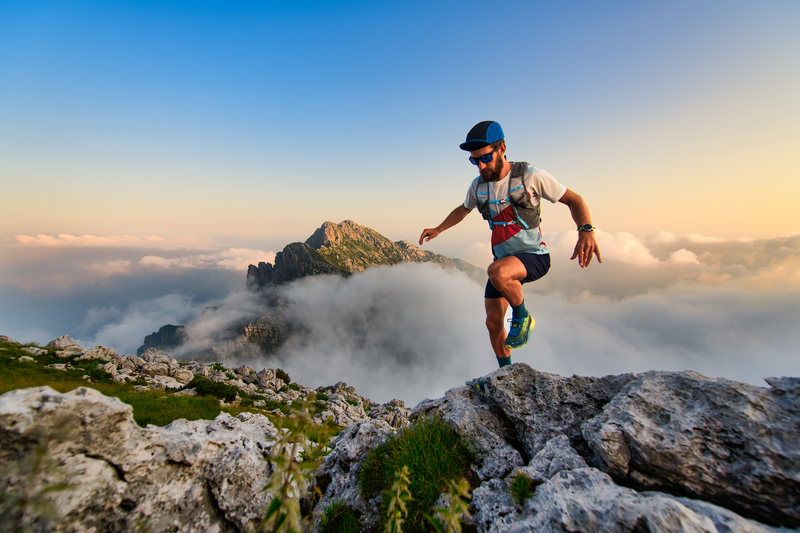
95% of researchers rate our articles as excellent or good
Learn more about the work of our research integrity team to safeguard the quality of each article we publish.
Find out more
OPINION article
Front. Energy Res. , 09 March 2022
Sec. Advanced Clean Fuel Technologies
Volume 10 - 2022 | https://doi.org/10.3389/fenrg.2022.861333
This article is part of the Research Topic Recent Advances in High-efficiency Development of Conventional/Unconventional Gas Reservoirs and CCUS Technologies View all 51 articles
At present, the petroleum industry is in the stage of rapid development from conventional oil and gas reservoirs to unconventional oil and gas reservoirs. As an important part of unconventional oil and gas resources, tight reservoirs have great potential development value (Zho et al., 2020; Xu et al., 2020; Yang et al., 2019). With the continuous improvement of industrial technology, tight reservoirs are becoming the highlight of unconventional oil and gas in the world (Wang et al., 2020; Yan et al., 2020; Jia et al., 2012). The development of low-permeability reservoirs includes reservoir description, hydraulic fracturing, reservoir production, and other aspects. They are a typical complex non-linear dynamic system (Yang et al., 2016). By analyzing the relationship and variation of various parameters, they can provide scientific basis for development of low-permeability reservoirs (Li et al., 2021). An important research content of reservoir development is the analysis and prediction of production data. The accuracy of their prediction results will directly affect the formulation of development plans, so it is very necessary to select appropriate methods.
The fracture distribution of tight reservoirs after hydraulic fracturing is complex (Su et al., 2016) (Figure 1), and it is difficult to describe the production by conventional methods. How to effectively develop and predict production has become a new problem (Guo et al., 2017; Su et al., 2018; Sheng et al., 2019). The current production prediction methods are mainly divided into the decline analysis method, semi-analytic method, numerical simulation method, quantitative characterization method, and artificial neural network. This paper summarizes the existing production capacity prediction methods and discusses the advantages and disadvantages of each method, so as to summarize the latest progress and challenges of production capacity prediction.
FIGURE 1. Mass transfer between multi-scaled pore media in shale reservoirs (Su et al., 2016).
The production decline analysis method is mainly based on seepage mechanics. By measuring the dynamic changes of pressure, production, and temperature of oil and gas wells, we can study and determine the production capacity, well-controlled reserves, and physical parameters of oil and gas reservoirs. Then, the reservoir can be evaluated.
