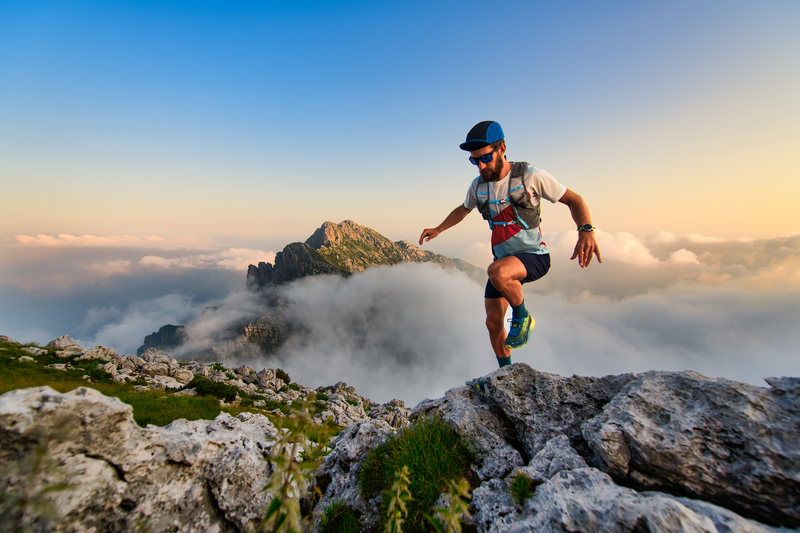
94% of researchers rate our articles as excellent or good
Learn more about the work of our research integrity team to safeguard the quality of each article we publish.
Find out more
ORIGINAL RESEARCH article
Front. Energy Res. , 26 April 2022
Sec. Process and Energy Systems Engineering
Volume 10 - 2022 | https://doi.org/10.3389/fenrg.2022.831462
This article is part of the Research Topic Spotlight on Europe: Process and Energy Systems Engineering View all 8 articles
Electricity markets are currently experiencing a period of rapid change. The intermittent nature of renewable energy is disrupting the conventional methods used in operational planning of the electrical grid, causing a shift from a day-ahead forecast policy to a real-time pricing of delivered electric power. A path towards a more renewable, robust and intelligent energy system is inevitable but poses many challenges to researchers and industry. In the field of process industry, strategies based on demand side response are receiving attention and could represent a partial solution for this challenge. Coordination between production scheduling and procurement of electric power is of high importance and can contribute to reducing cost and emissions associated with production. A methodology to quantify such benefits is presented here with a case study, which reveals the potential benefits of flexible operation. In this case, the minimum compensation for flexibility services ranges between 5 and 20 € per unit (MWh) of restricted power. However, such a compensation depends on geographic location (electricity prices) and the frequency of restrictions. The method follows a rolling scheduling approach that provides optimization of the short-term schedule. This work introduces the concept of representing flexible processes as ‘equivalent batteries’ which store electricity from low-cost periods as intermediate products and consume the embedded energy during high-cost periods. Cost related to providing flexibility combined with the profits from optimized process scheduling contribute toward monetization of flexibility as an ancillary service for the grid. Balancing this service with the cost of implementing DSR solutions provides a means for calculating a pricing strategy for grid flexibility.
Renewable technologies have seen a considerable market penetration in the past few years, translated by a decrease in 5% of the carbon dioxide (CO2)-equivalent intensity, from 308 to 290 gCO2-eq. per kWhel, between 2017 and 2018 (ENTSO-E, 2019). Deep electrification paired with renewable-based generation has been identified by Europe as the main pathway for achieving a climate neutral economy by 2050. Future outlooks show that the share of electricity in final energy demand will at least double by the same year, reaching 53%, and electricity production will increase by up to 2.5 times current levels, depending on the options selected for the energy transition (EUR-Lex, 2018). Reliable operation of the electricity grid is a fundamental requirement for this transition, but theconventional approach is constantly embattled by developments in variable generation, distribution outages and unexpected load changes (Albadi and El-Saadany, 2008). Reliable power systems must guarantee a constant balance between supply and demand, which is achievable by efficient communication and flexible relations between suppliers and consumers (Alizadeh et al., 2016). In this regard, demand side response (DSR) can play a significant role in handling variability of electricity systems and therefore contribute towards balancing the grid. Moreover, the progressive electrification of numerous sectors could trigger competitive markets in ancillary services and encourage industry to provide responsive loads. Fair remuneration strategies should be identified to balance the incremental operating cost and open the door to flexible industrial consumers.
