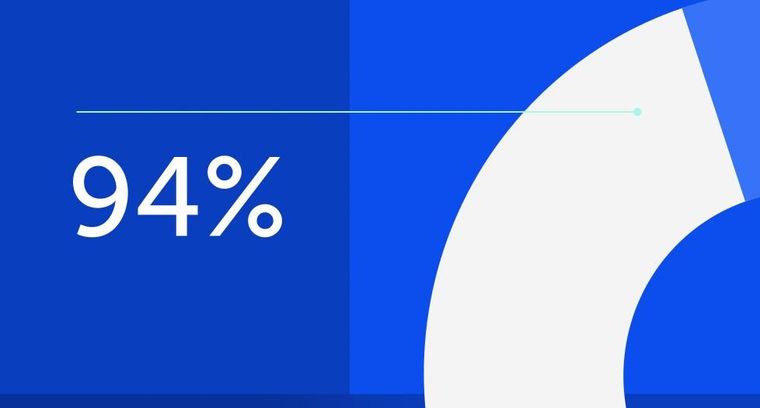
94% of researchers rate our articles as excellent or good
Learn more about the work of our research integrity team to safeguard the quality of each article we publish.
Find out more
ORIGINAL RESEARCH article
Front. Energy Res., 07 March 2022
Sec. Bioenergy and Biofuels
Volume 10 - 2022 | https://doi.org/10.3389/fenrg.2022.830236
This article is part of the Research TopicSustainable Aviation FuelsView all 26 articles
This work analyses, experimentally and numerically, the combustion behavior of three aviation fuels: a standard Jet A-1, a high aromatic content fuel, and an isoparaffinic Alcohol to Jet (ATJ) fuel. The goal is to demonstrate the ability of a chemical kinetic model to capture the chemistry underlying the combustion behavior of a wide range of jet fuels, starting from compositional information. Real fuels containing up to hundreds of components are modeled as surrogates containing less than 10 components, which represent the chemical functionalities of the real fuel. By using an in-house numerical optimizer, the fuel components and their relative quantities are selected, and a semi-detailed kinetic model (containing about 450 species) is used to simulate the formation of the main oxidation products and reaction intermediates. Calculations are compared with species profiles measured in a laminar flow reactor to validate the model and provide insights into the reactivity of the fuels. Finally, starting from the results, general observations on the strengths and limits of the approach are provided, highlighting areas where further investigations are required.
Hard-to-decarbonize sectors, such as aviation, require mid-to long-term solutions to meet climate change mitigation targets. According to the recent reports emerging from the 2050 Waypoint project (Aviationbenefits, 2021), an Air Transport Action Group initiative, the greatest opportunity for decarbonizing the aviation sector comes from an aggressive transition to sustainable aviation fuels (SAFs): Hydroprocessed Esters and Fatty Acids (HEFA), Fisher Tropsch, Alcohol to Jet (ATJ), and Power to Liquid (PtL) fuels. The diversity of resources from which SAFs can be produced, will unavoidably expand the chemical complexity of future fuels.
For this reason, programs such as JETSCREEN (European Commission, 2022) have been supported by the EU to effectively tackle the critical process of fuel optimization, qualification and approval. The final goal is to provide tools that, by means of experimental tests and accurate models, will be capable of assessing a priori the compatibility of new SAFs (and mixtures of SAFs and traditional fuels) with existing infrastructures (i.e., engines, fuel system). In this framework, a key step is the capability of correctly predicting relevant combustion properties such as auto-ignition propensity, laminar flame speed and pollutants emissions (e.g., soot) starting from compositional information. The development of detailed chemical kinetic models serves the goal of predicting fuel behavior ahead of possible experimental campaigns for any new candidate fuel or fuel component that might be of interest in the area of SAFs.
Specifically, when a novel fuel mixture emerges, simpler multicomponent fuel surrogates are developed by means of optimization algorithms that take into account compositional data (e.g., GCxGC data) and other target properties such as viscosity, density, distillation curve, heat of combustion, H/C ratio, smoke point, etc. In the context of kinetic modeling, such surrogates are typically composed of 3–10 compounds, which, mixed together, allow to match the properties of the fuel under investigation. For each of these components a dedicated kinetic subset (i.e., a network of elementary chemical reactions) is developed to model its combustion properties (e.g., ignition delay times, laminar flame speed, intermediate and by-products formation). Such models are validated by means of comparisons with experimental data available in literature for pure components and their blends in 0-D or 1-D laminar reactors and flames, where the chemical kinetic effects are entirely, or at least significantly, decoupled from heat and mass transfer phenomena. Validated kinetic models can then be used to perform targeted parametric analysis to unravel temperature, pressure and composition dependency of the combustion characteristics in such simple systems. Furthermore, skeletal model reduction can be used to bring down the size of such models, making them applicable to large scale (2-D or 3-D) fluid dynamic simulation of real, or close-to-real, systems. This approach can speed up the fuel screening process and support the optimization of combustion devices, favoring the full market implementation of SAFs. Indeed, even by exercising the model on simple systems, it is possible to draw relevant conclusions about the ignitability of mixtures, their burning velocities, their soot propensity and blending behavior.
On these premises, this work analyzes, experimentally and numerically, the combustion behavior of three aviation fuels: a standard Jet A-1 (A1), an isoparaffinic Alcohol to Jet fuel (B1), and a high aromatic fuel (C1). The standard jet fuel, formulated in a previous study (Pelucchi et al., 2021), is used as a reference, while the renewable ATJ fuel (derived from iso-butanol) and the high aromatic content fuel, present extreme compositional features: the ATJ is almost entirely constituted by a single highly-branched iso-alkane, while the high aromatic fuel contains unusually high fractions of mono- and di-aromatics. Compositional information and global composition indexes such as H/C ratio and average molecular weight for the three fuels are reported in Table 1.