Researchers have done a lot of research on the decline curve method. Chen et al. (2008) combined the Arps index decline model with Weng’s model to deduce a compound decline model. It can be used to calculate the recoverable reserves of gas wells and has been well verified in practical application. Through the analysis and comparison of several production decline models, Liu et al. (2010) believe that most of the production data of oil reservoirs show that the decline conforms to the Arps hyperbolic decline model. However, the applicable conditions of the Arps model are relatively harsh, such that the bottom-hole pressure remains unchanged, the constant pressure boundary. Considering that the Arps model cannot be applied to the early production prediction of gas wells, Duan et al. (2013) improved the shortage that the Arps model can only predict the stable flow state by using the power exponential decline model. Based on the power exponential decline model, the production data of a multi-fractured horizontal well of shale gas reservoirs are analyzed. It is found that the power exponential decline model is very consistent with the prediction results from Topaze. Lu (2018) improved the production decline method by normalizing the pseudo-production and material balance time and enhanced its adaptability to shale gas productivity prediction.
The decline analysis method is mainly aimed at the late stage of reservoir development, which is not applicable to the early and middle stages of reservoir development, and cannot predict the increase in production. At the same time, the decline curve method cannot consider the impact of other parameters on production, so it is difficult to design and adjust some fracturing schemes.
The semi-analytical method is a new method for productivity calculation of fractured horizontal wells, which combines analytical and discrete methods. This method can overcome many shortcomings of the analytical method, such as a narrow application range and difficult solution process. At the same time, it is faster and more effective than the numerical simulation method, which has obvious advantages. Therefore, it is favored by more and more scholars.
Zeng et al. (2007) combined the fracture morphology and seepage mechanism of oil and gas in the fractures of fractured horizontal wells. A productivity calculation model considering the interference between fractures is established. The factors affecting the productivity of fractured horizontal wells are analyzed. Su et al. (2014) divided the fracturing reservoir area into three parts, including the main fracture, the stimulated reservoir volume, and the unstimulated reservoir volume, and established the composite flow model of multi-fractured horizontal wells. The semi-analytical solution of pressure and production was obtained by Stehfest numerical inversion. Li et al. (2018) considered the starting pressure gradient and hydraulic fracture conductivity. Based on the dual media seepage differential equation, the point source theory and pressure drop superposition principle are adopted. A semi-analytical productivity calculation model for staged fracturing of horizontal wells in low-permeability reservoirs is established. The calculation and analysis are carried out with an example. Xu et al. (2021) established an improved five-zone composite seepage model considering the adsorption, diffusion, and slippage effects of shale gas. At the same time, the reliability of the model is verified by the actual production data. Moreover, based on the analysis of productivity-influencing factors, the fracturing parameters are optimized.
The semi-analytic method is widely used to calculate a single-phase production of multi-fractured horizontal wells based on many assumptions. There is a certain gap between the semi-analytic model and the actual oil well, and there are some limitations in describing actual reservoir production. It cannot accurately describe complex geological conditions.
For the numerical simulation method, Peng et al. (2014) established the numerical simulation model of multi-fractured horizontal wells according to the geological characteristics of tight reservoirs and studied the sensitivity of influencing factors of production of multi-fractured horizontal wells. Based on the numerical simulation method, Guo et al. (2018) used the multiple regression method to analyze the impact of multiple factors on production capacity. And the primary and secondary relationships of the impact of various factors on production capacity are clarified. Wu et al. (2018) established a dual media model based on Eclipse. Based on the analysis of the influence law of different influencing factors on production capacity, the production capacity change characteristics are divided into three stages. At the same time, the influence of parameters on production capacity in different production stages is quantified. Pu et al. (2021) established a single-phase flow mathematical model based on oilfield production data and considering the complex characteristics of steering fracture morphology. The local grid encryption technology was used to predict the productivity, and the influencing factors of productivity were analyzed.
