- 1School of Electrical and Information Engineering, Changsha University of Science and Technology, Changsha, China
- 2Hunan Institute of Engineering, Xiangtan, China
- 3Shenzhen Power Supply Bureau Co., Ltd., Shenzhen, China
The Ubiquitous Power Internet of Things (UPIoT) is a concrete manifestation of the Internet of things (IoT) in the power industry, which is a deep integration of the interconnected power network and communication network, realizing full perception of the system status and full business penetration in all links of power production, transmission, and consumption. The introduction of edge computing in UPIoT fully meets the requirements of rapid response, real-time perception, and to some extent, privacy protection. However, there is currently no comprehensive investigation on the application of edge computing technology in UPIoT. First, this paper introduces the development background and construction of UPIoT and its technical architecture. Then the challenges faced by UPIoT in the process of construction are analyzed. Furthermore, the paper elaborates on the functions and features of edge computing, proposes that the support of edge computing technology can solve the challenges of efficient, fast, and secure processing of massive edge data faced by the traditional cloud-based centralized big data processing technology of UPIoT, and analyzes the architecture of the edge computing-assisted UPIoT. For the three typical scenarios of UPIoT, namely power monitoring system, smart energy system and power metering system, the edge computing architecture of the three scenarios are analyzed, and the specific application methods and roles played by edge computing in the three scenarios are also elaborated. Finally, we discuss the challenges of edge computing in UPIoT, in terms of policy challenges, market challenges, and technical challenges, as well as outline the outlooks of the technical challenges.
1 Introduction
The combination of the energy revolution with the digital revolution has led to the development of the fourth industrial revolution. With the application of these new technologies, such as IoT, edge computing, 5G communication, and artificial intelligence (AI) in the power system, the power system is being promoted to become intelligent, digital, and networked. The aim is for these modern technologies to break through the bottleneck of power development and realize 100% renewable electricity. Meanwhile, the development trends of the power grid are to adapt to the diverse needs of “new loads,” improving the flexibility and flexibility of the power grid, and opening up the blue ocean of the digital economy (state grid corporation of China, 2019a). In 2019, the state grid corporation of China set the goal of constructing the UPIoT, which integrates the power network and communication networks through various information sensing technologies, intelligent collection technologies, big data technologies, and other modern technologies to connect massive power-related entities, realizing comprehensive intelligent perception, identification, and management of power equipment, information interaction, and data sharing, as well as rapid response to demand.
With the continuous construction and development of UPIoT, a large number of intelligent terminals and devices are accessed, so the computing model with traditional cloud computing as the core is no longer effective in real-time transmission, computation, and storage for the process of the billions or trillions of data that are generated by heterogeneous massive intelligent terminals. Edge computing, a new computing model proposed under the rapid development of the Internet of Things, artificial intelligence, big data, and cloud computing, which is an open platform that uses network, computing, storage, and application core capabilities as a whole on the side close to the physical environment or data source, and the edge computing platform is deployed in the network measurement close to the data source to provide the nearest end service nearby, so as to get faster network service response and meet the basic needs of the industry in terms of real-time services, application intelligence, security, and privacy protection (Bai et al., 2020). Therefore, some services from the original cloud can be allocated to the edge side of the network for processing, so as to meet the real-time requirements of various tasks while ensuring overall system performance (Sharma and Wang, 2017; Fu et al., 2018; Zhang et al., 2018; Maier and Ebrahimzadeh, 2019; Song et al., 2019; Xu et al., 2019). Especially for supporting ubiquitous IoT, the processing will be done at the local edge computing layer, based on edge computing technology that can localize the computation, analysis, and control to provide a faster response to users without handing over responsibility to the cloud, thus enhancing the processing efficiency and reducing the data processing load in the cloud. Therefore, edge computing technology is naturally similar to UPIoT in terms of agile connectivity, computation, topology, real-time services, data optimization, application intelligence, security, and privacy protection, and can well support the construction of UPIoT.
There is much discussion in the literature of edge computing but very little work has been done towards the edge computing technology that is applied in the UPIoT. For edge computing, some surveys have studied basic characteristics, research challenges, and opportunities of different edge computing paradigms (Shi et al., 2016; Hu et al., 2015; Varghese et al., 2016; Dustdar et al., 2019; Caprolu et al., 2019). The concept of edge computing has been extended to the wider IoT. Scholars have discussed the application of edge computing in some IoT fields (Yu et al., 2017). conducted a survey to examine how edge computing can enhance the implementation and the performance of IoT, and compared the performance of the different loT applications that are based on the EC and cloud computing architectures. Alrowaily and Lu (2018) reviewed the concepts, features, security, and application of edge-computing-enabled IoT as well as its security features in the data-driven world. Porambage et al. (2018) surveyed multiaccess edge computing, and they presented a holistic overview of this paradigm in relation to IoT. The integration of multiaccess edge computing into IoT applications and their synergies are also analyzed and discussed. Pan and McElhannon (2018) investigated the key rationale, the efforts, the key enabling technologies, and typical IoT applications benefiting from edge cloud. Omoniwa et al. (2019) presented a survey on EC-based IoT literature in the period from 2008 to 2018, including services, enabling technologies, and open research issues, and briefly displayed how the EC-IoT was applied in real-life cyber-physical systems, such as the intelligent transportation system or smart grid. Qiu et al. (2020) introduced the concept of industrial IoT (IIoT), and presented the research progress and future architecture of the EC-assisted IIoT. As can be seen above, most articles mainly elaborate on the architecture, key technologies, advantages, and challenges of EC-assisted IoT, and there are few implementation plans and deployments for the specific application scenarios of edge computing. However, for the power grids, it simply explains the application and role of edge computing in the smart grid, and it does not specifically cover the various levels of the power generation, transmission, and distribution of power grids, and there is currently no comprehensive investigation on the application of edge computing technology in UPIoT.
This paper focuses on edge computing in UPIoT and combs through many research achievements concerning edge computing in UPIoT, discussing the architecture of edge computing in UPIoT. Then, the application of the three scenarios is explored, namely power monitoring system, smart energy system, and advanced metering infrastructure; meanwhile, the advantages of applying edge computing in the three scenarios of the UPIoT, in terms of data privacy protection, security, and communication time, are analyzed. Finally, we elaborate on the challenges and future directions for the application of edge computing in UPIoT.
2 Autonomous Ubiquitous Internet of Things in Electricity
Promoting the construction of UPIoT is an important initiative to realize the energy Internet, which is a strategic deployment for the development of the world economy and the upgrading of the world energy infrastructure. UPIoT is essentially a kind of Internet of things, a specific expression and application of Ubiquitous Internet of Things in the power industry. (the state grid corporation of China, 2019b). Around each link of the electric power system, modern information technologies and advanced communication technologies such as mobile Internet and artificial intelligence are fully applied to realize the interconnection of all things and human-computer interaction in each link of the electric power system (state grid corporation of China, 2020). It is a new form of network with a deep integration of traditional industrial technology and IoT technologies, and it is a concrete manifestation of the IoT in the power industry. Overall, it is a smart service system with state awareness, efficient information processing, and convenient and flexible application features. The architecture of UPIoT is shown in Figure 1.
Like IoT, UPIoT also includes a sensing layer, a network layer, a platform layer (management layer), and an application layer.
2.1 Perception Layer (Terminal Layer)
Smart sensing is the core foundation technology of the perception layer of UPIoT (Jiang et al., 2019b). Smart sensing devices are used to collect, monitor, and sense real-time data from end-of-grid operation equipment. The data flow of the power grid operation runs through the whole chain of power generation, power transformation, transmission, distribution, and consumption. For the primary measurement equipment of power system transmission and distribution, part of it is the basic data of the equipment, and the other part is the real-time operation status data of the equipment (Wang et al., 2019). The real-time data is monitored and sensed by the corresponding intelligent monitoring equipment, such as infrared thermometers, monitoring cameras, or inspection robots. For the secondary equipment on the distribution side, such as relay protection devices and electrical energy monitoring equipment, they can collect the system and equipment operation data. The most typical smart sensor of electricity consumption side equipment is the smart meter, which can sense the basic electricity consumption of users in real time and transmit the user’s energy consumption and electric energy trading data to the system platform.
2.2 Network Layer
This is used to achieve efficient and secure data transmission over a wide area between the sensing layer (terminal layer) and the platform layer (Li Z. S. et al., 2018; Wang, 2019). In order to meet the access of different types of sensors, it may include different types of network compositions such as mobile communication, limited IoT, and local area network. They have specific communication protocols and specifications and have strong extensibility. With the rapid development of 5G technology in China, 5G communication technology will be gradually applied in electric power IoT. 5G communication has the characteristics of wide wireless coverage, short transmission delay, system security engagement, and high transmission rate, which is the core technology to realize and build electric power IoT.
2.3 Platform Layer
The data transmitted by the network layer is stored and managed through a unified data center, meaning some of the data can also be shared across departments, subjects, and even industries. Using cloud computing technology, deep learning, big data analysis, and other core technologies, it realizes efficient data processing and IoT management. The platform layer is the basis for realizing advanced applications, and is dedicated to improving collaborative computing and real-time response to meet users’ power supply needs and business response.
2.4 Application Layer
The application layer is the core goal of UPIoT, which provides control and decision support for users, electricity sellers, and grid operators. Through the interconnection of power and information, the application layer realizes the production and operation of the grid, operation management, and related energy services and breeds new business models and emerging businesses. These new models include micro-grid operation and management, operation and management of electric vehicles, campus energy management, and system multi-dimensional resource management.