TABLE 1. Mass composition (%mass), H/C ratio, average molecular weight and density of the three fuels.
While other literature works discuss the development and validation of fuel surrogates and models for specific fuels (Dooley et al., 2010; Kim et al., 2014; Liu et al., 2019; Prak et al., 2022), this paper focuses on the methodological aspects involved in the definition of general models aiming at capturing fundamental aspects of the fuel chemistry. In this work, combustion chemistry models for three highly diverse fuels are obtained by coupling a single comprehensive kinetic mechanism and a surrogate formulation approach incorporating compositional information. The fuel models are then validated against well-characterized kinetic data from the DLR flow reactor. The final goal is to demonstrate how the workflow here presented allows capturing the speciation profiles of a broad range of fuels with high accuracy and enables the analysis of the relative behavior of the fuels on a more fundamental level. The rationale is that reaction intermediates are strictly related to the composition of the active radical pool that controls fuel oxidation and ultimately determines global combustion characteristics such as auto-ignition, flame behavior and soot formation. This successful validation, therefore, represents an important step towards predictive models for new candidate fuels able to predict features of practical interest such as burning velocity, emissions (Saffaripour et al., 2014; Pelucchi et al., 2021), high altitude relight (Martinos et al., 2021), and lean blow off (Yi et al., 2009; Rock et al., 2021).
The first step towards the development of validated models for the combustion of jet fuels is the experimental evaluation of their oxidative behavior in well-characterized conditions. To achieve this goal, species profiles for selected fuels have been measured by DLR in a high-temperature flow reactor coupled to a molecular beam mass (MBMS) spectrometer. This set up allows for in-depth investigation of relevant combustion chemistry features by identifying simultaneously multiple intermediates and, therefore, reaction channels controlling the formation of products (Oßwald and Köhler, 2015). The species profiles measured provide useful validation data for the development of detailed chemical kinetic model, enabling the assessment of the impact of fuel composition on emissions in technical combustors. Figure 1 provides an overview of the experimental apparatus. Since a comprehensive literature was recently produced on this specific experimental setup (Köhler et al., 2018; Bierkandt et al., 2019; Chu et al., 2019), only a brief description is given here.
FIGURE 1. Schematic DLR high-temperature flow reactor and photographs from (Oßwald and Köhler, 2015). The zoomed cutout shows a detailed view of the sampling interface and the ion source. Note that the high-temperature oven is mounted on moveable rails and sampling is performed inside the tube at ambient pressure.
The system can be divided into two segments: a high-temperature laminar flow reactor including gas supply and a vaporizer system, and a molecular beam mass spectrometry (MBMS) time-of-flight detection (TOF) system. The reactor exit is positioned to the sampling nozzle of the MBMS-TOF system and gas is sampled directly from the reactor outlet and transferred to the high-vacuum detection system.
The reactor features a ceramic tube (total length of 1,497 mm); a laminar flow of highly diluted (> 99% Ar) mixture is fed into the reactor. The high dilution suppresses significant volumetric heat release allowing a better control on the temperature profile in the reactor. The relatively large inner diameter (40 mm) allows minimizing boundary effects. A commercial setup (Bronkhorst, CEM) is used in the mixture preparation section to vaporize the fuel. All input streams are metered in high precision (accuracy ±0.5%) by Coriolis mass flow meters. The high dilution of the system also guarantees the complete evaporation of the fuels, whose partial pressures are maintained below100 Pa.
Operating conditions are designed to yield constant carbon flow at slightly rich (Φ = 1.2) and lean (Φ = 0.8) conditions, respectively. Oxygen concentration is adjusted according to the desired stoichiometry. The exact stoichiometry was determined by measuring the hydrogen content of the fuels using low resolution pulsed NMR (ASTM D7171). The heteroatoms content is assumed to be negligible. The obtained H-content is summarized in Table 2 with the respective inlet flow conditions.
Homogeneous flow conditions are obtained by feeding the premixed gases through a tempered flange equipped with a porous bronze plug. The reaction segment is 1,000 mm long, and is contained in customized high-temperature oven (Gero, Type HTRH 40-1,000), capable of reaching temperatures up to 1900 K. Samples taken at the reactor exit are transferred to high vacuum (10−6 mbar) by a two-stage differential pumping system. The rapid expansion quenches chemical reactions immediately by lowering temperatures and concentrations, effectively “freezing” the composition. Detection is carried out using an electron impact (EI) time-of-flight (TOF) mass spectrometer (Kaesdorf, mass resolution R = 3,000). This system is able to determine the elemental composition of combustion intermediates within a C/H/O system. Soft electron energies are applied (10.6 eV) to avoid species fragmentation during the ionization process. Additionally, a quadrupole mass spectrometer is positioned in the ionization chamber (off beam) and operated at a higher electron energy (70 eV) to track major species contemporarily to the MBMS-TOF measurements. Details on the experimental setup, including a schematic and its instrumentation, may be found in previous publications (Oßwald and Köhler, 2015; Köhler et al., 2018).
A monotonically decreasing temperature ramp (−200 K/h) is applied to the oven and all measurements are performed maintaining a constant inlet mass flow. A temperature window spanning from 600 to 1200 K was scanned, covering all regimes between the absence of reactions to full conversion and thermal equilibrium. The flow regime is laminar for all the temperature conditions, but previous studies demonstrated how this system can be successfully simulated treating the system as one-dimensional, adopting a predefined axial temperature profile derived from the experiments and the plug flow hypothesis for kinetic calculations (Oßwald and Köhler, 2015; Kathrotia et al., 2017). Temperature profiles along the reactor axis were measured during the temperature ramps, providing the necessary boundary condition for the kinetic model.