The numerical simulation method can analyze the influence of various reservoir characteristics such as different reservoir geological shapes, fluid physical properties, reservoir heterogeneity, boundaries, and faults on the production performance and can also predict the distribution of remaining oil. However, the establishment of the numerical simulation model requires a large number of reservoir data, and the process of establishing the model is more complicated and the calculation time is large. Numerical simulation is highly dependent on the accuracy of parameters. At present, the embedded discrete fracture model does not need to consider the fracture morphology in the reservoir when meshing, which greatly reduces the complexity of meshing. It could be a new breakthrough (Zhang et al., 2019).
The quantitative characterization model (QCM) uses some key parameters with clear physical meaning to describe the changes of oil production and water content in combination with actual production dynamics. Compared with the traditional decline model, it has the advantages of predicting the increase process of production. Hou et al. (2008) established a quantitative characterization model of polymer flooding production performance based on four characteristic parameters with clear physical significance and used this model to quantitatively characterize the oil increment and water cut change trend of polymer flooding in different types of reservoirs in the Shengli Oilfield. Based on the traditional quantitative characterization model, Yu et al. (2009) predicted the production capacity and obtained the quantitative change rule of polymer flooding oil increment curve and water content change curve and the quantitative relationship between oil increment and water content change. Chen et al. (2021) introduced four new characteristic parameters to deduce and establish the quantitative characterization model of vertical shale oil horizontal wells. Combined with the SPSA algorithm, the fitting, prediction, and parameter interpretation inversion of the whole life cycle production dynamic curve are realized.
The quantitative representation model is simple and intuitive. Based on this model, four key parameters can be fitted according to the production performance of oil wells, and then the production can be predicted. However, the four key parameters cannot be obtained for new wells and wells without decline stage, which leads to prediction failure. Therefore, for a new well, although its geological parameters and fracturing parameters are known, its key parameters for the QCM are uncertain. How to establish the relationship between them remains to be further studied.
In recent years, the artificial neural network (ANN) as a non-linear mapping model has been widely used in reservoir injection–production dynamic prediction. On this basis, the sample library is established by collecting data, and the strong adaptive and self-learning functions of the artificial neural network are used to train the sample library, which can achieve good prediction results in oil fields. Li et al. (2008) regard the wavelet basis as the hidden layer of neural network, which has better convergence. It can predict the oilfield production under the conditions of complex geological conditions and uncertain influencing factors and achieve good prediction results. Wang et al. (2017) used a three-layer BP neural network to predict the productivity. Six productivity parameters of shale gas horizontal wells are defined as neural network input layers. The number of neurons in the hidden layer is 12, and the output layer is the average daily gas production of gas wells. The prediction results show that the BP neural network has high prediction accuracy. Tang and Peng (2020) associate reservoir logging parameters, rock mechanics parameters, and fracturing operation parameters with initial productivity. On the basis of single-factor correlation analysis, the learning effect is controlled by setting the error of sample learning. The neural network toolbox of MATLAB is used to predict productivity. This method has high coincidence rate.
The artificial neural network model has a strong advantage in the prediction of oilfield development productivity, but there are few studies on the production performance prediction of multi-fractured shale reservoirs based on the artificial neural network model. At the same time, the training of the neural network model requires a large number of sample data. However, due to the high logging price, the oilfield data are often incomplete, resulting in fewer samples of the oilfield. How to achieve high-precision prediction in a less-sample database has become a problem.
(1) The traditional methods, including the decline analysis method, semi-analytic method, and numerical simulation method, were widely used in the production prediction. However, it is difficult to describe the complex production behavior by the traditional production prediction model.
(2) The quantitative characterization model uses some key parameters with clear physical meaning to describe the changes of oil production and water content in combination with actual production dynamics. However, it is difficult to predict new wells without production history.
