- 1Botswana International University of Science and Technology (BIUST), Palapye, Botzwana
- 2Deggendorf Institute of Technology, Deggendorf, Germany
Solar Photo Voltaic (PV) powered community microgrids are a promising sustainable solution for neighborhoods, residential quarters, and cities in sub-Saharan Africa (SSA) to meet their energy demands locally and to increase energy independence and resilience. This review provides a comprehensive study on the nature of solar PV community microgrids. Through their capacity to operate in both grid-connected and island modes, community microgrids improve utility system resiliency while also boosting energy security in local states and towns. The integration of solar PV microgrids with the electricity utility grid requires control strategies to facilitate the load sharing between distributed generation units, voltage and frequency control, as well as emergency islanding. Control strategies such as hierarchical control and droop are discussed in the review article. To identify the effectiveness of control strategies through system simulation, a review of various modeling designs of individual components in a solar PV microgrid system is discussed. The article goes on to talk about energy optimization approaches and their economic impact on microgrid systems. Finally, the review concludes with an overview of the technical challenges encountered in the integration of solar PV systems in microgrids.
1 Introduction
Renewable Energy Sources (RES) such as solar, wind, and bioenergy through technology advancement have become affordable and environmentally sustainable energy solutions to generate electricity for developing countries (Yekini et al., 2013). The establishment of electricity infrastructures is costly, thereby small to medium-scale decentral energy solutions like mini-grids and microgrids can be favorable for developing countries (Hirsch, Parag and Guerrero, 2018). Microgrids provide a platform for combining Renewable Energy Technologies (RETs) into a more secure and sustainable electricity supply network (Phurailatpam, Rajpurohit and Pindoriya, 2011). Hence, renewable microgrids present benefits such as enhancing local energy security and promoting environmental sustainability by introducing energy generation sources with low carbon emissions (Wang, Zhu and Yan, 2018). Microgrids classify as small to medium-scale solutions consisting of Distributed Generation (DG), electrical loads, control, and energy management devices (Ustun, Ozansoy and Zayegh, 2011). Community solar microgrids are described as ten or more residential households alongside the local businesses interconnected with each other to attain the affordability and resilience traits of the microgrid system (Qazi, 2017). Community microgrids in cities have been reported in the literature to make electricity more affordable to individual customers through the shared usage of RES (Palaniappan et al., 2017). A study by (Wang, Zhu and Yan, 2018) indicates that matching solar generation to electrical load demand in a microgrid system has significant economic benefits. Solar PV microgrids are gaining a lot of traction in the residential and commercial sectors as the modern lighting equipment and electrical appliances such as Light Emitting Diode (LED) lights and dryers will shift to DC power type (Cuzner, Palaniappan and John Shen, 2015). Solar PV systems provide significant benefits such as easy installation, lower carbon emissions, and high efficiency in comparison with other RES (Basak et al., 2012).
The global solar PV capacity increased from 290 Giga Watts (GW) to 580 GW between 2016 and 2019 (IRENA, 2019). This global rise of solar PV systems installation is due to factors like technological progress and declining costs of PV modules (Castrejon-Campos, Aye and Hui, 2022). Solar power plants have cheaper maintenance and repair costs than any other energy-related systems that use RES (Gürtürk, 2019). Between 2010 and 2019, the Levelized Cost of Electricity (LCOE) for PV systems decreased from USD 0.378 per kWh to USD 0.068 per kWh (IRENA, 2019). Projections from a study conducted by (Cole et al., 2017) suggest that LCOE for residential systems will further go down to USD 0.05 per kWh by the year 2030. PV module projections reveal a cost decrease from USD 0.61 per Wp to USD 0.16 per Wp between the years 2015 and 2050 in the best-case scenario (Mayer et al., 2015). As solar energy is an intermittent generation type, stand-alone microgrid systems are equipped with an Energy Storage System (ESS) to provide continuous power flow. Depending on the microgrid system’s energy requirements, an ESS in the form of batteries are used to charge and discharge the microgrid DC bus system. The interaction between the components of microgrids and power flow is achieved through a control and Energy Management System (EMS) (Yang et al., 2019). Multiple control strategies have been developed in literature and the most documented effective way to manage power flow in the microgrid is the hierarchical control scheme (Planas et al., 2013; Meng et al., 2016). As outlined in a study conducted by (Tayab et al., 2017) for parallel-connected inverters in a microgrid, the droop control technique is appropriate. Optimized EMS through machine learning techniques are also explored in the review. The structure of the paper is as follows; Section 2 of the review provides an microgrid classification and control. Section 3 then presents the modeling and simulation overview of the various components of solar PV microgrids. Section 4 presents the optimization of microgrid system through EMS. Section 5 discusses the challenges of integrating solar PV systems into microgrid systems, then finally Section 6 presents the conclusions of the review.
2 Microgrid classification and control
A microgrid can be defined as “a group of interconnected loads and Distributed Energy Resources (DER) that act as a single controllable entity with respect to the grid” (USDOE, 2012). A microgrid has three main sections namely, generation, loads, and controls, and these three work within a specialized controlled network together with the grid or in an islanded state (Donahue, 2019). DG units from RES such as solar energy, wind and hydropower characterize renewable microgrids. A transformer features the Point of Common Coupling (PCC), which serves the function of linking the microgrid to the main grid (Perera, Ciufo and Perera, 2013).
2.1 Microgrid topology
Based on the operational frequency of power generation, three types of microgrid topology can be distinguished (Hossain et al., 2019), namely; Direct Current (DC) microgrids, Alternating Current (AC) microgrids, and hybrid AC/DC microgrids.
2.1.1 Direct current microgrid system
A DC microgrid system comprises a Low Voltage AC transmission network (LVAC) to Medium Voltage AC (MVAC) transmission network. DG units with DC power output interact with the other components of the microgrid through a DC transmission bus line (Fusheng, Ruisheng and Fengquan, 2016a). LVDC microgrids are more prevalent for where the bulk of the loads are responsive electronic devices (Salomonsson, Söder and Sannino, 2009). Medium Voltage DC (MVDC) microgrid systems are widely utilized for heavy industrial loads that service activities such as offshore oil and gas drilling (Reed et al., 2012; Kounev et al., 2014). A solar PV system requires a DC-DC converter to regulate DC voltage output. Some electrical appliances are powered by an AC power supply thereby for a system in Figure 1, a DC/AC converter is required (Justo et al., 2013). Excess power in the DC bus line will be stored in the ESS. Figure 2 illustrates a typical structure of a microgrid system.
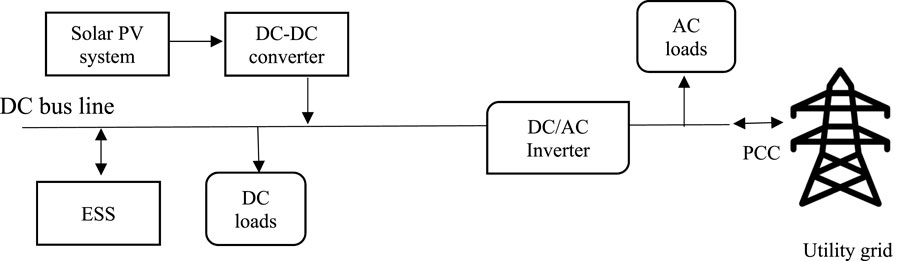
FIGURE 1. Structure of a DC microgrid with DC and AC loads (Justo et al., 2013).
DC microgrid systems are preferred over AC microgrid systems because they are more effective due to the lack of converter requirements. Energy losses occur during each conversion phase thus more energy losses occur in the AC microgrid system compared to the DC microgrid (Shuai et al., 2018; Hossain et al., 2019). Other advantages of DC microgrids include fewer synchronization issues for grid connection and the absence of the need for reactive power compensation.
2.1.2 Alternating current microgrid system
An AC microgrid system comprises a LVAC transmission network to MVAC transmission network. DG units that generate AC power output connect directly to the transmission line while DG units that generate DC power are connected to the transmission line through the DC/AC converter (Justo et al., 2013; Hossain et al., 2019). Conventional electrical infrastructure comprises AC power thereby making the integration of AC microgrid configuration and the utility grid effortless (Hasan and Arif, 2018). DG units for this version of microgrid, include hydropower, biogas, Wind Energy Conversion System (WECS), tidal, and wave turbines (Baran and Mahajan, 2003). The AC/DC inverter serves the role of converting AC power to DC power type to supply DC loads and ESS (Lotfi and Khodaei, 2017). The AC/DC inverter usage possesses various challenges such as infringing protection, communication, and operation of the microgrid (Phurailatpam, Rajpurohit and Pindoriya, 2011). Figure 3 depicts the structure of an AC microgrid system.
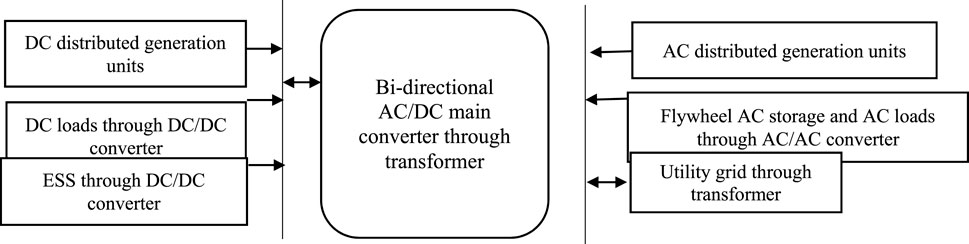
FIGURE 3. Layout of hybrid AC/DC microgrid system (Jain and Raju, 2013).
2.1.3 Hybrid alternating current/direct current microgrid systems
A hybrid AC/DC microgrid system configuration comprises multiple AC and DC sources as well as multiple loads connected to the respective AC and DC networks (Liu, Wang and Loh, 2011). AC loads and sources communicate via an AC transmission bus line while the respective DC loads and sources interact via a DC transmission bus line. A key component in this topology is the bidirectional inverter, which facilitates power flow and maintains stable voltage between both buses (Jain and Raju, 2013). Components such as the ESS and DC loads communicate via the DC bus of the system to ease the power supply. The hybrid system can achieve minimal conversion losses, as individual components in the microgrid are directly wired to their respective power source (Hasan and Arif, 2018). The DC loads are connected to the DC grid and the AC loads are connected to the AC grid hence the lack of need of converters. Owing to the lack of need for phase and frequency modules, the DC-coupled hybrid microgrid system is more economical compared to the AC-coupled hybrid microgrid system (Kabalci, Irgan and Kabalci, 2018).
Depending on the direction of power flow in the microgrid system, the bidirectional/Interlinking Converter (IC) performs the duties of either a rectifier or an inverter (Gupta, Doolla and Chatterjee, 2018; Kabalci, Irgan and Kabalci, 2018; Raviteja, Yadagiri and Jithendar, 2021). The IC connects to the DC and AC grid. Due to energy requirements of the loads, there is bidirectional power flow between the sub-grids via the IC. The IC’s control features include reducing harmonics, controlling power imbalances, and coordinating storage. Hybrid AC/DC microgrids residential applications are 2.06% more efficient than DC microgrids, according to a study by (Hamza et al., 2019).