The shape of UPIoT will gradually evolve as key technologies, such as sensor technology, communication technology, and cloud/edge computing technology, are deepened in the smart grid.
3 Architecture of Edge Computing for Ubiquitous Power Internet of Things
3.1 Edge Computing for Ubiquitous Power Internet of Things
Many new challenges are introduced by the development of the UPIoT. Although current cloud computing has been applied in the UPIoT, it still cannot meet the challenges that we summarize as follows (Chen et al., 2019): 1)low latency requirement, 2)Network bandwidth constraints, 3)Restricted equipment resources, 4)Uninterrupted connection and interaction with the cloud center, and 5)Privacy protection and data security.
In order to overcome these challenges and realize the construction of UPIoT, three key technologies in IoT are important components of its overall architecture: sensor technology, communication technology, and edge computing technology. Among them, edge computing is the important carrier for constructing it, and it is the most important core technology to support the UPIoT in realizing real-time response, short-period data analysis, various types of edge intelligent services, and so on.
Edge computing refers to a new computing model that analyzes and processes a portion of data using the computing, storage, and network resources distributed on the paths between data sources and the cloud computing center (Shi et al., 2016). Edge computing focuses on real-time, short-period data analysis, close to the device side, and it is better able to support local business real-time analysis and intelligent processing. Meanwhile, it has features such as distributed, low latency, high efficiency, and relieves traffic pressure, and it is more efficient and secure compared to simple cloud computing. The main advantages of edge computing applied to the UPIoT are as follows (Industrial Internet Consortium:Edge Computing Task Group, 2018; Du et al., 2021):
3.1.1 Improved System Performance
With edge computing, in addition to collecting and transmitting data to the cloud platform, data collected at the edge can be analyzed and processed in milliseconds. For example, the advanced measurement system in the smart grid, where the user electricity consumption data collected by smart meters is uploaded to the edge intelligent fusion terminal, without uploading tens of thousands of data to the cloud platform for processing. The computing software of the edge platform can analyze these data in real-time and only upload the fused or processed data to the cloud center, which greatly reduces the communication broadband, shortens the data transmission time, and improves the overall performance of the system.
3.1.2 Protected Data Security and Privacy
Cloud platform service providers give customers a comprehensive system of centralized data security protection solutions. However, once centralizing stored data get leaked, it will lead to serious consequences. Edge computing migrates computing closer to the device, avoiding the need to upload data to the cloud, which greatly reduces the risk of private data being compromised or corrupted during transmission, and also reduces the security risk of cyber attacks to the cloud center that result in leakage of all stored data stored.
3.1.3 Reduced Operational Costs
Since cloud computing requires uploading data to the cloud center for processing, cloud computing possesses the characteristics of data migration, bandwidth, and latency of cloud computing, which makes it very expensive to use cloud computing, whereas edge computing can significantly reduce operational costs by reducing the amount of data upload, thereby reducing data migration, bandwidth requirements, and latency.
3.2 Architecture of Edge Computing in the Ubiquitous Power Internet of Things
Thousands of power terminal devices and sensing nodes access the smart grid in a variety of ways, and can sense or control the power grid. These nodes are usually organized or self-organized into various clusters to form edge networks (Cornel–Cristian et al., 2019). By deploying edge computing into the UPIoT, it can locally process massive heterogenous data and the acquisition of signals, solving the problem of fast response and centralized service, and reducing cloud pressure and communication overhead. The edge computing reference paradigm in UPIoT is as shown in Figure 2. The reference paradigm consists of device layer, edge layer, and cloud application layer; its architecture comprehensively describes the characteristics of UPIoT and edge computing. This paper clearly elaborates the inner architecture of the edge computing gateway in the edge layer. In edge computing gateway, which has certain computation resources that can provide a chance to offload part of the workload from the cloud, the edge not only requests service and content from the cloud, but also performs the computing tasks from the cloud. Meanwhile, the gateway can process amounts of data from various terminals, smart devices, and end users of the power terminal layer, and provides a distributed information computing service with large volumes of data and fast responses.
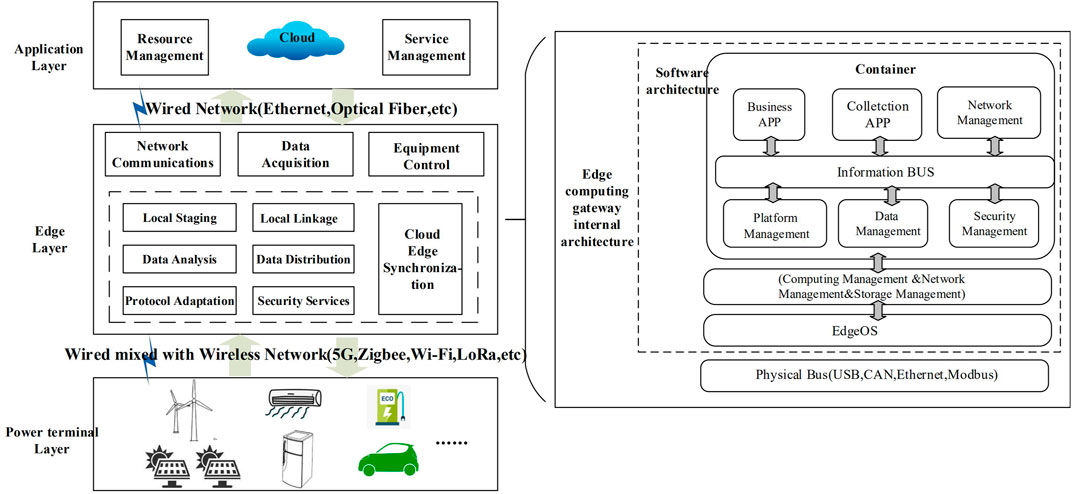
FIGURE 2. Proposed reference architecture of edge computing architecture and the inner architecture of the edge computing gateway in UPIoT.
Edge can perform computing offloading, data storage, caching, and processing, as well as distribute request and delivery service from cloud to user. Under such circumstances, this mode can satisfy the demand of rapid responses required by devices and users in the smart grids, The internal architecture of the edge computing gateway is shown in Figure 2 (Liu et al.,2019); its software system consists of a host operating system and multiple containers. APPs are running inside the container systems; the cloud server and the edge computing device perform data interaction instructions for uplink and downlink, and the terminal node uploads data to the edge computing device, various software applications run on edge computing devices. By encapsulating the application functions as APPs that are loaded in the container, this can provide support for advanced applications of smart grids such as edge autonomy of smart substations, intelligent online monitoring, rapid request distribution, and service delivery.