(3) The artificial neural network model has a strong advantage in oilfield development productivity prediction because of its strong adaptive and self-learning functions. It will be a more efficient production prediction method by combining the traditional quantitative characterization model with the artificial neural network model.
JG conceptualized the research idea; investigated, visualized, and processed the data; reviewed and edited the paper; and performed formal analysis. JG, JT, and LC performed the methodology. LC and JT supervised the project and were involved in funding acquisition. LC obtained the resources and was involved in project administration. YG and TW were responsible for language modification and reviewing the paper.
This study was supported by the National Natural Science Foundation of China (Nos. 52004033, 51922007, and 51874044).
The authors declare that the research was conducted in the absence of any commercial or financial relationships that could be construed as a potential conflict of interest.
All claims expressed in this article are solely those of the authors and do not necessarily represent those of their affiliated organizations, or those of the publisher, the editors, and the reviewers. Any product that may be evaluated in this article, or claim that may be made by its manufacturer, is not guaranteed or endorsed by the publisher.
Chen, J., Xu, G., Fan, H., Yu, Y., and Wang, L. (2008). Research on a Compound Production Decline Model. Nat. GAS EXPLORTION DEVELOPMENT 2008 (03), 39–41+8586. doi:10.3969/j.issn.1673-3177.2008.03.010
Chen, Y., ZhouLiang, Y. C., Xu, T., and He, Y. (2021). Quantitative Characterization Model of Shale Oil Horizontal Well Production Change. J. Southwest Pet. University(Science Technol. Edition) 43 (05), 97–103. doi:10.11885/j.issn.16745086.2021.03.01.07
Duan, Y., Cao, T., Wang, R., and Wei, M. (20132013). Analysis on Shale Gas Production with the Power Law Exponential Decline Method. J. Southwest Pet. University(Science Technol. Edition) 35 (05), 172–176. doi:10.3863/j.issn.1674–5086.2013.05.025
Guo, X., Ge, D., Qiang, H., Liu, J., and Zhou, B. (2017). Research on Performance Prediction of Flowing Well at Early Production Stage Based on Well Test Data. Well Test. 26 (05), 39–42+46+77. doi:10.3969/j.issn.1004-4388.2017.05.012
Guo, Y., Wang, W., Liu, H., and Hu, X. (2018). Research on the Production Influencing Factors of Shale Gas Multi-Stage Fractured Horizontal Well. BULLETIN SCIENCE TECHNOLOGY 34 (04), 72–78+83. doi:10.13774/j.cnki.kjtb.2018.04.015
He, F., Yue, M., and Yang, L. (2016). A Finite-Element Method for Nonlinear Seepage Flow of Shale Gas. Sci. Sin.-Tech. 46 (02), 127–134. doi:10.1360/N092016-00010
Hou, J., Guo, L., Yuan, F., Du, Q., and Yu, Bo. (2008). Quantitative Characterization of Polymer Flooding Production Performance in Different Reservoirs of Shengli Oilfield. ACTA PETROLEI SINICA 29 (04), 577–581. doi:10.3321/j.issn:0253-2697.2008.04.019
Jia, C., Zou, C., Li, J., Li, D., and Zheng, M. (2012). Assessment Criteria, Main Types, Basic Features and Resource Prospects of the Tight Oil in China. Acta Petrolei sinica 33 (03), 343–350. doi:10.7623/syxb201203001
