- 1Sichuan Energy Internet Research Institute, Tsinghua University, Chengdu, China
- 2State Grid Energy Research Institute Co., Ltd., Beijing, China
- 3Changzhou Power Supply Company of State Grid Jiangsu Electric Power Co. Ltd., Changzhou, China
Introduction
Renewable energy has become an important source of power because of the benefits of a low-carbon economy. Hydrogen is a clean fuel and has also aroused great concern around the world. Renewable energy can be used to produce hydrogen energy. Zhang et al. presented a novel approach to coordinated control of renewable energy and hydrogen production, and the operation economics of hydrogen fueling stations and the energy system had been improved (Zhang et al., 2022). Much work, so far, has focused on renewable energy systems and energy internet (Zhang, 2018). Notably, photovoltaic (PV) technologies have always been a hotspot in renewable energy systems. There is an urgent need, but it is still a significant challenge to control PV systems in an uncertain environment. A two-stage PV structure was investigated by Fu et al. (2019), who presented an effective technique for PV power integration using μ theory. Li et al. presented a maximum power point tracking method, which can ensure stable PV power generation under partially shaded conditions (Li et al., 2021). With the increase of PV capacity in renewable energy systems, the grid-connected configuration is changing the operation mode of energy networks (Eftekharnejad et al., 2015). To reduce the cost of a PV generation system with battery storage, Hao al. presented a bi-level control method, which also ensured stable PV power generation (Hao et al., 2021). It is worthwhile mentioning that solar PV projects will play an important role in the economy of future electric generation portfolios (Vithayasrichareon et al., 2015). One of the great challenges is that uncertainty in PV modules makes distributed generation planning extra hard in distribution networks. It is generally accepted that statistical machine learning is an effective technology for modeling uncertainties in PV power (Fu et al., 2020). In terms of a distribution network with PV generation, we often need to configure reactive power devices to improve the performance of the energy networks (Fu, 2022). Fu et al. presented an adaptive reactive power control strategy to balance the trade-off between power quality and power loss, and the method enhanced the friendliness of the utility connection for a PV solar system (Fu et al., 2015). In terms of a centralized PV power generation, power factor control and voltage control are the key technologies of PV-grid connection. Awadhi and Moursi invented a novel controller for a centralized PV plant to avoid voltage imbalance, and the transient response was also enhanced (Awadhi and Moursi, 2017). Emmanuel et al. presented a power factor control method based on wavelet variability, and they reported the analysis results of the influence of power factor on the output of centralized photovoltaic power station (Emmanuel et al., 2017).
Scholars have performed a lot of research work on deploying and controlling distributed PV, but they pay little attention to the relationship between distributed and centralized PV power generation. However, due to a decrease in capacity installations, the reliability of operation of power systems may be affected by the superposition of distributed and centralized PV power generation. The components of distributed and centralized PV power generation are the same, and solar panel is made of polycrystalline silicon or thin-film. Distributed PV panels are always installed on the roofs of farm houses and greenhouses, and then the project is mainly concentrated in North and South China. Centralized PV power generation is mainly installed in the Gobi deserts, which spreads on Ningxia, Gansu, Xinjiang, Qinghai, Northwest China.
Numerical Power Balance
Solution Framework
As shown in Figure 1, we proposed a solution framework for analyzing the power balance of a regional power system with distributed and centralized PV power generation. Four modules are given to realize different functions. With respect to the first module, a scenario simulation model of distributed PV generation should be developed for making day-ahead forecasts. The predicted PV power generation results are provided to the dispatching center. We deal with the uncertainty of distributed PV power generation in two ways. Demand-side energy management is given priority to balance the output of distributed PV generation, so as to improve the consumption of local distributed PV generation and prevent the reverse power flow from the PV generation to the distribution networks. With respect to the second module, the aim of acquisition of operation scheduling model is to estimate the electricity supply-demand gap, and the power plant should meet the demands of residential electricity, industrial electricity, and so on.