2.2 Features of community solar microgrids
Beyond its classification by topology, microgrids can be classified by location as urban or remote. Community solar microgrids can exist in both urban and remote areas. Communities in remote villages can utilize RES such as solar and wind to provide electricity. Microgrid systems in remote areas are not connected to the grid and therefore mainly depend on power supply from DG units supplemented by energy storage (Phurailatpam, Rajpurohit and Pindoriya, 2011). Community solar microgrids establishment in urban areas has more benefits as compared to in remote areas due to multiple benefits arising from the on-grid operation state (Faure et al., 2017). Grid-connected microgrids pose various benefits such as improving power quality, reducing transmission power losses thereby improving system economics. Smart peak shaving in an urban community network through Demand Side Management (DSM) enables customers to make significant cost savings in their annual electricity purchases from the electric utility grid (Faure et al., 2017). Urban microgrid systems provide the platform for smart grid technologies such as monitoring and control that are core to enhancing the resiliency of the electrical power grid (Howell et al., 2017). In a study of a hybrid microgrid system (Bifaretti et al., 2017), utilized DG units such as fuel cell and grid support to offset the high energy demand during low insolation hours in a residential community. During times of high solar insolation, the solar PV system served as the main source of power for the loads in the microgrid system, and any extra power was fed into the grid (Da Marcello et al., 2017). conducted a study in which a battery was used to meet the energy demands of electrical loads when the microgrid system operates in island mode during a power outage. As soon as the grid is restored, the battery of the microgrid system is charged. Solar PV microgrids in urban settlements play a vital role in minimizing GHGs as they produce an infinitesimal amount of emissions (Ashford and Pagkalinawan, 2019). As per a study done by the Italian Agency for New Technologies, Energy and Sustainable Economic Development (ENEA) done in the year 2017, globally, there are over 70 operational microgrid projects implemented in urban settlements (Faure et al., 2017). Smart power-sharing amongst electrical loads in an urban microgrid leads to the effective usage of the power generated by the DG units thereby resulting in cost savings. According to a study on the energy scheduling of commercial loads in microgrids by (Ansari, Zeinoddini-Meymand and Niknam, 2019), the hybrid microgrid structure has lower network power costs than separate DC and AC microgrid structures. Load management plays the critical role of prioritizing load power-sharing between the priority critical and low priority loads. Notable traits of the community solar microgrids in urban settlements include power-sharing and self-sufficiency.
2.2.1 Power-sharing
Power-sharing in a microgrid system allows for individual customers in a community solar microgrid to utilize power from the solar PV system thus enabling them to save money from their monthly electricity bill purchases (Palaniappan et al., 2017). Due to its capacity to regulate power through a bidirectional converter, hybrid microgrid systems have seen an increase in popularity due to the high introduction of DC compatible loads. Customers in a community solar microgrid may include local businesses, schools, hospitals, and residential households. In the city of Boston, Massachusetts USA, there are several solar community microgrids divided by district zones the city. The community solar microgrids have been set up in locations with critical loads found in hospitals, emergency housing, and supermarkets thereby improving the utility grid network. The community solar microgrid establishment resulted in cost savings between $692 and $180,000 annually for the customers in the city of Boston (Morgan et al., 2016). A campus community solar microgrid study done by (Gašparović et al., 2016) investigated two microgrid community cases in the University of Split (Croatia) to identify optimum scenarios for the economic share of electrical loads in the settlement. The results from the study revealed that electricity purchases from the national electricity grid were offset by 17% through the campus microgrid system.
2.2.2 Self-sufficiency
Another trait of urban microgrids is the capability to disconnect from the electricity grid and operate in the island mode. Disconnection from the grid-connection state to island mode can be a result of a power outage or when the DG unit is capable to provide solely continuous power to the electrical loads without support from the utility grid. According to (Wanitschke, Pieniak and Schaller, 2017), ‘Time-base autarky is defined as the degree of self-sufficiency of the microgrid and is determined to the overall period in which power is neither taken nor fed into the overlying electricity grid-level. A key aspect of self-sufficiency in a microgrid system is the availability of an ESS. Examples of urban microgrid systems reported in the literature are listed in Table 1.
2.3 Microgrid control
Communication and execution of instructions between the individual components of a solar PV microgrid system are enabled by a control scheme that operates via the system’s energy requirements (Cagnano, Tuglie and Mancarella, 2020). The major functions of the control strategies are to divide load demand amongst different DG units and maintain the power quality in the microgrid (Hasan and Arif, 2018). (Nasir et al., 2018) utilized a Newton-Raphson power flow analysis for the power-sharing amongst the components of a microgrid system. Effective power management control in a microgrid is possible through machine learning via a technique namely Model Predictive Control (MPC). The MPC technique manages the power variations between the components of a microgrid system (Tungadio et al., 2018). Evaluated the performance of various versions of MPC and discovered that closed-loop MPC was more robust to power changes than open-loop MPC. Microgrids that are dominated by solar PV use converter-level and grid-level MPC to control and manage power flow (Hu et al., 2021). MPC comprises aspects such as cost function and solving algorithms for the optimization of critical parameters like active and reactive power regulation, harmonic reduction, and ripple minimization in microgrid systems (Garcia-Torres et al., 2021). Quadratic programming was used by (Wibowo et al., 2017) to economically dispatch power inside a hybrid microgrid system. The key focus of control techniques is to manage the microgrid system through the modes of operation: grid-connected, island mode, and transition from grid-connected to island operation. The transition mode activates either emergency or through a planned state (Mahmoud, Azher Hussain and Abido, 2014). Grid-connected microgrids are associated with multiple control strategies compared with the other states mainly because of their connection to the utility grid. Notable objectives of the hierarchical control system include the facilitation of load sharing between DG units, voltage and frequency control, energy reserve management, and emergency islanding (Hirsch, Parag and Guerrero, 2018; Cagnano, Tuglie and Mancarella, 2020). The hierarchical control consists of four levels, namely, the inner level, primary, secondary and tertiary control. The stages of hierarchical control for microgrids are depicted in Figure 4.
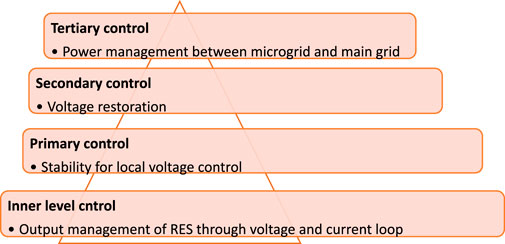
FIGURE 4. Hierarchical level control structure for microgrids. SOURCE: (Shuai et al., 2018).
The hierarchical control encapsulates other various control schemes such as autonomous control and agent-based control (Basak et al., 2012; Hasan and Arif, 2018). A common widely adopted control scheme for DERs under autonomous control is the droop control method (Planas et al., 2013). Various components of a microgrid require instructions for operations based on the system requirements. The droop control technique provides the capacity for wireless communication between components in the microgrid system (Palizban and Kauhaniemi, 2015). Droop control is more appropriate for microgrids that run in island mode than grid-connected mode (Al-Ismail, 2021). Grid-connected microgrids can also use the droop control technique if the ESS can interchange power with the utility grid (Neto et al., 2020). The agent-based control provides a software environment where machines through the operating system provide Agent Management Services (AMS) via agents (electrical loads) (Oyarzabal et al., 2002; Bhaskara and Chowdhury, 2012; Shayanfar and Malek, 2015).
2.3.1 Inner level control
The inner level control includes the current and voltage control loop for managing the output power of DERs in a microgrid system (Palizban and Kauhaniemi, 2015). PV modules, Maximum Power Point Tracking (MPPT), DC/DC converter, and DC/AC inverter are all part of the solar PV system’s control framework. This degree of control supports both the solar PV system’s island mode and its grid-connected condition. On the one hand (Vignesh and Sundaramoorthy, 2016) argue that the active and reactive power relationship should be controlled for the grid-connected state, on the other hand, voltage and frequency relationships are to be controlled for the off-grid state.
2.3.2 Primary control
The primary control has the function of providing stability for local voltage control thus enhancing system performance (Andishgar, Gholipour and Hooshmand, 2017; Garcia-Torres et al., 2021). It achieves stability through the adjustment of the frequency and amplitude of the voltage references for the internal voltage and current loops (Guerrero et al., 2011; Palizban and Kauhaniemi, 2015). A key aspect of this control method is the capability of the microgrid to operate at the reference frequency and voltage of the main grid. The communication between the microgrid and the main grid occurs via a power converter. A power converter is a bidirectional inverter connecting the energy storage of the microgrid and the main grid to facilitate power flow (Fusheng, Ruisheng and Fengquan, 2016b). The power converter manages the reactive power (Q) and active power (P) over the ESS and manages the output power of the DG units. Available inverter control strategies include the droop control method, power-frequency control, and voltage-frequency control (Zhou, Guo and Ma, 2017; Hasan and Arif, 2018). For the grid-connected state, a control structure must be set to manage the PV infeed into the microgrid depending on the supply and battery storage (Porsinger et al., 2017). An ESS along with the Voltage Source Converter (VSC) can assist in reducing output fluctuation of solar PV generation by providing constant power control in the microgrid system. The droop control approach utilizes the grid frequency as a shared signal between DG units to align the system’s active power generation dynamically (Borade and Jain, 2018). The droop control technique performs the key function of voltage sag reduction in an effective manner (Adhikari and Li, 2014; Vignesh and Sundaramoorthy, 2016). The main attraction of the droop control technique is the wireless communication that is achieved between inverters (Shuai et al., 2018). This type of control operates without any communication links and is more reliable and economic than other methods (Tayab et al., 2017; Jiang et al., 2019). The relationship between the frequency/active power (Eq. 2.1) and the frequency/reactive (Eq. 2.2) power is mathematically expressed as below:
Where
Where
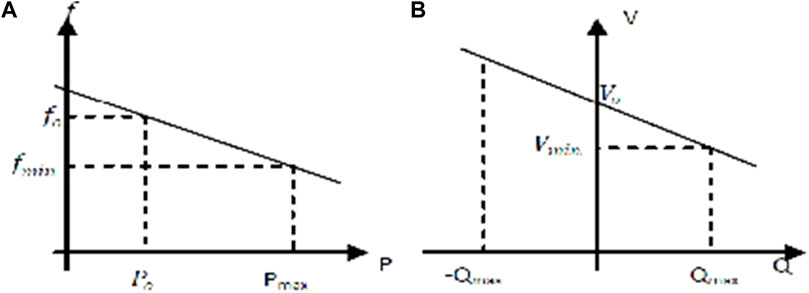
FIGURE 5. Conventional droop characteristics (A) P-f droop (B) Q-V droop (Borade and Jain, 2018).
From Figure 5, a rise in the frequency (f) and voltage (V) coincides with a decrease in both the active and reactive power, respectively. A change in active power and reactive power will lead to the linear change in the voltage - frequency as per the droop character line (Zhou, Guo and Ma, 2017). Figure 5B indicates a negative correlation relationship between the voltage magnitude and the reactive power while Figure 5A shows the negative correlation between the frequency magnitude and the active power. Figure 6 shows the electrical description of the droop control.Where PI Proportional Integral (PI) controller,
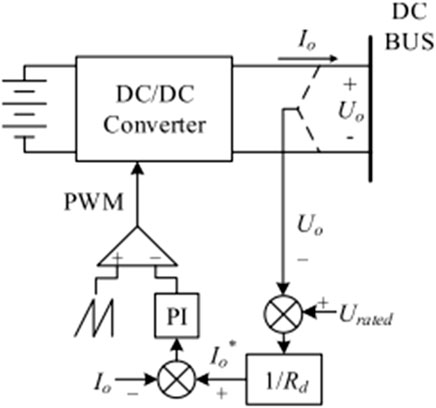
FIGURE 6. Control loop of the droop technique (Jiang et al., 2019).