Recently, the allowance of unconventional grid resources such as demand response (DR) (Ela et al., 2016) contributed to grid balancing and improved the quality of the supplied electric power. Grid customers can benefit from lower wholesale market prices, increased reliability and system security (Siano, 2014), while guaranteeing favorable conditions to the grid operator. Peak load reduction translates into reducing requirements for expensive generation reserves and avoided capacity costs, such as the need for distribution and transmission infrastructure upgrades (Aghaei and Alizadeh, 2013). DR techniques based on real-time load shifting can additionally support variable generation (Denholm and Hand, 2011), fostering the proliferation of distributed renewable energy resources as power generation devices connect to the grid.
DSR studies are oftentimes linked with grid management and planning strategies, particularly on the power and thermal nexus. Numerous studies are focused on urban design and scheduling which is, among all society sectors, the one showing the largest demand flexibility (Golmohamadi, 2022). However, problems frequently faced in managing and balancing the grid at urban level are similar to those faced at industrial scale, both amplified by the recent increase in renewable energy penetration. A common challenge is to shift flexible loads to periods with high-renewable generation share, when the price and associated grid emissions are lower, simultaneously decreasing the need for storage capacity. Although a few industrial sectors possess hard constraints on operation, due to interdependent processes that do not allow interruption, some others are able to accommodate changes, hence potentially providing ancillary services to the power grid. This industrial demand response feature is a complex task, not only because it demands the ability to store (intermediate) products but also because it requires changes in production patterns as frequently as changes in grid demand, triggered by price signals. However, being able to do it can have a large impact in reducing demand on request (Otashu and Baldea, 2020), due high power consumption and lack of some limitations (e.g., comfort constraints), which restrain the applicability of some DR solutions in other sectors such as the residential one.
As pointed out by (Shahnewaz Siddiquee et al., 2021), multiple industrial demand response studies have been carried out to promote a more sustainable industrial sector and potentially reduce production associated costs. (Summerbell et al., 1972017) showed the financial and environmental savings in using a flexible schedule in the cement industry, reducing costs and emissions by close to 4%, whereas (Ma et al., 2020) incorporated uncertainty in price signaling by developing a robust mixed-integer quadratic program cement plant self-scheduling operation, showing the importance of material flows storage in providing flexibility to the power system. (Bao et al., 2020) applied a hierarchical model predictive strategy to an aluminium plant, using a mixed integer quadratic formulation, highlighting the potential benefits of participating in an ancillary services framework. (Alarfaj and Bhattacharya, 2019) developed a mixed integer nonlinear programming structure to address an oil refining energy management system, showing that participating in a DR provisioning system significantly reduces peak demand as the system is more resilient and able to respond faster to grid signaling.
(Zhang et al., 2018) showed how ancillary services are provided by industrial power load coordination and energy storage features. Real-time and day-ahead operations are tackled by a model predictive control determining hourly operation and an optimal scheduling approach for daily operation, respectively. (Kelley et al., 2019) addressed a scheduling production based on time-dependent grid emissions. The model, a simplified mixed-integer linear programming (MILP) representation, embedded dynamic features directly on the scheduling procedure, consistently yielding savings on costs and emissions when compared with constant production strategy. More recently, (Vergara-Fernandez et al., 2022), developed a MILP approach capable of scheduling a water supply system based on two major decisions: optimal hourly operating load and optimal electricity use with hourly consumption profile. However, no storage or buffer units are used, jeopardizing the enhanced feature of a rolling scheduling approach.
Contingency services are based on contracts between utility providers and customers (Tuan and Bhattacharya, 2001), which stipulate payments for load interruption using peak load reduction programs. Attempts have been made by researchers to formulate methods for designing such contracts, starting from the application of non-linear models based on Game Theory (Fahrioglu and Alvarado, 2000), to more recent solutions that exploit pool-based mechanisms for market clearing, aiming at maximising the social welfare of the participants through reschedulable demand (Samadi et al., 2010; Papadaskalopoulos and Strbac, 2013; Luo et al., 2019), or by a coordinated electricity-heat auction as proposed by (Wang et al., 2019). Although such methods provide effective pricing techniques in flexible power systems (for instance (Wang et al., 2019) showed average energy cost savings of 20%, while guaranteeing adequately supply levels), they do not use realistic models for quantifying consumer marginal costs due to power restrictions. This lack of complete methods for ensuring fair sharing of costs and benefits among stakeholders has motivated the research presented in this work. Our method allows quantification of the minimum financial compensation for industrial consumers to provide flexible load shifting services. Such results could either represent a starting value for the stipulation of fair contracts between stakeholders, or a minimum bid in a competitive and liberalized market of ancillary services that might be incorporated in industrial business model plans, as discussed in (Behrangrad, 2015).