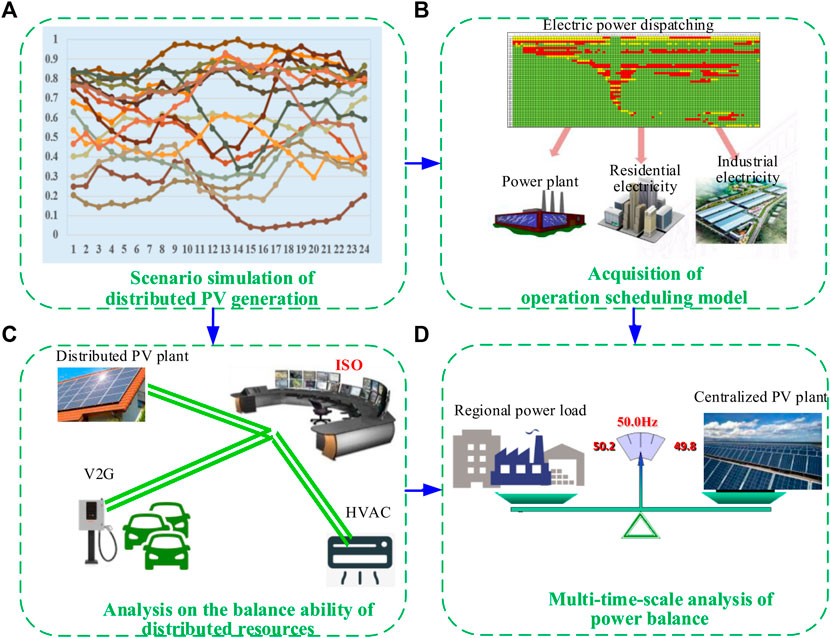
FIGURE 1. Framework for multi-time-scale power balance considering centralized PV generation. (A) Scenario simulation of distributed PV generation. (B) Acquisition of operation scheduling model. (C) Analysis on the balance ability of distributed resources. (D) Multi-time-scale analysis of power balance.
The day-ahead balance scheme mainly provides support for economic operation. Relying on the day-ahead prediction data, the day-ahead generation schedules are given. The day-ahead balance scheme is passed to the intraday balance analysis, and the day-ahead balance scheme is further adjusted according to the ultra-short-term PV generation forecast to improve both economy and security. The real-time balancing scheme takes security as the sole purpose and provides an absolutely safe balancing scheme for real-time scheduling.
Key Technologies
We address three key technologies for multi-time-scale power balance considering coordination between distributed and centralized PV power generation. 1) It is generally accepted that energy storage technology offers significant benefits for the multi-time-scale power balance in a power system with a high proportion of PV power generation. However, the project-oriented economic performance is a difficult one to improve the potential for the expansion of PV plus storage systems. We can assume that regardless of the project-oriented economic performance, no one can deny that energy storage is the most effective way to deal with the uncertainty in PV power. 2) There is thereby an urgent need but it is still a significant challenge to accurately predict the power generation amount from PV for integration with power systems. The forecast results of PV power for the next day can never be completely right, no matter how good the algorithm is. However, an accurate PV power generation prediction will significantly reduce the percentage of reserve capacity or the amount of PV power generation abandoned. 3) Optimization under uncertainty is critical for the analysis of power balance considering coordination between distributed and centralized PV power generation. In terms of deterministic generation and demand, power balance surplus is deterministic. In terms of the power grid with high penetration PV power generation, power balance surplus is uncertain due to the volatility and randomness of the PV power generation, and this was such a big challenge for power balance measures. When the power balance measures are too conservative, the economic performance of the economic dispatch scheme for the power balance will be greatly reduced. On the contrary, power balance measures with better economic performance often bring security risks to the safe operation of energy networks with high proportion of PV power generation. Therefore, the power balance scheme must balance a trade-off between the security level and economic performance, and stochastic programming provides a framework to obtain the optimal balance scheme.