The quantitative evaluation of the species was performed adopting well established techniques (Herrmann et al., 2013; Schenk et al., 2013; Oßwald and Köhler, 2015) performing direct binary (species/Ar) calibration measurements or estimating the ionization cross section based on the RICS (Relative Ionization Cross Section) method. Calibration by direct cold gas measurements was performed for most species. The estimation procedure (RICS) was applied for all radicals, as well as for C2H2O, C7H8, C8H6, C8H8, C9H8, C12H8, C12H10, C13H10, and C14H10. Note that species predominantly showing a fuel-like behavior (i.e., maximum concentration at low temperature) are calibrated internally using the respective fuel composition determined by two dimensional gas chromatography (GCxGC) obtained by IFPEN.
For the three fuels, more than 500 quantitative species profiles could be obtained at two equivalence ratio conditions. Results are obtained as a function of the oven temperature. Further details about the experimental set-up and additional data are available in (Oßwald et al., 2021). Figure 2 summarizes the major species (product and reactants) for all initial compositions.
A similar global reaction behavior was observed for all fuels with moderate temperature shifts across the fuels. The highly-branched paraffinic ATJ (B1) fuel is shifted to slightly higher temperatures, indicating a longer total ignition delay time. Figure 3 summarizes some selected soot precursor intermediate species: benzene C6H6, indene C9H8, naphthalene C10H8, and anthracene C14H10. Note that naphthalene is also contained in the real fuel, therefore starting with a non-zero concentration. For these species a clear correlation with the fuel composition can be drawn. As can be expected, soot precursor species are more abundant in fuels with higher aromatic content (or low hydrogen content). Hydrogen content is considered to be a useful indicator for sooting propensity at technical combustors’ conditions such as jet engines (Schripp et al., 2018).
Describing the chemistry controlling the combustion of a real fuel in terms of its fundamental kinetic processes is a daunting task: typically, real fuels are mixtures of hundreds of components whose exact chemical structure is often unknown. Moreover, the full combustion of each fuel component is the result of tens, hundreds, if not thousands of elementary reactions whose rate needs to be determined. Finally, suitable solvers are needed to effectively compute the ODE (Ordinary Differential Equation) and DAE (Differential Algebraic Equation) systems describing the chemical evolution of the system. The following sections detail the approaches and the different steps used to obtain an accurate and predictive model able to represent the chemistry involved in the combustion of jet fuels of practical interest.
Detailed kinetic models attempt to identify all the important reaction pathways controlling the combustion of fuel components, and to assign to each elementary step temperature and pressure dependent reaction rates (i.e., the larger is the molecule, the higher is the number of reactions involved in its oxidation). Thermodynamic properties are used to define rate constants of backward reactions for reversible elementary steps. The two main challenges a kinetic model has to address are: i) the identification of the relevant reaction intermediates (whose number dictates the number of equations required to calculate the composition of the system), and ii) the determination of the thousands of reaction rate parameters it includes, together with thermodynamic properties of each species.
Because of the complexity of detailed kinetic models and of the computational burden associated with their use, reduced models including a smaller number of intermediates and global reactions are generally preferred for practical calculations and combustors’ design. The model proposed by the CRECK Modelling Lab of Politecnico di Milano attempts to couple the fundamentals of detailed chemical kinetic models and the practicality of reduced models, by limiting the number of species (and therefore of reactions) through isomer lumping (Ranzi et al., 2001).
By doing so, it is possible to simulate the fundamental processes controlling combustion and by-products formation of complex mixtures of large molecules with a relatively low number of species (few 100s), reducing considerably the computational cost of simulations and easing the interpretation of relevant chemical pathways. The CRECK model (which is meant to be general and applicable to a wide window of operating conditions) can be further reduced for computational fluid-dynamic applications based on the specific windows of compositions and thermodynamic conditions of interest (e.g., T, p, φ).
The other main challenge is the determination of reaction rates. While an extensive corpus of experimental and fundamental work exists on the reaction rates of small hydrocarbons (1-2 carbon atoms), most of the reactions involved in the oxidation of heavier molecules cannot be easily measured or calculated using quantum-chemical approaches. To overcome this issue, a systematic approach based on modularity, hierarchy and self-consistency is used in the construction of models for large fuel molecules.
The model is built hierarchically from light to heavy fuel species starting from a reaction mechanism core which describes the oxidation and pyrolysis of small gas-phase hydrocarbons. The current version of the CRECK model (Pejpichestakul et al., 2019) adopts the Aramco 2.0 (Metcalfe et al., 2013), (Burke et al., 2015) as its core. The core, which includes species up to three carbon atoms in size (C3), provides the basis for additional modules including larger molecules (Ranzi et al., 2012; Ranzi et al., 2014). At high temperature, the first step in the oxidation of large hydrocarbons is their decomposition to smaller fragments. Reactions involving C3 hydrocarbons or lower are subsets of the oxidation mechanisms of larger species which form them by fragmentation. By expanding the model towards heavier fuels, it is possible to describe the oxidation of larger molecules through the addition of blocks of reactions, which are built and validated starting from the core and moving up. Jet fuels include components with a number of carbons in the C7-C16 range and their kinetic models require the determination of a large number of parameters to quantify the reaction rates involved. From a micro-kinetic standpoint, the primary oxidation steps controlling combustion show strong similarities within a certain family of fuel components (paraffins, olefins, aromatics, naphthenes, etc.). For this reason, using analogy rules, it is possible to estimate reaction rates for molecules that have not been studied before. Adhering to principles of hierarchy, modularity, and self-consistency among reaction classes built on structural similarities, the CRECK team developed models for many components relevant to mid-distillates’ combustion. Among these, based on the compositional analysis provided by IFPEN, a set of components representative of the ones detected in the real fuels were selected (e.g., C12 n- and iso-alkanes, decalin, butylbenzene, butylcyclohexane, etc.).
