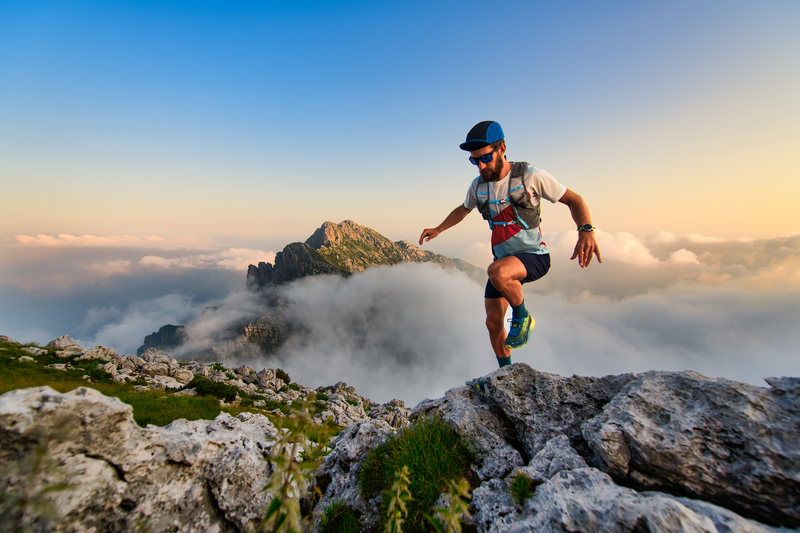
95% of researchers rate our articles as excellent or good
Learn more about the work of our research integrity team to safeguard the quality of each article we publish.
Find out more
OPINION article
Front. Energy Res. , 23 May 2022
Sec. Process and Energy Systems Engineering
Volume 10 - 2022 | https://doi.org/10.3389/fenrg.2022.901906
This article is part of the Research Topic Advanced Technologies for Planning and Operation of Prosumer Energy Systems View all 111 articles
A power grid enterprise, as often as not, which is closely related to economic development, is the core enterprise supporting regional economic development. In the field of infrastructure planning of a smart grid, the study of the excavation of development characteristics consolidates the theoretical basis for the formulation of distinguished source-network-load-storage cost-sharing mechanisms for power grids at different development levels (Hall and Foxon, 2014; Li et al., 2021). The existing models and standards of appraisal which are incommensurate to the key technical characteristics of the backbone planning framework of a smart grid with a reliable grid connection of a high percentage of renewable energy and power electronic equipment application, therefore, are in urgent need of being improved correspondingly (Falahati et al., 2012; Munshi and Mohamed, 2017). This paper proposes a multi-closed-loop assessment mechanism for the development level of smart grids with the growth potential of power demand, economic growth potential, and growth potential of renewable energy penetration as error correction procedures. Furthermore, some opinions on the existing assessment methods are put forward. Based on the result of assessment, some suggestions for distinguished infrastructure planning goals of a smart grid at disparate development levels are presented (Wang et al., 2014).
Considering the “periodical” and “saturated” characteristics during the evolution process of a smart grid, this paper forms a multi-closed-loop assessment mechanism for the development level of smart grids. The key contributions of this opinion are two-fold:
1) A multi-closed-loop assessment mechanism for the development level of smart grids is formulated. Data analysis methods such as correlation analysis of the time difference and a machine learning algorithm such as principal component analysis (PCA) are applied for an indicator which can comprehensively, objectively, and accurately evaluate the current development level of the power grid. The generalized logistic growth curve with excellent adaptability to data is applied to excavate the time sequence characteristics of smart grid development and clarify infrastructure planning objectives for each development level.
2) A calibration model of the multi-closed-loop assessment mechanism is proposed. Firstly, the general situation of the regional power grid and economic development is investigated to sort out regional energy endowment and a future development plan. Then, based on the infrastructure planning goals of the smart grid and considering the growth potential of power demand, regional economy, and new energy access, the calibration model of the assessment mechanism is constructed to verify the assessment results of the development level of the smart grid and improve the accuracy of smart grid development trend prediction.
At present, most researchers evaluate the single attribute of a smart grid from the aspects of power grid economy, power grid development coordination, and power grid reliability. Time-sequential simulation was applied to analyzing the characteristics of curtailment and trend of power system in a previous work (Jia et al., 2019). The operation reliability under the influence of the sequential characteristics of austerity and shifting of a flexible reserve provider is evaluated. The sustainable evolution ability of the smart grid from the aspects of environmental protection and resource development level was evaluated in another study (Li et al., 2007), and took the sustainable development ability as a key indicator in which the development level of the power grid is incarnated. The research on the single attribute assessment of the power grid has a relatively thorough evaluation model and system, but it restrictedly concentrates on reflecting the development level of a certain aspect of the smart grid, lacks the comprehensive evaluation indicator, and the evaluation results lack objectivity and comprehensiveness, which does not provide high guidance for the goal of smart grid infrastructure planning.
