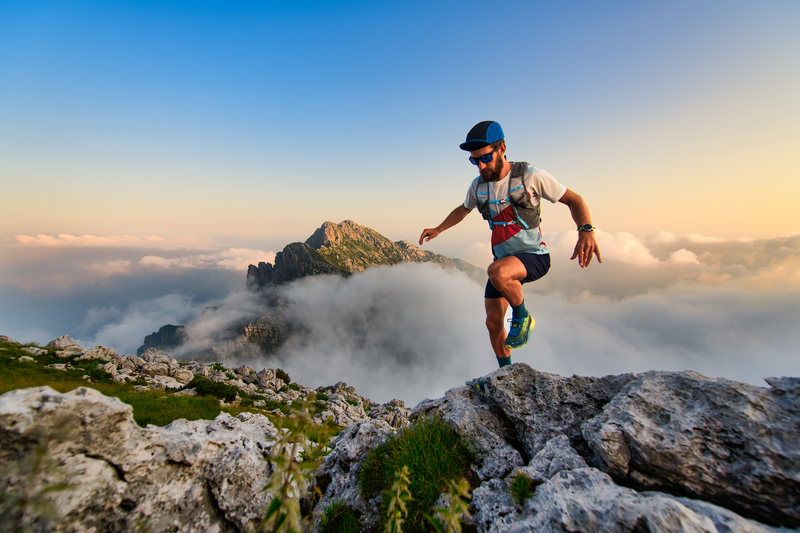
95% of researchers rate our articles as excellent or good
Learn more about the work of our research integrity team to safeguard the quality of each article we publish.
Find out more
ORIGINAL RESEARCH article
Front. Energy Res. , 18 May 2022
Sec. Smart Grids
Volume 10 - 2022 | https://doi.org/10.3389/fenrg.2022.861571
This article is part of the Research Topic Advanced AI Applications for Modelling, Optimization, Control, and Planning of Smart Grid View all 39 articles
The purpose of this research is to provide power grid energy efficiency solutions. In this paper, a comprehensive review and its optimal solution is proposed considering the various challenges of smart grid demand-side management. The main technique is based on a novel idea in the Smart Grid—demand response optimization which enables autonomous energy management on the demand side for a wide variety of customers. The first section of this research examines the smart grid issue and evaluates the state-of-the-art load management techniques in terms of the work’s scope. The demand-side load management architecture consists of three primary levels, two of them in line planning and low-cost scheduling, while the third layer, demand response which is a significant expansion of this domain. The implementation of the proposed architecture in MATLAB/Simulink, with test results. demonstrating the significance of the proposed solution
This work aims to optimize the smart grid demand side, a development technology that influences the electrical grid structure by combining contemporary communications technologies. Coal and nuclear power generation in several EU and US countries [2011 Commission), Simon and Belles (2009)] provide the bulk, but regulatory and grid exchange equates to high-speed absorption (Reddy and Manohar, 2018). Increased global energy use, fossil fuel speculation, and global warming have all contributed to a surge in interest in renewable energy during the last 2 decades. (Khan et al., 2017; Kotsampopoulos et al., 2019). However, energy sources such as wind and solar energy have inherent instability that might compromise the stability of the system by accounting for a sizable portion of overall output. To ensure energy supply and distribution in the twenty-first century, scientists and several businesses are working to modernize power grid resources and network technology. The utilities, transportation, and distribution businesses, consumers, equipment manufacturers, service providers, and power traders are all significant stakeholders in the electrical systems business. (Gunduz and Das, 2019; Hossain et al., 2019). Solar and wind generation are not yet substantial enough to ensure network stability, but structural and technical changes will be required over the next decade if governments pursue green energy programs (Majzoobi and Khodaei, 2016; Shapsough et al., 2016).
This work seeks to provide an innovative vision of smart grid usage and energy management as a tool to assist choices on sustainable energy and energy market expenditure. The study’s goal is to tackle the requirement of optimization challenges and provide an independent architecture of the system for this purpose. This study adds to the harmonization of numerous planning and optimization approaches to benefit from the time-scale separation of home energy demand. In this scenario, the architecture is layered, with distinct time scales and regulations in each module. The system consists of three primary layers, which proportionally address runtime, optimal scheduling and demand for energy trading. In this manner, energy requirements may be regulated and the environment flexible, while remaining optimistic.
The blackout of 14 August 2003, with an estimated $7–10 billion in consumer consequences in the North-East United States and certain areas of Canada, was a significant incident that highlighted worries about the stability of North American power networks. The U.S. government recognized at that time the need and need to update domestic energy infrastructure and policies (Majzoobi and Khodaei, 2016; Meraj et al., 2016; Wang et al., 2016). With the expansion of distributed power generation and the large proportion of renewable resources, existing grids catering to the needs of the market based on centralized carbon production are faced with several challenges like increasing the energy transit and quality while lowering carbon emissions.
Furthermore, user involvement in the electricity markets, incorporation through standardization and inter-operability of newer technologies, a high level of stability, and capital investment in so many European Union Member States are all important factors contributing to the formation of Smart Grids in Europe (Abd et al., 2020). Although updating the entire grid can be expensive, previous accomplishments in this sector have already demonstrated its benefits. Energy can now be produced and consumed within a single area of the grid, for example, thanks to the integration of distributed generation (DG) and removable energy sources (RES), allowing utilities to provide power in the event of higher demand without improving centralized production or growing transmission capacity (Sgouras et al., 2014; Hu et al., 2015; Xie et al., 2019). Nonetheless, enterprises must develop toward a new grids design, behind which there are a plethora of various conceivable advancements on both the hardware and software levels to integrate technologies such as DG, RES, and PHEVs to enable energy conservation in the next decades (Javed and Muqeet, 2021). The intelligent grid is a futuristic idea of power infrastructure. describes Smart Grid functionally as a “electrical network that integrates all the consumers and manufacturers’ actions for efficient, sustainable and safe distribution of electricity.” In the same context, Schneider Electric describes the Smart Grid as “an electrical network that intelligently integrates the behaviour of all users linked to it - generators, consumers and both - to supply sustainable, economic and secure supplies of electricity effectively.” A CISCO business case study is given more weight. (Navilgone and Thesis, 2008; Wang and Lu, 2013; Yang et al., 2020). The smart grid is described as “an integrated view using the information network to improve the operation of the energy grid.” The Power Systems Perception is an electric grid that incorporates energy production, transmission, and distribution to meet the needs of customers. (Zhang et al., 2011; Sindhuja and Lalitha, 2016; RAMADHAN et al., 2017).
The functioning of such a system is enabled from the Information System View, using a communication architecture that connects everything from all over the grid. To make this integration effective and efficient, control mechanisms at all levels of the grid are required. The “Control System View,” in which the Smart Grid might be considered a system of systems, complements the power and information views (Liang et al., 2013; Tan et al., 2017; Gunduz and Das, 2020). In keeping with this viewpoint, J. McDonald emphasized that the Smart Grid is fundamentally a control challenge, which includes (Scholar et al., 2016; Zhang et al., 2017; Zolfaghari et al., 2019): Improvements in the power system delivery; requirement of the optimization.
Changing demand to match supply is one method for improving solar and wind supply. Such techniques necessitate customer-to-service communication as well as customer-side commuting capabilities. In this regard, building automation; Smart measurement are two fundamental technologies that enable demand-side load optimization.
Smart energy shipment among Smart Grid customers would immediately deploy smart meters and benefit from an optimal energy system at home. (capable of managing devices and doing cost-cutting above-the-line activities). Power pricing, renewable power options, CO2 management, and use pattern observation are just a few of the numerous building automation applications that may be explored. District and energy buildings will be created with the help of distributed generation (solar, wind, biomass, geothermal, cogeneration) and storage (batteries, fuels, PHEVs, compressed air) (L Cui L et al., 2020; Goldsmith et al., 2009; Syarif et al., 2016; Yan et al., 2012).
Setting up demand to balance out the load factor during peak hours can significantly improve efficiency in power grids and minimize operating expenses. The demand/response technique, in which energy prices are dynamic and consumers may alter demand based on supply conditions, is one of the aspects of smart grid-to-home management technology. Since the latter point has been well researched in the literature. In (Brandstetter et al., 2015; Rawat and Bajracharya, 2015; Minhas and Member, 2016; Wang et al., 2019) authors provides a comprehensive list of sources. At a D/R market-clearing price, the energy supply is inelastic, and the utility manages the peak formation system using a supply offering scheme. Essentially, each client sends a supply function to the corporation, which determines the energy price based on consumer offers. The consumer is therefore priced and pledges to shed or increase his use according to his offer and the energy price (Yang et al., 2011; Shinkhede, 2014; Brandstetter et al., 2015; Dias, 2018). This latter research reveals that a global balance that maximizes social welfare is reached in a price-taking market. Conversely, according to (Babar et al., 2020; J.O. Petinrin and M. Shaaban, 2015; Peng et al., 2019), “the system achieves a Nash balance three unique in an oligopoly, which enables customers to optimize another complementary and global objective function.” A distributed D/R framework with user flexibility is provided (Dari and Essaaidi, 2016; Sanjab et al., 2016; Gunduz and Das, 2020). A system like this is based on the proportionately fair price (PFP) of [13] [41], and [42], which asserts that each user declares the price of his/her flow willing to be paid per unit. Those who pay more will obtain more capacity under this arrangement (Kreikebaum et al., 2010; Adhikari et al., 2017; Hong and De León, 2017). This technique is well suited to the DSM architecture outlined in this work since power prices should be flexible for both utilities and customers. The aforementioned Demand/Answer scheme involves two-way communication between clients and the utilities. However, the establishment of an AMI is a work in which only on the hypothesis of active client engagement, costs can be justified.