The CRECK kinetic model covers both high (T > 1000 K) and low temperature (T = 500-1000K) reactions. High-temperature reactions are relevant to flame conditions and pollutant formation, while the low-temperature reactions (600–900 K) are required to predict the auto-ignition propensity of the fuel. At high pressure, low-temperature reactions become more important and they should be included in a comprehensive model to guarantee accurate predictions, particularly when transients are simulated.
Thermodynamic properties for all the species in the model have been adopted from the active thermochemical tables (Ruscic, 2015), from the online repository compiled by Burcat (Burcat and Ruscic, 2005), or determined based on group additivity methods. The final kinetic model includes about 460 species and 14,000 reactions, although, depending on the components and the operating window of interest, it is possible to reduce the size of the model for specific applications by removing non-relevant kinetic modules.
While the detailed composition of a full-blend fuel is rarely fully resolved, simpler analytical techniques allow to determine the breakdown into families of compounds, or their H/C ratio. Two dimensional gas chromatography (GCxGC) allows for a much more refined analysis, detecting both the family and the molecular weight distribution of the components.
Because of compositional complexity and lack of accurate information, it is not feasible to simulate the chemical behavior of a fuel reproducing its exact composition, especially when a detailed kinetic modelling approach is sought. In this case, modelers often adopt the surrogate approach: a simpler mixture (<10 components) matching a set of target properties of the real fuel is selected and used to represent the real fuel (Dooley et al., 2010; Kim et al., 2014; Prak et al., 2022). The selection of the surrogate can be performed “manually” by an expert user or, especially when many targets and fuel components are to be included, using an optimization tool.
In this work a hybrid approach has been selected, where the fuel palette used in the optimization is “manually” selected and, following the numerical optimization, minor variations are introduced to account for finer details relevant to the fuel composition (e.g., small amounts of a specific family of components that can play a role in the pollutant formation processes).
The first step in surrogate formulation is the definition of the palette of components to be included in the optimization. Since our goal is to generate a kinetic model for jet fuels, it is important to choose fuel components for which a reliable kinetic model exists (or can be easily built) and, ideally, has already been extensively validated. Figure 4 shows the set of components currently adopted for the fuel surrogate palette.
In the context of this project, POLIMI developed a fuel surrogate optimization tool. The optimization of the composition of a fuel surrogate is a multi-target multidimensional problem. The number of targets to be matched can be in the order of the 10s, while the dimensionality is equal to the number of components included in the palette minus one, with multiple solutions. Different optimization strategies have been proposed in literature for this type of problems (machine learning and genetic algorithms are among them) (Kim and Violi, 2022; Yu et al., 2022). The optimizer used in this work exploits the optimization package available in Matlab and is inspired by a previous literature work by Narayanaswamy et al. (Narayanaswamy and Pepiot, 2018). Different optimization algorithms are available within the tool developed at CRECK, including local optimization and a genetic algorithm.
The optimization targets available at this stage are DCN, H/C ratio, distribution within the different family of components, average molecular weight, threshold sooting index, density, distillation curve, and liquid viscosity. More targets will be added in future works to accommodate all the properties deemed important for the scopes of SAFs design, optimization and approval.
Based on this optimization process, surrogates were formulated for fuels A1 and C1. Fuel B1 is a synthetic fuel with a well-defined composition: the GCxGC data provided by IFPEN clearly identified iso-alkanes as the only components and, based on the process involved in its production and the compositional information available, it is possible to infer that these iso-alkanes are strongly branched oligomers of iso-C4 units. The GCxGC indicates that a C12 iso-paraffin is the main component and a surrogate based on iso-dodecane and iso-cetane was selected accordingly. Table 3 summarizes the composition of the three surrogates.
Figure 5 compares the distillation curves measured for the three jet fuels using the ASTM D86 standard method against the distillation curve calculated for the surrogates using a standard equilibrium approach for ideal mixtures (i.e., a linear combination of partial pressures calculated using the Antoine coefficients from (Yaws, 2005)). The distillation curve calculated for the surrogate of fuel A1 matches with good approximation the experimental one targeted in the surrogate optimization process. Similarly, the calculated distillation curve for the surrogate of fuel B1, which was formulated directly from the compositional information provided by IFPEN, agrees very well with the measurements. The distillation curve for fuel C1, as anticipated, shows greater deviations. Because of the lack of fuel components suitable to reproduce both the distillation curve and the H/C ratio, priority was given to the H/C ratio, a fundamental chemical property. Future works will add new components to the surrogate palette to overcome the current limitation. In particular, based on the GCxGC analysis, the need for higher molecular weight alkyl-cycloparaffins emerged. The surrogate for fuel C1, still, results to be the least volatile among the three fuels, reproducing, at least qualitatively, the relative behavior of the fuels.
FIGURE 5. Measured distillation curves of the three fuels considered in the model (symbols) and calculated equilibrium distillation curves of their surrogates.