The multi-attribute comprehensive assessment methods need to select indicators highly related to the main business of the power grid. Generally speaking, these indicators have standardized statistical caliber, collection methods, and recording methods. The power grid enterprise has built a long-term data accumulation mechanism and data repository to further enhance the vertical and horizontal comparability of the same indicator data. Meanwhile, dynamic indicators have higher reference value, generally speaking, when solving infrastructure planning problems. An evaluation mechanism for the coordinated development of a renewable-dominated power grid considering the three dimensions of a power system, transmission, distribution, and power dispatching, was proposed in an earlier study (Dai et al., 2011). The comprehensive evaluation index system and method of a power grid considering the combination of macro and micro perspectives of medium and low voltage electrical networks was formulated in a previous work (Song et al., 2014). However, the variational process of smart grid characteristics in a dynamic environment with a high renewable energy penetration rate has not been studied. At present, most research findings on the multi-attribute assessment of a power grid can merely present the development status of the power grid statically which results in the lack of dynamic indicators with high timeliness in the model structures (Niu et al., 2013).
In this paper, the highly accessible annual per capita electricity consumption is taken as the benchmark indicator, which excludes the influence of demographic change, has strong comparability, and skyscraping correlation with the main business of the power grid. Based on data analysis methods such as the correlation analysis of the time difference, the consistent indicators matching splendidly with the basic cycle of electricity consumption in the multi-dimensional attribute of the power grid is explored. In the field of machine learning, dimensionality reduction algorithms such as principal component analysis algorithms are applied for the purpose of reducing data dimensions and maximizing the retention of information from original data so as to provide accurate quantitative indicators for smart grid development trend prediction.
The logistic growth model is diffusely applied to researching the field of the growth process of social economy, business, science, and technology. The logistic growth model was used to predict urban saturation load in a previous work (Wang and Fang, 2012), and the calculation methods of each sub-model and boundary parameters are confirmed. In addition, from the perspective of life cycle, the identification model of development level of industrial parks based on the logistic growth model was structured and gave suggestions in an earlier study (Wang and Zhang, 2021). The current research achievements based on the logistic growth model universally lack the verification procedure for the development potential of the object of study when exploring the law of development. The logistic growth model, meanwhile, lacks a sufficient correction margin due to poor adaptability to data. By contrast, the generalized logistic growth model introduces the shape factor, which makes the model reflect the actual growth process of a power grid more accurately and objectively by adjusting the shape factor according to the variation trend of data.
This paper proposes a multi-closed-loop assessment mechanism for the development level of smart grids considering growth potential in electricity demand, regional economy, and renewable energy penetration. In the calibration sub-model, the power consumption elasticity coefficient and the growth rate of renewable energy penetration rate are used to determine the respective weights of power demand growth potential, economic growth potential, and renewable energy access growth potential. Parameter sensitivity analysis is required in the selection of error correction procedures, which is adjusted according to the physical truth. Furthermore, the subjective and objective combination weighting method is adopted to evaluate the growth potential of the three factors, and the results are matched with the partition of the acceleration curve. Iterative matching can be achieved by adjusting the saturation predicted value of the consistent index and benchmark index in the case of mismatching results. Input parameters should be adjusted within the adjacent range of the open-loop input parameters, and kept the same order of magnitude with the original parameters as far as possible. In addition, the physical significance of the model should be taken into consideration to reflect the adjustment potential of parameters practically. The multi-closed-loop assessment mechanism for the development level of smart grids is presented in Figure 1.
At the initial development level, the structure of a smart grid will inevitably experience a process from scratch. At this time, the principal feature of the smart grid is the famine of capital, talents, and infrastructure, and the development space is relatively broad and the development speed is relatively slow. Therefore, combined with the advantages of regional development, considering the economic development conditions and regional development policies, the infrastructure projects of a power grid are vigorously promoted to expedite the evolution of grid structure to the rapid development level. At the benefit exploration phase, the infrastructure construction of a power grid presents explosive growth, and management technology and operation efficiency should be improved, so as to form a virtuous cycle with positive feedback characteristics between the advancement of the smart grid and regional economic development. At the undersaturated development level, the high percentage of renewable energy access and the extensive use of power electronic devices make the security and stability of the power grid become one of the core topics concerned by smart grid planning. It is necessary to constantly stimulate the innovation of the power grid in technology, management, service, and market. At the saturation development level, the risk of disconnection of the source network is in pressing need of researchers’ concern. At the same time, the barriers of inter-regional electricity trading should be broken, and the trans-regional electricity market trading system should be improved, so as to achieve the optimal allocation of electricity resources and promote the high-quality development of the economy (Zhang et al., 2010; Erdiwansyah et al., 2021).