The PI control is utilized for the reduction of the losses that occur during frequency synchronization (Tegling et al., 2016). A study by (Khaledian and Aliakbar Golkar, 2017) provides an overview of the virtual impedance loop-based control technique by analyzing the active and reactive power dependency through applying a step change via a controlled current source. The simulation results revealed that the increase of the reactive power droop gain improved the power-sharing performance between the components in the microgrid system.
2.3.3 Secondary control
The secondary level of hierarchical control provides managerial control as compared to the primary level. Its core function is to provide supervision and monitoring service by adjusting any variations of frequency and voltage in the system (Palizban and Kauhaniemi, 2015; Tegling et al., 2016; Andishgar, Gholipour and Hooshmand, 2017). A simulation study done by (Guerrero et al., 2011) indicates the compensation of voltage deviations from the primary control corrected by the secondary control. Another function of this level is the facilitation of the transition from an islanded state to a grid-connected mode in the microgrid system (Hou, Hu and Hui, 2010). This level of control consists of two sections, namely, centralized, and decentralized control.
2.3.3.1 Centralized control
The centralized control comprises a Microgrid System Central Controller (MGCC) which oversees the operation of the microgrid (Mingsheng et al., 2018). For grid-connected states, a reference value of frequency and voltage amplitude is established from the utility grid and compared with that from the DERs (Palizban and Kauhaniemi, 2015). Some of the functions of the central controller include synchronization loop for the facilitation of transition from off-grid to on-grid, an approximation of grid impedance for forecast control, and operation of the import or export of active and reactive power to and from the grid (Planas et al., 2013).
2.3.3.2 Decentralized control
For the decentralized control, each subsystem in the microgrid possesses its controller and through it, be able to make and execute instructions based on information obtained from local measurements (voltage and frequency) (Pourbabak, Chen and Zhang, 2017). Each sub-system has the capability of communicating with other sections via a communication link and through it, be able to correct measurement errors via the primary control. For DC microgrids, this type of control achieves the detection of voltage variations via interface converters in the DC common bus (Zhang et al., 2018).
2.3.4 Tertiary control
This is the highest level of hierarchical control whose sole purpose is the management of power flow between the main grid and the microgrid. The management occurs via the regulation of amplitude voltage and frequency (Palizban and Kauhaniemi, 2015; Andishgar, Gholipour and Hooshmand, 2017). Other functions of this level include the restoration of the secondary control reserve in the case of an emergency. The regulation of the amplitude voltage and frequency is accomplished through the comparison of the active/reactive power of the grid with the microgrid with the desired reference (Guerrero et al., 2011; Planas et al., 2013; Mingsheng et al., 2018). A list of control techniques examined for microgrid systems may be found in Table 2.
3 Modeling and simulation of solar photo voltaic microgrids
This section of the comprises of the components utilized for the modeling of solar PV microgrids during both the grid-connected and island mode of operation. Components of solar PV microgrids include DC/DC converter, inverter, solar PV modules, ESS, and electrical loads. The review encapsulates the developed solar PV modules and their performance under various software environments. The DC/DC converter performs the function of converting the DC voltage source to another DC voltage source of different magnitude. The DC/AC inverter performs the function of converting a DC voltage source to an AC voltage source and synchronizes the AC output to the utility grid (Yang et al., 2019). The usage of an inverter in a microgrid system results in drawbacks such as harmonics and voltage imbalances to mention a few. To address the mentioned drawbacks, the inverters are equipped with Pulse Width Modulation (PWM) to maintain stability in the output waveforms. To ensure an efficient operation for a solar PV microgrid, an ESS is necessary to reduce the intermittent and variability of solar PV output (Gao, 2015a). For community solar microgrids, there are two forms of ESS configurations namely aggregate and distribution. Battery Energy Storage System (BESS) which is a form of an ESS has been widely reported in the literature for usage in community solar microgrid systems. Of the various BESS models, the lithium-ion battery models have displayed a higher efficiency in comparison with lead-acid and nickel-cadmium battery models (Vetter and Rohr, 2014; Kularatna, 2015; Breeze, 2018).
3.1 Solar photo voltaic system
3.1.1 Model structure
A series of PV cells connected form a solar PV module. A collection of modules connected in either series or parallel arrangement form a solar PV array (Villalva, Gazoli and Filho, 2009). A common model for a solar cell is the one diode model (Adhikari and Li, 2014). The model shows the equivalent circuit of an ideal PV cell. Figure 7 is a schematic of a practical single solar PV cell.
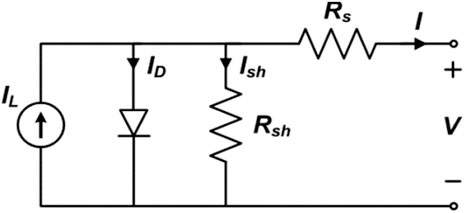
FIGURE 7. Equivalent circuit solar cell (Adhikari and Li, 2014).
In the circuit diagram in Figure 7,
The solar cell is expressed by the parameters
3.1.2 Solar photo voltaic array models
Various solar PV array modules have been developed and integrated into a software environment such as MATLAB/Simulink, Personal Simulation Program with Integrated Circuit Emphasis (PSpice), and PSCAD to mention a few (Nguyen and Nguyen, 2015; Ahlawat, Gupta and Gupta, 2017). MATLAB/Simulink environment includes a developed solar PV module in the Simscape toolbox designed following Eq. 3.1. The major trait of the model is that it is convenient for usage and identifies radiation and temperature sensitivity on the PV module through simulations (Khalil and Ateea, 2015). (Dey et al., 2016) found that the PV module did not function effectively at high temperatures through MATLAB/Simulink simulations in a study done for the Single-Diode Model (SDM) type. The Double-Diode Model (DDM), another popular model type for solar PV systems, has been shown in the literature to predict the performance of a solar PV cell more closely than the SDM. In a study conducted by (Soon and Low, 2015; Yadav and Jaladi, 2018), the simulation analysis revealed the DDM generated more precise and smooth graphs of power generated in comparison with the SDM. A comparison investigation showed that the DDM generated 25 Wp under STC of a 50 Wp solar PV module while the iterative Newton-Raphson model only produced 23 Wp (Bhuvaneswari and Annamalai, 2011). The PSpice software environment also includes PV modules designed through the single PV cell (Eq. (3.1)) offers an analysis of PV modules by conducting simulations through various partially shaded conditions (PSC). The PSpice environment allows for performance analysis for a PV module under PSC through the adjustment of the current source (Jiang et al., 2011). Table 3 provides an overview of the PV array modules developed in various software environments. It encapsulates the performance of the various PV module types and designs under various temperatures and irradiance values.
3.2 Battery energy storage system modeling
The BESS performs the function of mitigating the power supply variability between the load and generation (Gao, 2015a; Abdi, Mohammadi-ivatloo and Javadi, 2017; Alzahrani et al., 2017). The necessity for a BESS is vital for urban microgrid systems to provide power to loads during the island mode. The BESS interfaces with the components DC/DC converter, DC/AC inverter, DC link capacitor, and the transformer within the community solar PV microgrid system. The DC/DC converter topology adopted for urban microgrids is the buck-boost converter which plays the role of charging and discharging the BESS in the microgrid system as it allows bidirectional power flow (Gao, 2015b; Farrokhabadi et al., 2018; Kondrath, 2018). An ESS is capable of balancing power in a solar PV microgrid by working as the load or generator during the charging and discharging modes (Abdi, Mohammadi-ivatloo and Javadi, 2017). BESS models of solar PV microgrids exist in two ESS configurations namely aggregated and distributed ESS. The aggregated ESS is more suited for microgrids compared to distributed ESS as they suppress power fluctuation effectively in the microgrid system (Kook et al., 2006; Li and Joós, 2007; Gao, 2015a). The BESS serves the function of energy management in the microgrid system through functions such as load leveling and peak load shifting (Xia et al., 2015). The system is capable of providing services such as providing local voltage support, grid contingency support, and load shifting. The BESS models can incorporate the commonly developed four electrochemical batteries or controlled voltage sources in the microgrid system (Tremblay, Dessaint and Dekkiche, 2007; Farrokhabadi et al., 2018). (Narayanan, Seema and Singh, 2018) present a solar PV-BES microgrid system with multifunctional Voltage Source Inverters (VSI). VSI is used to generate an output waveform in which parameters such as frequency, voltage, and harmonic content are regulated. Harmonic content generated can have negative effects on electrical devices such as the cause of power quality degradation, distortion of output voltage, and deration of equipment just to mention a few (Kharjule, 2015). Another interesting aspect for BESS is its capability to outperform regular reserve suppliers because of its capability to respond and fully activate the reserve within 20 milliseconds (Adrees, Andami and Milanovic, 2016).
3.2.1 Model structure
The capacity and lifecycle of BESS are reliant on factors such as Depth of Discharge (DOD), rate of discharge, and temperature. The capacity of a battery is mathematically expressed by Eq. 3.2 (Alzahrani et al., 2017):
Where
3.2.2 Economics of battery energy storage system
Numerous studies have been done in the literature on the economics of using different types of batteries in microgrid systems. Battery types like lithium-ion are becoming more affordable, making them competitive for inclusion in microgrid systems (Cammardella et al., 2019). On a cost comparison analysis (Moncecchi et al., 2018), through simulation analysis found out that lead-acid batteries were replaced three times whilst the lithium-ion battery was replaced only once during a certain study period. In a study of battery storage for solar PV pumping system (Khiareddine, Gam and Mimouni, 2019), found lithium-ion batteries to be more economically viable than lead-acid batteries with a lower total net present cost. Table 4 presents an overview of the battery types and applications reported in the literature.
The efficiency of lithium-ion batteries is dependent on factors such as depth of discharge (DoD), charge factor, current, time of float charge, and discharge (Kurzweil, 2015). Li-ON batteries are mostly utilized in solar community solar microgrids as they display an 80% DoD as compared to the 50% DoD of lead-acid batteries (Vetter and Rohr, 2014).