Since the early 1990s, researchers have been studying demand-side load management. Wacks presented the general idea of demand side load management for altering energy demand/offer balance in (Monteiro et al., 2011; Miura and Wu, 2014; Lotfi et al., 2016; Jiang, 2019). To that purpose, the energy services have developed three types of load control systems: local control, direct control, and distributed control. It should be noted that they all require real-time access to utility information, in-house computer intelligence, home automation, and power-saving devices. Local control includes voluntary consumer cooperation to cut load peaks by considering various energy rates, depending on daylight (Riaz et al., 2016; Schaer et al., 2016; Eba et al., 2020). Customers who use a lot of electricity but don’t need it right now are advised to modify the price over time. Although this strategy is inexpensive and simple to implement, it may have limited success since consumers seldom understand each device’s kilowatt-hour use and associated cost, limiting their ability to operate their options efficiently (Ennaji and Boulmalf, 2009; Dohn, 2011; Hu et al., 2020; Rose et al., 2020). The remote switching of forced devices is the foundation of direct control. Following the acquisition of financial incentives, customers can install remotely controlled switches in their home systems that, if necessary, manage the load by disconnecting selected appliances (Muqeet et al., 2019). This implies that the air conditioning is turned on and off dependent on the outside temperature, the time of day, and the utility demands (Motoyama et al., 2014; Andreasson et al., 2019; Hasan et al., 2020; Kirakosyan et al., 2020). Similarly, the water heater would be restricted from operating during the hottest hours of the day, for example. Decentralized control is a hybrid approach that relies on customer involvement and service communication. The utility has the ability to change energy costs in real time based on the energy market and system load, but the user must modify its consumption based on its own judgments. Home automation plays a key part in this scenario (Meier et al., 2016; Mishra and Tiwari, 2017; Stellios et al., 2018; Ullah et al., 2020). For example, a dishwasher like the HEM (Home Energy Manager) can provide the user the option of running the cycle on demand or transferring it to economic benefit for specific periods of time. Wacks’ piece concludes by highlighting how important home automation is for controlling the electrical demand, and how smart gadgets should be constructed accordingly. This 1991 paper addresses the key principles that led to smart homes and intelligent grids today (Ye et al., 2005; Firouzi et al., 2017; Pan and Yang, 2018; Shahab et al., 2021).
Energy use profiling may be one of the most appealing aspects for consumers and utilities once the connection between appliances and home energy management is established. (N Cui N et al., 2020; Guerrero et al., 2013; Pérez-Guzmán et al., 2018). In actuality, such information would assist consumers in better planning their household activities in light of rising energy expenditures. It would be extremely beneficial in optimizing energy dispatch on the utility side. Since the late 1970s, this has been one of the most researched topics in energy management. There are many references in this topic and this problem seems to have been examined using totally various methodologies, capable of illuminating different features and providing solutions accordingly. Consumption of buildings can be separated into electricity and thermal energy. As mentioned in (Kreikebaum et al., 2010; Khan et al., 2017; Kotsampopoulos et al., 2019), the forecasting process can be based on top-down or bottom-up methodologies. The first technique leverages data from energy providers on regional consumption to regard users as energy sinks, whereas the second approach uses information from the user level and advances in the modelling process to match energy suppliers to aggregate data. Because historical data has been combined with macroeconomic indicators (income, oil price, etc.), peace in technological progress, and climate, the top-down technique does not imply that single user consumption cannot be split and anticipated. The simplicity of this approach, which just requires aggregate data that is widely available, is its benefit. Furthermore, past data give the model some “inertia.” We consider the inability to capture technology or climatic information to be more inconvenient than the inability to infer particular user information. Despite this, this technique provides reasonable forecasts for long-term energy demand across vast regions. Bottom-up approaches, including statistical and technological procedures, appear to be more feasible (Meraj et al., 2016; Wang et al., 2016; Gunduz and Das, 2019; Hossain et al., 2019). These approaches employ data from individual end-users, groups of homes, or communities to extrapolate a model for a whole area or even a country depending on the representativeness of consumer groups or sub-groups used throughout the modelling process. Statistics and engineering approaches are used in the bottom-up approach. Statistical models use historical data to relate household energy use to specific end applications and employ various regression approaches. Once a link between end uses and energy consumption has been established, the model is used to estimate home energy consumption. Among the s are regression, conditional demand analysis, and neural networks. Instead, engineering approaches aim to forecast household thermal energy consumption, equipment consumption profiles (together with market penetration statistics for the most common goods), and household behaviour (Navilgone and Thesis, 2008; Wang and Lu, 2013; Motoyama et al., 2014; Hu et al., 2020). Distributions, archetypes and samples are among the most important ways of engineering. The archetypal approach entails categorizing homes by era, size, style of residence, and so on. Then data and features can be aggregated on devices to make up the model. The more archetypes accessible, the more precise and realistic the estimate of energy consumption for a certain location may be (Zhang et al., 2011; Sindhuja and Lalitha, 2016; Adhikari et al., 2017; Tan et al., 2017). Because the consumption of each item is available, this present technology appears to be a promising choice for increasing the capabilities of the Home Energy Manager. Residential geometry, presence of equipment and equipment, indoor and outdoor temperature, and occupancy schedules are all common input data for downstream approaches. This level of detail is an important component of the bottom-up method, since it allows for the modelling of technological growth in society. However, because of the irrational behaviour of unmolded families, the bottom-up method may be so exact that the building’s energy requirement is underestimated. This latest issue is the weakness and significant dependence on family behaviour of the engineering approaches (Monteiro et al., 2011; Miura and Wu, 2014; Scholar et al., 2016; Gunduz and Das, 2020). An approach that disaggregates consumption data and categorizes it by device and day type might be useful (weekday, weekend, Sunday, etc.). To that purpose, a predictive model for energy use, based on a statistical study of historical data, can be used for Bayesian inference. 15 consumers 15. The authors provide a behavioural model for household energy use in (Yan et al., 2012; Guerrero et al., 2013; Rawat and Bajracharya, 2015; Zolfaghari et al., 2019). Their approach is more a matter of psychology than of engineering. Your study is however helpful in explaining and interpreting measurement data. A. Capasso presents an intriguing development of the latter approach in (Hu et al., 2020; J.O. Petinrin and M. Shaaban, 2015; Wang et al., 2019; Yang et al., 2011), where a customized bottom-up approach is built The authors integrate statistical and engineering concepts with Monte Carlo consumption simulations to demonstrate how the model can reasonably anticipate the family’s energy demands throughout the day. While this analysis was conducted for the Italian energy market, taking into consideration Italian home habits and equipment ownership, this model may be adapted to other countries provided the required data from surveys. Again, this strategy can easily be combined with a planning approach for energy management at home because HEM can provide information about the use of appliances, statistics and house occupancy information, (Kumar and Bhimasingu, 2015; Dari and Essaaidi, 2016; Peng et al., 2019; Babar et al., 2020), offers a relevant charge pattern based on measurements to guarantee that the user is allocated an acceptable energy pricing. This would result in more equitable energy production, transmission, and distribution rates. The bottom-up strategy is more appealing than the top-down one for achieving this aim, and modelling home habits is required. (Ahshan, 2013; Sanjab et al., 2016; Petrenko and Makoveichuk, 2017; Gunduz and Das, 2020).
The optimum answer to energy management and peak load problems should be Dynamic DSM in the smart grid environment [55]. Minimizing energy costs and reducing peak loads are the key concerns under consideration. For home appliances planning, many optimization-based techniques are available. PSO is one of the HEMS scheduling techniques [56, 57]. In [57], HMES based on PSOs was referred to as the future smart grid The Binary Particle Swarm Algorithm (BPSA) is used for energy management to reduce overall energy expenses while taking into consideration specific limits on the use of electrical devices: power constraints and electrical customers’ personal lifestyle. A working time chart is provided by mathematical calculations for appliances that meet the reduced tariffs and power limits of end-users and providers. The utility and consumers are linked; the utility routinely checks customer demand and requests that appliances be turned off or delayed when consumption exceeds the maximum limit. When demand is low, utilities urge that end customers utilize the devices to shift load. The operation time of the appliances has been controlled, and an ideal timetable has been set using BPSA. 100 end-users with 11 devices and a random operating time were taken into account. (Firouzi et al., 2017; Hong and De León, 2017; Pérez-Guzmán et al., 2018; Kirakosyan et al., 2020).