When facing a period of energy revolution, a high percentage of renewable energy connected to the smart grid is the strategic technology leading this revolution. Sticking with the consumption of renewable energy, as a matter of course, has become an inevitable problem. For the purpose of effective adaptation of centralized and distributed access, a smart grid with high toughness and high flexibility is a feasible option. Of course, the next thing issue would be the requirement of additional investment. Adhering to the principle of “who benefits, who pays”, therefore, according to distinguished development levels of a smart grid, formulating differentiated schemes to recover the costs triggered by the above investment from the source-network-load-storage is conducive to gradually realizing the fairness of transmission and distribution cost sharing result from access engineering, system flexibility transformation, and auxiliary services. The model proposed in this paper provides a feasible technical scheme for development level assessment for smart grids. With the unremitting advancement of smart grid construction, in the future, the amount of data generated in the field of development level assessment of smart grids will increase exponentially. Researchers should keep an open and optimistic attitude when dealing with advanced technologies such as artificial intelligence and data analysis methods, and constantly explore their effective application in different scenarios of smart grids (Ozay et al., 2016; Hossain et al., 2019; Xiao et al., 2020; Desai and Makwana, 2021; Veeramsetty, 2021; Zhang et al., 2022).
Conceptualization, HX; methodology, HX, ZL, and QY; writing–original draft, HX and AW; writing–review and editing, ZL and ZP.
This work is supported by the Hunan Natural Science Foundation of China under grant (No. 2021JJ10019).
Author ZL was employed by State Grid Zhangjiajie Electric Power Supply Company.
The remaining authors declare that the research was conducted in the absence of any commercial or financial relationships that could be construed as a potential conflict of interest.
All claims expressed in this article are solely those of the authors and do not necessarily represent those of their affiliated organizations, or those of the publisher, the editors and the reviewers. Any product that may be evaluated in this article, or claim that may be made by its manufacturer, is not guaranteed or endorsed by the publisher.
Dai, H., Li, Q., and Wang, X. (2011). Research on the Evaluation Index System for Coordinated Development of New Energy and Smart Grid. Energy Technol. Econ. 5, 18–23. doi:10.3969/j.issn.1003-2355.2011.05.006
Desai, J. P., and Makwana, V. H. (2021). A Novel Out of Step Relaying Algorithm Based on Wavelet Transform and a Deep Learning Machine Model. Prot. Control Mod. Power Syst. 6 (4), 500–511. doi:10.1186/s41601-021-00221-y
Erdiwansyah, E., Taleb, M. A., Husni, H., Nasaruddin, S., and Zaki, M.Muhibbuddin (2021). A Critical Review of the Integration of Renewable Energy Sources with Various Technologies. Prot. Control Mod. Power Syst. 6, 37–54. doi:10.1186/s41601-021-00181-3
Falahati, B., Fu, Y., and Wu, L. (2012). Reliability Assessment of Smart Grid Considering Direct Cyber-Power Interdependencies. IEEE Trans. Smart Grid 3, 1515–1524. doi:10.1109/TSG.2012.2194520
Hall, S., and Foxon, T. J. (2014). Values in the Smart Grid: The Co-evolving Political Economy of Smart Distribution. Energy Policy 74, 600–609. doi:10.1016/j.enpol.2014.08.018
Hossain, E., Khan, I., Un-Noor, F., Sikander, S. S., and Sunny, M. S. H. (2019). Application of Big Data and Machine Learning in Smart Grid, and Associated Security Concerns: A Review. IEEE Access 7, 13960–13988. doi:10.1109/ACCESS.2019.2894819
Jia, H., Ding, Y., Song, Y., Singh, C., and Li, M. (2019). Operating Reliability Evaluation of Power Systems Considering Flexible Reserve Provider in Demand Side. IEEE Trans. Smart Grid 10, 3452–3464. doi:10.1109/TSG.2018.2827670
Li, L., Yu, L., Xu-bo, G., Fu-cheng, C., and Zhong-fu, T. (2007). The Index System and Evaluation Method on the Sustainable Development of Power Grid Company. J. Electr. Power 1, 1–4. https://qikan.cqvip.com/Qikan/Article/Detail?id=24520190&from=Qikan_Search_Index.