In this work, we investigate the effect of responsive loads on marginal cost due to power restrictions imposed on industrial consumers by the grid operator. The method follows a demand side response strategy for optimal operations scheduling with corrective actions when unexpected events occur, i.e. power restrictions. A prediction-based optimizer with a 24-h time horizon is applied to an industrial batch process and simulated over 1 month with hourly shifts of the rolling window. A scheduling model is embedded in the controller using a MILP formulation and solved at each iteration of the algorithm. Restrictions on the power consumption are simulated using the Monte Carlo method with pseudo-random drawings from a Sobol sequence, and implemented as operating constraints. The intensities of the power restrictions are calculated as percentage deviation from the optimal power profile, which is obtained by operating the process in unperturbed state. We also introduce the concept of industrial processes as equivalent batteries that allow flexible operation by storing electricity as intermediate product during certain periods and consuming it in others. Finally, we analyse the effect of power constraints on process performance and investigate the influence of their intensity and frequency on the marginal cost by simulating the process in different European countries.
The process is divided into two sub-systems producing different types of final products, A and B. Each product is characterized by its sequence that uses only one raw material (raw A and raw B), as shown in Figure 1. Raw materials are converted to products through a number of independent jobs which comprise single or multiple operations, each of them requiring only electricity. In total, there are 17 operations to be accounted for, belonging to 10 jobs (details provided in Tables 1 and 2). The intermediates produced by each job can either be stored or directly sent to the next job in the sequence, with instantaneous material transfer. The batch size is fixed and equal among all operations, leading to a constant batch processing time. Changeovers are disregarded. A total of five production lines can be used for scheduling the operations. The process runs at 70% (production requirements provided in Table 3) of its full load capacity reflecting real operating conditions of the case study: the industrial production of shower gel (product A) and conditioner (product B).
FIGURE 1. Scheme of the use case batch process, comprising 10 jobs and 17 operations (Tables 1 and 2). Intermediates have dedicated storage units with finite capacity.
TABLE 2. Power demand [kW] and processing time (in no. of time slots) of operations in sub-system B.
TABLE 3. Delivery schedule (April 2018) in number of batches of raw materials and final products (production demand).
The formulation of the scheduling problem is based on a discrete time representation. The time window is divided into intervals of equal duration (20 min). Each iteration of the algorithm therefore involves solving the scheduling problem over a single day rolling window for a total of 72 time slots. The duration of the time step was selected as the smallest common factor among all processing times required by the operations. Based on this representation of the time horizon, the following variables 1 are introduced:
• xt,r,j,p binary variable1 if operation p of job j is scheduled in resource r at time t, 0 otherwise.
• Im,t continuous variable representing the level of inventory of material m at time t. m is defined within M, a set that contains all materials involved in the system (raw materials, intermediates and final products).
The two variables x and I are subject to constraints that represent process requirements and external impositions such as deliveries of raw materials and demand satisfaction. The constraints (1–12) can be identified:
1. Not allowed resources: Eq. 1 restricts the usage of resources for operation p, excluding those which cannot process job j.
where ARj is the set of the allowed resources and Oj is the sequence of ordered operations, also denoted as
2. Allocation constraints: Ensures that at most one operation is scheduled in production line r at time t, as expressed in (Eq. 2).