Li, H., Shi, Y., Yao, Z., Li, X., Song, P., and Tan, L. (2021). Design Optimization and Productivity Prediction of Horizontal Wells in Low-Permeability Conglomerate Reservoir in Well Block Ma18. Spec. Oil Gas Reservoirs 28 (06), 83–90. doi:10.3969/j.issn.1006-6535.2021.06.011
Li, Y., Zhou, W., Zhao, J., and Zhang, L. (2018). Semi-analytical Productivity Calculation and Sensitive Factors for the Multi-Stage Fractured Horizontal Well in Low Permeability Reservoirs. RESERVOIR EVALUATION DEVELOPMENT 8 (02), 52–57. doi:10.13809/j.cnki.cn32-1825/te.2018.02.009
Li, Z., Zhao, Z., Zhong, Y., Li, J., and Liu, D. (2008). Application of Wavelet Neural Network in Oilfield Production Prediction. Pet. Geology. Oilfield Develop. Daqin 27 (06), 52–54. doi:10.3969/j.issn.1000-3754.2008.06.014
Liu, M., Liu, C., Chen, H., and Zeng, M. (2010). Analysis and Comparison of Several Production Decline Models. Energy Conservation Pet. Petrochemical Industry 26 (08), 30–32+36. doi:10.3969/j.issn.1002-641X.2010.8.008
Lu, B. (2018). Application of Production Decline Curve of Shale Gas Well in South Pingqiao Area. Chem. Eng. Equipment 47 (07), 125–127. doi:10.19566/j.cnki.cn35-1285/tq.2018.07.052
Peng, H., Liu, Y., Ran, Q., Wang, X., and Tong, M. (2014). Study on the Horizontal Well Production in Tight Oil Reservoirs. Nat. Gas Geosci. 25 (05), 771–777. doi:10.11764/j.issn.1672-1926.2014.05.0771
Pu, X., Guo, D., Lan, T., He, Y., and Zhao, Y. (2021). Productivity Prediction and Influencing Factors of Low Permeability Reservoirs after Steering Fracturing Stimulation. XINJIANG PETROLEUM GEOLOGY. 42 (01), 76–80. doi:10.7657/XJPG20210110
Sheng, G., Su, Y., and Wang, W. (2019). A New Fractal Approach for Describing Induced-Fracture Porosity/permeability/Compressibility in Stimulated Unconventional Reservoirs. J. Pet. Sci. Eng. 179, 855–866. doi:10.1016/j.petrol.2019.04.104
Su, Y., Han, X., Wang, W., Sheng, G., Yang, Z., He, Y., et al. (2018). Production Capacity Prediction Model for Multi-Stage Fractured Horizontal Well Coupled with Imbibition in Tight Oil Reservoir. J. Shenzhen Univ. Sci. Eng. 35 (04), 345–352. doi:10.3724/SP.J.1249.2018.04345
Su, Y., Sheng, G., Wang, W., Yan, Y., and Zhang, X. (2016). A Multi-media Coupling Flow Model for Shale Gas Reservoirs. Nat. Gas Industry 36 (02), 52–59. doi:10.3787/j.issn.1000-0976.2016.02.007
Su, Y., Wang, W., and Sheng, G. (2014). Compound Flow Model of Volume Fractured Horizontal Well. Acta Petrolei Sinica 35 (03), 504–510. doi:10.7623/syxb201403012
Tang, J., and Peng, C. (2020). Predicting Method of the Initial Productivity for the Horizontal Well in Fuling Shale Gas Reservoirs. Pet. Geology. Oilfield Develop. Daqing 39 (06), 160–167. doi:10.19597/j.issn.1000-3754.201907059
Wang, D., Dong, Y., Sun, D., and Yu, B. (2020). A Three-Dimensional Numerical Study of Hydraulic Fracturing with Degradable Diverting Materials via CZM-Based FEM. Eng. Fracture Mech. 237, 107251. doi:10.1016/j.engfracmech.2020.107251
Wang, Z., Wang, Y., Dong, H., and Wang, X. (2017). Production Main Control Factors Analysis and Productivity Prediction for Shale Gas of Horizontal Well. WELL LOGGING TECHNOLOGY 41 (05), 577–582. doi:10.16489/j.issn.1004-1338.2017.05.016
Wu, L., Guo, X., Luo, W., Zhang, Z., and Zhang, W. (2018). Influence Factors Controlling the Productivity of Horizontal Well by Volume Fracturing in Tight Oil Reservoirs. UNCOVVETONAL OIL & GAS 5 (03), 56–62. doi:10.3969/j.issn.2095-8471.2018.03.009