The flow reactor data collected at DLR have been simulated using OpenSmoke++ (Cuoci et al., 2015). Calculations allowed to estimate the gas composition at the exit of the reactor for temperatures between 800 and 1150 K (nominal temperature) at two equivalence ratios (φ = 0.8 and 1.2). Not all the measured species can be directly compared with the experiments, as the surrogates mimic the composition of the real fuel by targeting the moieties it contains, and not the actual fuel component concentrations. A clear example comes from naphthalene, which can be both a secondary product formed during combustion and a fuel component. For this reason, in the following comparisons, we focus mostly on the smaller species that are formed during the decomposition and oxidation of the initial fuel and that may have an impact on the following soot and NOx formation processes. However, because of the relevance of polycyclic species to the formation of PAHs, naphthalene concentration profiles are shown for all the fuels.
Figures 6, 7 show the comparisons between calculations and experiments for Jet fuel A-1 (i.e., the standard jet fuel). The fuel model (constituted by the combination of the surrogate and the relative kinetic model) correctly reproduces the profiles of the major products, the consumption of the oxidizer (O2) and the formation of the final combustion products. H2O and CO2 are well captured, while small discrepancies are observed for H2 and CO. The peak in H2 concentration is measured at about 1020 K; the model accurately predicts its rate of formation, but has a slight delay in the onset of its consumption. The CO peak is reproduced correctly, although its shape is somewhat sharper. This could be partially related to the simplified approach adopted in the simulation of the flow reactor, which neglects the axial and radial inhomogeneity that may be present in the real device.
FIGURE 6. Comparison between measured (symbols) and calculated (lines) species profiles in the DLR flow reactor: Fuel A1, Φ = 0.8. Series are labeled using matching colors.
FIGURE 7. Comparison between measured (symbols) and calculated (lines) species profiles in the DLR flow reactor: Fuel A1, Φ = 1.2. Series are labeled using matching colors.
The agreement with minor species (ethylene, acetylene and other unsaturated species) is generally satisfactory. A systematic deviation is the over-prediction of C4H8 formation compensated by an under-prediction in C4H4 concentration. As mentioned, a direct comparison for C10H8 experimental and model profiles is not possible, as the real fuel already contains some naphthalene, not present in the surrogate (the representative species adopted to match the di-aromatics content in the surrogates is α-methyl-naphthalene). Despite the difference in the initial concentration, the model captures the timing of naphthalene formation and its consumption. The entity of naphthalene formation (i.e., the delta between the initial value and the peak value) is still well reproduced. The overall agreement at lean conditions is generally satisfactory.
At rich conditions (Figure 7) the model/experiment comparisons show similar features. However, it appears that the model presents more abrupt variations in the concentrations of some species, even though the peaks are generally captured correctly. The experimental and modeling results are, however, comparable to the ones obtained for other fuels previously tested on the same rig and simulations performed using other kinetic models. These deviations appear to be somewhat systematic for the stoichiometry condition here considered (φ = 1.2). More fuel-rich conditions seem to be captured by most models significantly better. Further analysis will focus on understanding if these discrepancies are the results of deviations from the plug flow reactor hypothesis used in the simulations or actual deficiencies in the model.
Figures 8, 9 show the comparisons between calculations and experiments for Jet fuel B1 (ATJ). When compared with the other two fuels, fuel B1 shows an earlier onset of the reactivity. This shows in the form of an early initial drop in the oxygen concentration associated with the formation of formaldehyde. It should be noted that B1 fuel was simulated using a two component surrogate formulated from compositional information only, and the chemical composition of the fuel used in the experiment matches almost perfectly the composition of the surrogate. Fuel simplicity eases the surrogate formulation step, leaving all the weight of the discrepancies on the kinetic mechanism. As the B1 mixture is composed largely of iso-dodecane, the simulation results are strongly dependent on how accurate its model is. The CRECK mechanism for iso-dodecane has been developed applying the analogy rules introduced in Section 2.2.1, using the mechanism of iso-octane (a widely studied fuel sharing strong structural similarities) as reference for the reaction rates. Unfortunately, experimental data available for the validation of the iso-dodecane kinetic model are still somewhat limited, and no speciation data were available at the time of the model formulation. Future works will focus on the refinement of the iso-dodecane model, also including data from Gutzman et al. (Guzman et al., 2019).
FIGURE 8. Comparison between measured (symbols) and calculated (lines) species profiles in the DLR flow reactor: Fuel B1, Φ = 0.8. Series are labeled using matching colors.
FIGURE 9. Comparison between measured (symbols) and calculated (lines) species profiles in the DLR flow reactor: Fuel B1, Φ = 1.2. Series are labeled using matching colors.
Despite the limited validation, the agreement with the flow reactor data obtained for this fuel is comparable with what seen for the A1 fuel, confirming, at least in this instance, that reasonably accurate models can be built starting from the fundamentals even in the absence of extensive validation data. Notably, both the experiments and the simulations show that this fuel produces a very limited amount of soot precursors (e.g., C6H6, C10H8) even at slightly rich conditions.
Figures 10, 11 show the comparisons between calculations and experiments for the C1 fuel (the high aromatic content fuel). The agreement with the experimental data is satisfactory also in this case, even though the same issues that plague fuel A1 at rich conditions can be observed. The presence of naphthalene in the fuel (not in the surrogate) is particularly evident here, but the C10H8 peak ends up being relatively close to the experimental one and the consumption temperature is well captured.
FIGURE 10. Comparison between measured (symbols) and calculated (lines) species profiles in the DLR flow reactor: Fuel C1, Φ = 0.8. Series are labeled using matching colors.
FIGURE 11. Comparison between measured (symbols) and calculated (lines) species profiles in the DLR flow reactor: Fuel C1, Φ = 1.2. Series are labeled using matching colors.