Considers 3 GA applications in electrical distribution, including network layout for loss reduction, optimal safety disposal, and priority management in distributive network domains. The paper presents preliminary test results obtained through the use of genuine circumstances. (Lotfi et al., 2016; Riaz et al., 2016; Trinklein et al., 2020).
The electrical requirements of Pakistan are going to quadruple by 2050. If adequate resources are not allocated, the country’s energy situation would intensify. An autonomous national energy organization is required to develop and implement long-term strategies for indigenous regenerative resources such as hydroelectricity, coal, nuclear, and renewables (Muqeet et al., 2021). It is also vital to analyze the options available for importing energy from neighbouring nations in order to secure the country’s future. When TAPI and IPI are compared to LNG import possibilities, it is clear that LNG is suited for gas pipelines. It is critical to make the most use of existing thermal power plants and combined cycle power plants. Pakistan has a large potential for renewable energy resources. Circular debt can be reduced by incorporating more renewable energy into the national grid.
With the expanding trend of smart grid implementation, Pakistan’s energy producers are searching for domestic and international investment. This will assist utilities in managing the electricity shortage. The existing electrical market structure is unsuitable for investors due to WAPDA and KESC’s monopolistic posture. The government should encourage private investors to make smart grid contributions (Javed et al., 2021). The regulation reform will make the market more competitive and will thus establish a smart grid and DSM environment for customers to better satisfy their consumers in terms of cost reduction and quality services. In a competitive market, smart grid implementation is considerably easier than in a monopoly market, since potential buyers are able to acquire electricity from several sources. The government should fundamentally reform policies to enhance the competitiveness of the power industry by promoting domestic and foreign investment.
In future energy management systems, DSM is intended to play an essential role. This section gives a full description, comparison and optimization approach for the planning of intelligent home equipment. Dynamic pricing-based energy consumption scheduling (ECS) is highlighted, with peak load reduction and a residential decrease in energy bills by consumers. In addition, the chapter describes domestic energy dynamic pricing, accompanying optimization methodologies and the comparative examination of recent systems. The majority of dynamic energy management systems are built on the assumption that modern information, communication and control infrastructures are available. In general, however, the realization of the smart grid and in particular of successful DSM still confront several hurdles.
Naturally, the energy management work is an optimization issue in which the goal is energy consumption and user comfort, among other things, while energy availability and device-specific needs are regular restrictions. The next sections cover the optimization difficulties together with case studies of certain optimization strategies.
Optimization plays an essential function in smart home planning to smooth the load pro-file and save user costs. The task of energy management comprises several goals and restrictions. In order to tackle these challenges, the researchers highlighted numerous optimization strategies. In the following sections, several relevant strategies have been outlined, followed by a discussion of how these strategies might be effectively applied to energy management concerns. At the end of this chapter also a full comparison of possible optimization approaches is offered.
Knapsack is a combinatorial optimization problem that optimizes a set of pieces, each with a mass and value. The number of items in a collection will be chosen in such a way that the total weight is less than or equal to the stated limit. (Mantovani et al., 2015; Kulkarni and Thorat, 2019; Zardari et al., 2019; Ardabili et al., 2020; Frequency et al., 2020; Hu et al., 2020; Ji et al., 2020; Lin et al., 2020). In other terms, the problem with knapsack is a problem in which several things with varied weights are presented and the one with not more weight is chosen than a fixed one called W. Two kinds of knapsack problems exist: 0-1 Knapsack issue, fractional knapsack issue (Saharia et al., 2016; Wei and Hu, 2018; Riaz et al., 2019; Vigneswari et al., 2019).
If the item is taken first or not (accepted/r ejected), there are no additional alternatives such as OFF (0) or ON, in our situation (1). Items in fractional situations are divisible, and any fractional value can be considered. The mathematical solution to this issue is as follows: Assume there are n articles, Zi to Zn, where Zi has both Vi and Wi values. Xi is the number of copies of the preceding item Zi, which must be either zero or one. W is the maximum bag weight. The assumption is prevalent that all values have to be non-negative.
Maximize the value of objects in the knapsack to a minimum or equivalent weight of the knapsack capacity (W)[164There are several algorithms that might solve the 0–1 crash problem, such as brute strength, dynamic programming, memory functions, gullible algorithm, branch and bound, GA, and so on [165] [166].
Optimization techniques characterized their ease of use, rapid convergence and skills in solving multi-optimization issues which are not linear and non-differential. Many evolutionary strategies fight for the optimum answer to problems of optimization. PSO is one of the most robust and diverse approaches (A. et al., 2016; Tantrapon et al., 2020; Zhang et al., 2014). Kennedy and Eberhart [88–90] devised PSO after being inspired by avian flocculation. A swarm of birds hunting for food in a search area can explain PSO. During the search, each bird has a position and a speed. Every bird’s speed and position are updated based on its position and the position of the bird nearest to food (Karami and Guerrero-Zapata, 2015; Lakshmanaprabu et al., 2019; Boussaad and Boucetta, 2020).
PSO is a computer technique in which each particle is a solution of a swarm population [25], [94–102]. Every particle in the swarm travels in the space of search, and each particle discovers its own experience as well as the particles around it. The PSO algorithm’s stages are as shown in Figure 1.
James Kennedy and Eberhart hosted a classical optimization of BPSO during the year (1995). The fitness parameters for this Particle Swarm Optimization are determined from the (swarm) trajectory movement of persons (particles). An n-length vector is defined that indicates its position and a vector v that indicates its present position (Hosseini and Shahgholian, 2017; Siva Subramanyam Reddy et al., 2017; Ruth et al., 2019). The velocity vector is determined using the equation shown below;
R1 and R2 are the random functions, and C1 and C2 are the training coefficients. This is the inertia weight dimension. The following outcome can be characterized as:
The PSO formula remained unaffected. A logistic conversion S(Vik) is used to achieve this amendment that is written in
The function S (
GA is an optimization strategy that is based on the theoretical idea of natural evolutionary processes such as mutation, inheritance, crossover, and selection. Non-linear issues are easily handled by GA [106]. In GA, a population of chromosomes is created, and each chromosome represents a solution, with the population size determined by the difficulty of the issue. The fitness value of each person in the population is assessed using a fitness function, and comparatively fit chromosomes are chosen to convey information to the next generation, as well as genetic techniques such as mutation, selection, and crossover. Individual fitness improves as the number of generations grows. This technique is repeated until the best group of chromosomes according to a specific criterion is found. (Mahsal Khan et al., 2013; Teek et al., 2013; Papamartzivanos et al., 2018; Kotyan and Vargas, 2020).
A generic algorithm, often known as a global heuristic algorithm, predicts an ideal answer by generating different individuals. Focused fitness function is one of the algorithm techniques. This section discusses the basic pieces of a generic algorithm. Figure 2 shows the general mechanism of the genetic algorithm (Yusoff et al., 2011; Kubat, 2017; Zhang et al., 2019; Mishra et al., 2021).
The genetic algorithm starts with a basic population of random chromosomes containing genes with a 0 s or 1s sequence. The programme then directs people through repetitious processes, including crossover and selection operators, to an optimum answer. A new population is evolving in two ways: stationary GA and generational GA (Hong et al., 2001; Chen et al., 2015; Elngar, 2018; Kulkarni and Thorat, 2019; Strader et al., 2020). In the former case, a generational GA replaces one or two of the population at the same time that it replaces all the generated humans of a generation.
The genetic algorithm defines the fitness function as a system for rating each chromosome based on its qualification. The allocated score is a characteristic of future replication. Because of the problem’s reliance on the fitness function, the problem cannot be described in the event of specific problems. Individuals are naturally permitted to pass on to the next generation based on their fitness. Individuals’ fate is therefore determined by their score [105] [118]–[123].
Every successive generation produces a new generation by adopting individuals of the present generation to cater to the foundations of their fitness. People with better fitness ratings are more likely to be chosen, resulting in preferred adoption of the best answer. Most functions comprise an element that is stochastically designed to accommodate a small number of people who are less suitable for maintaining diversity in the population. Among the many selection methods, Roulette-Wheel is adopted to differentiate proper individuals with the probability of:
Where fitness chromosomes and population size are Fi and “n.” Each person is allocated a value between 0 and one according to the roulette wheel.
The main step of manufacturing is the process of crossing or reproduction. In reality, sexual reproduction mechanisms that carry down genetic characteristics from generation to generation are duplicated. The crossover step in the reproduction process takes a few people as parents through the breeding process. The process in the new population continues to grow until it reaches the goal size. In general, there are several crossover operations, each with its own set of goals. The simplest approach to divide patent roles is by a single point [16] [60], [95] [124]–[129].