Additionally, a second relation should ensure a proper duration of the scheduled operations. This is achieved by preventing an operation from starting before the end of the previous operation on the same production line (Eq. 3).
with T′ = ⟨t,… ,t + RTj,p − 1: h ≤ tn⟩ and Z a sufficiently large positive number calculated reflecting the problem size:
3. Initialisation constraints: These constraints simplify the problem in the early time slots. Three main constraints can be defined. The first (Eq. 4) avoids scheduling an operation if insufficient time has passed to conclude all previous operations within the same job. This constraint accounts for the schedule of the previous iteration and avoids re-scheduling jobs that have already started.
where RTj, v is the required processing time in number of time slots,
where T′′ = ⟨t1, … , RTj,p − Cr,j,p⟩ are the first RTj,p − Cr,j,p time slots allocated to operation p of job j. Moreover, to ensure proper sequencing in the early time slots, a third constraint must be added,
that is valid only if
4. Operations precedence: The constraint in Eq. 7 stipulates that an operation cannot start if the previous one within the same job has not been scheduled.
5. Operations sequence: Job operations must be performed sequentially and without stops since storage is not available between operations of the same job (Eq. 8). This constraint can be formulated similarly to Eq. 7 with the difference being that an operation is set to 1 if its previous one in the sequence
6. Material sufficiency: Eq. 9 ensures that a job cannot start processing if the level of inventory of a material Im,t is insufficient. The constraint is formulated by introducing a new set JRm denoting all the jobs requiring m and defined as a proper subset of J (JR ⊂ J).
where Im is the level of inventory at the beginning of the schedule (Eq. 10). This parameter is initialized to the level of inventory at the step length s (Im,s) of the previous iteration, where s is equal to the number of time slots shifted at each iteration of the rolling window. RI is the raw materials and intermediates set contained in M (RI ⊂ M). The other two sets introduced in Eq. 9, DD and RM, represent the delivery dates and raw material sets (RM ⊂ RI), respectively. Finally, DSt, m is the delivery size, in number of batches, of material m at the start of time slot t.
7. Inventory balance: This constraint ensures the mass balance in each inventory of material m. It is constructed as a sum of contributions as shown in Eq. 11.
where RTj is the total time required by job j
8. Maximum storage capacity: Allows the storage capacity to be constrained for each intermediate m in the intermediates set I (m ∈ I). This constraint (Eq. 12) can be written in the optimization problem as:
where SCm is a parameter representing the maximum storage capacity of m.
The auxiliary parameter Cr,j,p is used to link two consecutive iterations. The results of the optimized schedule at iteration i are passed to the next iteration i+1 by setting C of iteration i+1 (for simplicity called Ci+1), equal to an additional auxiliary variable (y) that is calculated once the optimal schedule of iteration i is found. yi allows the identification of the operations that are not ended at iteration i and must be considered in the next moving window, i+1. This variable has the same structure as C and its value depends on the step length s. Since this method considers day-ahead spot-electricity prices and a time-slot duration (w) of 20 min, the step length is set to three. The relations (13–16) can then be identified:
with
Finally, the scheduling variable x allows the calculation of the total electricity power consumption at time t, denoted by Pt, through the relation expressed in Eq. 17.
where T‴ = ⟨t − RTj,p + 1, … , t: q ≥ t1⟩ and ej,p is the power required by p. The minimization of the operating cost can be finally expressed using the variable Pt as shown in Eq. 18:
where ct is the electricity price at time t.
The rolling scheduling model is solved on an hourly basis with continuity between iterations. The day-ahead electricity price forecasts are queried from the ENTSO-E API at the end of each hour (i) and converted into input data for the next iteration (i + 1). The operation scheduling problem is then solved and the optimal decision variable x is found to schedule the operations on the available production lines for the next hour. The procedure (Figure 2A) is repeated until the iteration counter reaches its maximum allowed value N, namely the total number of simulated hours. At the end of each iteration, the scheduling variable x is used to derive variables I and y as provided in Eqs. (11), (14). These two variables are converted into parameters and injected again into the model by means of the relations expressed in Eqs. (10), (13). The algorithm is run for the considered countries allowing the identification of the optimal power consumption profile. N is set to 720 for a single month simulation or to 4,344 for a 6-month simulation. The obtained profiles represent the best-case scenarios, in which operations can be scheduled under optimal operating conditions. Deviations from such profiles constitute sub-optimal solutions and are due to the introduction of external perturbations.
FIGURE 2. Flow diagram of the on-line scheduling algorithm with rolling window approach. (A) Rolling process. (B) Modified rolling process.