The application of edge computing in the IoT has attracted a great deal of research. Some typical IoT scenarios are applied, such as smart transportation, smart healthcare, the smart home, and the smart building. We roughly analyze how to apply edge computing in these four scenarios, and then elaborate on the current related articles on the application of edge computing to smart grids. For example, applying edge computing technology to smart transportation, mobile edge computing (MEC) puts the mobile base stations at the edge of the network, and the mobile base stations are deployed in a decentralized manner, providing servers for applications in base stations close to the edge of the network, allowing data to be processed as close to the vehicle and road sensors as possible, thus reducing the round-trip time for data. The server-side application of mobile edge computing can obtain local messages directly from the vehicle and road sensor applications, identify high-risk data and sensitive information that needs to be transmitted in near real-time through algorithm analysis, and send early warning messages directly to other vehicles in the area to facilitate early decision making by drivers to allow nearby vehicles to avoid hazards, slow down, or change routes (Li and Liu, 2017). It provides relatively real-time driving parameters setting guidelines for different vehicles, and the cloud service platform obtains the historical data from the area edge servers in different regions and applies these to the upper-level applications such as vehicle scheduling across regions, violation monitoring, and traffic map construction and updating. For smart healthcare, with the improvement of living standards, the demand for high quality medical and health care is increasing (Rahmani et al., 2018). Healthcare can also be aided by edge computing. Edge computing applied to smart healthcare systems comprehensively improves global healthcare, and at the same time, brings safer, timely, and effective medical assistance to patients in different regions. In the smart medical system, edge computing and 5G technology are combined in the system to achieve real-time patient information acquisition by IoT devices, ensuring low latency and real-time computing through high-quality network transmission and computing power, realizing remote diagnosis, remote surgery, and emergency rescue, providing flexible and personalized medical services for patients, and meeting the patient’s ability to send data about their health condition without leaving home. For example, MEC can help health advisers to assist their patients, independent of their geographical location. MEC enables smartphones to collect patient physiological information, such as pulse rate and body temperature, from smart sensors and send it to the cloud server. Health advisers who have access to the cloud server can immediately diagnose patients and assist them accordingly (Stantchev et al., 2015). Furthermore, the smart home system is the hot application under the IOT, through computer and communication technologies, the system produces an amount of sensitive data locally, and the data collected by the sensor is transmitted to the decision-making unit, which calculates the appropriate control signals to achieve the predetermined goal. The gateway is applied as the edge that is between the home device and the cloud, through internet services, such as Bluetooth,Wi-Fi, and home LAN, to realize locally controled kinds of smart home devices and remotely operated home devices. Therefore, the edge gateway form the home edge network and the intelligent home service platform, reducing uplink data transmission, service cloud platform load, and responding to user needs with ultra-low latency (Trimananda et al., 2018). Moreover, for the smart building, smart building control systems consist of wireless sensors that are deployed in different parts of buildings. Sensors are responsible for monitoring and controlling building environments, such as temperature, gas level, or humidity. In a smart building environment, sensors installed with edge computing are capable of sharing information and become reactive to any abnormal situation. These sensors can maintain the building atmosphere on the basis of collective information received from other wireless nodes. For example, if humidity is detected in the building, MEC can react and perform actions to increase the air in the building and blow out the moisture. As one of the IoT applications, the smart grid is a cyber physical system covering various smart devices. For instance, Tencent Cloud and Pengmai Energy Technology took edge computing into account and released the overall architecture of energy IoT solution in Cloud Tencent and Energy IoT Pengmai (2018). (Okay., 2016).proposed a fog computing-based smart grid model, and presented an example scenario, the smart homes, in terms of latency and security, of the advantages of the model. Sun H. Y. et al. (2019) introduced an edge computing technology for power distribution internet of things (PD-loT), and provided the architecture of edge computing for PD-loT, meanwhile, they analyzed the internal and external interaction mechanism of the data center construction under this architecture and the cloud-side collaboration mechanism based on the data center. Furthermore, they have analyzed the application of edge computing in the typical service of power distribution “orderly charging of electric vehicles.” Li B. et al. (2018) discussed the application of edge computing in demand response, and analyzed the application of edge computing in specific scenarios in the field of power supply and demand, such as home energy gateways, non-intrusive load monitoring, and orderly power management. Gong et al. (2018) proposed a packet transport network (PTN) physical architecture model of active distribution network (ADN) based on edge computation, and constructed a cyber physical system (CPS) management and control model of ADN based on edge computation. Kumar et al. (2016) proposed a generalized architecture for data management based on vehicular delay-tolerant network (VDTN) using edge computing for smart gird, and also proposed an energy efficient virtual machine migration utilizing load forecasting. Zahoor et al. (2018) proposed a three-layered framework named cloud–fog-based smart grid, analyzed the edge computing layer close to the consumers’ region that performs effective management of the network resource with low latency, and considered two scenarios for performance evaluation of their cloud–fog-based smart grid model. Liu et al. (2019) presented an architecture of edge computing-driven autonomous ubiquitous IoT in Electricity, which is based on the edge computing architecture and virtual synchronization technology, and introduced the applications that may be deployed in the edge computing gateways. Chen et al. (2019) introduced the services of IoT-based smart grid supported by edge computing, and proposed an architecture introducing edge computing into IoT-based smart grid, as well as presented the three scenarios of the smart grid. Long et al. (2020) discussed the advantages of edge computing technology used in the demand-side management of power consumption in the smart grid. Khan et al. (2020) highlighted the role of edge computing in realizing the vision of smart cities, and reviewed the state-of-the-art literature focusing on edge computing applications in smart cities, including smart transportation, smart health-care, smart grid, and smart farming.
Following an investigation, we found that there are only a few surveys discussing the current status of the applications of edge computing in UPIoT, since it is too new to have attracted too many people’s attention. This paper will analyze the architecture and application of edge computing in three scenarios of power IoT, including distribution network automation monitoring system, smart energy system, and power metering system, as well as analyze the advantages of introducing edge computing in the three scenarios. It provides a significant reference for follow-up researchers, designers, and beginners.
4 Application of Edge Computing in Ubiquitous Power Internet of Things
As shown in Figure 3 (Chen et al., 2019), applying edge computing technology, IoT technology, and 5G communication technology in three major scenarios, improves the performance of the power system and makes the power system more intelligent and automated.
4.1 Power Monitoring System
The power monitoring system is composed of the control center at all levels, such as substations, power line surveillance, and so on (Tao et al., 2017). The system applies modern control technology, visualization technology, modern communication technology, and Internet of things technology to intelligently monitor power equipment hot spots, power, and environment. It intelligently analyzes data, and realizes comprehensive visualization display and intelligent linkage alarm, at the same time, it effectively assists power equipment informatization, overhaul, and operation. Overall, it serves for smart grid overhaul, operation, and whole life cycle management (Li, 2019). As shown in Figure 4 it mainly describes the two service applications of transmission lines and the intelligent substation in the power monitoring system based on edge computing.
4.1.1 Transmission Line Monitoring
On the one hand, in order to realize the intelligent inspection of the transmission line, the unmanned aerial vehicles obtain the image, video, and other data of the surrounding environment of the power transmission through the fixed-focus camera in the pan-tilt. The front-end edge computing detection module recognizes and locates the acquired video stream and the condition of the overhead line. The application of edge computing device nodes in the power line realizes the autonomous, procedural, and standardized collection of image information in the process of the transmission line equipment. Meanwhile, the acquired images and video image data are processed and analyzed locally, reducing invalid information sent back to the cloud platform, and improving the effectiveness of image data information. This method of utilizing the edge computing detection module that reduces the pressure on broadband reduces the load pressure on the cloud platform, and reduces the complexity of subsequent defect diagnosis. Furthermore, the manual participation in inspection operations is reduced, and the efficiency is improved by 5–7 times. On the other hand, it is neccesary to improve the intelligent level of transmission line monitoring and early warning, operation and maintenance, and overhaul. By deploying PMU, FTU, and other sensing units to collect local information, the data types include second-level switch position signals, fault signals, and minute-level signals. Equipment status data, such as electrical information and connector temperature, partial discharge signal, tower status, and channel status, support normalized operations, such as line inspection and troubleshooting. Among them, electrical information, switch status, and local analysis results will be uploaded to the master station layer as remote information, which realize real-time online monitoring and state detection and improve the intelligent level of environmental monitoring and early warning of important transmission channels, as well as realize intelligent operation, maintenance, and overhaul of the power grid.
4.1.2 Intelligent Monitoring of Substation
With the continuous improvement of the IEC 61850 standard and the continuous improvement and deepening of key technologies such as electronic voltage transformers, current transformers, MU, intelligent terminals, and process layers, as well as the continuous construction and deployment of UPIoT, he substation tends to be digital and intelligent (Tang et al., 2021). The system of the substation monitoring system is divided into process layer, bay layer, and station control layer. Substations need to monitor a huge number of secondary equipment such as lines, switch, and circuit breakers, and collect the electrical quantities of the lines and the on-off status of control equipment through the process-level intelligent integrated device of the terminal, then upload them to the GOOSE/SV network. SV messages and GOOSE messages are standard message formats in the IEC61850 communication protocol, they are the line state value and the switch state value, respectively. These messages are transmitted from the process layer to the bay layer, and the bay layer performs line stability control and relay according to the message content. The protection action is performed, and then protection is uploaded and information is relayed to the station control layer in MMS messages, so as to realize the analysis and processing of the monitoring data. Finally, the remote control host at the station control layer transmits it to the dispatch center through the communication protocol in the monitoring system, and waits for the analysis result and task dispatch of the dispatch center. However, both station domain protection and line protection of substations have extremely low delay requirements, and the impact of factors such as message analysis, communication congestion, and network packet loss will bring harm and hidden dangers to the operation of the power grid. By deploying the edge computing platform at the station control layer, the platform process, and storing part of the information on-site by capturing and parsing MMS messages, this would reduce the burden on telecontrol communications while ensuring the quality of application services (Bai et al., 2020). The architecture of edge computing in the integrated automation system of substation as shown in Figure 5.
4.2 Smart Energy Systems
The smart energy system is an integrated management system, which is made up of a distributed generator, energy storage devices, flexible loads, and energy conversion devices. The integrated energy management platform coordinates the electrical energy interactions in the power network and uses a microgrid central controller, a distributed power grid connection interface device, and an intelligent control terminal to implement the basic functions of the smart energy system.
The edge computing architecture for the smart energy system is shown in Figure 6. The architecture consists of three layers: device layer, edge layer, and cloud layer. The cloud layer takes the cloud platform as the core and provides various cloud services. For different scales, the cloud layer can deploy the public cloud, private cloud, or hybrid cloud. The equipment layer consists of various types of power devices, including uncontrolled distributed power sources such as photovoltaic and wind turbines, controlled distributed power sources such as diesel generators and power conversion devices such as inverters, energy storage devices such as electric vehicle charging piles and batteries, and various types of loads. The edge layer is the core of the entire architecture; it consists of edge gateway, edge platform, and edge services. The edge layer provides computing, storage, application deployment, and other functions at the edge side near the data source of the device. The edge gateway is the core device in the edge computing architecture, which collects the operation data of distributed power supplies, loads, power conversion devices, and energy storage devices in real-time, and then uploads them to the edge platform. Under the coordination of the edge platform, each edge gateway executes the control commands derived from the calculation results at the edge side to control the dispatchable power devices (Xu et al., 2020).
We will analyze the typical applications and the related advantages brought by the architecture based on the EC-IoT smart energy systems, including identification of malicious behavior of electricity consumption and Real-time perception of distributed energy power generation status, efficient data processing, and fast reactive voltage response.