We discussed DSM strategies in the smart grid, including load shedding, incentive-based DLC, and dynamic pricing-based ECS. In the area of smart appliance scheduling, optimization techniques such as Knap-sack, PSO, and GA have been presented. A comparison of multiple dynamic pricing-based ECS is offered, taking into account various criteria such as billing mechanism, user fairness, algorithm processing times, and so on. Analyses were performed on ten contemporary and important ECS designs. The maximum level of fairness (73 percent) is achieved in [55], while the biggest PAR decrease (38.1 percent) among the evaluated schemes is offered in [11]. To manage energy usage, many DLC systems have been used These systems are more beneficial for heavier loads with a higher potential for peak load reduction. ECS enables more effective DSM, particularly for residential loads, by utilizing efficient optimization approaches while protecting customers’ privacy and comfort. Efficient DSM methods are critical for reducing energy use. To reap the benefits of DSM in smart grid, a variety of technologies, including ICTs and improved control mechanisms, are necessary. Finding adequate communication and control infrastructure, developing DSM rules, and optimizing energy usage are continuing research issues in smart grid efficiency [99] [101], [106] [130]–[133].
Modelling the load level of the aggregator is studied. Consumer device data is provided to the local aggregator and the aggregator is accountable to gather and reprograming consumer devices according to the service provider’s answer. For this model, there are two types of dwellings (Drotar, 2000; Sahraie et al., 2015; Kalita and Emilia, 2018; Ardabili et al., 2020; Ganesh Kumar et al., 2020; Tigga and Garg, 2020). Houses with no DGs (n) and Houses with no DGs (n) (m). In this proposed approach, 6.000 home customers were divided into consumer appliance load profiles in order to collect data and establish flexible hours when consumers likely to use the following equipment. Figure 3 shows the rescheduling of the consumer appliances.
The above Figure 3 shows the step by step procedure to solve the proposed. It is based on the search method to explore the optimal cost during the execution. The rearrangement model is intended for customers that use schedulable devices. Once the individual device’s location is discovered, the procedure begins for a time during which the utility estimate is lower than the prediction desired by the consumer. (Shahinfar et al., 2014; López Pineda et al., 2015; Mantovani et al., 2015; Özel et al., 2016; Lin et al., 2019; Zardari et al., 2019). The next stage is to discover random devices that require time to work. Then an aggregator identifies a residence that uses the selected device and replaces it at various times (Farran et al., 2019). When the reprogramming of the appliance is completed, the data gets updated in the system. This occurs after rescheduling to ensure that consumer forecast data is current. When this rescheduling is finished, the model checks to see whether the consumer forecast is bigger than predicted and if so, the model will run for a maximum of 20 replacement schedules per instant to reduce the consumer forecast load from time to time. This limit ensures that the rescheduling devices are completed within a time range and respects the satisfaction of the consumer by restricting the number of devices scheduled (Alehegn and Joshi, 2017; Barriga and Yoo, 2017; Ferrão, 2017; Ghani et al., 2019; Islam Ayon and Milon Islam, 2019; Latha and Vetrivelan, 2019; Melotti and Premebida, 2020; Yoon and Park, 2020; Ahmad et al., 2021). The software returns back to the optimization model once the rescheduling is completed and needs to start running again since the appliances are now rescheduled in separate time intervals [152]-[162].
IEEE 4-bus radial distribution feeder with a rated voltage of 4.16 kV line per line is utilized for numerical analysis of the proposed model [13]. Each node is presumed to be controlled by a local aggregator and nodes two to four are modelled with the users following Table 1 line impedances. This radial feeder is modelled on unidirectional power flow and the following information is obtained from the information on the available IEEE four bus feeder[13].
Table 2 below displays optimization limits and their initial states. The voltage limitations should be about one p. u. Since This model is meant to analyze the influence of actual power on the system; each time, real power fluctuation is limited to 0.3%. The reactive power restrictions are relaxed to accommodate tough constraints such as power factor and voltage fluctuation.
The following analyses are simulated and the results illustrate the voltage deviation and distribution supply power factors for 30 consumer projections following rescheduling of consumer loads.
The figure below shows the utility projections. The three-different utility forecast was examined to evaluate the reprogramming of customer loads as described. Figure 4 shows the consumer energy demand forecast, while Figure 5 shows the three-utility forecast.
The simulation’s results were further examined by classifying them into two groups. In the first instance, how does rescheduling assist the customer, and in the second instance, how does rescheduling benefit the utility at the distribution level. The impetus for this work and for two of our aims to discover the benefits for customers and the utility. In terms of consumer benefit, the number of appliances reprogrammed by the DR programme is used to assess customer satisfaction. Table 3 shows the average number of reprogrammed consumer appliances for 30 consumer predictions, with the same number of appliances for each utility estimate. Similarly, Table 4; Table 5 shows the results of utility two and utility three respectively. Electric iron and random equipment have been reprogrammed to all utilities’ projections more than other equipment. The reason for this is that both electrical iron and random device categories are less powerful. It is therefore time for 1700 1800 1900 2000 2100 2200 2300 2400 2500 to reduce considerable power reduction and archive. 6:00 a.m. 9:00 a.m. 12:00 p.m. 15:00 p.m. 12:00 p.m. 6:00 p.m. Forecast for utility load (kW) Forecast one Projection two Projection 3 30 Expected load forecast profile utilities. They are also scheduled to function over a broader duration. The reprogramming process has therefore selected both these devices more than the other.
The distribution level advantage is considered by examining the voltage differential and how the rescheduled customer demand profile reflects the projected utility charging profile. This work intends to retain the power factor within the limitations of each node by further rearranging the load for the consumer appliance. Finally, after rescheduling occasions where customer demand exceeds the scheduled utility load, the energy is evaluated above the predicted energy to be used in the following day generation. For each utility prediction, the average voltage variation before and after rescheduling for each node is provided below. Figure 6 show the node voltages after reprogramming for utility predictions 01 without rescheduling while Figure 7 displays node voltages. The reprogrammed nodal tensions are maintained at near to 1.0 per unit and within 0.9 and 1.1 limits.
Figure 6 depicts the voltages for utility forecast 02 without rescheduling and Figure 7 depicts the voltages with rescheduling, while the voltage without scheduling is given in Figure 8.
Voltages for utility forecast 03 without rescheduling is shown in Figure 9 and rescheduling is shown in Figure 10.
For the utility forecast, the average for simulations was 01, indicating an average of 170 kWh of energy that could not be delivered with up to 240 kWh and 125 kWh of energy that could not be supplied in both of its 30 scenarios. When the 30 simulations for the 02 utility forecast were run, the greatest average power that could not be utilized was roughly 290 kWh on average. It could not serve up to 340 kWh of electricity in accordance with the utility forecast 02. The simulations for the Utility 03 forecast show the average energy of 260 kWh between 01 and 03. These utility forecast profiles imply that a more precise model is needed to anticipate the consumer forecast for the next day. As already indicated, the closer the utility load estimate gets, the minimum number of opportunities are that consumer appliances have to be reprogrammed and that benefit the consumers in general.
As the number of equipment in this model is not modified and power factors are also employed as tough limitations for the objective faction, the Figure 11 show a slight difference while the Figure 12 shows the average number of the times of reschedule. . The 0.86 0.88 0.9 0.92 0.94 0.96 0.98 1 1.02 nevertheless, 6 a.m. 9 a.m. 12 a.m. 3 a.m. 6 a.m. 9 a.m. 12:00 p.m. 6:00 p.m. Power Factor Node two Node three Node 4 0.86 0.88 0.92 0.94 0.96 0.98 1 1.02 Power Factor Time Node 2 6:00 a.m. 9:00 Uhr 12:00 Uhr 15:00 Uhr 18:00 Uhr 9:00 Uhr 12:00 p.m. 6:00 p.m. The power factor time node two Node three Node 4 41 is within the range of 0.8 and 1. The outcome within the expected range or better gives this research an extra value.
This research examines the feasibility of reprogramming consumer products at high loads during system overloads according to the requirements of the distribution system. This analysis considers three different levels of utility load forecasts to evaluate the possibility of changing consumer appliances at various times of the day to aid the local substation in providing an uninterrupted power supply without additional generating devices or acquiring power from nearby energy providers. The analysis shows the number of devices reprogrammed for all utility projections. On average, reprogramming succeeded in reducing peak demand. As projected, additional needs were created during lower demand periods. This implies that decreased demand times can sustain additional demand and fulfil the requirements of the distribution system. The scope of this work can be extended to include the following subjects. 1) The cost model, which could be used to indicate rescheduling, reduced costs generated by assigning different prices for different levels of the projected charge of the utility. 2) Data about consumer equipment usage, including desired time of use if the requisite time is not available. This would allow for a better knowledge of client wants and conduct. In addition, the unexpected time provided would assist this strategy by rescheduling loads to those times. 3) Active consumer participation can be utilised to determine how much electricity the utility can reschedule at different percentages level in each node depending on the number of customers. 4) increase the number of schedulable sorts of devices like thermostats, HVAC etc and prioritise the device to be scheduled randomly. Redesigning large-scale electricity consumables e.g. electric iron from a single house instead of modifying 20 dwellings can be helpful for the consumers. 5) Moreover, the growing number of DGs might be employed in a house while compared to a standard house without DG to analyse the demand profile.