Power limitations imposed by the grid operator are simulated and their effect on the system performance is quantified regarding additional operating costs incurred by the process. Given the optimal power consumption profile
where λt, within the interval [0, 1], is the power factor representing the intensity of the restriction and TC is a proper subset of T (TC ⊂ T) containing the time slots for which a power constraint is imposed. The newly defined constraint is added to the scheduling problem and the rolling process model modified as depicted in Figure 2B. The parameter tstart, within the interval [1, s ×N], represents the initial time slot of the rolling window that is randomly selected for the power restriction. Together with TC and λt, tstart constitutes a 3-dimensional space in which power curtailments can be applied to the process for different times and variable intensities. A Sobol sequence is used to draw samples and simulate the process with a Monte Carlo approach that ensures low discrepancy of the explored space. After each draw the variables are fed into the modified scheduling problem that is solved for a total of Nmin iterations. The latter parameter Nmin represents the minimum number of iterations required by the controller before converging to the unperturbed state Popt.
The execution of the modified algorithm enables analysis of system behaviour when unforeseen events such as power restrictions occur, causing operations re-scheduling and therefore deviations from the optimal profile. Balancing the increased operating cost due to the implementation of such a DSR-based control strategy and the grid service offered by the process, will promote the definition of a methodology for pricing electrical flexibility related to the severity of the power restriction (EUR/MWh). Finally, the effect of restriction frequency on the incurred cost can be investigated by testing different sizes of the set TC.
Figure 3A shows the concept of the process as an equivalent battery plotted together with the electricity prices for Germany in the month of April 2018. The state of charge is defined as the cumulative electrical energy that is consumed to produce intermediates which are stored in buffers. The results show that the buffers are either charged or discharged during times of low electricity prices and kept constant in high-cost periods. Flat segments in the equivalent battery profile do not necessarily indicate a complete production stop but simply that the produced intermediates are directly sent to the next job in the sequence instead of being stored. In such time periods, the process runs at the lowest possible capacity to minimize the operating cost, while still meeting the delivery schedule (according to Table 3).
Power restrictions from the grid operator would be expected either during periods of high price (reflecting high demand) or low renewable generation; however, a relationship between price and restriction could not be established from existing literature. Therefore, restrictions on power consumption are simulated by assuming random behaviour of the grid operator. The latter can require the process to completely or partially cut its power demand at any time of the schedule with notification ranging from one to 24 hours. Whenever the process is required to decrease its consumption, the schedule is completely updated and the process incurs some operating losses when compared to the optimal schedule. Figure 3B shows the deviation of the modified power profile (in red) from the optimal one (in blue) due to four restrictions with hourly duration for a total of 1.9 MWh. The convergence to the optimal consumption profile is reached after approximately 73 iterations of the modified rolling process (Figure 2B) as shown by the vertical dashed line in Figure 3B. All electricity demand restrictions simulated in this study are found to converge in less than 80 iterations; therefore, Nmin was set to this value.
The same procedure is repeated several times using different frequencies and restriction intensities. The results of the simulations for Germany are illustrated in Figure 4, which shows the incremental operating costs associated to electricity consumption in Euros per MWh of constrained electrical energy. Each point in the plot represents a simulation and different colors are used for each tested frequency f, measured in restrictions per day. Linear trends were identified in the results with increasing slope related to restriction frequency, meaning that the number of power constraints imposed on the process impacts marginal operating cost. Moreover, it is observed that the obtained linear relations divide the graphs into two parts. The unfavourable zone is located in the lower side of the plot and represents situations that are likely unprofitable for the industrial customer. Any financial compensation placed in this zone and paid by the grid operator to the service provider would often be insufficient to compensate financial losses incurred by the consumer. Conversely, contracts for provision of a flexibility service that are placed above the marginal cost line can be defined as potentially favourable. Here, industry can either profit from providing grid services or potentially incur financial losses if load shifting techniques, such as the one presented in this work, are not adopted.
FIGURE 4. Marginal operating costs due to power restrictions (Germany). Correlations are denoted in the upper left corner. y and x represent cost variation and curtailed power, respectively. The slope is the remuneration (EUR/MWh) required by the showcased industry to behave as an equivalent battery or, otherwise stated, the minimum bid in a liberalized market of ancillary services. The value in brackets () is the curtailment frequency, f. (A) April 2018. (B) January–june 2018.
Moreover, Figure 4 shows that the maximum admissible severity of a single restriction depends on f. This effect is represented by the different lengths of the marginal cost lines. Indeed, each single restriction cannot exceed a maximum threshold to avoid infeasible solutions of the schedule and to allow the process to meet its delivery requirements.