Discussion
Difference Analysis
The existing literature talks more about distributed and centralized PV power generation and less about centralized PV power generation. Therefore, we are here to discuss the difference between distributed and centralized PV power generation. First, distributed and centralized PV plants are under different grid voltage conditions. Usually, 380 V is regarded as the grid-connected voltage of distributed PV plants, and the specific grid-connected voltage of a centralized PV power generation shall be 35 or 110 kV. In terms of a PV central power station above 30 MW, a main transformer is necessary to connect 110 kV transmission lines. Second, a centralized PV power generation has a long distance power transmission, while the distributed PV power generation is consumed by local demands. Third, distributed and centralized PV plants have different requirements in electricity power generation. The number of equipment for centralized PV generation is greater than that of distributed PV generation. The primary equipment for a centralized PV generation should include a station transformer, switch cabinets, various mutual inductors, arc suppression coil, and main transformer. The secondary equipment for a centralized PV generation should include microcomputer protection, watt hour meters, and dispatch screen. In terms of a distributed PV power station, a wall-mounted solar inverter is necessary and its size is small. Finally, the environmental factors of the installation site also make characteristics of distributed and centralized PV power vary greatly.
Difficulty Analysis
The centralized PV power generation model and regional dispatching model are easy to obtain, and these models are accurate. The difficulty of power balance analysis is that distributed PV power generation is difficult to predict accurately. The real problem is not only the difficulty of modeling distributed PV power, but also the lack of historical data and even the lack of power data. The variability of micro meteorology makes a single distributed PV power generation uncertain. The overall impact of all distributed PV plants in a region poses a great challenge to the power balance, and the result is that the local utility should use more controllable resources to deal with uncertainty. In view of the aforementioned difficulties, the authors put forward a feasible scheme. First, the region is divided into small spaces, and the models of distributed PV power generation in different spaces are established respectively. Second, the installation of distributed PV measuring devices does not need to achieve full coverage of each household, but we can select typical households for the deployment of measuring devices in each space. Advanced machine learning algorithms can be used to simulate distributed PV data of those without a measuring device, and the inputs of machine learning algorithms are weather data and PV power generation data of neighbors having installed with measuring devices. The proposed method can greatly reduce the purchase cost of PV measuring devices. Third, the comprehensive impacts of time, space, and weather are considered to help make short-term and ultra-short-term predictions of a distributed PV power generation in a space. Finally, a power flow model based on the spatiotemporal correlation theory can be built to analyze the overall impact of distributed PV power generation in different regions on the power balance of the whole region.
Conclusion
With the high proportion of distributed PV generation access, the traditional centralized energy management and scheduling operation will inevitably face many new problems, such as high communication cost, low optimization efficiency, frequent changes in operating conditions, and so on. Coordinated control of a large number of uncontrollable distributed PV generations will significantly increase the difficulty and safety risk of power grid dispatch. Through the multi-time-scale analysis of power balance considering coordination between distributed and centralized PV power generation, it is conducive to fully tap the safety and stability to support the ability of PV power generation to the power grid. Establishing a multi-time-scale dynamic aggregation model of distributed PV power generation in the whole region is the key to realize the power balance analysis under different operation scenarios. Power balance analysis provides a decision-making basis for day-ahead scheduling and real-time dispatching.
Author Contributions
All authors listed have made a substantial, direct, and intellectual contribution to the work and approved it for publication.
Funding
This study was supported by the Science and Technology Foundation of SGCC (SGJSCZ00KJJS2102057).
Conflict of Interest
WK was employed by State Grid Energy Research Institute Co., Ltd.
YG and HZ were employed by Changzhou Power Supply Company of State Grid Jiangsu Electric power Co., Ltd.
The remaining authors declare that the research was conducted in the absence of any commercial or financial relationships that could be construed as a potential conflict of interest.
Publisher’s Note
All claims expressed in this article are solely those of the authors and do not necessarily represent those of their affiliated organizations, or those of the publisher, the editors, and the reviewers. Any product that may be evaluated in this article, or claim that may be made by its manufacturer, is not guaranteed or endorsed by the publisher.