Xu, G., Yin, H., and Xing, C. (2020). Analysis on Production Decline of Well YP in Fuyu Tight Oil Layer of Daqing Changyuan. Block 49 (07), 1424–1427. doi:10.13840/j.cnki.cn21-1457/tq.2020.07.040
Xu, Y., Hu, Z., Duan, X., Chang, J., and Zhang, Y. (2021). Shale Gas Improved Five-Zone Composite Productivity Prediction Model and its Influencing Factors. Nat. Gas Geosci. 32 (2), 274–287. doi:10.11764/j.issn.1672-1926.2020.09.002
Yan, L., Chen, F., Wang, Z., Yan, Y., Cao, J., and Wang, K. (2020). Challenges and Technical Countermeasures for Effective Development of Shale Oil in China. Pet. Drilling Techiques 48 (03), 63–69. doi:10.11911/syztjs.2020058
Yang, J., Wang, Q., Cao, Z., and Yang, X. (2019). Method to Predict Stop Time of Natural Flow Out of Gusher wells at Halfaya Oil-Field in Iraq. Drilling Prod. Technol. 42 (05), 59–61+9. doi:10.3969/J.ISSN.1006-768X.2019.05.17
Yu, B., Zhao, H., Gao, D., and Hou, J. (2009). Quantitative Law of Production Performance of Polymer Flooding. J. Shengli Coll. China Univ. Pet. 23 (02), 1–3. doi:10.3969/j.issn.1673-5935.2009.02.001
Zeng, F., Guo, J., Xu, Y., and Zhao, J. (2007). Factors Affecting Production Capacity of Fractured Horizontal wells. PETROLEUM EXPLORATION DEVELOPMENT 2007 (04), 474–477+482. doi:10.3321/j.issn:1000-0747.2007.04.016
Zhang, L., Liu, S., Yong, R., Li, B., and Zhao, Y. (2019). EDFM-based Numerical Simulation of Horizontal Wells with Multi-Stage Hydraulic Fracturing in Tight Reservoirs. J. Southwest Pet. University(Science Technol. Edition) 41 (04), 1–11. doi:10.11885/j.issn.1674-5086.2018.11.21.05
Keywords: low-permeability reservoirs, decline analysis method, semi-analytic method, numerical simulation method, quantitative characterization method, artificial neural network
Citation: Gong J, Cao L, Guan Y, Tian J and Wang T (2022) Productivity Prediction Methods of Multi-Fractured Horizontal Wells in Low-Permeability Reservoirs: Progress and Challenges. Front. Energy Res. 10:861333. doi: 10.3389/fenrg.2022.861333
Received: 24 January 2022; Accepted: 14 February 2022;
Published: 09 March 2022.
Edited by:
Qi Zhang, China University of Geosciences Wuhan, ChinaReviewed by:
Xiaopu Wang, China University of Petroleum, ChinaCopyright © 2022 Gong, Cao, Guan, Tian and Wang. This is an open-access article distributed under the terms of the Creative Commons Attribution License (CC BY). The use, distribution or reproduction in other forums is permitted, provided the original author(s) and the copyright owner(s) are credited and that the original publication in this journal is cited, in accordance with accepted academic practice. No use, distribution or reproduction is permitted which does not comply with these terms.
*Correspondence: Lin Cao, MzYzNzY5OTAyQHFxLmNvbQ==
Disclaimer: All claims expressed in this article are solely those of the authors and do not necessarily represent those of their affiliated organizations, or those of the publisher, the editors and the reviewers. Any product that may be evaluated in this article or claim that may be made by its manufacturer is not guaranteed or endorsed by the publisher.
Research integrity at Frontiers
Learn more about the work of our research integrity team to safeguard the quality of each article we publish.