By comparing Figures 4A,B, it is also noticeable that the correlations do not vary considerably for different durations of the time horizon. Similar slopes of the linear fits are obtained by constricting the power over a single month (April 2018) or a 6-month period (January - June 2018). The relative differences between linear correlations of same frequency in the two periods reach a maximum value of 3.3% for f = 7. It can be concluded therefore, that results are relatively independent of the time horizon and are thus applicable throughout the year with little to be gained from examining extended periods of time.
A similar effect to the influence of frequency f on the maximum admissible severity of a restriction is observed on the standard deviation of the simulated points (Table 4). The results reveal more scatter around the average values, identified by the marginal cost lines, at lower frequencies. Furthermore, the probability that a power restriction strategy would generate either very high or very low marginal cost is inversely proportional to the number of restrictions per day. This effect is demonstrated by the decreasing standard deviations of distributions associated to the tested frequencies. Table 4 gathers all the results obtained by testing the method in seven countries of the Euro zone for which a total of 4,773 simulations are collected. A similar behavior to that obtained for Germany can be deduced. Although the minimum compensation for flexibility services is dependent on the user case, it ranges between 5 and 20 €/MWh of restricted power (as of April 2018) for the set of European countries considered, further highlighting the dependency on geographic location (electricity prices) as well as the strategy adopted concerning the frequency of restrictions.
TABLE 4. Simulation results for each country as of April 2018. The number of simulations is given between brackets in superscript. f, the curtailment frequency, is measured in power restrictions per day.
In this work, we propose a new methodology for pricing industrial flexibility as an ancillary service for electrical grids. Such a compensation is calculated in terms of service marginal cost per unit of restricted electrical energy and it represents the minimum indemnity that would encourage an industrial consumer to participate in grid balancing by load shifting. The service is achieved by implementing a demand side response strategy that minimizes the incremental cost through reactive response. Similar DSR solutions could be either used to shift energy consumption to off-peak hours or to aid grid operators in balancing variable generation from renewable sources.
Additionally, we introduce the concept of industrial processes as flexible storage solutions for demand response with respect to the electrical grid. From the perspective of the electrical grid, the processes can be regarded as a battery with capacity related to their buffer capacity. Decoupled process operations, as in the case study used herein, can therefore mimic storage by temporally displacing operations with large energy requirements. Overall, only electricity consumption was considered, discarding other energy demands such as thermal needs. However, in the contest of electrification of end-use sectors, DSR strategies based on reactive load allocation, such as the one here proposed, become key for the reliability of the power system.
The findings of this work show that the minimum bid to participate in a competitive and liberalized market of ancillary services varies depending on the intensity and frequency of power restrictions. Similar marginal cost relations are found for almost all of the European countries considered. The proposed method provides a new quantitative approach to estimate costs associated with industrial flexibility for providing grid services and is applicable for any industrial batch process. The concept of industrial processes as equivalent batteries introduces possibilities to extend the work for optimal scheduling and resource sharing between partners in an industrial cluster. This framework also provides the basis for equitable contract negotiation between partners and with grid operators, which should be explored further in future work. Sensitivity of the impact of schedule capacity on marginal cost should also be investigated.
The raw data supporting the conclusions of this article will be made available by the authors, without undue reservation.
AS: Conceptualization, methodology, formal analysis, writing—original draft, editing. IK: Conceptualization, methodology, supervision, writing—review. RC-A: Formal analysis, writing—editing, review. FM: Conceptualization, Supervision, writing—review.
This work was supported by the European Union’s Horizon 2020 research and innovation program under grant agreement No. 723575 (Project CoPro) in the framework of the SPIRE PPP, the Marie Sklodowska-Curie grant agreement No 754354, and the Swiss Competence Center for Energy Research SCCER-EIP.
The authors declare that the research was conducted in the absence of any commercial or financial relationships that could be construed as a potential conflict of interest.
All claims expressed in this article are solely those of the authors and do not necessarily represent those of their affiliated organizations, or those of the publisher, the editors and the reviewers. Any product that may be evaluated in this article, or claim that may be made by its manufacturer, is not guaranteed or endorsed by the publisher.
1Variables are expressed using italic font while roman font is used for parameters.