3.3 Inverter modeling
Energy conversion is necessary for a microgrid system to enable the power flow interactions amongst the individual components (Shintre and Mulla, 2016). Inverters can be classified as Current Source Inverters (CSI) and VSIs concerning the power supply (Gao and Sun, 2016). The DC input type being voltage source and current source respectively categorizes a VSI circuit and the CSI. A VSI utilizes the DC input voltage obtained from sources such as a cell battery, rectifier, or a photovoltaic array (Gao, 2015b; Gao and Sun, 2016; Shintre and Mulla, 2016). A common waveform generated by a VSI is a Pulse Width Modulation (PWM) with the voltage source being a three-level Adjustable Speed Drive (ASD) (Kharjule, 2015). Three-phase VSI is made up of three inverter legs and they are mostly utilized for medium to high power applications (>5 kW) e.g. AC electric drives (Kharjule, 2015; Siwakoti, Forouzesh and Pham, 2018). The layout of the inverter is such that it contains three half-bridge inverters connected in a parallel configuration. The three inverters have a phase difference of 120° and the configuration of the inverters generates a three-phase voltage source (Manias, 2017). The power requirements of the microgrid system dictate the exchanges of power between the microgrid systems and the utility grid via a bidirectional inverter/rectifier (Debela, Ensermu and Bhattacharya, 2017). VSI generates an output waveform in which parameters such as frequency, voltage, and harmonic content are controlled (Narayanan, Seema and Singh, 2018). A study by (Ashabani and Mohamed, 2014), demonstrated the high response time of using VSI to track grid frequencies in the grid-connected state of a microgrid system. According to a study by (Vazquez et al., 2012),a parallel connected VSI system was used to balance the current and regulate the output voltage in a microgrid system.
3.3.1 Harmonics in inverters
Harmonics are characterized by distortion and divergence of the current and voltage waveforms from the sinusoidal waveforms (Gray and Morsi, 2015). Low order harmonics have more adverse effects on inverters than high order harmonics (Onduction and Vsi, 2015). To ensure proper operation of inverters, control techniques, and modulation techniques are adopted to reduce the harmonics in the system. Power quality analysis in inverters is performed by analyzing Total Harmonic Distortion (THD) in a microgrid system. According to IEEE standards, the THD of a line converter must be kept below 5% (Alhamrouni et al., 2019). In a study by (Kulkarni and Nehete, 2014), a comparative analysis of the simulated and measured output voltage revealed a voltage difference of 6% at a system THD of 1.25%. A study by (Nomm, Ronnberg and Bollen, 2018) indicated that high voltage THD is more common in an island mode of operation than in a microgrid system that is connected to the grid. Control of three-phase VSI is achieved by techniques such as the PWM and stepped-wave inverter. PWM is the most utilized device capable of reducing harmonics in devices with a history that ranges from 50 years mainly because of its capability to operate at lower frequencies (Aguilera et al., 2018). PWM inverters are capable of achieving control of the amplitude output voltage of the VSI (Kim, 2017). The inverter achieves lower harmonics by undergoing multiple switching within the inverter at a constant DC input voltage. According to a study done by (Sarwar and Asghar, 2011), changing the angles of a multi-winding transformer led to a decrease in THD. At an angle of 110° the recorded THD was 22.9%, while at 125% the recorded THD was about 32%. There are three common control techniques for PWM inverters, and they include single PWM, Multiple PWM, and Sinusoidal Pulse Width Modulation (SPWM). A study conducted by (Debela, Ensermu and Bhattacharya, 2017) found that using an SPWM inverter produced a 300 V peak on the AC power supply, resulting in a 95% conversion efficiency under steady-state conditions. The most frequently used modulation is the SPWM because of its apparent usage in industrial applications (Kim, 2017). For single PWM control, there is one pulse for every half cycle. The voltage generated is adjusted by varying the width of the single pulse (Kharjule, 2015; Aguilera et al., 2018).
3.3.2 Sinusoidal pulse width modulation inverter
The SPWM technique is capable of voltage modulation for each cycle with a fixed switching frequency (Kim, 2017). For each half-cycle of this technique, multiple pulses occur, and the duration of each pulse varies according to the sine wave scale. The sinusoidal waveform is generated from the comparison between two waveforms namely, the triangular wave (a carrier wave) and the control wave (sinusoidal). The sinusoidal AC voltage reference signal Vref and the high-frequency carrier signal Vc go through a comparison device and the intersection of the two signals indicate the switching states of the inverter (Gao and Sun, 2016; Manias, 2017). Figure 8 illustrates a schematic view of the sinusoidal comparison technique process.
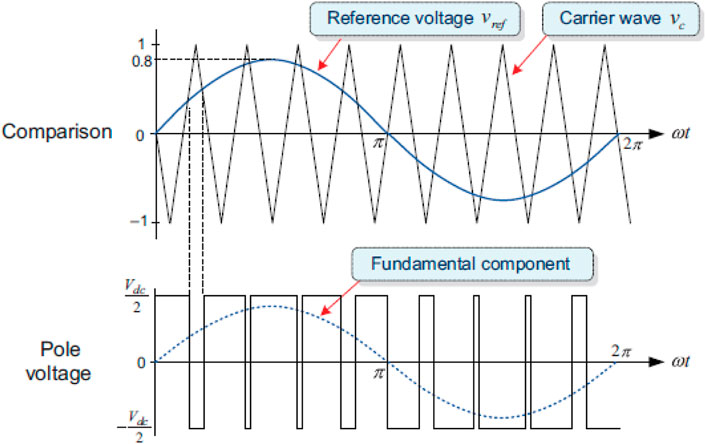
FIGURE 8. The sinusoidal PWM technique process (Kim, 2017).
It is visible that when Vref > Vc, the pole voltage (Vdc/2) is at its maximum, and when Vref < Vc, the pole voltage is at its minimum (- Vdc/2). Linear modulation can only be achieved when the amplitude of Vref remains below the peak of the Vc, i. e Vref ≤ Vdc/2 (Kim, 2017). Through the variation of the modulation waveform, control of the amplitude and frequency of the output can be achieved. The relation between the carrier and modulating waves is denoted by the Modulation Index (MI) (Eq. 3.3.) (Aguilera et al., 2018).
Where
3.4 Electrical load modeling
Modeling of electric loads in a microgrid system is necessary for energy management amongst the components in the system. Loads in a microgrid system affect the control strategies and stability of the microgrid (Peng et al., 2022). Critical, general, sensitive, and non-sensitive, controllable, and non-controllable loads are all types of loads found in microgrid systems. A load management system is essential in a microgrid system because critical loads found in hospitals and nursing homes require an ongoing supply of electricity (Moran, 2016). Load modeling is a key technique for energy management strategies that involve estimating the energy consumption of an electrical load. Creating load models is a mammoth task due to a variety of factors such as weather and dynamic human behaviors (Alzahrani et al., 2017). mention that, for simplicity reasons, load models can be developed using a constant electric impedance. The objective of load modeling is to develop simple math modeling to approximate load conduct (Arif et al., 2018). Load models exist in two states, namely, static, and dynamic models. Due to the nature of modeling loads, the electrical appliances in a residential home can be modeled as static load models as most of them do not characterize as highly non-linear loads. A common load modeling technique is measurement-based load modeling which gathers load data from data acquisition equipment to deduce load characteristics (Jin, Renmu and Hill, 2006). Another load modeling technique, component-based load modeling, extracts energy information by inspecting the composition of the electrical load (Ju et al., 2018). Component-based load models are used to aggregate electrical loads in residential homes (Arif et al., 2018). Energy consumption data from smart meters is utilized to infer the household characteristics in a residential household (Fahim and Sillitti, 2019). Energy usage patterns are used to represent user behavior characteristics (Collin et al., 2014; Mcloughlin, Duffy and Conlon, 2015). (Labeeuw and Deconinck, 2016) propose a load model that creates load profiles through the clustering characterization technique. It’s an unsupervised technique whose sole function is to identify various types of energy consumption behavior in large aggregated residential customers (Jin et al., 2017). (Tang et al., 2019) present a data-driven online aggregated load modeling technique to model the load of aggregated users.
3.4.1 Static load models
Static load models represent the active power and the reactive power in a system as a function of the voltage and frequency variations (Arif et al., 2018; EL-Shimy et al., 2018). The models are represented mathematically by algebraic equations. Examples of static loads are resistive loads and light bulbs. A drawback of static loads is the lack of accurately representing the dynamic behavior of loads (IEEE, 1995). Static models that have been developed include the Zero-Inflated Poisson (ZIP) model, exponential model, frequency-dependent model, and the Electric Power Research Institute (EPRI) LOADSYN Model. Among various literature studies reported, the ZIP model is the most utilized for analyzing the static characteristics of electrical loads (Gu, Ai and Wu, 2005; Arif et al., 2018). The ZIP model which includes active power (Eq. 3.4), and reactive power model (Eq. 3.5) is represented mathematically by the equation below:
Where
3.4.2 Dynamic models
Dynamic load models represent the active and reactive powers in a system as a function of voltage and time (Arif et al., 2018). An example of a dynamic load model is the Induction Motor (IM) of which portrays a highly nonlinear behavior (Moyano and Ledwich, 2010). A dynamic model used for a residential home is the exponential dynamic load model (Yamashita et al., 2012). The IM model is characterized by a time-based response to a voltage change. The IM model is used for transient simulations due to the fast-changing nature of dynamic loads (Yamashita et al., 2012; Abdelsalam et al., 2014). Table 6 comprises of a list of common electrical load components in a microgrid system.
4 Optimization of microgrids through energy management system strategies
Control and EMS strategies are essential to the effectiveness of a microgrid systems. Optimization of microgrid systems can be conducted in optimization software such as HOMERPro (Baral, Behera and Kisku, 2022). performed an optimization analysis for a standalone hybrid microgrid system on HOMERPro, and the results revealed that the optimal system cost $377,902 compared to $707,752 for the same capacity diesel generator. A rapidly rising technique utilized in the optimization of a microgrid is machine learning. Effective energy management inside the microgrid is achieved by machine learning through approaches including linear and dynamic programming which enable schedule economic dispatch and unit commitments of the microgrid’s components (Shrivastwa et al., 2019). An example of the energy scheduling strategies has been reported in a DC community solar microgrid in the city of Wisconsin, Milwaukee. The DC community microgrid composes of nine dwellings interconnected through a 380 Vdc transmission line. The microgrid system composes of a solar PV system as the main DG unit, a Home Energy Management System (HEMS), and Microgrid Energy Management System (MEMS). Customers in the community solar microgrid take advantage of the HEMS and MEMS service platforms that are linked to the internet cloud to maximize cost reductions in their households (Palaniappan et al., 2017).
4.1 Linear programming
The Linear Programming (LP) technique utilizes forecasts and predictions to optimize microgrid systems. Optimization models such as Distributed Energy Resources Customer Adoption Model (DER-CAM) have been utilized to encompass Mixed-Integer Linear Programming (MILP) for microgrids with various energy types (Mashayekh et al., 2017). found out that through MILP, multi-modeling nodes were utilized for optimal siting of electrical and heating/cooling networks (Santos et al., 2021). used MILP for optimal dispatch strategy for grid-connected microgrids in the simulation environment, HOMERPro and MATLAB, and the results demonstrated that the MILP strategy produced lower NPC costs than the Load Following (LF) and Cycle Charging (CC) strategies. Through linear programming, the standard and forecast load profiles of the community will aid in the effective sizing and design techniques of the microgrid system through control algorithms. In a microgrid system, LP optimization reduced the cost of variable-priced electricity by 19% less than heuristic state machine logic, according to a study by (Shufian and Mohammad, 2022).