The original contributions presented in the study are included in the article/Supplementary Material, further inquiries can be directed to the corresponding author.
Conceptualization, SB and MA; methodology, HA and MS; software, SB validation, HJ and formal analysis, MH and AM; investigation, HH; writing—“SB and MA; writing—” review and editing, MH and HH, AM; All authors have read and agreed to the published version of the manuscript.
This research was funded by Princess Nourah bint Abdulrahman University Researchers Supporting Project number (PNURSP2022R125), Princess Nourah bint Abdulrahman University, Riyadh, Saudi Arabia.
The authors declare that the research was conducted in the absence of any commercial or financial relationships that could be construed as a potential conflict of interest.
All claims expressed in this article are solely those of the authors and do not necessarily represent those of their affiliated organizations, or those of the publisher, the editors and the reviewers. Any product that may be evaluated in this article, or claim that may be made by its manufacturer, is not guaranteed or endorsed by the publisher.
We are thankful to our University for providing the research environment.
A, . R., ElSeidy, E., and Ibrahim, B. (2016). A Novel Efficient Forecasting of Stock Market Using Particle Swarm Optimization with Center of Mass Based Technique. Int. J. Adv. Comput. Sci. Appl. 7 (4), 342–347. doi:10.14569/ijacsa.2016.070445
Adhikari, U., Morris, T., and Pan, S. (2017). WAMS Cyber-Physical Test Bed for Power System, Cybersecurity Study, and Data Mining. IEEE Trans. Smart Grid 8 (6), 2744–2753. doi:10.1109/TSG.2016.2537210
Ahmad, H. F., Mukhtar, H., Alaqail, H., Seliaman, M., and Alhumam, A. (2021). Investigating Health-Related Features and Their Impact on the Prediction of Diabetes Using Machine Learning. Appl. Sci. 11 (3), 1–18. doi:10.3390/app11031173
Ahshan, R. (2013). Modeling and Analysis of a Micro-grid System Powered by Renewable Energy Sources. Torej 6 (1), 7–22. doi:10.2174/1876387101306010007
Alehegn, M., and Joshi, R. (2017). Analysis and Prediction of Diabetes Diseases Using Machine Learning Algorithm: Ensemble Approach. Int. Res. J. Eng. Techn. 4 (10), 426–436.
Ali Zardari, Z., He, J., Zhu, N., Mohammadani, K., Pathan, M., Hussain, M., et al. (2019). A Dual Attack Detection Technique to Identify Black and gray Hole Attacks Using an Intrusion Detection System and a Connected Dominating Set in MANETs. Future Internet 11 (3), 61. doi:10.3390/fi11030061
Andreasson, P., Johansson, J., Liljestrand, S., and Granath, M. (2019). Quantum Error Correction for the Toric Code Using Deep Reinforcement Learning. Quantum 3, 183. doi:10.22331/q-2019-09-02-183
Anitha Ruth, J., Sirmathi, H., and Meenakshi, A. (2019). Secure Data Storage and Intrusion Detection in the Cloud Using MANN and Dual Encryption through Various Attacks. IET Inf. Security 13 (4), 321–329. doi:10.1049/iet-ifs.2018.5295
Ardabili, S. F., Mosavi, A., Ghamisi, P., Ferdinand, F., Varkonyi-Koczy, A. R., Reuter, U., et al. (2020). COVID-19 Outbreak Prediction with Machine Learning. Algorithms 13 (10), 249. doi:10.3390/a13100249
Babar, M., Tariq, M. U., and Jan, M. A. (2020). Secure and Resilient Demand Side Management Engine Using Machine Learning for IoT-Enabled Smart Grid. Sust. Cities Soc. 62. 102370. doi:10.1016/j.scs.2020.102370
Barriga, J. J. A., and Yoo, S. G. (2017). Malware Detection and Evasion with Machine Learning Techniques: A Survey. Int. J. Appl. Eng. Res. 12 (18), 7207–7214.
Boussaad, L., and Boucetta, A. (2020). An Effective Component-Based Age-Invariant Face Recognition Using Discriminant Correlation Analysis. J. King Saud Univ. - Comput. Inf. Sci. doi:10.1016/j.jksuci.2020.08.009
Brandstetter, M., Schirrer, A., and Mileti, M., (2015). Hierarchical Predictive Load Control in Smart Grids, 1–10.
Chen, Z.-Y., Lin, W.-C., Ke, S.-W., and Tsai, C.-F. (2015). Evolutionary Feature and Instance Selection for Traffic Sign Recognition. Comput. Industry 74, 201–211. doi:10.1016/j.compind.2015.08.007
Cui, L., Qu, Y., Gao, L., Xie, G., and Yu, S. (2020). Detecting False Data Attacks Using Machine Learning Techniques in Smart Grid: A Survey. J. Netw. Comput. Appl. 170, 102808. doi:10.1016/j.jnca.2020.102808
Cui, N., Zou, X., and Xu, L. (2020). Preliminary CT Findings of Coronavirus Disease 2019 (COVID-19). Clin. Imaging 65 (32), 124–132. doi:10.1016/j.clinimag.2020.04.042
Dari, E. Y., and Essaaidi, M. (2016). “An Overview of Smart Grid Cyber-Security State of the Art Study,” in Proceedings of 2015 IEEE International Renewable and Sustainable Energy Conference (Marrakech, Morocco: IRSEC). doi:10.1109/IRSEC.2015.7455097
Dias, A. (2018). ETArch-SG : Uma Proposta de Arquitetura Baseada em SDN para Suporte de Comunicação em Smart Grids.
Drotar, D. (2000). Writing Research Articles for Publication, 347–374. doi:10.1007/978-1-4615-4165-3_16
Eba, M. D., Biogas-e, S. T., and Fernández, D., (2020). D6.1 - Mapping the State of Play of Renewable Gases in Europe, 1–60.857796
Elngar, A. A. (2018). IoT-based Efficient Tamper Detection Mechanism for Healthcare Application. Int. J. Netw. Security 20 (March3), 11. doi:10.6633/IJNS.201805.20
Ennaji, R., and Boulmalf, M. (2009). Routing in Wireless Sensor Networks. Int. Conf. Multimedia Comput. Syst. -Proceedings (September), 495–500. doi:10.1109/MMCS.2009.5256646
Farran, B., AlWotayan, R., Alkandari, H., Al-Abdulrazzaq, D., Channanath, A., and Thanaraj, T. A. (2019). Use of Non-invasive Parameters and Machine-Learning Algorithms for Predicting Future Risk of Type 2 Diabetes: A Retrospective Cohort Study of Health Data from Kuwait. Front. Endocrinol. 10 (September), 1–11. doi:10.3389/fendo.2019.00624
Firouzi, S. R., Vanfretti, L., Ruiz-Alvarez, A., Hooshyar, H., and Mahmood, F. (2017). Interpreting and Implementing IEC 61850-90-5 Routed-Sampled Value and Routed-GOOSE Protocols for IEEE C37.118.2 Compliant Wide-Area Synchrophasor Data Transfer. Electric Power Syst. Res. 144, 255–267. doi:10.1016/j.epsr.2016.12.006
Frequency, M., Attenuation, F., and Dynamics, C., (2020). Considering Dynamics and Allowable Deviation, 1–23.