The last set of comparisons shown in Figure 12 focuses on the relative behavior of the three fuels at lean conditions. The model captures very well the differences in reactivity highlighted by the drop in the O2 concentration and the sharp rise in water. Fuel B1 appears to be the most refractory to high temperature oxidation at the conditions of the flow reactor. The high aromatic content fuel is the second least reactive and, compared with the other fuels, produces a lower amount of water, compatibly with his lower H/C ratio. In terms of oxidation products, fuel B1 oxidation starts at lower temperature with an early formation of CH2O. The shape and magnitude of the CH4 and CH2O peaks are captured correctly by the model, which also hints at their earlier formation.
FIGURE 12. Relative selectivity of the three fuels towards their oxidation intermediates at Φ = 0.8, experiments (A) and calculations (B).
Finally, the last row of Figure 12 highlights the relative formation of C6H6 and C10H8 measured by the experiments and predicted by the model. Beside the initial amount of naphthalene present in the fuel, the model does a good job at reproducing the relative concentrations. Fuel B1 produces only very limited amounts of benzene and naphthalene, while the high aromatic fuel, as expected, is the most prone to the formation of aromatic rings. These results could be correlated to the sooting tendencies of the three fuels as the formation of mono- and, later, di-aromatics is the first step along the growth of larger PAHs and soot. The ability of the model to capture the differences in reactivity and the relative selectivity to products reinforces the idea that, in future analyses, a modeling grounded approach based on composition-based surrogates and detailed kinetics can be used to predict the combustion behavior of novel fuels, assisting the certification process of fuel candidates.
This paper describes the procedures used to characterize the reactivity of three representative jet fuels from the fuel palette selected by the JETSCREEN program. A standard Jet fuel, an ATJ fuel and a high aromatic content fuel were investigated experimentally and numerically in a flow reactor at temperatures in the 800-1150K range and two fuel air ratios (Φ = 0.8 and 1.2) at atmospheric pressure. Semi-detailed models reproducing the oxidation mechanism of the fuels were developed by coupling the CRECK chemical kinetic model and a customized numerical tool for the formulation of fuel surrogates. Comparisons of the experimental data collected by DLR were used to validate the model and support the analysis of the combustion behavior.
From the numerical and experimental results it is possible to conclude that the general modeling framework can capture major combustion characteristics of the real fuels and reproduce with good accuracy the selectivity towards different intermediates during the oxidation of the real fuels. It is evident that the fuel with the highest aromatic content has a significantly greater tendency to form soot precursors, while the ATJ fuel (B1) has the least one. The high temperature reactivity of the ATJ is also reduced compared to a traditional Jet A-1 (A1). The intermediate species peaks for the ATJ extend to higher temperatures compared to the ones of the two fossil-based fuels (A1 and C1). Moreover, fuel B1 (ATJ) also presents an earlier onset of the oxygen (and fuel) consumption, associated with the formation of formaldehyde.
From a modelling perspective, it emerged that more validation is needed for some of the compounds used in the surrogate palette, particularly for the aromatic and naphthenic fractions. Recently published data may also offer the opportunity for improving the current iso-dodecane model. Because of the entanglements introduced when considering complex mixtures, these experiments are not generally suitable for the validation of specific submodels, although it is fair to conclude that some systematic discrepancies may be related to deficiencies in the kinetic model. The simulations consistently underestimate the formation of C4H8 in favor of more dehydrogenated species (C4H4), pointing to inaccuracies in the oxidation and pyrolysis of small species. This mechanism may influence soot growth phenomena, since strongly unsaturated linear species are prone to condensation reactions leading to PAHs.
Moreover, for fuels with similarities to the high aromatic fuel C1, more components (high molecular weight ones, in particular) may be needed to capture both the chemical and physical properties of the target fuel (e.g., its distillation curve). While n-alkanes have been extensively studied in well-characterized reacting systems (e.g., flow reactor, jet-stirred reactors, shock-tubes), data for high molecular weight alkyl-aromatics and alkyl-cycloalkanes are more scarce, as their low volatility, combined with their slower reactivity, makes their probing more challenging. In the absence of data, models can only be constructed based on similarity rules, as described in the kinetic model section, leading to higher uncertainties. Therefore, further research will have to locate the optimum between uncertainties in the surrogate formulations and in the kinetic model of the components it contains.
The raw data supporting the conclusions of this article will be made available by the authors, without undue reservation.
Experimental work by PO, Modelling activity by MM and MP.
This work was performed in the context of JetSCREEN, a project funded from the European Union’s Horizon 2020 research and innovation program under agreement No. 723525.
The authors declare that the research was conducted in the absence of any commercial or financial relationships that could be construed as a potential conflict of interest.
All claims expressed in this article are solely those of the authors and do not necessarily represent those of their affiliated organizations, or those of the publisher, the editors and the reviewers. Any product that may be evaluated in this article, or claim that may be made by its manufacturer, is not guaranteed or endorsed by the publisher.
Aviationbenefits (2021). Waypoint 2050. Available at: https://aviationbenefits.org/environmental-efficiency/climate-action/waypoint-2050/ (Accessed Jan 14, 2022).