4.2 Dynamic programming
Another machine learning technique for the optimization model of microgrid energy management is Dynamic Programming (DP). The DP technique uses the lowest production costs and meets the necessary constraints (Borra and Debnath, 2018). Multi-parameter dynamic programming was utilized for optimization through addressing issues of non-linear loads and power supply intermittency of DG units (Wang et al., 2020). In a study conducted by (Zeng et al., 2019), an approximate DP was used for real-time energy management of a microgrid system and the results showed a reduction in daily microgrid costs network versus reference approaches. Table 7 presents list of the optimization techniques studied in literature.
5 Challenges of solar community microgrids
The integration of solar PV as a DG unit in community microgrids brings about technical challenges through the three modes of operation. The challenges experienced by solar community microgrids in urban settlements are more prominent during the on-grid operation mode (Qazi, 2017; Hossain et al., 2019). Through islanding, a balance between the generation and load must be kept to avoid faults in distribution transmission lines of which can be harmful to the individual components in the microgrid system (Faure et al., 2017). The technical challenges of microgrids include control, protection, and re-synchronization to mention a few.
5.1 Microgrid stability
Grid-connected microgrids are more vulnerable to instability issues in comparison with island microgrids due to the synchronization of the voltage and frequency with the main utility grid. Conventional power stations in SSA are of unidirectional power type and thereby susceptible to faults that may result in power outages (Sampath, Prasad and Samikannu, 2018). Thereby, there is a need for advanced control strategies that can safeguard the integration of microgrids with utility grids in SSA. The integration of solar microgrids with the utility grid requires a control strategy to avoid deviation of the system voltage and frequency from its setpoint value (Sivarasu, Chandira Sekaran and Karthik, 2015; Kumar and Ravikumar, 2016).
5.2 Protection
The integration of micro-sources with a unidirectional electricity network poses challenges in analyzing the direction and magnitude of current flow in the system. Solar PV is weather-dependent RETs and thereby it experiences intermittent power generation throughout the day (Mariam, Basu and Conlon, 2016). The variability in current flow in the system results in a mal-operation of protection devices in networks as the fault current path changes depending on fault location (Miveh et al., 2012; Hossain et al., 2019). (Salomonsson, Söder and Sannino, 2009) propose a protection system for low-voltage DC microgrids that incorporates the use of fuses and circuit breakers to isolate faults quickly and minimize the effects of disturbances. The implementation of both grid-connected and island microgrids is heavily reliant on control strategies that can manage and monitor the energy flow while also ensuring the protection of the components in the microgrid settlement (Hasan and Arif, 2018).
6 Conclusion
The paper provides a comprehensive examination of microgrid system control techniques, simulation modeling, and optimization strategies. Through the shared use of renewable energy resources integrated into their building, energy management in a microgrid settlement allows an individual customer to realize cost savings. Control strategies such as droop control have been widely used in microgrid systems due to their ability to operate without communication links and their economical nature. The review encompasses the performance of the distinct model components of microgrids which were evaluated using a variety of software environments, including MATLAB/Simulink, PSCAD, and Pspice. Simulation analysis revealed double-diode models depicted a solar PV module more accurately in comparison with single-diode models. Lithium-ion batteries have been preferred as storage devices for microgrid systems due to their ability to have high energy density and longer lifespans compared to other battery types such as lead-acid and nickel-cadmium batteries. Optimization techniques for microgrid systems utilize machine learning tools for economic dispatch of power usage. The integration of solar PV as a DG unit in community solar microgrids brings about technical challenges through the three modes of operation. The challenges experienced by solar microgrids in urban settlements are more prominent during the on-grid operation mode due to synchronization and protection issues. Techniques such as the Model Predictive Control and other various communication protocols which encompass machine learning can alleviate the challenges of grid-connected microgrids. A concept of microgrid digital twins, which is a digital representation of a microgrid that interacts with the physical microgrid in real-time, will be developed in the near future. Protection issues rising from faults can be mitigated through the new technology of microgrid digital twins. The integration of solar PV systems into micro-grids is more explored due to their affordability and advancements in technology can alleviate their problems stemming from their intermittent nature.
Author contributions
All authors listed have made a substantial, direct, and intellectual contribution to the work and approved it for publication.
Conflict of interest
The authors declare that the research was conducted in the absence of any commercial or financial relationships that could be construed as a potential conflict of interest.
Publisher’s note
All claims expressed in this article are solely those of the authors and do not necessarily represent those of their affiliated organizations, or those of the publisher, the editors and the reviewers. Any product that may be evaluated in this article, or claim that may be made by its manufacturer, is not guaranteed or endorsed by the publisher.
References
Abdelsalam, A. A., Gabbar, H. A., Musharavati, F., and Pokharel, S. (2014). Dynamic aggregated building electricity load modeling and simulation. Simul. Model. Pract. Theory 42, 19–31. doi:10.1016/j.simpat.2013.12.005
Abdi, H., Mohammadi-ivatloo, B., and Javadi, S. (2017). Energy storage systems, distributed generation systems. Elsevier. doi:10.1016/B978-0-12-804208-3.00007-8
Adhikari, S., and Li, F. (2014). Coordinated V-f and P-Q control of solar photovoltaic generators with MPPT and battery storage in microgrids. IEEE Trans. Smart Grid 5 (3), 1270–1281. doi:10.1109/TSG.2014.2301157
Adrees, A., Andami, H., and Milanovic, J. V. (2016). “Comparison of dynamic models of battery energy storage for frequency regulation in power system,” in Proceedings of the 18th Mediterranean Electrotechnical Conference: Intelligent and Efficient Technologies and Services for the Citizen. doi:10.1109/MELCON.2016.7495314
Aguilera, R. P., Acuna, P., Konstantinou, G., Vazquez, S., and Leon, J. I. (2018). Basic control principles in power electronics, control of power electronic converters and systems. Elsevier. doi:10.1016/B978-0-12-805245-7.00002-0
Ahlawat, A., Gupta, D., and Gupta, S. K.PG Scholar, Deen Bandhu Chotu Ram University of Science and Technology, Sonepat, Haryana, India.Project Engineer, Wipro Technology, Greater Noida, India.Professor, Department of Electrical Engineering, DCR University of Science and Technology, Sonepat, Haryana, India (2017). Modeling of a pv array and implementation of an efficient mppt based control mechanism in stand-alone photovoltaic systems. i-manager’s J. Circuits Syst. 5 (2), 51. doi:10.26634/jcir.5.2.13665
Al-Ismail, F. S. (2021). DC microgrid planning, operation, and control: A comprehensive review. IEEE Access 9, 36154–36172. doi:10.1109/ACCESS.2021.3062840
Alhamrouni, I., Wahab, W., Salem, M., Rahman, N. H. A., and Awalin, L. (2019). Modeling of micro-grid with the consideration of total harmonic distortion analysis. Indonesian J. Electr. Eng. Comput. Sci. 15 (2), 581–592. doi:10.11591/ijeecs.v15.i2.pp581-592
Alzahrani, A., Ferdowsi, M., Shamsi, P., and Dagli, C. H. (2017). Modeling and simulation of microgrid. Procedia Comput. Sci. 114, 392–400. doi:10.1016/j.procs.2017.09.053
Andishgar, M. H., Gholipour, E., and Hooshmand, R. allah (2017). An overview of control approaches of inverter-based microgrids in islanding mode of operation. Renew. Sustain. Energy Rev. 80, 1043–1060. doi:10.1016/j.rser.2017.05.267
Ansari, S., Zeinoddini-Meymand, H., and Niknam, T. (2019). “Optimal Operation of AC and DC hybrid Microgrid in two management scenarios,” in ICEE 2019 - 27th Iranian Conference on Electrical Engineering, 856–862. doi:10.1109/IranianCEE.2019.8786484
Arif, A., Wang, Z., Wang, J., Mather, B., Bashualdo, H., and Zhao, D. (2018). Load modeling - a review. IEEE Trans. Smart Grid 9 (6), 5986–5999. doi:10.1109/TSG.2017.2700436
Ashabani, S. M., and Mohamed, Y. A. R. I. (2014). New family of microgrid control and management strategies in smart distribution grids-analysis, comparison and testing. IEEE Trans. Power Syst. 29 (5), 2257–2269. doi:10.1109/TPWRS.2014.2306016
Ashford, M., and Pagkalinawan, L. (2019). Despite challenges, Urban microgrids increase resilience, Chemonics. Available at: https://www.climatelinks.org/blog/despite-challenges-urban-microgrids-increase-resilience (Accessed: February 10, 2020).
Baral, J. R., Behera, S. R., and Kisku, T. (2022). “Design and economic optimization of community load based microgrid system using HOMER pro,” in 2022 International Conference on Intelligent Controller and Computing for Smart Power (ICICCSP).
Baran, M., and Mahajan, M. (2003). DC distribution for industrial systems: Opportunities and challenges. IEEE Trans. Ind. Appl. 39 (9), 1596–1601. doi:10.1109/tia.2003.818969
Basak, P., Chowdhury, S., Halder nee Dey, S., and Chowdhury, S. (2012). A literature review on integration of distributed energy resources in the perspective of control, protection and stability of microgrid. Renew. Sustain. Energy Rev. 16 (8), 5545–5556. doi:10.1016/j.rser.2012.05.043
Bellia, H., Youcef, R., and Fatima, M. (2014). A detailed modeling of photovoltaic module using MATLAB. NRIAG J. Astronomy Geophys. 3 (1), 53–61. doi:10.1016/j.nrjag.2014.04.001
Bhaskara, S. N., and Chowdhury, B. H. (2012). “Microgrids - a review of modeling, control, protection, simulation and future potential,” in IEEE Power and Energy Society General Meeting, 1–7. doi:10.1109/PESGM.2012.6345694
Bhuvaneswari, G., and Annamalai, R. (2011). “Development of a solar cell model in MATLAB for PV based generation system,” in Proceedings - 2011 Annual IEEE India Conference: Engineering Sustainable Solutions. doi:10.1109/INDCON.2011.6139509
Bifaretti, S., Cordiner, S., Mulone, V., Rocco, V., Rossi, J. L., Spagnolo, F., et al. (2017). “Grid-connected microgrids to support renewable energy sources penetration,” in Energy Procedia, 2910–2915. doi:10.1016/j.egypro.2017.03.658
Bila, M., Opathella, C., and Venkatesh, B. (2016). Grid connected performance of a household lithium-ion battery energy storage system. J. Energy Storage 6, 178–185. doi:10.1016/j.est.2016.04.001
Bokhari, A., Alkan, A., Dogan, R., Diaz-Aguilo, M., de Leon, F., Czarkowski, D., et al. (2014). Experimental determination of the ZIP coefficients for modern residential, commercial, and industrial loads. IEEE Trans. Power Deliv. 29 (3), 1372–1381. doi:10.1109/TPWRD.2013.2285096
Borade, S. R., and Jain, P. A. M. (2018). Analysis of voltage-frequency ( VF ) droop control method for AC microgrid application. Int. J. Adv. Res. Sci. Eng. 7 (2), 409–417.
Borra, V. S., and Debnath, K. (2018). Dynamic programming for solving unit commitment and security problems in microgrid systems. 2018 IEEE Int. Conf. Innovative Res. Dev. 2018, 1–6. doi:10.1109/ICIRD.2018.8376313
Breeze, P. (2018). An introduction to energy storage technologies. Power System Energy Storage Technologies. Amsterdam, Netherlands: Elsevier BV, 1–11.