Ganesh Kumar, S., Jain, R., and Chauhan, A. S. (2020). A Novel Job portal with Resume Evaluation System Based on Text Mining and Nlp Techniques. J. Crit. Rev. 7 (8), 1234–1236. doi:10.31838/jcr.07.08.255
Ghani, M. U., Alam, T. M., and Jaskani, F. H. (2019). “Comparison of Classification Models for Early Prediction of Breast Cancer,” in 3rd International Conference on Innovative Computing (Lahore: ICIC). doi:10.1109/ICIC48496.2019.8966691
Goldsmith, A., Jafar, S. A., Maric, I., and Srinivasa, S. (2009). Breaking Spectrum Gridlock with Cognitive Radios: An Information Theoretic Perspective. Proc. IEEE 97, 894–914. doi:10.1109/JPROC.2009.2015717
Guerrero, J. M., Loh, P. C., Lee, T.-L., and Chandorkar, M. (2013). Advanced Control Architectures for Intelligent Microgrids-Part II: Power Quality, Energy Storage, and AC/DC Microgrids. IEEE Trans. Ind. Electron. 60 (4), 1263–1270. doi:10.1109/TIE.2012.2196889
Gunduz, M. Z., and Das, R. (2018). Analysis of Cyber-Attacks on Smart Grid Applications. 2018 Int. Conf. Artif. Intelligence Data Process. IDAP 2018 (1), 1. doi:10.1109/IDAP.2018.8620728
Gunduz, M. Z., and Das, R. (2020). Cyber-security on Smart Grid: Threats and Potential Solutions. Computer Networks 169, 107094. doi:10.1016/j.comnet.2019.107094
Hasan, M. K., Alam, M. A., Das, D., Hossain, E., and Hasan, M. (2020). Diabetes Prediction Using Ensembling of Different Machine Learning Classifiers. IEEE Access 8, 76516–76531. doi:10.1109/ACCESS.2020.2989857
Hong, T.-P., Huang, K.-Y., and Lin, W.-Y. (2001). Adversarial Search by Evolutionary Computation. Evol. Comput. 9 (3), 371–385. doi:10.1162/106365601750406046
Hong, T., and de Leon, F. (2017). Controlling Non-synchronous Microgrids for Load Balancing of Radial Distribution Systems. IEEE Trans. Smart Grid 8 (6), 2608–2616. doi:10.1109/TSG.2016.2531983
Hossain, E., Khan, I., Un-Noor, F., Sikander, S. S., and Sunny, M. S. H. (2019). Application of Big Data and Machine Learning in Smart Grid, and Associated Security Concerns: A Review. IEEE Access 7, 13960–13988. doi:10.1109/ACCESS.2019.2894819
Hosseini, E., and Shahgholian, G. (2017). Output Power Levelling for Dfig Wind Turbine System Using Intelligent Pitch Angle Control. Automatika 58, 363–374. doi:10.1080/00051144.2018.1455017
Hu, F., Chen, H., and Wang, X. (2020). An Intuitionistic Kernel-Based Fuzzy C-Means Clustering Algorithm with Local Information for Power Equipment Image Segmentation. IEEE Access 8, 4500–4514. doi:10.1109/ACCESS.2019.2963444
Hu, J., Zhang, T., Du, S., and Zhao, Y. (2015). An Overview on Analysis and Control of Micro-grid System. Ijca 8 (6), 65–76. doi:10.14257/ijca.2015.8.6.08
Islam Ayon, S., Milon Islam, M., and Milon Islam, M. (2019). Diabetes Prediction: A Deep Learning Approach. Ijieeb 11 (2), 21–27. doi:10.5815/ijieeb.2019.02.03
Javed, H., and Muqeet, H. A. (2021). Design , Model & Planning of Prosumer Microgrid for MNSUET Multan Campus. Sir Syed Univ. Res. J. Eng. Techn. 11 (2), 1–7. doi:10.33317/ssurj.381
Javed, H., Muqeet, H. A., and Shehzad, M. (2021). Optimal Energy Management of a Campus Microgrid Considering Financial and Economic Analysis with Demand Response Strategies. Energies, 1–24. doi:10.3390/en14248501
Ji, T., Senior, M., and Yang, Y., (2020). Impacts of Long-Term Transactions on Strategic Bidding in Electricity Market. Csee Jpes. doi:10.17775/cseejpes.2020.02740
Jiang, W., Zhao, J., Qu, K., Mao, L., Zhu, Y., and Liu, H. (2019). “An Enhanced Drop Control Method for DC Microgrids with Accurate Current Sharing and DC Bus Voltage Restoration,” in 2019 4th International Conference On Intelligent Green Building And Smart Grid (IGBSG) (IEEE), 727–731. doi:10.1109/IGBSG.2019.8886270
J.O. Petinrin, J. O. P., and M. Shaaban, M. S. (2015). Overcoming Challenges of Renewable Energy on Future Smart Grid. Telkomnika 10 (2), 229. doi:10.12928/telkomnika.v10i2.781
Kalita, J., and Emilia, V. (2018). Advances in Intelligent Systems and Computing 740 Recent Developments in Machine Learning and Data Analytics.
Karami, A., and Guerrero-Zapata, M. (2015). A Fuzzy Anomaly Detection System Based on Hybrid PSO-Kmeans Algorithm in Content-Centric Networks. Neurocomputing 149, 1253–1269. doi:10.1016/j.neucom.2014.08.070
Khan, R., McLaughlin, K., Laverty, D., and Sezer, S. (2017). Design and Implementation of Security Gateway for Synchrophasor Based Real-Time Control and Monitoring in Smart Grid. IEEE Access 5, 11626–11644. doi:10.1109/ACCESS.2017.2716440
Kirakosyan, A., El-Saadany, E. F., Moursi, M. S. E., Yazdavar, A. H., and Al-Durra, A. (2020). Communication-Free Current Sharing Control Strategy for DC Microgrids and its Application for AC/DC Hybrid Microgrids. IEEE Trans. Power Syst. 35 (1), 140–151. doi:10.1109/TPWRS.2019.2925779
Kotsampopoulos, P., Georgilakis, P., Lagos, D. T., Kleftakis, V., and Hatziargyriou, N. (2019). Facts Providing Grid Services: Applications and Testing. Energies 12 (13), 2554. doi:10.3390/en12132554
Kotyan, S., and Vargas, D. V. (2020). Towards Evolving Robust Neural Architectures to Defend from Adversarial Attacks. GECCO 2020 Companion - Proc. 2020 Genet. Evol. Comput. Conf. Companion (1), 135–136. doi:10.1145/3377929.3389962
Kreikebaum, F., Das, D., Yang, Y., Lambert, F., and Divan, D. (2010). Smart Wires A Distributed, Low-Cost Solution for Controlling Power Flows and Monitoring Transmission Lines.in IEEE PES Innovative Smart Grid Technologies Conference Europe. Berlin: ISGT. 1–8. doi:10.1109/ISGTEUROPE.2010.5638853
Kulkarni, Y. R., and Thorat, S. A. (2019). Network Malware Detection Using Soft Computing and Machine Learning Techniques. Ijeat 9 (2), 879–885. doi:10.35940/ijeat.a1654.129219
Kumar, Y. V. P., and Bhimasingu, R. (2015). “Performance Analysis of green Microgrid Architectures by Comparing Power Quality Indices,” in 2014 18th National Power Systems Conference (NPSC). doi:10.1109/NPSC.2014.7103873
Latha, R., and Vetrivelan, P. (2019). Decision Making Patient Assistive Strategies in Wireless Body Area Networks for Remote Healthcare System. Int. J. Recent Techn. Eng. 8 (1), 2199–2203.
Lin, C.-C., Chang, C.-C., and Wang, Z.-M. (2019). Reversible Data Hiding Scheme Using Adaptive Block Truncation Coding Based on an Edge-Based Quantization Approach. Symmetry 11 (6), 765. doi:10.3390/sym11060765
Lin, J., Dong, P., Liu, M., Huang, X., and Deng, W. (2020). Research on Demand Response of Electric Vehicle Agents Based on Multi-Layer Machine Learning Algorithm. IEEE Access 8, 224224–224234. doi:10.1109/ACCESS.2020.3042235
López Pineda, A., Ye, Y., Visweswaran, S., Cooper, G. F., Wagner, M. M., and Tsui, F. (2015). Comparison of Machine Learning Classifiers for Influenza Detection from Emergency Department Free-Text Reports. J. Biomed. Inform. 58 (September), 60–69. doi:10.1016/j.jbi.2015.08.019
Lotfi, H., Khodaei, A., and Bahramirad, S., (2016). Optimal Design of Hybrid AC/DC MicrogridsCIGRE Grid of the Future Symposium.
Mahsal Khan, M., Masood Ahmad, A., Muhammad Khan, G., and Miller, J. F. (2013). Fast Learning Neural Networks Using Cartesian Genetic Programming. Neurocomputing 121, 274–289. doi:10.1016/j.neucom.2013.04.005
Majzoobi, A., and Khodaei, A. (2016). Set of Energy Storage Systems. Application of Microgrids in Supporting Distribution Grid Flexibility, 1–10.
Mantovani, R. G., Rossi, A. L. D., Vanschoren, J., Bischl, B., and de Carvalho, A. C. P. L. F. (2015). Effectiveness of Random Search in SVM Hyper-Parameter Tuning. Proc. Int. Jt. Conf. Neural Networks 2015-Septe. doi:10.1109/IJCNN.2015.7280664
Meier, R., Knecht, U., and Wiest, R. (2016). Brainlesion: Glioma, Multiple Sclerosis. Stroke and Traumatic Brain Injuries 10154, 184. doi:10.1007/978-3-319-55524-9
Melotti, G., and Premebida, C. (2020). Multimodal Deep-Learning for Object Recognition Combining Camera and LIDAR Data.