4.2.1 Identification of Malicious Behavior of Electricity Consumption
With the massive access of a large number of distributed energy sources and terminals containing power electronic equipment, the terminal equipment in the smart energy system is vulnerable to permission attacks, data storage and encryption attacks, vulnerability threats, and remote control (Lei et al., 2020). These risks will lead to abnormal activities of the terminal’s feedback data, allowing the micro-grid central controller to collect wrong information and then make wrong decision-making activities, causing the local or even the entire system of the micro-grid to collapse (Komninos et al., 2014; Lei et al., 2020). In order to monitor malicious behaviors of users online in real time, the smart energy system uses the microgrid central controller, distributed energy grid-connected interface devices, and charging piles, as edge computing modules to form an edge computing platform. For example, the edge computing module establishes the power generation behavior of each device with the characteristics of power and time through the distributed energy generation collected in real time. The edge computing module uses electricity consumption and time as parameters for the user’s electricity consumption behavior in the microgrid, and then establishes the user’s electricity consumption behavior pattern and constructs the database for users. Through the edge module, which can extract power generation and power consumption data anytime and anywhere, then realizes data interaction with the main station, identifies the characteristics of power generation and consumption, and realizes the power balance of smart energy and the identification of malicious behavior (Mao, 2020).
4.2.2 Real-Time Perception of Distributed Energy Power Generation Status, Efficient Data Processing, and Fast Reactive Voltage Response
By installing the edge computing equipment on the grid-connected inverter side of photovoltaic and other power generation equipment, the output electrical quantity and fault information of the inverter in real-time can be monitored and the main data can be uploaded to the integrated energy management system. On the one hand, edge computing devices can collect data from grid-connected inverters, box transformers, and combiner boxes in real time. On the other hand, the architecture of the edge computing module is composed of hardware and software, and has embedded control software. When the grid voltage and frequency collected in real time fluctuate abnormally, there is no need to wait for the control instructions of the cloud platform. The control algorithm adaptively controls the power output of the inverter, and quickly responds to the grid voltage and frequency, so as to realize the comprehensive perception of the state of the power generation unit and efficient data processing (Sun et al., 2021), and quickly support the safe and stable operation of the grid.
4.3 Advanced Metering Infrastructure
Advanced metering infrastructure (AMI) consists of smart meters, data concentrators, data centers, and communication networks. AMI is interconnected with the communication network to achieve two-way communication of power data. In the AMI, the smart meters are uploading their power usage information to the data concentrators through wired and wireless communication in the Neighborhood Area Network (NAN). And then the data center actively requests power data from data concentrators through the wide area network (WAN), or data concentrators pass through the WAN at a preset time interval, and they centrally upload power consumption data to the data center, then the data center distributes electricity price information to users and implements related measures such as load management, demand response, and meter control commands to improve customer service (Liang et al., 2021).
With the rapid development of the smart grid, the data generated by smart meters and other power terminal devices has exploded. Facing the computing demand of massive data, traditional cloud computing solutions face huge challenges in transmission bandwidth, transmission delay, data storage, and real-time response. The introduction of edge computing technology and the introduction of edge computing modules in the data concentrator constitute the AMI edge computing framework, as shown in Figure 7. The data collected by the terminal is processed locally in the concentrator, and only the calculation results are uploaded to the cloud, thus reducing the network burden, lowering transmission costs, and meeting users’ real-time response needs, etc.
We will present the typical applications and the related advantages brought by the architecture based on the EC-IoT advanced metering infrastructure, including real-time power forecasting and efficient abnormal detection.
4.3.1 Real-Time Power Consumption Prediction
By adding an edge computing module under the current power concentrator, the massive power metering data is passed to the concentrator, and the edge module is an edge device. Based on the computing power of the edge module, the combination of deep learning and machine learning methods is adopted, such as online learning combined with machine learning algorithms (GBDT, XGBOOST, Linear Regression, etc.), which is used to train the time-series consumption data, then realize the real-time prediction of metering data and real-time feedback of consumption bills and the reasonable strategy of the power consumption to users that meet the demand response. Since the data from the massive power terminals are transferred to the edge device, they are no longer transferred to the cloud, and processed directly by the edge device’s own computing resources, which greatly improves the data processing efficiency. In addition, for the new power data generated every moment, the online learning method can be used to quickly complete training and make predictions, which greatly improves the real-time data processing (Chen et al., 2019).
4.3.2 Efficiently Abnormal Detection
The traditional anomaly detection method relies on cloud computing technology to analyze and process all collected data in the data center. A distributed detection method based on edge computing is proposed, which sets up edge node detectors on the edge side of the grid to collect, store, and detect data directly instead of the original central processor. The edge computing-based detection method transforms the traditional centralized detection into a distributed detection method. Combined with the deep learning approach, the anomaly detection model is constructed, and the training process is separated from the edge nodes and placed at the central node to complete. The distributed detection model of the power metering system based on edge computing is shown in Figure 8. At the edge, the edge node concentrator is responsible for collecting and storing smart meters and related data around them, uploading the processed real-time data and the stored related historical data to the central node, which can perform local processing and detection based on the data they monitor and collect, and sending the trained anomaly detection model down to the edge nodes. In the cloud center, it undertakes the training task of the detection model, and after the model is trained, it is then downlinked to the corresponding edge node. The edge node can realize offline detection, which can realize more efficient and safe monitoring of the power grid and reduce the pressure on the cloud center.
5 Challenges and Opportunity of the Edge Computing in the Ubiquitous Power Internet of Things
The UPIoT seeks to achieve the information-physical-social integration of power energy systems, however, the construction of the UPIoT is still in its infancy at this stage. Although edge computing technology has been gradually applied to various aspects of the power grid, there are still huge challenges to the application of edge computing in the UPIoT. These challenges come partly from the limitations of edge computing technology itself and partly from the potential constraints that exist when edge computing is combined and applied with UPIoT. This section analyzes the market, policy, and technology risks of edge computing in UPIoT applications, and discusses the technical challenges and corresponding outlook for UPIoT based on edge computing.
5.1 Market Challenge
Edge computing enables UPIoT. Constructing UPIoT requires the installation of massive amounts of edge devices, and power operators update traditional solutions to upgrade power infrastructure according to emerging development needs, but it is difficult to quantify its effect on power production, business capacity improvement, and cost reduction, and the corresponding business model is still in the exploration stage. Therefore, the development of the edge computing that is applied in the UPIoT will be faced with market and economic challenges, such as the possibility of investing hundreds of billions of dollars and how to develop operational and business models. Will the return on investment achieve the expected results? And how will the financial crisis be dealt with on the impact of grid planning, investment and operation? All these questions require grid companies, operators, governments, and others to think deeply.
5.2 Policy Challenge
Edge computing realizes the interconnection of power equipment and sensors through the integration of wireless networks, mobile center networks, Internet, and other communication networks. The collection of massive power terminal data must be transmitted to the edge platform through communication, and then the data is processed and analyzed on the edge platform, so it is essential to ensure the security of the edge side network. On the one hand, it is necessary to ensure that data can be reliably and quickly transmitted from the remote end to the platform, and on the other hand, it is necessary to prevent data leakage and resist cyber attacks. Therefore, how to develop new information security protection technologies and systems is a difficult point for edge computing to be applied in the UPIoT, and there is a lack of a unified standard system in the power industry. In Europe and the United States, the development of smart grids is relatively advanced, and the development of security and stability standards is more detailed and comprehensive, For example, the European Telecommunications Standards Institute (ETSI) has defined typical service scenarios and engineering implementation guidelines for edge computing (European Telecommunications Standards Institute, 2016), so the application of edge computing can be promoted in the EU and other countries. However, in China, the security protection of the power secondary system follows the overall protection principle of “security zone, network dedicated, horizontal isolation, and vertical authentication” to ensure the security of the power data network. On the one hand, the standard system related to edge computing is not complete; on the other hand, due to the huge scale of China’s power industry and the relatively conservative policy implementation, it is difficult to apply a new technology immediately. Therefore, in order to realize ubiquitous IOT intelligent sensing, the introduction of edge computing will inevitably lead to network and information security issues, and the relevant specific policies are not yet perfect, so it is difficult to apply and implement edge computing perfectly. Therefore, there are corresponding policy risks for edge computing to be applied to UPIoT, which require in-depth thinking by countries all over the world.
5.3 Technical Challenge
There are many distinctive technical challenges in UPIoT edge computing environments, including management and processing of massive heterogeneous power terminal devices accessing edge side, data offloading and load balancing, edge intelligence, edge network security, data sharing security, and privacy protection.
We summarize these technical challenges in detail and outline potential research directions.