Cagnano, A., Tuglie, E. De, and Mancarella, P. (2020). Microgrids : Overview and guidelines for practical implementations and operation. Appl. Energy 258, 114039. doi:10.1016/j.apenergy.2019.114039
Cammardella, N. J., Moye, R. W., Chen, Y., and Meyn, S. P. (2019). “An energy storage cost comparison: Li-Ion batteries vs distributed load control,” in Clemson University Power Systems Conference. doi:10.1109/PSC.2018.8664042
Carneiro, A. S., Araujo, L. F., Pereira, J. L. R., Garcia, P. A., Melo, I. D., Amaral, M. B., et al. (2017). Static load modeling based on field measurements. IEEE Manch. PowerTech, 1–5. doi:10.1109/PTC.2017.7981230
Castrejon-Campos, O., Aye, L., and Hui, F. K. P. (2022). Effects of learning curve models on onshore wind and solar PV cost developments in the USA. Renew. Sustain. Energy Rev. 160, 112278. doi:10.1016/j.rser.2022.112278
Cole, W. J., Frew, B. A., Gagnon, P. J., Richards, J., Sun, Y., Margolis, R. M., et al. (2017). SunShot 2030 for photovoltaics (PV): Envisioning a low-cost PV future’, U.S. National renewable energy laboratory. Available at: https://www.nrel.gov/docs/fy17osti/68105.pdf%0Ahttp://www.osti.gov/servlets/purl/1392206/.
Collin, A. J., Tsagarakis, G., Kiprakis, A. E., and McLaughlin, S. (2014). Development of low-voltage load models for the residential load sector. IEEE Trans. Power Syst. 29 (5), 2180–2188. doi:10.1109/TPWRS.2014.2301949
Cuzner, R. M., Palaniappan, K., and John Shen, Z. (2015). “System specification for a DC community microgrid and living laboratory embedded in an urban environment,” in 2015 International Conference on Renewable Energy Research and Applications, ICRERA, 1119–1125. doi:10.1109/ICRERA.2015.7418584
Neves, M. D. S., Aredes, M. A., Khezri, H., Ida, E. T., and Aredes, M. (2017). “Advantages of grid-tied DC microgrid,” in 14th Brazilian Power Electronics Conference, COBEP, 2018-January, 1–6. doi:10.1109/COBEP.2017.8257249
Davari, M., Gao, W., Jiang, Z. P., and Lewis, F. L. (2021). An optimal primary frequency control based on adaptive dynamic programming for islanded modernized microgrids. IEEE Trans. Autom. Sci. Eng. 18 (3), 1109–1121. doi:10.1109/TASE.2020.2996160
Debela, T., Ensermu, G., and Bhattacharya, A. (2017). “Design, control and simulation of grid connected DC/AC microgrid for residential applications,” in Proceedings of the 2017 2nd IEEE International Conference on Electrical, Computer and Communication Technologies, 6–11. doi:10.1109/ICECCT.2017.8117998
Dey, B. K., Khan, I., Mandal, N., and Bhattacharjee, A. (2016). “Mathematical modelling and characteristic analysis of Solar PV Cell,” in 7th IEEE Annual Information Technology, Electronics and Mobile Communication Conference, IEEE IEMCON. doi:10.1109/IEMCON.2016.7746318
Donahue, E. J. (2019). Microgrids: Applications, solutions. IntechOpen: Case Studies and Demonstratons. doi:10.5772/intechopen.835560
El-Shimy, M., Mostafa, N., Afandi, A., Sharaf, A., and Attia, M. A. (2018). Impact of load models on the static and dynamic performances of grid-connected wind power plants: A comparative analysis. Math. Comput. Simul. 149, 91–108. doi:10.1016/j.matcom.2018.02.003
Fahim, M., and Sillitti, A. (2019). Analyzing load profiles of energy consumption to infer household characteristics using smart meters. Energies 12 (773). doi:10.3390/en12050773
Farrokhabadi, M., Konig, S., Canizares, C. A., Bhattacharya, K., and Leibfried, T. (2018). Battery energy storage system models for microgrid stability analysis and dynamic simulation. IEEE Trans. Power Syst. 33 (2), 2301–2312. doi:10.1109/TPWRS.2017.2740163
Faure, M., Salmon, M., El Fadili, S., Payen, L., and Kerlero, G. (2017). Urban microgrids :overview, challenges and opportunities. Available at: http://www.enea-consulting.com/wp-content/uploads/2017/02/Urban-Microgrids-Public-report_VF3.pdf.
Fusheng, L., Ruisheng, L., and Fengquan, Z. (2016a). “Composition and classification of the microgrid,” in Microgrid technology and engineering application, 11–27. doi:10.1016/b978-0-12-803598-6.00002-4
Fusheng, L., Ruisheng, L., and Fengquan, Z. (2016b). “Control and operation of the microgrid,” in Microgrid technology and engineering application (Elsevier), 47–67.
Gao, D. W. (2015a). “Applications of ESS in renewable energy microgrids,” in Energy storage for sustainable microgrid, 36–76. doi:10.1016/B978-0-12-803374-6.00002-0
Gao, D. W. (2015b). “Interfacing between an ESS and a microgrid,” in Energy storage for sustainable microgrid, 80–116. doi:10.1016/B978-0-12-803374-6.00003-2
Gao, D. Z., and Sun, K. (2016). “DC–AC inverters,” in Electric renewable energy systems, 355–381. doi:10.1016/B978-0-12-804448-3/00016-5
Garcia-Torres, F., Zafra-Cabeza, A., Silva, C., Grieu, S., Darure, T., and Estanqueiro, A. (2021). Model predictive control for microgrid functionalities: Review and future challenges. Energies 14 (5), 1296–1326. doi:10.3390/en14051296
Gašparović, G., Kilkis, S., Krajacic, G., and Duic, N. (2016). Campus and community micro grids integration of building integrated photovoltaic renewable energy sources: Case study of Split 3 area, Croatia - Part A. Therm. Sci. 20 (4), 1135–1145. doi:10.2298/TSCI151203080G
Gray, M., and Morsi, W. G. (2015). New power quantities definition for low and high order harmonic distortion. Electric Power Systems Research, 11–18. doi:10.1016/j.epsr.2014.09.004
Gu, C., Ai, Q., and Wu, J. (2005). A study of effect of different static load models and system operating constrains on static voltage stability, 44–49.
Guerrero, J. M., Vasquez, J. C., Matas, J., de Vicuna, L. G., and Castilla, M. (2011). Hierarchical control of droop-controlled AC and DC microgrids - a general approach toward standardization. IEEE Trans. Ind. Electron. 58 (1), 158–172. doi:10.1109/TIE.2010.2066534
Gunasekaran, M., Ismail, H. M., Chokkalingam, B., Mihet-Popa, L., and Padmanaban, S. (2018). Energy management strategy for rural communities’ DC micro grid power system structure with maximum penetration of renewable energy sources. Appl. Sci. Switz. 8 (4), 585. doi:10.3390/app8040585
Gupta, A., Doolla, S., and Chatterjee, K. (2018). Hybrid AC-DC microgrid: Systematic evaluation of control strategies. IEEE Trans. Smart Grid 9 (4), 3830–3843. doi:10.1109/TSG.2017.2727344
Gürtürk, M. (2019). Economic feasibility of solar power plants based on PV module with levelized cost analysis. Energy 171, 866–878. doi:10.1016/j.energy.2019.01.090
Hamza, A., Tahir, H. B., Siraj, K., and Nasir, M. (2019). “Hybrid AC/DC microgrid for residential applications,” in 2019 IEEE Third International Conference on DC Microgrids, 1–5.
Hasan, M. A., and Arif, M. S. Bin (2018). “Microgrid architecture, control, and operation,” in Hybrid-renewable energy systems in microgrids, 24–36. doi:10.1016/B978-0-08-102493-5/00002-9
Hirsch, A., Parag, Y., and Guerrero, J. (2018). Microgrids: A review of technologies, key drivers, and outstanding issues. Renew. Sustain. Energy Rev. 90, 402–411. doi:10.1016/j.rser.2018.03.040
Hossain, A., Pota, H. R., Hossain, M. J., and Blaabjerg, F. (2019). Evolution of microgrids with converter-interfaced generations: Challenges and opportunities. Int. J. Electr. Power & Energy Syst. 109, 160–186. doi:10.1016/j.ijepes.2019.01.038
Hou, C., Hu, X., and Hui, D. (2010). “Hierarchical control techniques applied in micro-grid,” in 2010 International Conference on Power System Technology: Technological Innovations Making Power Grid Smarter, POWERCON, 1–5. doi:10.1109/POWERCON.2010.5666418
Howell, S., Rezgui, Y., Hippolyte, J. L., Jayan, B., and Li, H. (2017). Towards the next generation of smart grids: Semantic and holonic multi-agent management of distributed energy resources. Renewable and Sustainable Energy Reviews, 193–214. doi:10.1016/j.rser.2017.03.107
Hu, J., Shan, Y., Guerrero, J. M., Ioinovici, A., Chan, K. W., and Rodriguez, J. (2021). Model predictive control of microgrids – an overview. Renew. Sustain. Energy Rev. 136, 110422. doi:10.1016/j.rser.2020.110422
IEEE (1995). Standard load models for power flow and dynamic performance simulation. IEEE Trans. Power Syst. 10 (3), 1302–1313.
Jain, T., and Raju, E. S. N. P. (2013). “Hybrid AC/DC micro grid: An overview,” in Fifth International Conference on Power and Energy Systems, Kathmandu, 28–30. doi:10.13140/2.1.1847.8081
Jiang, W., Yang, C., Liu, Z., Liang, M., Li, P., and Zhou, G. (2019). A hierarchical control structure for distributed energy storage system in DC micro-grid. IEEE Access 7, 128787–128795. doi:10.1109/ACCESS.2019.2939626
Jiang, Y., Qahouq, J. A. A., Orabi, M., et al. (2011). Matlab/pspice hybrid simulation modeling of solar PV cell/module, 1244–1250.
Jin, L., Lee, D., Sim, A., Borgeson, S., Wu, K., Spurlock, C. A., et al. (2017). “Comparison of clustering techniques for residential energy behavior using smart meter data,” in Artificial intelligence for smart grids and smart buildings, 260–266.
Jin, M., Renmu, H., and Hill, D. J. (2006). “Composite load modeling via measurement approach,” in 2006 IEEE Power Engineering Society General Meeting, PES, 663–672. doi:10.1109/pes.2006.1708962
Ju, P., Wu, F., Chen, Q., Han, J., Tang, Y., Fan, Y., et al. (2018). “Modeling methods for electric loads in a real power grid,” in 2018 5th International Conference on Electrical and Electronics Engineering, ICEEE, 166–172. doi:10.1109/ICEEE2.2018.8391323
Justo, J. J., Mwasilu, F., Lee, J., and Jung, J. W. (2013). AC-microgrids versus DC-microgrids with distributed energy resources: A review. Renew. Sustain. Energy Rev. 24, 387–405. doi:10.1016/j.rser.2013.03.067
Kabalci, E., Irgan, H., and Kabalci, Y. (2018). “Hybrid microgrid system design with renewable energy sources,” in Proceedings - 2018 IEEE 18th International Conference on Power Electronics and Motion Control, PEMC, 387–392. doi:10.1109/EPEPEMC.2018.8521840
Khaledian, A., and Aliakbar Golkar, M. (2017). Analysis of droop control method in an autonomous microgrid. J. Appl. Res. Technol. 15 (4), 371–377. doi:10.1016/j.jart.2017.03.004
Kharjule, S. (2015). “Voltage source inverter,” in 2015 International Conference on Energy Systems and Applications, (Icesa), 537–542. doi:10.1109/ICESA.2015.7503407
Khiareddine, A., Gam, O., and Mimouni, M. F. (2019). “Techno-economic analysis of the lithium-ion and lead-acid battery in Photovoltaic pumping system,” in in 2019 19th international conference on Sciences and Techniques of Automatic control & computer engineering (STA), Sousse, Tunisia, March 24-26, 2019, 417–422.