Meraj, T., Sharmin, S., and Mahmud, A. (2015). Studying the Impacts of Cyber-Attack on Smart Grid. IEEE, 461–466. doi:10.1109/EICT.2015.7391997
Minhas, D. M., and Member, G. S. (2016). Load Balancing in Smart DC Micro-grid Using Delay Tolerant User Demands. doi:10.1109/SmartGridComm.2015.7436293
Mishra, E., and Tiwari, S. (2017). Comparative Analysis of Fuzzy Logic and PI Controller Based Electronic Load Controller for Self-Excited Induction Generator. Adv. Electr. Eng. 2017, 1–9. doi:10.1155/2017/5620830
Mishra, P. K., Satapathy, S. C., and Rout, M. (2021). Segmentation of MRI Brain Tumor Image Using Optimization Based Deep Convolutional Neural Networks (DCNN). Open Comput. Sci. 11 (1), 380–390. doi:10.1515/comp-2020-0166
Miura, H., and Wu, G. (2014). Voltage Stabilization of Distribution System Integrated by Renewable Power Generations by Cooperated Control of STATCOM and Interconnecting Microgrids. Sgce 3 (1), 96–103. doi:10.12720/sgce.3.1.96-103
Monteiro, J., Fernando, J., Pinto, S., and Palm, J. (2011). Unified Power Flow Controllers without Energy Storage: Designing Power Controllers for the Matrix Converter Solution. Energy Storage in the Emerging Era of Smart Grids. doi:10.5772/21421
Motoyama, K., Hanamoto, T., and Yamada, H., (2014). Study of Matrix Converter Based Unified Power Flow Controller Applied PI-D Controller. J. Eng. Sci. Techn. 9, 30–38.
Muqeet, H. A., Munir, H. M., and Javed, H., (2021). An Energy Management System of Campus Microgrids : State-Of-The-Art and Future Challenges. Energies 14 (Issue 20), 1–34. doi:10.3390/en14206525
Muqeet, H. A., Sajjad, I. A., Ahmad, A., Iqbal, M. M., Ali, S., and Guerrero, J. M. (2019). Optimal Operation of Energy Storage System for a Prosumer Microgrid Considering Economical and Environmental Effects. IEEE, 1–6. doi:10.1109/RAEE.2019.8887002
Muqeet, H. A. U., Ahmad, A., and Member, G. S. (2020). Optimal Scheduling for Campus Prosumer Microgrid Considering Price Based Demand Response. IEEE Access 8. 71378–71394. doi:10.1109/ACCESS.2020.2987915
Navilgone, J., and Thesis, G. (2008) Unified Power Quality Conditioner for Grid Integration of Wind Generators. doi:10.21427/D7260W
Özel, G., Açıkkalp, E., Fevzi Savaş, A., and Yamık, H.(2016). Comparative Analysis of Thermoeconomic Evaluation Criteria for an Actual Heat Engine. J. Non-Equilib. Thermodyn. 41 (3), 225–235. doi:10.1515/jnet-2015-0053
Pan, J., and Yang, Z. (2018). Cybersecurity Challenges and Opportunities in the New "Edge Computing + IoT" World.in. Proceedings of the 2018 ACM International Workshop on Security in Software Defined Networks and Network Function Virtualization. 29–32. doi:10.1145/3180465.3180470
Papamartzivanos, D., Gómez Mármol, F., and Kambourakis, G. (2018). Dendron : Genetic Trees Driven Rule Induction for Network Intrusion Detection Systems. Future Generation Comput. Syst. 79, 558–574. doi:10.1016/j.future.2017.09.056
Peng, C., Sun, H., Yang, M., and Wang, Y.-L. (2019). A Survey on Security Communication and Control for Smart Grids under Malicious Cyber Attacks. IEEE Trans. Syst. Man. Cybern, Syst.IEEE 49 (8), 1554–1569. doi:10.1109/TSMC.2018.2884952
Perez-Guzman, R. E., Salgueiro-Sicilia, Y., and Rivera, M. (2018). Communication Systems and Security Issues in Smart Microgrids.in Proceedings - 2017 IEEE Southern Power Electronics Conference. 1–6. doi:10.1109/SPEC.2017.8333659
Petrenko, S. A., and Makoveichuk, K. A. (2017). Ontology of Cyber Security of Self-Recovering Smart Grid. CEUR Workshop Proc. 2081, 98–106.
Ramadhan, M. M., Sitanggang, I. S., Nasution, F. R., and Ghifari, A. (2017). Parameter Tuning in Random Forest Based on Grid Search Method for Gender Classification Based on Voice Frequency. dtcse. doi:10.12783/dtcse/cece2017/14611
Rawat, D. B., and Bajracharya, C. (2015). “Cyber Security for Smart Grid Systems: Status, Challenges and Perspectives,” in Conference Proceedings - IEEE SOUTHEASTCON 2015-June(June). doi:10.1109/SECON.2015.7132891
Reddy, G. M., and Manohar, T. G. (2018). Fuzzy Logic Controller Based STATCOM for Grid Connected Wind Turbine System, 8, 2.
Riaz, M., Jaskani, F., and Awan, T. (2019). An Artificial Neural Network Based Digital Differential Protection Scheme for Synchronous Generator Stator Winding Protection. EAI Endorsed Trans. Creat. Tech. 6 (18), 160837. doi:10.4108/eai.30-1-2019.160837
Riaz, W., Ahmad, Z., and Muhammad, A. (2016). A Smart Metering Approach towards Measuring Flows in Small Irrigation Outlets. Proced. Eng. 154, 236–242. doi:10.1016/j.proeng.2016.07.459
Rose, T., Kifayat, K., Abbas, S., and Asim, M. (2020). A Hybrid Anomaly-Based Intrusion Detection System to Improve Time Complexity in the Internet of Energy Environment, J. Parallel Distributed Comput. 145, 124–139. doi:10.1016/j.jpdc.2020.06.012
Saharia, B. J., Manas, M., and Sen, S. (2016). “Comparative Study on Buck and Buck-Boost DC-DC Converters for MPP Tracking for Photovoltaic Power Systems,” in Proceedings - 2016 2nd International Conference on Computational Intelligence and Communication Technology, Ghaziabad, India (CICT), 382–387. doi:10.1109/CICT.2016.81
Sahraie, H., Mirani, M. R., Ahmadi, M. H., and Ashouri, M. (2015). Thermo-economic and Thermodynamic Analysis and Optimization of a Two-Stage Irreversible Heat Pump. Energ. Convers. Manag. 99. 81–91. doi:10.1016/j.enconman.2015.03.081
Sanjab, A., Saad, W., and Guvenc, I., (2016). Smart Grid Security: Threats, Challenges, and Solutions.
Schaer, R., Müller, H., and Depeursinge, A. (2016). Optimized Distributed Hyperparameter Search and Simulation for Lung Texture Classification in CT Using Hadoop. J. Imaging 2 (2), 19. doi:10.3390/jimaging2020019
Scholar, M. T., Lamba, A., and Goyal, S. (2016) Improvement of Power Quality Using UPFC. Grid. 5(1): 186–190.
Sgouras, K. I., Birda, A. D., and Labridis, D. P. (2014). “Cyber Attack Impact on Critical Smart Grid Infrastructures,” in 2014 IEEE PES Innovative Smart Grid Technologies Conference (Istanbul Turkey: ISGT). doi:10.1109/ISGT.2014.6816504
Shahab, M., Wang, S., and Junejo, A. K. (2021). Improved Control Strategy for Three-phase Microgrid Management with Electric Vehicles Using Multi Objective Optimization Algorithm. Energies 14 (4), 1146. doi:10.3390/en14041146
Shahinfar, S., Page, D., Guenther, J., Cabrera, V., Fricke, P., and Weigel, K. (2014). Prediction of Insemination Outcomes in Holstein Dairy Cattle Using Alternative Machine Learning Algorithms. J. Dairy Sci. 97 (2), 731–742. doi:10.3168/jds.2013-6693
Shapsough, S., Qatan, F., Aburukba, R., Aloul, F., and Al Ali, A. R. (2016). “Smart Grid Cyber Security: Challenges and Solutions,” in Proceedings - 2015 International Conference on Smart Grid and Clean Energy Technologies (ICSGCE), 170–175. doi:10.1109/ICSGCE.2015.7454291
Shinkhede, S. (2014). Implementation of the Micro- Grid Concept and Balancing Massive Energy Production from. Renew. Sourc. 3 (1), 76–84.
Sindhuja, P., Lalitha, B., and Lalitha, B. (2016). Reactive Power Compensation for Grid Connected Distribution System Using Dstatcom for Different Loads. Ijett 40 (5), 257–264. doi:10.14445/22315381/ijett-v40p242
Siva Subramanyam Reddy, R., Gowri Manohar, T., and Kumar Reddy, M. S. (2017). Optimal Placement and Sizing of Unified Power Flow Controller Using Heuristic Techniques for Electrical Transmission System. ARPN J. Eng. Appl. Sci. 12 (22), 6357–6363.