Bierkandt, T., Oßwald, P., Schripp, T., and Köhler, M. (2019). Experimental Investigation of Soot Oxidation under Well-Controlled Conditions in a High-Temperature Flow Reactor. Combustion Sci. Tech. 191, 1499–1519. doi:10.1080/00102202.2018.1554651
Burcat, A., and Ruscic, B. (2005). Third Millenium Ideal Gas and Condensed Phase Thermochemical Database for Combustion (With Update from Active Thermochemical Tables). Lemont: Argonne National Laboratory. doi:10.2172/925269
Burke, S. M., Burke, U., Mc Donagh, R., Mathieu, O., Osorio, I., Keesee, C., et al. (2015). An Experimental and Modeling Study of Propene Oxidation. Part 2: Ignition Delay Time and Flame Speed Measurements. Combustion and Flame 162 (2), 296–314. doi:10.1016/J.COMBUSTFLAME.2014.07.032
Chu, T.-C., Buras, Z. J., Oßwald, P., Liu, M., Goldman, M. J., and Green, W. H. (2019). Modeling of Aromatics Formation in Fuel-Rich Methane Oxy-Combustion with an Automatically Generated Pressure-dependent Mechanism. Phys. Chem. Chem. Phys. 21 (2), 813–832. doi:10.1039/C8CP06097E
Cuoci, A., Frassoldati, A., Faravelli, T., and Ranzi, E. (2015). OpenSMOKE++: An Object-Oriented Framework for the Numerical Modeling of Reactive Systems with Detailed Kinetic Mechanisms. Comp. Phys. Commun. 192, 237–264. doi:10.1016/J.CPC.2015.02.014
Dooley, S., Won, S. H., Chaos, M., Heyne, J., Ju, Y., Dryer, F. L., et al. (2010). A Jet Fuel Surrogate Formulated by Real Fuel Properties. Combustion and Flame 157 (12), 2333–2339. doi:10.1016/j.combustflame.2010.07.001
European Commission (2022). JET Fuel SCREENing and Optimization | JETSCREEN Project | Fact Sheet | H2020 | CORDIS | European Commission. Available at: https://cordis.europa.eu/project/id/723525 (Accessed Jan 14, 2022).
Guzman, J., Kukkadapu, G., Brezinsky, K., and Westbrook, C. (2019). Experimental and Modeling Study of the Pyrolysis and Oxidation of an Iso-Paraffinic Alcohol-To-Jet Fuel. Combustion and Flame 201, 57–64. doi:10.1016/j.combustflame.2018.12.013
Herrmann, F., Oßwald, P., and Kohse-Höinghaus, K. (2013). Mass Spectrometric Investigation of the Low-Temperature Dimethyl Ether Oxidation in an Atmospheric Pressure Laminar Flow Reactor. Proc. Combustion Inst. 34 (1), 771–778. doi:10.1016/J.PROCI.2012.06.136
Kathrotia, T., Naumann, C., Oßwald, P., Köhler, M., and Riedel, U. (2017). Kinetics of Ethylene Glycol: The First Validated Reaction Scheme and First Measurements of Ignition Delay Times and Speciation Data. Combustion and Flame 179, 172–184. doi:10.1016/J.COMBUSTFLAME.2017.01.018
Kim, D., Martz, J., and Violi, A. (2014). A Surrogate for Emulating the Physical and Chemical Properties of Conventional Jet Fuel. Combustion and Flame 161 (6), 1489–1498. doi:10.1016/j.combustflame.2013.12.015
Kim, D., and Violi, A. (2022). Uncertainty-based Weight Determination for Surrogate Optimization. Combustion and Flame 237, 111850. doi:10.1016/j.combustflame.2021.111850
Köhler, M., Oßwald, P., Krueger, D., and Whitside, R. (2018). Combustion Chemistry of Fuels: Quantitative Speciation Data Obtained from an Atmospheric High-Temperature Flow Reactor with Coupled Molecular-Beam Mass Spectrometer. JoVE 132, 56965. doi:10.3791/56965
Liu, Y.-X., Richter, S., Naumann, C., Braun-Unkhoff, M., and Tian, Z.-Y. (2019). Combustion Study of a Surrogate Jet Fuel. Combustion and Flame 202, 252–261. doi:10.1016/j.combustflame.2019.01.022
Prak, D. J. l., Simms, G. R., Dickerson, T., McDaniel, A., and Cowart, J. S. (2022). Formulation of 7-Component Surrogate Mixtures for Military Jet Fuel and Testing in Diesel Engine. ACS Omega 7, 2275–2285. doi:10.1021/acsomega.1c05904
Martinos, A.-D., Zarzalis, N., and Harth, S.-R. (2021). “Analysis of Ignition Processes at Combustors for Aero Engines at High Altitude Conditions with and without Effusion Cooling,” in Proceedings of ASME Turbo Expo 2020 Turbomachinery Technical Conference and Exposition GT2020, September 21-25, 2020. doi:10.1115/GT2020-16173
Metcalfe, W. K., Burke, S. M., Ahmed, S. S., and Curran, H. J. (2013). A Hierarchical and Comparative Kinetic Modeling Study of C1 − C2 Hydrocarbon and Oxygenated Fuels. Int. J. Chem. Kinet. 45 (10), 638–675. doi:10.1002/kin.20802
Narayanaswamy, K., and Pepiot, P. (2018). Simulation-driven Formulation of Transportation Fuel Surrogates. Combustion Theor. Model. 22 (5), 883–897. doi:10.1080/13647830.2018.1464210
Oßwald, P., and Köhler, M. (2015). An Atmospheric Pressure High-Temperature Laminar Flow Reactor for Investigation of Combustion and Related Gas Phase Reaction Systems. Rev. Scientific Instr. 86 (10), 105109. doi:10.1063/1.4932608
Oßwald, P., Zinsmeister, J., Kathrotia, T., Alves-Fortunato, M., Burger, V., van der Westhuizen, R., et al. (2021). Combustion Kinetics of Alternative Jet Fuels, Part-I: Experimental Flow Reactor Study. Fuel 302, 120735. doi:10.1016/j.fuel.2021.120735