Kondrath, Nisha (2018). An overview of bidirectional DC-DC converter topologies and control strategies for interfacing energy storage systems in microgrids. J. Electr. Eng. 6 (1), 11–17. doi:10.17265/2328-2223/2018.01.002
Kook, K. S., McKenzie, K. J., Liu, Y., and Atcitty, S. (2006). “A study on applications of energy storage for the wind power operation in power systems,” in 2006 IEEE Power Engineering Society General Meeting, PES, 1–5. doi:10.1109/pes.2006.1708884
Kounev, V., Tipper, D., Grainger, B. M., and Reed, G. (2014). “Analysis of an offshore medium voltage DC microgrid environment — Part II: Communication network architecture,” in 2014 IEEE PES T&D Conference and Exposition. doi:10.1109/tdc.2014.6863567
Kularatna, N. (2015). “Rechargeable battery technologies,” in Energy storage devices for electronic systems. doi:10.1016/B978-0-12-407947-2.00002-X
Kulkarni, V., and Nehete, R. (2014). Simulation and analysis of photo-voltaic ( PV ) based solar inverter system. Int. J. Soft Comput. Eng. (IJSCE) 3 (6), 114–120.
Kumar, Y. V. P., and Ravikumar, B. (2016). Integrating renewable energy sources to an urban building in India: Challenges, opportunities, and techno-economic feasibility simulation. Technol. Econ. Smart Grids Sustain. Energy 1 (1). doi:10.1007/s40866-015-0001-y
Kurzweil, P. (2015). “Lithium battery energy storage : State of the art including lithium air and lithium sulfur systems,” in Electrochemical energy storage for renewable sources and grid balancing (Elsevier B.V.), 269–307. doi:10.1016/B978-0-444-62616-5.00016-4
Labeeuw, W., and Deconinck, G. (2016). Residential electrical load model based on mixture model clustering and markov models. IEEE Trans. Ind. Inf. 9, 1561–1569. doi:10.1109/TII.2013.2240309
Li, W., and Joós, G. (2007). “Performance comparison of aggregated and distributed energy storage systems in a wind farm for wind power fluctuation suppression,” in 2007 IEEE Power Engineering Society General Meeting (PES, 1–6. doi:10.1109/PES.2007.386249
Liu, X., Wang, P., and Loh, P. C. (2011). A hybrid AC/DC microgrid and its coordination control. IEEE Trans. Smart Grid 2 (2), 278–286. doi:10.1109/TSG.2011.2116162
Lotfi, H., and Khodaei, A. (2017). AC versus DC microgrid planning. IEEE Trans. Smart Grid 8 (1), 296–304. doi:10.1109/TSG.2015.2457910
Mahmoud, M. S., Azher Hussain, S., and Abido, M. A. (2014). Modeling and control of microgrid: An overview. Journal of the Franklin Institute, 2822–2859. doi:10.1016/j.jfranklin.2014.01.016
Manias, S. N. (2017). “Inverters (DC - AC converters),” in Power electronics and motor drive systems. doi:10.1016/B978-0-12-811798-9.00006-8
Mariam, L., Basu, M., and Conlon, M. F. (2016). Microgrid: Architecture, policy and future trends. Renew. Sustain. Energy Rev. 64, 477–489. doi:10.1016/j.rser.2016.06.037
Mashayekh, S., Stadler, M., Cardoso, G., and Heleno, M. (2017). A mixed integer linear programming approach for optimal DER portfolio, sizing, and placement in multi-energy microgrids. Appl. Energy 187, 154–168. doi:10.1016/j.apenergy.2016.11.020
Mayer, J. N., Simon, P., Philipps, N. S. H., Schlegl, T., and Senkpiel, C. (2015). Current and future cost of photovoltaics long-term scenarios for market development. 82.
Mcloughlin, F., Duffy, A., and Conlon, M. (2015). A clustering approach to domestic electricity load profile characterisation using smart metering data. Appl. Energy 141, 190–199. doi:10.1016/j.apenergy.2014.12.039
Meng, L., Sanseverino, E. R., Luna, A., Dragicevic, T., Vasquez, J. C., and Guerrero, J. M. (2016). Microgrid supervisory controllers and energy management systems: A literature review. Renew. Sustain. Energy Rev. 60, 1263–1273. doi:10.1016/j.rser.2016.03.003
Mingsheng, Z., Peilei, F., Hesong, W., Wenkui, W., and Pengcheng, C. (2018). “Hierarchical control strategy for microgrid,” in Proceedings of 2018 2nd IEEE Advanced Information Management, Communicates, Electronic and Automation Control Conference IMCEC, 1528–1532. doi:10.1109/IMCEC.2018.8469405
Miveh, M. R., Gandomkar, M., Mirsaeidi, S., Gharibdoost, M. R., et al. (2012). “A review on protection challenges in microgrids,” in 2012 Proceedings of 17th Conference on Electrical Power Distribution, EPDC, 1–5.
Moncecchi, M., Brivio, C., Corigliano, S., Cortazzi, A., and Merlo, M. (2018). “Battery modeling for microgrid design: A comparison between lithium-ion and lead acid technologies,” in SPEEDAM 2018 - Proceedings: International Symposium on Power Electronics, Electrical Drives, Automation and Motion, 1215–1220. doi:10.1109/SPEEDAM.2018.8445343
Moran, B. (2016). “Microgrid load management and control strategies,” in Proceedings of the IEEE Power Engineering Society Transmission and Distribution Conference, 2016-July, 1–4. doi:10.1109/TDC.2016.7520025
Morgan, E. R., Valentine, S., Blomberg, C. A., Limpaecher, E. R., and Dydek, E. V. (2016). Boston community energy study – zonal analysis for urban microgrids. Massachusetts Institute of Technology Lincoln Laboratory.
Moyano, C. F., Ledwich, G., and Robinson, O. E. (2010). Load modeling in power systems: Induction motors. Electric Power Systems Transition. Nova Science Publishers, Inc., 75–136.
Narayanan, V., Seema, S., and Singh, B. (2018). “Solar PV - BES based microgrid system with multifunctional VSC,” in 2018 5th IEEE Uttar Pradesh Section International Conference on Electrical, Electronics and Computer Engineering, UPCON, 1–6. doi:10.1109/UPCON.2018.8597002
Nasir, M., Khan, H. A., Hussain, A., Mateen, L., and Zaffar, N. A. (2018). Solar PV-based scalable DC microgrid for rural electrification in developing regions. IEEE Trans. Sustain. Energy 9 (1), 390–399. doi:10.1109/TSTE.2017.2736160
Neto, P. J. D. S., Barros, T. A. d. S., Silveira, J. P. C., Filho, E. R., Vasquez, J. C., and Guerrero, J. M. (2020). Power management strategy based on virtual inertia for DC microgrids. IEEE Trans. Power Electron. 35 (11), 12472–12485. doi:10.1109/TPEL.2020.2986283
Nguyen, X. H., and Nguyen, M. P. (2015). Mathematical modeling of photovoltaic cell/module/arrays with tags in Matlab/Simulink. Environ. Syst. Res. (Heidelb). 4 (1), 24. doi:10.1186/s40068-015-0047-9
Nomm, J., Ronnberg, S., and Bollen, M. (2018). “Harmonic voltage measurements in a single house microgrid,” in Proceedings of International Conference on Harmonics and Quality of Power, ICHQP, 1–5. doi:10.1109/ICHQP.2018.8378921
Onduction, I. I. C., and Vsi, M. O. D. E. (2015). Implementation of three phase 12-step Vsi with harmonics reduction. Int. J. Adv. Eng. Res. Dev. 3 (02), 5–10. doi:10.21090/ijaerd.ncrretee01
Oyarzabal, J., Jimeno, J., Ruela, J., Engler, A., Hardt, C., et al. (2002). Agent based micro grid management system, 1–6.
Palaniappan, K., Veerapeneni, S., Cuzner, R., and Zhao, Y. (2017). “Assessment of the feasibility of interconnected smart DC homes in a DC microgrid to reduce utility costs of low income households,” in 2017 IEEE 2nd International Conference on Direct Current Microgrids, ICDCM, 467–473. doi:10.1109/ICDCM.2017.8001087
Palizban, O., and Kauhaniemi, K. (2015). Hierarchical control structure in microgrids with distributed generation: Island and grid-connected mode. Renew. Sustain. Energy Rev. 44, 797–813. doi:10.1016/j.rser.2015.01.008
Peng, J., Fan, B., Yang, Q., and Liu, W. (2022). Fully distributed discrete-time control of DC microgrids with ZIP loads. IEEE Syst. J. 16 (1), 155–165. doi:10.1109/JSYST.2020.3038664
Perera, B. K., Ciufo, P., and Perera, S. (2013). “Point of common coupling ( PCC ) voltage control of a grid-connected solar photovoltaic ( PV ) system,” in IECON 2013 - 39th Annual Conference of the IEEE Industrial Electronics Society, 7475–7480. doi:10.1109/IECON.2013.6700377
Phurailatpam, C., Rajpurohit, B. S., and Pindoriya, N. M. (2011). Embracing microgrids: Applications for rural and urban India, 1–10.
Planas, E., Gil-de-Muro, A., Andreu, J., Kortabarria, I., and Martinez de Alegria, I. (2013). General aspects, hierarchical controls and droop methods in microgrids: A review. Renew. Sustain. Energy Rev. 17, 147–159. doi:10.1016/j.rser.2012.09.032
Porsinger, T., Janik, P., Leonowicz, Z., and Gono, R. (2017). Modelling and optimization in microgrids. Energies 10 (4), 523–622. doi:10.3390/en10040523
Pourbabak, H., Chen, T., and Zhang, B. (2017). “Control and energy management system in microgrids,” in Clean energy microgrids.
Prakash, R., and Singh, S. (2016). Des. Model. Sol. Photovolt. Cell. Array 11 (2), 35–40. doi:10.9790/1676-1102033540
Qazi, S. (2017). “PV systems affordability, community solar, and solar microgrids,” in Standalone photovoltaic (PV) systems for disaster relief and remote areas, 177–202. doi:10.1016/B978-0-12-803022-6.00006-X
Raviteja, P., Yadagiri, A., and Jithendar, P. (2021). “Power management scheme for wind-PV-battery-based hybrid micro grids for power control,” in Proceedings of the 5th International Conference on Electronics, Communication and Aerospace Technology, ICECA, 245–251. doi:10.1109/ICECA52323.2021.9675923
Reed, G. F., Grainger, B. M., Sparacino, A. R., Mao, Z. H., et al. (2012). Ship to grid. IEEE Power and Energy Magazine, 70–79.