5.3.1 Management and Processing of Massive Heterogeneous Power Terminal Devices Accessing Edge Side
Due to the stage of construction and real-time operation of the power system, a large amount of historical and real-time data, such as control, monitoring, and metering, will be accumulated on the power IoT platform, which constitutes a multi-source heterogeneous data source for the platform layer of the UPIoT. Heterogeneous data sources arise from a variety of power devices, owing to the variety of power devices, the lack of unified access to the edge node standards, and the edge network center node itself which is more difficult to expand. However, moving the location of the edge computing center will bring a lot of delay. Furthermore, in the edge computing network, there are not only static end-devices (e.g., sensors or video cameras), but also dynamic ones such as UAVs and electrical vehicles, making the device management even more challenging. Meanwhile, since different devices have huge differences in hardware configurations and software functions, and the corresponding data computing, storage, and communication capabilities vary, this also offers a challenge (Qiu et al., 2020). Therefore, there is an issue in management of massive heterogeneous power devices accessing the edge nodes, and the edge network must be programmable to support application-specific requirements of edge terminal devices. For this challenge, SDN and NFV technology realize the management and control of multi-source heterogeneous terminal equipment, as well as the scheduling and routing of data flows. SDN and NFV are two of the latest technologies designed to introduce flexibility in network management and orchestration. SDN is mainly characterized by the decoupling of the control plane from the data plane; it provides programmability for network application development and supports the new technology in a unified manner (Bera et al., 2017). SDN and NFV are applied for the edge network. It will make the network more flexible and programmable (Han et al., 2018). Many researchers have carried out certain discussions and research on the application of software-defined network technology in the power Internet of Things, and have achieved relevant results (Yang et al., 2017; Wang et al., 2015; Zhong et al., 2021), However, the combination of edge computing with SDN, the application of which in the UPIoT is in its infancy, will become a trend and is worth in-depth research in the future. Furthermore, utilizing deep learning techniques on the edge computing platform to assist in edge network control for the large-scale heterogenous power terminal devices is also an area of focus research for studies. At the same time, with the development of 5G networks and its application to the power Internet of Things, in the future, we can study the application of 5G technology to UPIoT-assisted edge computing for edge terminal network management; the edge node management issues will be easy to handle in a 5G network (Kumareshan and Poongodi, 2016). Specifically, 5G core networks can collect status information of various nodes regularly (e.g., node location, resource use, task list, and adjacent nodes), monitor and update node management information and strategies, and optimize other strategies such as data processing strategy and network protection strategy according to the collected information. The combination of edge computing with 5G that adapt to the challenge of management and control in the unified access of massive heterogeneous terminals in the UPIoT, is worth exploring in depth in the future. This is only one side. On the other hand, for a large amount of multi-source heterogeneous data, the computing power is deployed at the edge nodes to deal with heterogeneous data. However, in the actual operation of the UPIoT, on the one hand, the algorithm iteration speed is very fast, and multiple versions of calculation programs cannot be stored locally for a long time. On the other hand, the interface types of terminal devices vary greatly due to the influence of operating environment, communication network, and other factors. There are both wired communication interfaces, such as Ethernet, PLC, RS485/232, and wireless communication interfaces, such as 4G/5G public network. There are both traditional wireless business terminals, such as RTU, PTU, and TTU, and intelligent business terminals. Therefore, it is difficult to process a large amount of multi-source heterogeneous data, which affects the efficiency and performance of edge computing. For this challenge, the technologies of UPIoT microservices, algorithm subscription, and container VM technology are worth studying in depth, which can be carried out to improve the flexibility of algorithm deployment, and then promote agile development and rapid iteration of edge computing in the UPIoT.
5.3.2 Realizing Edge Autonomy to Meet the Business Demand for Real-Time Response
With the construction and development of the UPIoT, the amount of power equipment has increased sharply, and the deployment area is also relatively wide. The on-site installation environment is not only complicated but also diverse, resulting in a sharp increase in the workload of installation, commissioning, management, and maintenance of power devices. Although edge computing is introduced, in current UPIoT systems based on edge computing, edge devices can only perform lightweight computing tasks. To enable edge devices and edge servers to perform more complex tasks with a higher data processing performance and lower latency, edge computing combined with artificial intelligence, big data analysis, and other technologies that are applied in the UPIoT assisted edge computing, which make edge devices and servers intelligent. However, edge equipment resources and computing capabilities are limited, it is difficult to achieve lean management, and there exists a challenge of realizing edge autonomy and Intelligence to meet the business demand for real-time response to improve the quality of service (QoS) and quality of experience (QoE).
Many scholars propose some ideas for coping with challenges from two aspects: the edge device itself and the edge model architecture. In terms of edge computing devices, this could be done by adding AI processor modules or re-designing the intelligent chip to improve the computing power of edge devices. In the design of chip architecture, the aim would be to support the edge computation paradigm and facilitate AI models (e.g., DNNs, CNN, etc) acceleration on the resource-limited IoT devices. Meanwhile, customized AI processors are developed to be better suited to specific edge devices and usage scenarios. For example, developing customized power chips and AI edge computing processors (Zhao et al., 2021) to meet the requirements of massive data processing and calculations. However, there exists a great challenge in developing devices more suited to edge AI and realizing the theoretical complementarity of edge computing and AI (Qiu et al., 2020). At present, in support of the intelligence of the edge computing for the UPIoT, the reconstruct of the edge device is not researched in-depth since some emerging technologies and architectures are under development. On the other hand, artificial intelligence models have been deployed at the edge layer. Although machine learning can be used to enhance the intelligence of edge devices, the high complexity of deep learning in many machine learning methods leads to the relatively high difficulty of the deployment. Due to the computing power of edge devices being generally weak, it is necessary to compress and simplify the model, and optimize the model architecture to adapt to the edge system to improve the processing performance. On the one hand, the technology of model compression for the edge device is worth researching since many approaches, such as model compression, conditional computation, and algorithm synchronization, are proposed to improve the efficiency of training and inference of deep AI models that are utilized in the edge. On the other hand, the architecture of the deep learning model is needed to optimize for the UPIoT assisted edge computing, As we know, the goal of the continuous development and construction of the UPIoT is realizing the transition from “collection + centralized control” to “collection + control + regional autonomy,” and gradually shifting from “vertical closure” to “horizontal openness,” The research of cloud-edge collaboration technology has become a developmental trend of edge computing in the UPIoT. Taking smart substation as an example, it will evolve from three stages as an important infrastructure for the construction of UPIoT: edge interconnection to edge intelligence, and then to edge autonomy. Utilizing the cloud-edge collaborative model for the substation, which remains in the initial stage, needs more efforts to be made, and is an effective way to realize the edge of substation autonomy, and can be the focus point for researchers that need to think and study in the next step.
5.3.3 Edge Network, Edge Node Security Protection, and Data Privacy Protection
Although applying edge computing to the UPIoT can bring the advantages of reduced transmitted data volume on the network, communication delay, computational costs, and enhanced flexibility, a large number of terminals accessed to the edge layer inevitably increase data interfaces that may be used as a springboard to attack edge nodes. On the other hand, edge nodes are close to the edge, and the network protection is weak. In the edge computing scenario, edge computing networks are distributed, scalable, and heterogeneous, the security measures of edge servers are weaker than traditional cloud servers, and they are vulnerable to attacks from malicious nodes in the network. Traditional security protection methods cannot satisfy the protection requirements of edge computing, as the security risks cannot be fully considered at the start of the design. Moreover, the integration of various technologies has also intensified the security threats related to data, networks, and applications. In UPIOT based on edge computing, there are challenges of edge network, edge node security protection, and data privacy protection. In response to this challenge, blockchain is being considered as a disruptive technology by academicians and industries that offers potential solutions to solve the security and privacy issues of edge computing networks and devices (Kang et al., 2019; Frey et al., 2019). The incorporation of blockchain and edge computing into a single framework, and then combining the attribute-based access control model, will make it possible to have reliable access and control over the network, storage, and distributed computational resources at the edge. Blockchain technology can also improve the security of the EC-assisted IoT paradigm as it permits only trusted IoT devices/nodes to interact with each other. On the one hand, blockchain-based trusted data management schemes (e.g., lightweight consortium blockchain) for cooperative authentication, authorization, and privacy preserving could be developed (Gai et al., 2019; Mao et al., 2020; Wang et al., 2020), meanwhile, utilizing blockchain to form a security mechanisms for edge nodes/devices could ensure the security and credibility of edge nodes in the UPIoT. These two proposed methods can not only prevent edge nodes from being attacked and lead to data privacy of the problem of leakage, but also ensure the integrity and security of the data sharing using the external network; therefore, it ensures the credibility of regional terminal computing tasks in the edge layer, and then returns the correct calculation results to the cloud and terminal users safely and reliably. Overall, these are potential research directions for studies, and need more research and developments. On the other hand, the combination of blockchain and machine learning methods (such as federated learning, deep learning, reinforcement learning, and deep reinforcement learning) to enhance the access control, secure storage, and privacy -preserving data of EC-assisted UPIoT, can be adopted to detect abnormal behavior, such as energy theft with energy privacy protection in the smart gird (Yao et al., 2019), which is an emerging field and a promising direction for research.
5.3.4 Edge Computation Offloading and Load Balancing Realize Demand Response
UPIoT is a promising solution to meet the increasing electricity demand of modern cities, while challenges face the real-time processing and analysis of huge data collected by the power terminal devices due to the limited computing capability of the devices and long distance transmission from the cloud center. Edge computing enables power terminal tasks to be offloaded on the edge side for in-situ processing. It reduces communication delays and energy consumption. However, the power consumption, computing power, and storage space of edge-layer servers are also limited. Computation offloading and load balancing are great challenges in UPIoT systems based on edge computing.