References
Awadhi, N. A., and Moursi, M. S. E. (2017). A Novel Centralized PV Power Plant Controller for Reducing the Voltage Unbalance Factor at Transmission Level Interconnection. IEEE Trans. Energ. Convers. 32 (1), 233–243. doi:10.1109/TEC.2016.2620477
Eftekharnejad, S., Heydt, G. T., and Vittal, V. (2015). Optimal Generation Dispatch with High Penetration of Photovoltaic Generation. IEEE Trans. Sustain. Energ. 6 (3), 1013–1020. doi:10.1109/TSTE.2014.2327122
Emmanuel, M., Rayudu, R., and Welch, I. (2017). Impacts of Power Factor Control Schemes in Time Series Power Flow Analysis for Centralized PV Plants Using Wavelet Variability Model. IEEE Trans. Ind. Inf. 13 (6), 3185–3194. doi:10.1109/TII.2017.2702183
Fu, X., Chen, H., Cai, R., and Yang, P. (2015). Optimal Allocation and Adaptive VAR Control of PV-DG in Distribution Networks. Appl. Energ. 137, 173–182. doi:10.1016/j.apenergy.2014.10.012
Fu, X., Fu, Q., and Tang, W. (2019). Grid Connection Technique Based on μ Theory for a Two‐stage PV Structure. IET Power Elect. 12 (6), 1545–1553. doi:10.1049/iet-pel.2018.5941
Fu, X., Guo, Q., and Sun, H. (2020). Statistical Machine Learning Model for Stochastic Optimal Planning of Distribution Networks Considering a Dynamic Correlation and Dimension Reduction. IEEE Trans. Smart Grid 11 (4), 2904–2917. doi:10.1109/TSG.2020.2974021
Fu, X. (2022). Statistical Machine Learning Model for Capacitor Planning Considering Uncertainties in Photovoltaic Power. Prot. Control. Mod. Power Syst. 1, 51–63. doi:10.1186/s41601-022-00228-z
Hao, Y., Su, D., and Lei, Z. (2021). A Bi-level Control Strategy for PV-BES System Aiming at the Minimum Operation Cost of BES. Front. Energ. Res. 9, 785420. doi:10.3389/fenrg.2021.785420
Li, D., Li, J., and Wang, N. (2021). A Novel Technique Based on Peafowl Optimization Algorithm for Maximum Power Point Tracking of PV Systems under Partial Shading Condition. Front. Energ. Res. 9, 801571. doi:10.3389/fenrg.2021.801571
Vithayasrichareon, P., Mills, G., and MacGill, I. F. (2015). Impact of Electric Vehicles and Solar PV on Future Generation Portfolio Investment. IEEE Trans. Sustain. Energ. 6 (3), 899–908. doi:10.1109/TSTE.2015.2418338
Zhang, K., Zhou, B., Or, S. W., Li, C., Chung, C. Y., and Voropai, N. (2022). Optimal Coordinated Control of Multi-Renewable-To-Hydrogen Production System for Hydrogen Fueling Stations. IEEE Trans. Ind. Applicat. 58 (2), 2728–2739. doi:10.1109/TIA.2021.3093841
Keywords: distributed generation, centralized electricity generation, photovoltaic module, balance of power, renewable energy
Citation: Wang J, Yu Z, Kong W, Gao Y, Zhang H and Fu X (2022) Multi-Time-Scale Analysis of Power Balance Considering Coordination Between Distributed and Centralized PV Power Generation. Front. Energy Res. 10:902779. doi: 10.3389/fenrg.2022.902779
Received: 23 March 2022; Accepted: 30 March 2022;
Published: 27 April 2022.
Edited by:
Bin Zhou, Hunan University, ChinaReviewed by:
Yan Zhang, Guangdong University of Finance and Economics, ChinaWolong Yang, China Power Engineering Consulting Group, China
Copyright © 2022 Wang, Yu, Kong, Gao, Zhang and Fu. This is an open-access article distributed under the terms of the Creative Commons Attribution License (CC BY). The use, distribution or reproduction in other forums is permitted, provided the original author(s) and the copyright owner(s) are credited and that the original publication in this journal is cited, in accordance with accepted academic practice. No use, distribution or reproduction is permitted which does not comply with these terms.
*Correspondence: Xueqian Fu, ZnV4dWVxaWFuQHRzaW5naHVhLmVkdS5jbg==