Aghaei, J., and Alizadeh, M.-I. (2013). Demand Response in Smart Electricity Grids Equipped with Renewable Energy Sources: A Review. Renew. Sustain. Energ. Rev. 18, 64–72. doi:10.1016/j.rser.2012.09.019
Alarfaj, O., and Bhattacharya, K. (2019). Material Flow Based Power Demand Modeling of an Oil Refinery Process for Optimal Energy Management. IEEE Trans. Power Syst. 34 (3), 2312–2321. ISSN 1558-0679. doi:10.1109/TPWRS.2018.2889773
Albadi, M. H., and El-Saadany, E. F. (2008). A Summary of Demand Response in Electricity Markets. Electric Power Syst. Res. 78 (11), 1989–1996. doi:10.1016/j.epsr.2008.04.002
Alizadeh, M. I., Parsa Moghaddam, M., Amjady, N., Siano, P., and Sheikh-El-Eslami, M. K. (2016). Flexibility in Future Power Systems with High Renewable Penetration: A Review. Renew. Sustain. Energ. Rev. 57, 1186–1193. doi:10.1016/j.rser.2015.12.200
Bao, P., Zhang, W., Cheng, D., and Liu, M. (2020). Hierarchical Control of Aluminum Smelter Loads for Primary Frequency Support Considering Control Cost. Int. J. Electr. Power Energ. Syst. 122, 106202. November 2020. ISSN 0142-0615. doi:10.1016/j.ijepes.2020.106202
Behrangrad, M. (2015). A Review of Demand Side Management Business Models in the Electricity Market. Renew. Sustain. Energ. Rev. 47, 270–283. ISSN 1364-0321. doi:10.1016/j.rser.2015.03.033
Denholm, P., and Hand, M. (2011). Grid Flexibility and Storage Required to Achieve Very High Penetration of Variable Renewable Electricity. Energy Policy 39 (3), 1817–1830. doi:10.1016/j.enpol.2011.01.019
Ela, E., Milligan, M., Bloom, A., Botterud, A., Townsend, A., Levin, T., et al. (2016). Wholesale Electricity Market Design with Increasing Levels of Renewable Generation: Incentivizing Flexibility in System Operations. Electricity J. 29 (4), 51–60. doi:10.1016/j.tej.2016.05.001
ENTSO-E (2019). Transparency Platform Restful API - Central Collection and Publication of Electricity Generation, Transportation and Consumption Data and Information for the Pan-European Market. Available at: https://transparency.entsoe.eu (Accessed October 13, 2019).
EUR-Lex (2018). Communication from the Commission to the European Parliament, the European Council, the Council, the European Economic and Social Committee, the Committee of the Regions and the European Investment Bank. A Clean Planet for All a European Strategic Long-Term Vision for a Prosperous, Modern, Competitive and Climate Neutral Economy. COM/2018/773 final.
Fahrioglu, M., and Alvarado, F. L. (2000). Designing Incentive Compatible Contracts for Effective Demand Management. IEEE Trans. Power Syst. 15 (4), 1255–1260. doi:10.1109/59.898098
Golmohamadi, H. (2022). Demand-side Management in Industrial Sector: A Review of Heavy Industries. Renew. Sustain. Energ. Rev. 156, 111963. ISSN 1364-0321. doi:10.1016/j.rser.2021.111963
Kelley, M. T., Baldick, R., and Baldea, M. (2019). Demand Response Operation of Electricity-Intensive Chemical Processes for Reduced Greenhouse Gas Emissions: Application to an Air Separation Unit. ACS Sustain. Chem. Eng. 7 (2), 1909–1922. ISSN 2168-0485, 2168-0485. doi:10.1021/acssuschemeng.8b03927
Tuan, L. A., and Bhattacharya, K. (2001). “Interruptible Load Management within Secondary reserve Ancillary Service Market,” in IEEE Porto Power Tech Proceedings (Cat. No.01EX502), Porto, Portugal, 10-13 Sept. 2001. doi:10.1109/PTC.2001.964571
Luo, G., He, Y., Zhao, C., Zhang, X., Lin, S., and Zhao, Y. (2019). “Coordinated Wholesale and Retail Market Mechanism for Providing Demand-Side Flexibility,” in 2019 IEEE Sustainable Power and Energy Conference (iSPEC), Beijing, China, Wednesday, November 20 – Sunday, November 24, 2019, 2569–2574. doi:10.1109/iSPEC48194.2019.8975245