Li, N., Hakvoort, R. A., and Lukszo, Z. (2021). Cost Allocation in Integrated Community Energy Systems - A Review. Renew. Sustain. Energy Rev. 144, 111001. doi:10.1016/j.rser.2021.111001
Munshi, A. A., and Mohamed, Y. A.-R. I. (2017). Big Data Framework for Analytics in Smart Grids. Electr. Power Syst. Res. 151, 369–380. doi:10.1016/j.epsr.2017.06.006
Niu, D. X., Liu, J. P., Liu, T., and Guo, H. C. (2013). Comprehensive Evaluation of Regional Grid Development Level of China under the Smart Grid Construction. Appl. Mech. Mater. 300-301, 640–644. doi:10.4028/www.scientific.net/amm.300-301.640
Ozay, M., Esnaola, I., Yarman Vural, F. T., Kulkarni, S. R., and Poor, H. V. (2016). Machine Learning Methods for Attack Detection in the Smart Grid. IEEE Trans. Neural Netw. Learn. Syst. 27 (8), 1773–1786. doi:10.1109/TNNLS.2015.2404803
Song, Y. W., Liu, J., Ning, L. Y., Han, Z. T., Liu, H., and Wang, S. J. (2014). Comprehensive Assessment System and Method of Smart Distribution Grid. Adv. Mat. Res. 860-863, 1901–1908. doi:10.4028/www.scientific.net/AMR.860-863.1901
Veeramsetty, V. (2021). Shapley Value Cooperative Game Theory-Based Locational Marginal Price Computation for Loss and Emission Reduction. Prot. Control Mod. Power Syst. 6 (4), 419–429. doi:10.1186/s41601-021-00211-0
Wang, C., Li, X., Liu, Y., and Wang, H. (2014). “The Research on Development Direction and Points in IoT in China Power Grid,” in 2014 International Conference on Information Science, Electronics and Electrical Engineering, Sapporo, Japan (Sapporo, Japan: IEEE), 245–248. doi:10.1109/InfoSEEE.2014.6948106
Wang, J., and Zhang, L. (2021). Identification of Development Stage of Industrial Parks Based on Logistic Curve. J. Shenyang Univ. Technol. 14, 242–250. doi:10.7688/j.issn.1674-0823.2021.03.08
Wang, W., and Fang, T. (2012). The Application of Per-Person Electricity Consumption Method in Saturation Load Forecasting. Power Demand Side Manag. 1, 21–23. doi:10.3969/j.issn.1009-1831.2012.01.008
Xiao, Z., Wang, J., Yang, X., Xiao, Y., Liu, H., Wu, Y., et al. (2020). Research on the Medium and Long Term Development Framework of Smart Grid under the Background of Energy Internet. Procedia Comput. Sci. 175, 769–773. doi:10.1016/j.procs.2020.07.114
Zhang, K., Zhou, B., Or, S. W., Li, C., Chung, C. Y., and Voropai, N. (2022). Optimal Coordinated Control of Multi-Renewable-To-Hydrogen Production System for Hydrogen Fueling Stations. IEEE Trans. Ind. Appl. 58 (2), 2728–2739. doi:10.1109/TIA.2021.3093841
Keywords: smart grid, renewable energy, generalized logistic growth model, dimension reduction algorithm, cost sharing
Citation: Xie H, Li Z, Yang Q, Peng Z and Wu A (2022) A Multi-Closed-Loop Assessment Mechanism for Development Level of Smart Grids. Front. Energy Res. 10:901906. doi: 10.3389/fenrg.2022.901906
Received: 22 March 2022; Accepted: 11 April 2022;
Published: 23 May 2022.
Edited by:
Jian Zhao, Shanghai University of Electric Power, ChinaReviewed by:
Xueqian Fu, China Agricultural University, ChinaCopyright © 2022 Xie, Li, Yang, Peng and Wu. This is an open-access article distributed under the terms of the Creative Commons Attribution License (CC BY). The use, distribution or reproduction in other forums is permitted, provided the original author(s) and the copyright owner(s) are credited and that the original publication in this journal is cited, in accordance with accepted academic practice. No use, distribution or reproduction is permitted which does not comply with these terms.
*Correspondence: Zhenqiu Li, Mjg0NjcwODAyQHFxLmNvbQ==; Haodong Xie, MTc0MzQ2NTU5NkBxcS5jb20=
Disclaimer: All claims expressed in this article are solely those of the authors and do not necessarily represent those of their affiliated organizations, or those of the publisher, the editors and the reviewers. Any product that may be evaluated in this article or claim that may be made by its manufacturer is not guaranteed or endorsed by the publisher.
Research integrity at Frontiers
Learn more about the work of our research integrity team to safeguard the quality of each article we publish.