36 (17), 4521–4533. doi:10.13334/j.0258-8013.pcsee.160492
Li, Z., Jiang, W., Abu-Siada, A., Li, Z., Xu, Y., and Liu, S. (2021). Research on a Composite Voltage and Current Measurement Device for HVDC Networks. IEEE Trans. Ind. Electron. 68 (9), 8930–8941. doi:10.1049/iet-gtd.2017.184510.1109/tie.2020.3013772
Liu, D., Sheng, W. X., Wang, Y., Lu, Y. M., and Sun, C. (2015). Key Technologies and Trends of Cyber Physical System for Power Grid. Proceeding CSEE 35 (14), 3522–3531. doi:10.13334/j.0258-8013.pcsee.2015.14.006
Liu, M. H., Hu, S. Q., Zhu, X. T., He, J., and Cao, X. (2019). Analysis and Display Method of Network Monitoring Business Chain in Smart Substation. Automation Electr. Power Syst. 43 (04), 160–165. doi:10.7500/AEPS20180313008
Ma, H., Liu, Z., Li, M., Wang, B., Si, Y., Yang, Y., et al. (2021). A Two-Stage Optimal Scheduling Method for Active Distribution Networks Considering Uncertainty Risk. Energy Rep. 7, 4633–4641. doi:10.1016/j.egyr.2021.07.023
Mohamed, M. A., Chen, T., Su, W., and Jin, T. (2019). Proactive Resilience of Power Systems against Natural Disasters: A Literature Review. IEEE Access 7, 163778–163795. doi:10.1109/access.2019.2952362
Mohamed, M. A., Mirjalili, S., Dampage, U., Salmen, S. H., Obaid, S. A., and Annuk, A. (2021). A Cost-Efficient-Based Cooperative Allocation of Mining Devices and Renewable Resources Enhancing Blockchain Architecture. Sustainability 13 (18), 10382. doi:10.3390/su131810382
Motter, A. E., and Lai, Y.-C. (2003). Cascade-based Attacks on Complex Networks. Phys. Rev. E 66 (6 Pt 2). doi:10.1103/PhysRevE.66.065102
Parandehgheibi, M., and Modiano, E. (2013). Robustness of Interdependent Networks: The Case of Communication Networks and the Power Grid. IEEE Glob. Commun. Conf. (GLOBECOM), 2164–2169. doi:10.1109/GLOCOM.2013.6831395
Qing, B. Y., and Liu, D. (2020). Research Progress and Prospects on Analysis and Control of Cyber-Physical System for Power Grid. Proceeding CSEE 40 (18), 5816–5827. doi:10.13334/j.0258-8013.pcsee.200422
Shao, J., Buldyrev, S. V., Havlin, S., and Stanley, H. E. (2011). Cascade of Failures in Coupled Network Systems with Multiple Support-Dependence Relations. Phys. Rev. E 83 (3 Pt 2). doi:10.1103/PhysRevE.83.036116
Shao, Z., Wang, Y., Chen, X., Zhang, Y., He, J., and Wang, Z. (2016). “A Network Risk Assessment Methodology for Power Communication Business,” in 2016 IEEE International Conference on Network Infrastructure and Digital Content (IC-NIDC), 40–43. doi:10.1109/ICNIDC.2016.7974532
Wu, Y., Chen, J., Ru, Y., Xu, H., Roger, M., and Ni, M. (2021). Research on Power Communication Network Planning Based on Information Transmission Reachability against Cyber-Attacks. IEEE Syst. J. 15 (2), 2883–2894. doi:10.1109/JSYST.2020.3026997
Xu, L., Guo, Q., Liu, X., and Sun, H. (2021). Robust Optimization Method of Communication Network to Improve Resilience of Cyber-Physical Power System. Automation Electr. Power Syst. 45 (03), 68–75. doi:10.7500/AEPS20200430022
Yang, N., Yang, C., Xing, C., Ye, D., Jia, J., Chen, D., et al. (2021). Deep Learning‐based SCUC Decision‐making: An Intelligent Data‐driven Approach with Self‐learning Capabilities. IET Generation Trans Dist 16, 629–640. doi:10.1049/gtd2.12315
Zhang, L., Xie, Y., Ye, J., Xue, T., Cheng, J., Li, Z., et al. (2021). Intelligent Frequency Control Strategy Based on Reinforcement Learning of Multi-Objective Collaborative Reward Function. Front. Energy Res. 9. in press. doi:10.3389/fenrg.2021.760525
Zhao, J. H., Wen, F. S., Xue, Y. S., Li, X., and Dong, Z. Y. (2010). Cyber Physical Power Systems: Architechture, Implementation Techniques and Challenges. Automation Electr. Power Syst. 34 (16), 1–7.
Zhen Huang, H., Cheng Wang, W., Ruj, S., Stojmenovic, M., and Nayak, A. (2013). “Modeling Cascading Failures in Smart Power Grid Using Interdependent Complex Neworks and Percolation Theory,” in 2013 IEEE 8th Conference on in Industrial Electronics and Applications (ICIEA), 1023–1028. doi:10.1109/ICIEA.2013.6566517
Keywords: electrical power communication network, cyber-physical power system, interdependent network, importance of nodes, load-capacity model
Citation: Li Y, Wang B, Wang H, Ma F, Zhang J, Ma H, Zhang Y and Mohamed MA (2022) Importance Assessment of Communication Equipment in Cyber-Physical Coupled Distribution Networks Based on Dynamic Node Failure Mechanism. Front. Energy Res. 10:911985. doi: 10.3389/fenrg.2022.911985
Received: 03 April 2022; Accepted: 25 April 2022;
Published: 03 June 2022.
Edited by:
Ziad M. Ali, Aswan University, EgyptReviewed by:
Srikanth Goud B, Anurag Group of Institutions, IndiaCopyright © 2022 Li, Wang, Wang, Ma, Zhang, Ma, Zhang and Mohamed. This is an open-access article distributed under the terms of the Creative Commons Attribution License (CC BY). The use, distribution or reproduction in other forums is permitted, provided the original author(s) and the copyright owner(s) are credited and that the original publication in this journal is cited, in accordance with accepted academic practice. No use, distribution or reproduction is permitted which does not comply with these terms.
*Correspondence: Bo Wang, d2h3ZHdiQHdodS5lZHUuY24=
Disclaimer: All claims expressed in this article are solely those of the authors and do not necessarily represent those of their affiliated organizations, or those of the publisher, the editors and the reviewers. Any product that may be evaluated in this article or claim that may be made by its manufacturer is not guaranteed or endorsed by the publisher.
Research integrity at Frontiers
Learn more about the work of our research integrity team to safeguard the quality of each article we publish.