S.K., L., Mohanty, S. N., S., S. R., Krishnamoorthy, S., J., U., and Shankar, K. (2019). Online Clinical Decision Support System Using Optimal Deep Neural Networks. Appl. Soft Comput. 81. 105487. doi:10.1016/j.asoc.2019.105487
Stellios, I., Kotzanikolaou, P., Psarakis, M., Alcaraz, C., and Lopez, J. (2018). A Survey of Iot-Enabled Cyberattacks: Assessing Attack Paths to Critical Infrastructures and Services. IEEE Commun. Surv. Tutorials, 20, 3453–3495. doi:10.1109/COMST.2018.2855563
Strader, T. J., Rozycki, J. J., and Root, T. H., (2020). Machine Learning Stock Market Prediction Studies : Review and Research Directions. J. Int. Techn. Inf. Manag. 28 (4), 63–83.
Syarif, I., Prugel-Bennett, A., and Wills, G. (2016). SVM Parameter Optimization Using Grid Search and Genetic Algorithm to Improve Classification Performance. Telkomnika 14 (4), 1502–1509. doi:10.12928/TELKOMNIKA.v14i4.3956
Tan, S., De, D., Song, W.-Z., Yang, J., and Das, S. K. (2017). Survey of Security Advances in Smart Grid: A Data Driven Approach. IEEE Commun. Surv. Tutorials 19 (1), 397–422. doi:10.1109/COMST.2016.2616442
Tantrapon, K., Jirapong, P., and Thararak, P. (2020). Mitigating Microgrid Voltage Fluctuation Using Battery Energy Storage System with Improved Particle Swarm Optimization. Energ. Rep. 6. 724–730. doi:10.1016/j.egyr.2019.11.145
Teek, R., Kruustük, K., Žordania, R., Joost, K., Kahre, T., Tõnisson, N., et al. (2013). Hearing Impairment in Estonia: An Algorithm to Investigate Genetic Causes in Pediatric Patients. Adv. Med. Sci. 58 (2), 419–428. doi:10.2478/ams-2013-0001
Tigga, N. P., and Garg, S. (2020). Prediction of Type 2 Diabetes Using Machine Learning Classification Methods. Proced. Comput. Sci. 167. 706–716. doi:10.1016/j.procs.2020.03.336
Trinklein, E. H., Cook, M. D., Parker, G. G., and Weaver, W. W. (2020). Exergy Optimal Multi-Physics Aircraft Microgrid Control Architecture. Int. J. Electr. Power Energ. Syst. 114, 105403. doi:10.1016/j.ijepes.2019.105403
Ullah, Z., Al-Turjman, F., Mostarda, L., and Gagliardi, R. (2020). Applications of Artificial Intelligence and Machine Learning in Smart Cities. Comput. Commun. 154. 313–323. doi:10.1016/j.comcom.2020.02.069
Vigneswari, D., Kumar, N. K., Ganesh Raj, V., Gugan, A., and Vikash, S. R. (2019). Machine Learning Tree Classifiers in Predicting Diabetes Mellitus. IEEE, 84. 388. doi:10.1109/ICACCS.2019.8728388
Wang, D., Wang, X., Zhang, Y., and Jin, L. (2019). Detection of Power Grid Disturbances and Cyber-Attacks Based on Machine Learning. J. Inf. Security Appl. 46, 42–52. doi:10.1016/j.jisa.2019.02.008
Wang, W., and Lu, Z. (2013). Cyber Security in the Smart Grid: Survey and Challenges. Comput. Networks 57 (5), 1344–1371. doi:10.1016/j.comnet.2012.12.017
Wang, Y., Gamage, T. T., and Hauser, C. H. (2015). Security Implications of Transport Layer Protocols in Power Grid Synchrophasor Data Communication. IEEE Trans. Smart Grid 7 (2), 1–10. doi:10.1109/TSG.2015.2499766
Wei, Z.-H., and Hu, B.-J. (2018). A Fair Multi-Channel Assignment Algorithm with Practical Implementation in Distributed Cognitive Radio Networks. IEEE Access 6, 14255–14267. doi:10.1109/ACCESS.2018.2808479
Xiao Liang, X., Kunlun Gao, K., Xiaokun Zheng, X., and Ting Zhao, T. (2013). A Study on Cyber Security of Smart Grid on Public Networks. IEEE Green. Tech. Conf., 301–308. doi:10.1109/GreenTech.2013.53
Xie, S., Hong, Y., and Wan, P. (2019). A Privacy Preserving Multiagent System for Load Balancing in the Smart Grid Extended Abstract, 2273–2275.
Yan, Y., Qian, Y., Sharif, H., and Tipper, D. (2012). A Survey on Cyber Security for Smart Grid Communications. IEEE Commun. Surv. Tutorials 14, 998–1010. doi:10.1109/SURV.2012.010912.00035
Yang, Y., Kim, D., and Oh, B. T. (2020). Deep Convolutional Grid Warping Network for Joint Depth Map Upsampling. IEEE Access 8, 147580–147590. doi:10.1109/ACCESS.2020.3015209
Yang, Y., Littler, T., Sezer, S., McLaughlin, K., and Wang, H. F. (2011). Impact of Cyber-Security Issues on Smart Grid. IEEE PES Innovative Smart Grid Tech. Conf. Europe, 1–7. doi:10.1109/ISGTEurope.2011.6162722
Ye, Z., Walling, R., and Miller, N., (2005). Facility Microgrids Facility Microgrids. Contract 38019 (May), 36.
Yoon, H. S., and Park, K. R. (2020). CycleGAN-Based Deblurring for Gaze Tracking in Vehicle Environments. IEEE Access 8, 137418–137437. doi:10.1109/access.2020.3012191
Yu, F. W., Ho, W. T., Chan, K. T., and Sit, R. K. Y. (2017). Probabilistic and Electricity Saving Analyses of Mist Coolers for Chiller System in a Hotel. Energ. Proced. 143, 154–160. doi:10.1016/j.egypro.2017.12.664
Yusoff, Y., Ngadiman, M. S., and Zain, A. M. (2011). Overview of NSGA-II for Optimizing Machining Process Parameters. Proced. Eng. 15, 3978–3983. doi:10.1016/j.proeng.2011.08.745
Zhang, H., Sun, F., and Qian, J. (2019). An Optimization Based on General Airport of Complex Condition. IEEE, 1–5. doi:10.1109/CITS.2019.8862029
Zhang, H., Wang, L., Sun, J., and Hu, X. (2017). “Research on the Voltage Stability of Grid Connected Wind Farm Based on STATCOM Technology,” in Proceedings of the 2017 2nd International Conference on Electrical, Automation and Mechanical Engineering, 86, 17–20. doi:10.2991/eame-17.2017.5
Zhang, X., Jiao, L., Paul, A., Yuan, Y., Wei, Z., and Song, Q. (20142014). Semisupervised Particle Swarm Optimization for Classification. Math. Probl. Eng. 2014, 1–11. doi:10.1155/2014/832135
Zhang, Y., Wang, L., Sun, W., Green II, R. C., and Alam, M. (2011). Distributed Intrusion Detection System in a Multi-Layer Network Architecture of Smart Grids. IEEE Trans. Smart Grid 2 (4), 796–808. doi:10.1109/TSG.2011.2159818
Keywords: energy management, smart grid, scheduling, load management, optimization, demand side managemant, energy efficiency
Citation: Balouch S, Abrar M, Abdul Muqeet H, Shahzad M, Jamil H, Hamdi M, Malik AS and Hamam H (2022) Optimal Scheduling of Demand Side Load Management of Smart Grid Considering Energy Efficiency. Front. Energy Res. 10:861571. doi: 10.3389/fenrg.2022.861571
Received: 24 January 2022; Accepted: 07 March 2022;
Published: 18 May 2022.
Edited by:
Chao Duan, Northwestern University, United StatesReviewed by:
Celestine Iwendi, School of Creative Technologies University of Bolton, United KingdomCopyright © 2022 Balouch, Abrar, Abdul Muqeet, Shahzad, Jamil, Hamdi, Malik and Hamam. This is an open-access article distributed under the terms of the Creative Commons Attribution License (CC BY). The use, distribution or reproduction in other forums is permitted, provided the original author(s) and the copyright owner(s) are credited and that the original publication in this journal is cited, in accordance with accepted academic practice. No use, distribution or reproduction is permitted which does not comply with these terms.
*Correspondence: Monia Hamdi, TXNoYW1kaUBwbnUuZWR1LnNh
Disclaimer: All claims expressed in this article are solely those of the authors and do not necessarily represent those of their affiliated organizations, or those of the publisher, the editors and the reviewers. Any product that may be evaluated in this article or claim that may be made by its manufacturer is not guaranteed or endorsed by the publisher.
Research integrity at Frontiers
Learn more about the work of our research integrity team to safeguard the quality of each article we publish.