For the challenge of the data offloading, scholars mostly combine artificial intelligence technologies (Lin et al., 2019; Sun W. et al., 2019) (such as deep reinforcement learning (Dinh et al., 2018; He et al., 2018; Dai et al., 2019; Luo et al., 2019; Min et al., 2019; Zhang et al., 2019a; Zhang et al., 2019b), such as markov chain decision-making, game theory, Lyapunov optimization, machine learning and so on) to improve the performance of computing offloading schemes. However, according to the characteristics of UPIoT, to find the optimal balance between energy consumption, delay, amount of data, bandwidth, it is necessary to design the computation offloading strategy according to the data volume, task type, and equipment capabilities (Jiang et al., 2019a; Pan et al., 2020), which is worthy of in-depth consideration, and is the outline for future studies by researchers at this stage. Furthermore, another challenge is considering how to allocate resources reasonably after making the data offloading decision, that is, the problem of where the resources are distributed. In other words, there is a new problem in that the data offloading scheme may lead to the overload of some edge devices, then creating another challenge of load balancing. Load balancing based on specific characteristics and scenarios of the UPIoT combining new technologies is a significant research direction. Considering the scale and frequency of scheduling are significantly larger, it is necessary to improve the existing load balancing algorithms to adapt the characters of the UPIoT edge system. A typical method depends on the NFV and SDN integrated edge cloud platform to orchestrate the resources to fulfill the offloaded tasks from the battery-constrained edge terminal devices. In UPIoT based on edge computing, using the task data and loading data from the equipment and edge servers, combined hierarchically with AI, a load balancing service can set up the load balancing scheme based on a machine learning model for each layer. In addition, SDN can be utilized to conduct load balancing scheduling from the global perspective of the edge network. To minimize the complexity of scheduling and routing, emerging SDN technology will have a significant impact on the routing scheme and communication mode of edge network, and brings more comprehensive and in-depth routing schemes for edge computing in UPIoT (Kaur et al., 2018; Li X. et al., 2018; Nayak et al., 2018; Al-Hubaishi et al., 2019). At present, some scholars propose to use SDN to establish a grid edge computing model, with minimum delay as the goal orientation, and use deep reinforcement learning to reasonably schedule and allocate computing resources (Shang et al., 2021). However, there is very little research on resource allocation strategies that target how to balance time delay and energy consumption, which is a direction worthy of research. On the other hand, Machine learning algorithms based on resource allocation in edge-cloud architecture, edge-edge architecture, could also solve the problem of load balancing. For example, based on the edge-cloud architecture, the computing tasks of the terminal cannot be completely offloaded to the edge side for execution, so some tasks are offloaded to the remote cloud server for calculation, and the result will be first returned to the edge server, and finally back to the terminal device. If a client’s requirement is more critical, it will be handled by the cloud; otherwise, servicing is done by the edge. However, the reality of how edge computing and cloud computing can work together efficiently and seamlessly is a significant research direction (Li et al., 2020). When considering the computation offloading in edge computing, it is necessary to consider the gaming and cooperation between the edge and the cloud for task scheduling and collaboration.
6 Conclusion
Edge computing integrates network, computing, and storage on the edge of the network. The introduction of edge computing can solve the problems of cloud computing architecture facing the UPIoT, which is unable to handle massive heterogeneous data, communication delays, high computing pressure, data privacy leakage, and difficulty in satisfying user demand response and other issues. First, this article introduces the edge computing technology and the framework of the UPIoT, and gives the architecture of the combination of edge computing and the UPIoT and the internal architecture of the edge computing layer. Moreover, one of the contributions of this paper is to analyze the technical application of edge computing in the three power Internet of Things scenarios: power monitoring system, smart energy system, and power metering system. It also gives the architecture of the edge computing in the three scenarios. Furthermore, the major contribution is putting forward the policy challenges, market challenges, and technical challenges of the application of edge computing in UPIoT, meanwhile, the technical challenges and outlooks in four major areas are analyzed in detail. This paper aims to obtain more attention from other researchers in edge computing in the UPIoT, and make power industry development more rapid and convenient.
Author Contributions
DL conceived the overall structure and framework of the article. HL conceived the outline of the manuscript. QZ and ZZ wrote the manuscript and generated the figures. XZ and ML helped perform the manuscript with constructive discussions. All authors contributed to the article and approved the submitted version.
Funding
This work was supported in part by the National Natural Science Foundation of China (Grant No. 52177068), the Research Project in Hunan Province Education Department (Grant No. 21C0577), Postgraduate Research and Innovation Project of Hunan Province, China (Grant No. CX20210791) and in part by Key Laboratory of Renewable Energy Electric-Technology of Hunan Province (Changsha University of Science and Technology).
Conflict of Interest
ML was employed by Shenzhen Power Supply Bureau Co., Ltd.
The remaining authors declare that the research was conducted in the absence of any commercial or financial relationships that could be construed as a potential conflict of interest.
Publisher’s Note
All claims expressed in this article are solely those of the authors and do not necessarily represent those of their affiliated organizations, or those of the publisher, the editors and the reviewers. Any product that may be evaluated in this article, or claim that may be made by its manufacturer, is not guaranteed or endorsed by the publisher.
References
Al-Hubaishi, M., Çeken, C., and Al-Shaikhli, A. (2019). A Novel Energy-Aware Routing Mechanism for SDN-Enabled WSAN. Int. J. Commun. Syst. 32 (17), e3724. doi:10.1002/dac.3724
Alrowaily, M., and Lu, Z. (2018). Secure Edge Computing in IoT Systems: Review and Case studies[Conference Presentation].”in 2018 IEEE/ACM Symposium on Edge Computing (SEC), Seattle, WA, USA, 440–444. doi:10.1109/SEC.2018.00060
Bai, Y. Y., Huang, Y. H., Chen, S. Y., Zhang, J., Li, B. Q., and Wang, F. Y. (2020). Cloud-edge Intelligence: Status Quo and Future Prospective of Edge Computing Approaches and Applications in Power System Operation and Control. Acta Automatica Sinica 46 (3), 397. doi:10.16383/j.aas.2020.y000001
Bera, S., Misra, S., and Vasilakos, A. V. (2017). Software-Defined Networking for Internet of Things: A Survey. IEEE Internet Things J. 4 (6), 1994–2008. doi:10.1109/jiot.2017.2746186
Caprolu, M., Di Pietro, R., and Lombardi, S. (2019). Edge Computing Perspectives: Architectures, Technologies, and Open Security issues[Conference Presentation]. Milan, Italy: IEEE International Conference on Edge Computing EDGE, 116–123. doi:10.1109/EDGE.2019.00035
Chen, S., Wen, H., Wu, J., lei, W., Hou, W., Liu, W., et al. (2019). Internet of Things Based Smart Grids Supported by Intelligent Edge Computing. IEEE Access 7, 74089–74102. doi:10.1109/ACCESS.2019.2920488
Cloud Tencent and Energy IoT Pengmai (2018). [Online]. Available: https://cloud.tencent.com/solution/energy_iot.
Cornel - Cristian, A., Gabriel, T., Calin-Arhip, M., and Zamfirescu, A. (2019). Smart Grid Integration of IoT.[Conference Presentation] 2019 54th International Universities Power Engineering Conference (UPEC). Bucharest, Romania, 1–5. doi:10.1109/UPEC.2019.8893551
Dai, Y., Xu, D., Maharjan, S., Chen, Z., He, Q., and Zhang, Y. (2019). Blockchain and Deep Reinforcement Learning Empowered Intelligent 5G beyond. IEEE Netw. 33 (3), 10–17. doi:10.1109/mnet.2019.1800376
Dinh, T. Q., La, Q. D., Quek, T. Q. S., and Shin, H. (2018). Learning for Computation Offloading in mobile Edge Computing. IEEE Trans. Commun. 66 (12), 6353–6367. doi:10.1109/tcomm.2018.2866572
Du, Y., Zhang, Z. Y., and Zhao, Y. (2021). Application Review of Edge Computing in Smart Grid. Hubei Electric Power(in Chinese) 45 (3), 72. doi:10.19308/j.hep.2021.03.012
Dustdar, S., Avasalcai, C., and Murturi, I. (2019). Invited Paper: Edge and Fog Computing: Vision and Research Challenges. San Francisco, CA, USA: IEEE International Conference Service-Oriented System EngineeringSOSE, 9609–9696. doi:10.1109/SOSE.2019.00023
European Telecommunications Standards Institute (2016). Mobile Edge computing(MEC)framework and Reference Architecture:GS MEC 003 V1.1.1. Sophia Antipolis, France: [Standard]FranceNiceETSI.
Frey, M., Gundogan, C., Kietzmann, P., Lenders, M., Petersen, H., Schmidt, T. C., et al. (2019). Security for the Industrial IoT: The Case for Information-Centric networking[Conference Presentation]. IEEE 5th World Forum Internet Things (WF-IoT), Limerick, Ireland, 424–429. doi:10.1109/WF-IoT.2019.8767183
Fu, J.-S., Liu, Y., Chao, H.-C., Bhargava, B. K., and Zhang, Z.-J. (2018). Secure Data Storage and Searching for Industrial IoT by Integrating Fog Computing and Cloud Computing. IEEE Trans. Ind. Inf. 14 (10), 4519–4528. doi:10.1109/tii.2018.2793350
Gai, K., Wu, Y., Zhu, L., Xu, L., and Zhang, Y. (2019). Permissioned Blockchain and Edge Computing Empowered Privacy-Preserving Smart Grid Networks. IEEE Internet Things J. 6 (5), 7992–8004. doi:10.1109/jiot.2019.2904303
Gong, G. J., Luo, A. Q., Chen, Z. M., Luan, J. Z., An, X. N., Wang, X. B., et al. (2018). Cyber Physical System of Active Distribution Network Based on Edge Computing. Power Syst. Technol. 42 (10), 3128. doi:10.13335/j.1000-3673.pst.2018.0119
Han, Y. Q., HeLou, Y. Q. F. D., and WangGuo, Y. B. C. X. (2018). Analysis and Application of SDN Based Dynamic Optimal Route Strategy for Cyber Layer in Cascading Failures. cyber-physical Power systemPower Syst. Technol. 42 (8), 2620
He, Y., Zhao, N., and Yin, H. (2018). Integrated Networking, Caching, and Computing for Connected Vehicles: A Deep Reinforcement Learning Approach. IEEE Trans. Veh. Technol. 67 (1), 44–55. doi:10.1109/tvt.2017.2760281
Hu, Y. C., Patel, M., Sabella, D., Sprecher, N., and Young, V. (2015). Mobile Edge Computing-A Key Technology towards 5G. ETSI White Paper 11 (11), 1
Industrial Internet Consortium:Edge Computing Task Group (2018). Introduction to Edge Computing in IIoT. https://production.iiconsortium.org/pdf/Introduction_to_Edge_Computing_in_IIoT_2018-06-18.pdf.(Accessed November 03, 2018).
