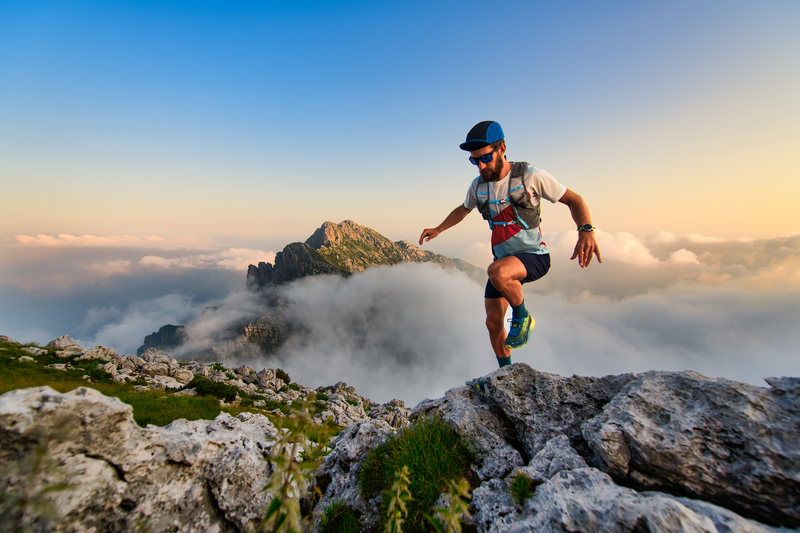
95% of researchers rate our articles as excellent or good
Learn more about the work of our research integrity team to safeguard the quality of each article we publish.
Find out more
EDITORIAL article
Front. Energy Res. , 06 June 2022
Sec. Process and Energy Systems Engineering
Volume 10 - 2022 | https://doi.org/10.3389/fenrg.2022.818906
This article is part of the Research Topic Forecasting Techniques for Energy Systems with Data Analytics and Machine Learning View all 4 articles
Editorial on the Research Topic
Editorial to the Special Issue“Forecasting Techniques for Energy Systems with Data Analytics and Machine Learning”
With the economic development and increasing load demand, global warming, the energy crisis, and renewable energy resources have become growing issues. Under this background, energy security and the integration of renewable energy resources has become a hot spot of concern in the international community. The stochastic nature of the resources and usage requires more detailed energy management in which forecasting techniques will play an important role. The use of data analytics technologies to forecast energy resources and usage is considered to be an effective means to handle this issue. Furthermore, the emergence of smart sensors allows energy companies to collect large-scale energy data, while how to utilize these data to solve practical problems is a matter worth discussing.
For forecasting problems of energy systems, such as wind power, load, market-based electricity price, photovoltaic power, and solar irradiation, the output variables are always random in nature. Therefore, forecasting techniques based on data analytics and machine learning can be implemented as effective tools to reduce the influence from the randomness, thus to achieve accurate forecasting results.
Therefore, the author of this editorial is very grateful to the staff and reviewers for their efforts in editing and selecting this collection Fang et al.; Yang et al.; Zheng and Zhu. In one paper Yang et al., the authors propose an improved generalized model based on the combination of wavelet packet (WP) and least squares support vector machine (LSSVM) to obtain higher accuracy point prediction results. Then, the error mixed distribution function is used to fit the probability distribution of the prediction error, and the probability prediction is performed to obtain the prediction interval of short-term photovoltaic power. In another paper Zheng and Zhu, addressing the problem of a wheeled mobile robot to track a reference trajectory within a finite task horizon, the authors propose a stochastic predictive control (MPC) model, in which the cost function is designed to penalize its tracking error and energy consumption. Then, based on quantile techniques, a learning-based approach is applied to transform the probability constraints to deterministic constraints, and to calculate the terminal constraint to guarantee recursive feasibility. In the final paper Fang et al. aiming at the state estimation error caused by inaccurate battery model parameter estimation, the authors propose a model-based state of charge (SOC) estimation method using a lithium-ion battery, with parameter identification using an adaptive genetic algorithm (AGA) and state estimation using a fractional-order unscented Kalman filter (FOUKF).
WF: Writing and editing.
The authors declare that the research was conducted in the absence of any commercial or financial relationships that could be construed as a potential conflict of interest.
All claims expressed in this article are solely those of the authors and do not necessarily represent those of their affiliated organizations, or those of the publisher, the editors and the reviewers. Any product that may be evaluated in this article, or claim that may be made by its manufacturer, is not guaranteed or endorsed by the publisher.
Keywords: energy system, data analytics, machine learning, forecasting techniques, randomness
Citation: Fu W (2022) Editorial: Forecasting Techniques for Energy Systems With Data Analytics and Machine Learning. Front. Energy Res. 10:818906. doi: 10.3389/fenrg.2022.818906
Received: 20 November 2021; Accepted: 19 April 2022;
Published: 06 June 2022.
Edited and reviewed by:
Francois M. A. Marechal, Swiss Federal Institute of Technology Lausanne, SwitzerlandCopyright © 2022 Fu. This is an open-access article distributed under the terms of the Creative Commons Attribution License (CC BY). The use, distribution or reproduction in other forums is permitted, provided the original author(s) and the copyright owner(s) are credited and that the original publication in this journal is cited, in accordance with accepted academic practice. No use, distribution or reproduction is permitted which does not comply with these terms.
*Correspondence: Wenlong Fu, Y3RndV9mdXdlbmxvbmdAMTI2LmNvbQ==
Disclaimer: All claims expressed in this article are solely those of the authors and do not necessarily represent those of their affiliated organizations, or those of the publisher, the editors and the reviewers. Any product that may be evaluated in this article or claim that may be made by its manufacturer is not guaranteed or endorsed by the publisher.
Research integrity at Frontiers
Learn more about the work of our research integrity team to safeguard the quality of each article we publish.