Pejpichestakul, W., Ranzi, E., Pelucchi, M., Frassoldati, A., Cuoci, A., Parente, A., et al. (2019). Examination of a Soot Model in Premixed Laminar Flames at Fuel-Rich Conditions. Proc. Combustion Inst. 37 (1), 1013–1021. doi:10.1016/J.PROCI.2018.06.104
Pelucchi, M., Oßwald, P., Pejpichestakul, W., Frassoldati, A., and Mehl, M. (2021). On the Combustion and Sooting Behavior of Standard and Hydro-Treated Jet Fuels: An Experimental and Modeling Study on the Compositional Effects. Proc. Combustion Inst. 38 (1), 523–532. doi:10.1016/j.proci.2020.06.353
Ranzi, E., Dente, M., Goldaniga, A., Bozzano, G., and Faravelli, T. (2001). Lumping Procedures in Detailed Kinetic Modeling of Gasification, Pyrolysis, Partial Oxidation and Combustion of Hydrocarbon Mixtures. Prog. Energ. Combustion Sci. 27 (1), 99–139. doi:10.1016/S0360-1285(00)00013-7
Ranzi, E., Frassoldati, A., Grana, R., Cuoci, A., Faravelli, T., Kelley, A. P., et al. (2012). Hierarchical and Comparative Kinetic Modeling of Laminar Flame Speeds of Hydrocarbon and Oxygenated Fuels. Prog. Energ. Combustion Sci. 38 (4), 468–501. doi:10.1016/J.PECS.2012.03.004
Ranzi, E., Frassoldati, A., Stagni, A., Pelucchi, M., Cuoci, A., and Faravelli, T. (2014). Reduced Kinetic Schemes of Complex Reaction Systems: Fossil and Biomass-Derived Transportation Fuels. Int. J. Chem. Kinet. 46 (9), 512–542. doi:10.1002/kin.20867
Rock, N., Stouffer, S., Hendershott, T., Heyne, J., Blunck, D., Zheng, L., et al. (2021). “Lean Blowout Studies,” in Fuel Effects on Operability of Aircraft Gas Turbine Combustors (Reston: American Institute of Aeronautics and Astronautics, Inc.), 143–196. doi:10.2514/5.9781624106040.0143.0196
Ruscic, B. (2015). Active Thermochemical Tables: Sequential Bond Dissociation Enthalpies of Methane, Ethane, and Methanol and the Related Thermochemistry. J. Phys. Chem. A. 119 (28), 7810–7837. doi:10.1021/acs.jpca.5b01346
Saffaripour, M., Veshkini, A., Kholghy, M., and Thomson, M. J. (2014). Experimental Investigation and Detailed Modeling of Soot Aggregate Formation and Size Distribution in Laminar Coflow Diffusion Flames of Jet A-1, a Synthetic Kerosene, and N-Decane. Combustion and Flame 161 (3), 848–863. doi:10.1016/j.combustflame.2013.10.016
Schenk, M., Leon, L., Moshammer, K., Oßwald, P., Zeuch, T., Seidel, L., et al. (2013). Detailed Mass Spectrometric and Modeling Study of Isomeric Butene Flames. Combustion and Flame 160 (3), 487–503. doi:10.1016/J.COMBUSTFLAME.2012.10.023
Schripp, T., Anderson, B., Crosbie, E. C., Moore, R. H., Herrmann, F., Oßwald, P., et al. (2018). Impact of Alternative Jet Fuels on Engine Exhaust Composition during the 2015 ECLIF Ground-Based Measurements Campaign. Environ. Sci. Technol. 52 (8), 4969–4978. doi:10.1021/acs.est.7b06244
Yaws, C. L. (2005). The Yaws Handbook of Vapor Pressure : Antoine Coefficients. Amsterdam: Elsevier.
Yi, T., Gutmark, E. J., and Walker, B. K. (2009). Stability and Control of Lean Blowout in Chemical Kinetics-Controlled Combustion Systems. Combustion Sci. Tech. 181 (2), 226–244. doi:10.1080/00102200802424559
Keywords: chemical kinetics, flow reactor, renewable fuels, combustion modeling, surrogates
Citation: Mehl M, Pelucchi M and Osswald P (2022) Understanding the Compositional Effects of SAFs on Combustion Intermediates. Front. Energy Res. 10:830236. doi: 10.3389/fenrg.2022.830236
Received: 06 December 2021; Accepted: 01 February 2022;
Published: 07 March 2022.
Edited by:
Michael P. Wolcott, Washington State University, United StatesReviewed by:
Joshua Heyne, University of Dayton, United StatesCopyright © 2022 Mehl, Pelucchi and Osswald. This is an open-access article distributed under the terms of the Creative Commons Attribution License (CC BY). The use, distribution or reproduction in other forums is permitted, provided the original author(s) and the copyright owner(s) are credited and that the original publication in this journal is cited, in accordance with accepted academic practice. No use, distribution or reproduction is permitted which does not comply with these terms.
*Correspondence: M. Mehl, bWFyY28ubWVobEBwb2xpbWkuaXQ=
Disclaimer: All claims expressed in this article are solely those of the authors and do not necessarily represent those of their affiliated organizations, or those of the publisher, the editors and the reviewers. Any product that may be evaluated in this article or claim that may be made by its manufacturer is not guaranteed or endorsed by the publisher.
Research integrity at Frontiers
Learn more about the work of our research integrity team to safeguard the quality of each article we publish.