Ma, S., Zhang, Y., Liu, Y., Yang, H., Lv, J., and Ren, S. (2020). Data-driven Sustainable Intelligent Manufacturing Based on Demand Response for Energy-Intensive Industries. J. Clean. Prod. 274, 123155. ISSN 0959-6526. doi:10.1016/j.jclepro.2020.123155
Otashu, J. I., and Baldea, M. (2020). Scheduling Chemical Processes for Frequency Regulation. Appl. Energ. 260, 114125. doi:10.1016/j.apenergy.2019.114125
Papadaskalopoulos, D., and Strbac, G. (2013). Decentralized Participation of Flexible Demand in Electricity Markets-Part I: Market Mechanism. IEEE Trans. Power Syst. 28 (4), 3658–3666. doi:10.1109/TPWRS.2013.2245686
Samadi, P., Mohsenian-Rad, A.-H., Schober, R., Wong, V. W. S., and Jatskevich, J. (2010). “Optimal Real-Time Pricing Algorithm Based on Utility Maximization for Smart Grid,” in 2010 First IEEE International Conference on Smart Grid Communications, Gaithersburg, MD, USA, 4-6 Oct. 2010, 415–420. doi:10.1109/SMARTGRID.2010.5622077
Shahnewaz Siddiquee, S. M., Howard, B., Bruton, K., Brem, A., Dominic, T., and O’Sullivan, J. (2021). Progress in Demand Response and It’s Industrial Applications. Front. Energ. Res. 9. ISSN 2296-598X. doi:10.3389/fenrg.2021.673176
Siano, P. (2014). Demand Response and Smart Grids-A Survey. Renew. Sustain. Energ. Rev. 30, 461–478. doi:10.1016/j.rser.2013.10.022
Summerbell, D. L., Khripko, D., Barlow, C., and Hesselbach, J. (1972). Cost and Carbon Reductions from Industrial Demand-Side Management: Study of Potential Savings at a Cement Plant. Appl. Energ. 197, 100–113. ISSN 0306-2619. doi:10.1016/j.apenergy.2017.03.083
Vergara-Fernandez, L., Aguayo, M. M., Moran, L., and Obreque, C. (2022). A MILP-Based Operational Decision-Making Methodology for Demand-Side Management Applied to Desalinated Water Supply Systems Supported by a Solar Photovoltaic Plant: A Case Study in Agricultural Industry. J. Clean. Prod. 334, 130123. ISSN 0959-6526. doi:10.1016/j.jclepro.2021.130123
Wang, D., Hu, Q. e., Jia, H., Hou, K., Du, W., Chen, N., et al. (2019). Integrated Demand Response in District Electricity-Heating Network Considering Double Auction Retail Energy Market Based on Demand-Side Energy Stations. Appl. Energ. 248, 656–678. ISSN 0306-2619. doi:10.1016/j.apenergy.2019.04.050
Keywords: demand side response, real-time scheduling, optimization, industrial scheduling, flexible operations, energy compensation policy
Citation: Santecchia A, Kantor I, Castro-Amoedo R and Maréchal F (2022) Industrial Flexibility as Demand Side Response for Electrical Grid Stability. Front. Energy Res. 10:831462. doi: 10.3389/fenrg.2022.831462
Received: 08 December 2021; Accepted: 02 March 2022;
Published: 26 April 2022.
Edited by:
Valerie Eveloy, Khalifa University, United Arab EmiratesReviewed by:
Mariano Martín, University of Salamanca, SpainCopyright © 2022 Santecchia, Kantor, Castro-Amoedo and Maréchal. This is an open-access article distributed under the terms of the Creative Commons Attribution License (CC BY). The use, distribution or reproduction in other forums is permitted, provided the original author(s) and the copyright owner(s) are credited and that the original publication in this journal is cited, in accordance with accepted academic practice. No use, distribution or reproduction is permitted which does not comply with these terms.
*Correspondence: Alessio Santecchia, YWxlc3Npby5zYW50ZWNjaGlhQGVwZmwuY2g=
Disclaimer: All claims expressed in this article are solely those of the authors and do not necessarily represent those of their affiliated organizations, or those of the publisher, the editors and the reviewers. Any product that may be evaluated in this article or claim that may be made by its manufacturer is not guaranteed or endorsed by the publisher.
Research integrity at Frontiers
Learn more about the work of our research integrity team to safeguard the quality of each article we publish.