Jiang, C., Cheng, X., Gao, H., Zhou, X., and Wan, J. (2019a). Toward Computation Offloading in Edge Computing: A Survey. IEEE Access 7, 131543–131558. doi:10.1109/ACCESS.2019.2938660
Jiang, X. C., Liu, Y. D., Fu, X. F., Xu, P., Wang, S. Q., and Sheng, G. H. (2019b). Construction Ideas and Development Trends of Transmission and Distribution Equipment of the Ubiquitous Power Internet of Things. High Voltage Engineering(in Chinese) 45 (5), 1345.
Kang, J., Yu, R., Huang, X., Wu, M., Maharjan, S., Xie, S., et al. (2019). Blockchain for Secure and Efficient Data Sharing in Vehicular Edge Computing and Networks. IEEE Internet Things J. 6 (3), 4660–4670. doi:10.1109/jiot.2018.2875542
Kaur, K., Garg, S., Aujla, G. S., Kumar, N., Rodrigues, J. J. P. C., and Guizani, M. (2018). Edge Computing in the Industrial Internet of Things Environment: Software-Defined-Networks-Based Edge-Cloud Interplay. IEEE Commun. Mag. 56 (2), 44–51. doi:10.1109/mcom.2018.1700622
Khan, L. U., Yaqoob, I., Tran, N. H., Kazmi, S. M. A., Dang, T. N., and Hong, C. S. (2020). Edge-Computing-Enabled Smart Cities: A Comprehensive Survey. IEEE Internet Things J. 7 (10), 10200–10232. doi:10.1109/jiot.2020.2987070
Komninos, N., Philippou, E., and Pitsillides, A. (2014). Survey in Smart Grid and Smart Home Security: Issues, Challenges and Countermeasures. IEEE Commun. Surv. Tutorials 16 (4), 1933–1954. doi:10.1109/comst.2014.2320093
Kumar, N., Zeadally, S., and Rodrigues, J. J. P. C. (2016). Vehicular Delay-Tolerant Networks for Smart Grid Data Management Using mobile Edge Computing. IEEE Commun. Mag. 54 (10), 60–66. doi:10.1109/mcom.2016.7588230
Kumareshan, N., and Poongodi, P. (2016). Dynamic Mobility Management Architecture to Improve Quality of Experience (QOE) in Wireless Networks.”in 2016 10th International Conference on Intelligent Systems and Control (ISCO) CoimbatoreIndia. [Conference presentation].
Lei, W., Xu, A., Lin, H., Hou, W., Zhang, Y., Jiang, Y., et al. (2020). On-line Detection of Malicious Activities Based on Edge Computing in Micro-grid System. J. Phys. Conf. Ser. 1646 (1), 012131–012136. doi:10.1088/1742-6596/1646/1/012131
Li, H. (2019). Research on Intelligent Electric Power Monitoring System Based on Internet of Things Technology. Jilin, China: Jilin university. [master’s thesis].
Li, Q. H., Zhang, Y. J., Chen, J. Q., Yi, Y. Q., and He, F. L. (2020). Development Patterns and Challenges of Ubiquitous Power Internet of Things. Automation Electirc Power Syst. 44 (1), 13. doi:10.7500/AEPS20190726001
Li, Z. Z., and Liu, J. X. (2017). Application of mobile Edge Computing in Internet of Vehicles. Mod. Sci. &Technology Telecommunications 47 (3), 37
Li, B., Jia, B. C., Chao, W. Z., Tian, S. M., QiSun, B. Y., Zhu, W. Y., et al. (2018). Prospect of Application of Edge Computing in the Field of Supply and Demand. Power Syst. Technol. 51 (11), 154. doi:10.11930/j.issn.1004-9649.201712051
Li, X., Li, D., Wan, J., Liu, C., and Imran, M. (2018). Adaptive Transmission Optimization in SDN-Based Industrial Internet of Things with Edge Computing. IEEE Internet Things J. 5 (3), 1351–1360. doi:10.1109/jiot.2018.2797187
Li, Z. S., Xie, R. C., Sun, L., and Huang, T. (2018). A Survey of mobile Edge Computing Telecommunication Science(in Chinese), 34.
Liang, H., Ye, C., Zhou, Y., and Yang, H. (2021). Anomaly Detection Based on Edge ComputingFramework for AMI[Conference Presentation]. China: IEEE International conference on Electrical Engineering and Mechatronics TechnologyQingdao, 385–390. doi:10.1109/ICEEMT52412.2021.9601888
Lin, C.-C., Deng, D.-J., Chih, Y.-L., and Chiu, H.-T. (2019). Smart Manufacturing Scheduling with Edge Computing Using Multiclass Deep Q Network. IEEE Trans. Ind. Inf. 15 (7), 4276–4284. doi:10.1109/tii.2019.2908210
Liu, D., Zeng, X., and Wang, Y. (2019). Edge-Computing-Driven Autonomous Ubiquitous Internet of Things in Electricity: Architecture and Challenges [Conference Presentation]. Changsha, China: The 3rd IEEE conference on Energy Internet and Energy System Integration. doi:10.1109/EI247390.2019.9062125
Long, Z., Wen, B., Chen, X., Wu, J., and Zhang, J. (2020). Demand-side Ubiquitous Electric Power Internet of Things: Architecture, Functionalities and Technologies[Conference Presentation]. 2020 Chinese Control and Decision Conference (CCDC 2020). Hefei, China. doi:10.1109/CCDC49329.2020.9164391
Luo, Y., Zeng, M., and Jiang, H. (2019). Learning to Tradeoff between Energy Efficiency and Delay in Energy Harvesting-Powered D2D Communication: A Distributed Experience-Sharing Algorithm. IEEE Internet Things J. 6 (3), 5585–5594. doi:10.1109/jiot.2019.2903873
Maier, M., and Ebrahimzadeh, A. (2019). Towards Immersive Tactile Internet Experiences: Low-Latency WiFi Enhanced mobile Networks with Edge Intelligence. J. Opt. Commun. Networking 11 (4), B10−B25. doi:10.1364/jocn.11.000b10
Mao, Z. F., Wang, X. C., Jain, D. K., Khan, H., Hongmin, G., and Zhen, W. (2020). A Blockchain-Based Trusted Data Management Scheme in Edge Computing. IEEE Transaction Ind. Inf. 16 (3), 2013. doi:10.1109/TII.2019.2933482
Mao, Z. Q. (2020). Research on Smart Energy Distribution Optimization Scheme Based on Edge Computing. [Chengdu(China)]: University of electronic science and technology of China. [Master thesis].
Min, M., Xiao, L., Chen, Y., Cheng, P., Wu, D., and Zhuang, W. (2019). Learning-based Computation Offloading for IoT Devices with Energy Harvesting. IEEE Trans. Veh. Technol. 68 (2), 1930–1941. doi:10.1109/tvt.2018.2890685
Nayak, N. G., Durr, F., and Rothermel, K. (2018). Incremental Flow Scheduling and Routing in Time-Sensitive Software-Defined Networks. IEEE Trans. Ind. Inf. 14 (5), 2066–2075. doi:10.1109/tii.2017.2782235
Okay, F. Y., and Ozdemir, S. (2016). Tunisia, 1–6. doi:10.1109/ISNCC.2016.7746062A Fog Computing Based Smart Grid Model2016 International Symposium on Networks Computers and Communications(ISNCC)Yasmine Hammamet
Omoniwa, B., Hussain, R., Javed, M. A., Bouk, S. H., and Malik, S. A. (2019). Fog/edge Computing-Based IoT (FECIoT): Architecture, Applications, and Research Issues. IEEE Internet Things J. 6 (3), 4118–4149. doi:10.1109/jiot.2018.2875544
Pan, J., and McElhannon, J. (2018). Future Edge Cloud and Edge Computing for Internet of Things Applications. IEEE Internet Things J. 5 (1), 439–449. doi:10.1109/jiot.2017.2767608
Pan, X., Jiang, A., and Wang, H. (2020). Edge-cloud Computing Application, Architecture, and Challenges in Ubiquitous Power Internet of Things Demand Response. J. Renew. Sust. Energ. 12, 062702. Available. doi:10.1063/5.0014059
Porambage, P., Okwuibe, J., Liyanage, M., Ylianttila, M., and Taleb, T. (2018). Survey on Multi-Access Edge Computing for Internet of Things Realization. IEEE Commun. Surv. Tutorials 20 (4), 2961–2991. doi:10.1109/COMST.2018.2849509
Qiu, T., Chi, J., Zhou, X., Ning, Z., Atiquzzaman, M., and Wu, D. O. (2020). Edge Computing in Industrial Internet of Things: Architecture, Advances and Challenges. IEEE Commun. Surv. Tutorials 22 (4), 2462–2488. doi:10.1109/COMST.2020.3009103
Rahmani, A. M., Gia, T. N., Negash, B., Anzanpour, A., Azimi, I., Jiang, M., et al. (2018). Exploiting Smart E-Health Gateways at the Edge of Healthcare Internet-Of-Things: A Fog Computing Approach. Future Generation Comput. Syst. 78, 641–658. doi:10.1016/j.future.2017.02.014
Shang, L., Cai., S,, Cui, J. B., Ji, C. H., Cui, K. J., and Li, B. G. (2021). SDN-based MEC Resource Allocation of a Power Grid. Power Syst. Prot. Control(in Chinese) 49 (20), 136. doi:10.19783/j.cnki.pspc.201623
Sharma, S. K., and Wang, X. (2017). Live Data Analytics with Collaborative Edge and Cloud Processing in Wireless IoT Networks. IEEE Access 5, 4621–4635. doi:10.1109/access.2017.2682640
Shi, W., Cao, J., Zhang, Q., Li, Y., and Xu, L. (2016). Edge Computing: Vision and Challenges. IEEE Internet Things J. 3 (5), 637–646. doi:10.1109/jiot.2016.2579198
Song, C., Zhang, M., Zhan, Y. Y., Wang, D. S., Guan, L. Y., Liu, W., et al. (2019). Hierarchical Edge Cloud Enabling Network Slicing for 5G Optical Fronthaul. J. Opt. Commun. Networking 11 (4), B60−B70. doi:10.1364/jocn.11.000b60
Stantchev, V., Barnawi, A., Ghulam, S., Schubert, J., and Tamm, G. (2015). Smart Items, Fog and Cloud Computing as Enablers of Servitization in Healthcare. Sensors Transducers 185 (2), 121–128.
State Grid Corporation of China, (2019b). Full Deploys of the Construction of Ubiquitous Power Internet of Things. http://h5ip.cn/ozIF (Accessed April 16, 2019).
State Grid Corporation of China (2019a). The Outline of the Construction of the Ubiquitous Power Internet of Things Official Release. http://www.chinasmartgrid.com.cn/news/20190311/632172.shtml (Accessed March 11, 2019).
State Grid Corporation of China, (2020). Ubiquitous Power Internet of Things Key Construction Task Outline in 2020. https://shupeidian.bjx.com.cn/html/20191211/1027517.shtml (Accessed December 2, 2019).
Sun, W. W., He, G. Q., Liu, C., Zhao, W. R., and Qi, L. W. (2021). Research and Application of Key Technologies for Edge Computing Equipment Used in Photovoltaic Power Generation under Background of IoT. Electric Power Automation Equipment 41 (7), 38–43.
Sun, H. Y., Zang, J. C., Wang, P., Lin, J. Y., Guo, C., and Chen, L. (2019). Edge Computation Technology Based on Distribution Internet of Things. Power Syst. Technol. 43 (12), 4314. doi:10.13335/j.1000-3673.pst.2019.1750
Sun, W., Liu, J., and Yue, Y. (2019). AI-enhanced Offloading in Edge Computing: When Machine Learning Meets Industrial IoT. IEEE Netw. 33 (5), 68–74. doi:10.1109/mnet.001.1800510
Tang, B., Li, T., Li, B., Chen, L. Z., Yang, M., and Liu, S. Y. (2021). Analysis and Display of Intelligent Deployment and Control Data in Substations Based on Edge Computing. Eng. Des. 40 (2), 79
Tao, H., Zhou, J., and Liu, S. (2017). A Survey of Network Security Situation Awareness in Power Monitoring System.”in IEEE Conference on Energy Internet and Energy System Integration (EI2). Beijing,china. doi:10.1109/EI2.2017.8245487
Trimananda, R., Younis, A., Wang, B., Xu, B., Demsky, B., and Xu, G. Vigilia: Securing Smart home Edge Computing.”in 2018 Third ACM/IEEE Symposium on Edge Computing. Seattle, WA, USA(2018), 74–89.
Varghese, B., Wang, N., Barbhuiya, S., Kilpatrick, P., and Nikolopoulos, D. S. (2016). Challenges and Opportunities in Edge computing[Conference Presentation]. New York, USA: IEEE International Conference Smart Cloud, 20–26. doi:10.1109/SmartCloud.2016.18
Wang, J., Wu, L. B., Wu, L., Choo, K.-K. R., and He, D. (2020). Blockchain-based Anonymous Authentication with Key Management for Smart Grid Edge Computing Infrastructure. IEEE Trans. Ind. Inf, 16, 1984–1992. doi:10.1109/tii.2019.2936278
Wang, J. Y., LiuWu, C. J. M., Zhang, X. J., and Yu, H. (2015). Research of Software Defined Service Resource Equitable Allocation Technology of Power WAN. Power Syst. Technol. 39 (5), 1425. doi:10.13335/j.1000-3673.pst.2015.05.038
Wang, Y., Chen, Q. X., Zhang, N., Feng, C., Teng, F., Sun, M. Y., et al. (2019). Fusion of the 5G Communication and the Ubiquitous Electric Internet of Things: Application Analysis and Research Prospects. Power Syst. Technology(in Chinese) 43 (5), 1575–1585. doi:10.13335/j.1000-3673.pst.2019.0635
Wang, Z. H. (2019). Researches and Applications of Safety Program for Power Internet of Things Relying on Cloud-Computing Services. Tech. Automation Applications(in Chinese) 38 (12), 93
Xu, C. K., Wu, C. H., Shu, J., Yang, Z. Y., and Zhang, H. R. (2020). An Edge Computing Architecture of Distributed Generation Microgrid and its Application. Adv. New Renew. Energ. 8 (5), 383. doi:10.3969/j.issn.2095-560X.2020.05.006
Xu, X., Li, D., Dai, Z., Li, S., and Chen, X. (2019). A Heuristic Offloading Method for Deep Learning Edge Services in 5G Networks. IEEE Access 7, 67734–67744. doi:10.1109/access.2019.2918585
Yang, Y., Lv, Y. X., and Liu, Z. W. (2017). Research on a Software-Defined Power Communication Network Service-Aware Traffic Dispatch Mechanism. Automation and Instrumentation 8, 146. doi:10.14016/j.cnki.1001-9227.2017.08.146
Yao, D., Wen, M., Liang, X., Fu, Z., Zhang, K., and Yang, B. (2019). Energy Theft Detection with Energy Privacy Preservation in the Smart Grid. IEEE Internet Things J. 6 (5), 7659–7669. doi:10.1109/jiot.2019.2903312
Yu, W., Liang, F., He, X., Hatcher, W. G., Lu, C., Lin, J., et al. (2017). A Survey on the Edge Computing for the Internet of Things. IEEE Access 6, 6900
Zahoor, S., Javaid, S., Javaid, N., Ashraf, M., Ishmanov, F., and Afzal, M. (2018). Cloud–fog–based Smart Grid Model for Efficient Resource Management. Sustainability 10 (6), 1154–1160. doi:10.3390/su10062079
Zhang, J. L., Zhao, Y. C., Chen, B., Hu, F., and Zhu, K. (2018). Survey on Data Security and Privacy-Preserving for the Research of Edge Computing. J. Commun. 39 (3), 1
Zhang, K., Leng, S., Peng, X., Pan, L., Maharjan, S., and Zhang, Y. (2019a). Artificial Intelligence Inspired Transmission Scheduling in Cognitive Vehicular Communications and Networks. IEEE Internet Things J. 6 (2), 1987–1997. doi:10.1109/jiot.2018.2872013
Zhang, K., Zhu, Y., Leng, S., He, Y., Maharjan, S., and Zhang, Y. (2019b). Deep Learning Empowered Task Offloading for mobile Edge Computing in Urban Informatics. IEEE Internet Things J. 6 (5), 7635–7647. doi:10.1109/jiot.2019.2903191
Zhao, J. J., Yang, R. Y., Zhang, X. Yu. D. P., Zhang, X. T., and Yuan, X. D. (2021). Design and Analysis of the Special AI Video Identification Chip for the Power Industry in Edge Computing of Smart Coal-Fired Power Plants. Energ. Sci. Technol. 19 (05), 41
Keywords: power internet of things, UPIoT, edge computing, edge computing applications, edge computing architecture, smart grid
Citation: Liu D, Liang H, Zeng X, Zhang Q, Zhang Z and Li M (2022) Edge Computing Application, Architecture, and Challenges in Ubiquitous Power Internet of Things. Front. Energy Res. 10:850252. doi: 10.3389/fenrg.2022.850252
Received: 07 January 2022; Accepted: 27 January 2022;
Published: 22 February 2022.
Edited by:
Fengji Luo, The University of Sydney, AustraliaCopyright © 2022 Liu, Liang, Zeng, Zhang, Zhang and Li. This is an open-access article distributed under the terms of the Creative Commons Attribution License (CC BY). The use, distribution or reproduction in other forums is permitted, provided the original author(s) and the copyright owner(s) are credited and that the original publication in this journal is cited, in accordance with accepted academic practice. No use, distribution or reproduction is permitted which does not comply with these terms.
*Correspondence: Dongqi Liu, bGl1ZG9uZ3FpQGNzdXN0LmVkdS5jbg==