Siwakoti, Y. P., Forouzesh, M., and Pham, N. H. (2018). “Power electronics converters — an overview,” in Control of power electronic converters and systems (Elsevier), 3–29. doi:10.1016/B978-0-12-805245-7.00001-9
Salomonsson, D., Söder, L., and Sannino, A. (2009). Protection of low-voltage DC microgrids. IEEE Trans. Power Deliv. 24 (3), 1045–1053. doi:10.1109/TPWRD.2009.2016622
Sampath, K. V., Prasad, J., and Samikannu, R. (2018). Barriers to implementation of smart grids and virtual power plant in sub-saharan region — Focus Botswana. Energy Rep. 4, 119–128. doi:10.1016/j.egyr.2018.02.001
Santos, L. H. S., Silva, J. A. A., Lopez, J. C., Arias, N. B., Rider, M. J., Da Silva, L. C. P., et al. (2021). “Integrated optimal sizing and dispatch strategy for microgrids using HOMER Pro,” in 2021 IEEE PES Innovative Smart Grid Technologies Conference - Latin America, ISGT Latin America. doi:10.1109/ISGTLatinAmerica52371.2021.9543015
Sarwar, A., and Asghar, M. S. J. (2011). Simulation and analysis of a multilevel converter topology for solar PV based grid connected inverter. Smart Grid and Renewable Ene, 56–62. doi:10.4236/sgre.2011.21007
Saxena, N., Hussain, I., Singh, B., and Vyas, A. L. (2017). “Solar PV interfaced to multifunctional VSC with a battery support,” in 2016 IEEE 7th Power India International Conference, PIICON, 1–6. doi:10.1109/POWERI.2016.8077415
Shayanfar, H. A., and Malek, S. (2015). “Photovoltaic microgrids control by the cooperative control of multi-agent systems,” in 30th Power System Conference (PSC2015), Niroo Research Institute, Tehran, Iran, 23-25 November 2015, 23–25.
Shintre, P., and Mulla, A. M. (2016). Study of micro grid topology and design of voltage source inverter and charge controller. Int. Res. J. Eng. Technol. (IRJET) 3 (3).
Shrivastwa, R. R., Hably, A., Melizi, K., and Bacha, S. (2019). “Understanding microgrids and their future trends,” in Proceedings of the IEEE International Conference on Industrial Technology, 2019-February, 1723–1728. doi:10.1109/ICIT.2019.8754952
Shuai, Z., Fang, J., Ning, F., and Shen, Z. J. (2018). Hierarchical structure and bus voltage control of DC microgrid. Renew. Sustain. Energy Rev. 82 (2), 3670–3682. doi:10.1016/j.rser.2017.10.096
Shufian, A., and Mohammad, N. (2022). Modeling and analysis of cost-effective energy management for integrated microgrids. Clean. Eng. Technol. 8, 100508. doi:10.1016/j.clet.2022.100508
Silva, F. S. F., Luiz, L. A., and De Matos, J. G. (2014). “Bidirectional DC-AC converter for isolated microgrids with voltage unbalance reduction capabilities,” in 2014 IEEE Energy Conversion Congress and Exposition, ECCE, 4985–4991. doi:10.1109/ECCE.2014.6954085
Sivarasu, S. R., Chandira Sekaran, E., and Karthik, P. (2015). Development of renewable energy based microgrid project implementations for residential consumers in India: Scope, challenges and possibilities. Renewable and Sustainable Energy Reviews, 256–269. doi:10.1016/j.rser.2015.04.118
Soni, U. S., and Talwekar, R. H. (2018). “Control strategies in droop controller of MicroGrid: A survey,” in Proceedings - 2018 International Conference on Smart Electric Drives and Power System, ICSEDPS, 239–244. doi:10.1109/ICSEDPS.2018.8536054
Soon, J. J., and Low, K. S. (2015). Optimizing photovoltaic model for different cell technologies using a generalized multidimension diode model. IEEE Trans. Ind. Electron. 62 (10), 6371–6380. doi:10.1109/TIE.2015.2420617
Tang, Y., Zhu, L., Ning, J., and Wang, Q. (2019). A data-driven approach for online aggregated load modeling through intelligent terminals. Int. J. Distributed Sens. Netw. 15 (1), 155014771982599. doi:10.1177/1550147719825996
Tayab, U. B., Roslan, M. A. B., Hwai, L. J., and Kashif, M. (2017). A review of droop control techniques for microgrid. Renew. Sustain. Energy Rev. 76, 717–727. doi:10.1016/j.rser.2017.03.028
Tegling, E., Andreasson, M., Simpson-Porco, J. W., and Sandberg, H. (2016). “Improving performance of droop-controlled microgrids through distributed PI-control,” in Proceedings of the American Control Conference, 2016-July, 2321–2327. doi:10.1109/ACC.2016.7525264
Tremblay, O., Dessaint, L. A., and Dekkiche, A. I. (2007). “A generic battery model for the dynamic simulation of hybrid electric vehicles,” in VPPC 2007 - Proceedings of the 2007 IEEE Vehicle Power and Propulsion Conference, 284–289. doi:10.1109/VPPC.2007.4544139
Tungadio, D. H., Bansal, R. C., Siti, M. W., and Mbungu, N. T. (2018). Predictive active power control of two interconnected microgrids. Technol. Econ. Smart Grids Sustain. Energy 3 (1), 3–15. doi:10.1007/s40866-018-0040-2
Unruh, P., Nuschke, M., StrauB, P., and Welck, F. (2020). Overview on grid-forming inverter control methods. Energies 13 (10), 2589. doi:10.3390/en13102589
USDOE (2012). Summary report: 2012 DOE microgrid workshop, U.S. Department of energy. Available at: http://energy.gov/sites/prod/files/2012 Microgrid Workshop Report 09102012.pdf.
Ustun, T. S., Ozansoy, C., and Zayegh, A. (2011). Recent developments in microgrids and example cases around the world - a review. Renew. Sustain. Energy Rev. 15 (8), 4030–4041. doi:10.1016/j.rser.2011.07.033
Vazquez, N., Gallegos, C., Hernandez, C., Rodriguez, E., and Cervantes, I. (2012). “Parallel DC/ac converters under connection/disconnection of power modules,” in Proceedings of the 6th Andean Region International Conference, Andescon, 21–24. doi:10.1109/Andescon.2012.15
Vetter, M., and Rohr, L. (2014). “Lithium-ion batteries for storage of renewable energies and electric grid backup,” in Lithium-ion batteries advances and applications (Elsevier), 293–309. doi:10.1016/B978-0-444-59513-3.00013-3
Vignesh, S. S., and Sundaramoorthy, R. S. (2016). The combined V-F , P-Q and Droop control of PV in microgrid. Int. J. Res. Appl. Sci. Eng. Technol. (IJRASET) 4, 989–994.
Villalva, M. G., Gazoli, J. R., and Filho, E. R. (2009). Comprehensive approach to modeling and simulation of photovoltaic arrays. IEEE Trans. Power Electron. 24 (5), 1198–1208. doi:10.1109/tpel.2009.2013862
Wang, F., Zhu, Y., and Yan, J. (2018). Performance of solar PV micro-grid systems: A comparison study. Energy Procedia 145, 570–575. doi:10.1016/j.egypro.2018.04.083
Wang, X., Ji, Y., Wang, J., Wang, Y., and Qi, L. (2020). Optimal energy management of microgrid based on multi-parameter dynamic programming. Int. J. Distributed Sens. Netw. 16 (6), 155014772093714. doi:10.1177/1550147720937141
Wanitschke, A., Pieniak, N., and Schaller, F. (2017). Economic and environmental cost of self-sufficiency-analysis of an urban micro grid. Energy Procedia 135, 445–451. doi:10.1016/j.egypro.2017.09.510
Wibowo, R. S., Firmansyah, K. R., Aryani, N. K., and Soeprijanto, A. (2017). “Dynamic economic dispatch of hybrid microgrid with energy storage using quadratic programming,” in IEEE Region 10 Annual International Conference, Proceedings/TENCON, 667–670. doi:10.1109/TENCON.2016.7848086
Xia, T., Li, M., Zi, P., Tian, L., Qin, X., An, N., et al. (2015). “Modeling and simulation of battery energy storage system (BESS) used in power system,” in 2015 5th International Conference on Electric Utility Deregulation and Restructuring and Power Technologies (DRPT), 2120–2125. doi:10.1109/DRPT.2015.7432597
Yadav, G., and Jaladi, K. K. (2018). “Comparison of different parameters using Single Diode and Double Diode model of PV module in a PV-Battery system using MATLAB Simulink,” in 2017 14th IEEE India Council International Conference, INDICON. doi:10.1109/INDICON.2017.8487608
Yamashita, K., Djokic, S., Matevosyan, J., Resende, F., Korunovic, L., Dong, Z., et al. (2012). Modelling and aggregation of loads in flexible power networks – scope and status of the work of CIGRE WG C4.605. IFAC Proc. Vol. (IFAC-PapersOnline) 8, 405–410. doi:10.3182/20120902-4-fr-2032.00072
Yang, Y., Kim, K. A., Blaabjerg, F., and Sangwongwanich, A. (2019). “PV system modeling, monitoring, and diagnosis,” in Advances in grid-connected photovolatic power conversion systems, 45–71. doi:10.1016/B978-0-08-102339-6.00003-8
Yekini, M., Mustafa, M. W., Bashir, N., Muhamad, N. A., and Mokhtar, A. S. (2013). Power sector renewable energy integration for expanding access to electricity in sub-Saharan Africa. Renew. Sustain. Energy Rev. 25, 630–642. doi:10.1016/j.rser.2013.04.033
Zeng, P., Li, H., He, H., and Li, S. (2019). Dynamic energy management of a microgrid using approximate dynamic programming and deep recurrent neural network learning. IEEE Trans. Smart Grid 10 (4), 4435–4445. doi:10.1109/TSG.2018.2859821
Zhang, C., Wei, Y. L., Cao, P. F., and Lin, M. C. (2018). “A review of control strategies in DC microgrid A review of control strategies in DC microgrid,” in First International Conference on Advanced Algorithms and Control Engineering, 1–9.
Zhao, B., Tang, Y., Zhang, W. C., and Wang, Q. (2010). “Modeling of common load components in power system based on dynamic simulation experiments,” in 2010 International Conference on Power System Technology: Technological Innovations Making Power Grid Smarter, POWERCON. doi:10.1109/POWERCON.2010.5666712
Keywords: solar PV systems, microgrid, hierarchical control, total harmonic distortion, load modeling
Citation: Seane TB, Samikannu R and Bader T (2022) A review of modeling and simulation tools for microgrids based on solar photovoltaics. Front. Energy Res. 10:772561. doi: 10.3389/fenrg.2022.772561
Received: 08 September 2021; Accepted: 07 September 2022;
Published: 29 September 2022.
Edited by:
K. Sudhakar, Universiti Malaysia Pahang, MalaysiaReviewed by:
Antonio Scala, National Research Council (CNR), ItalyAjith Gopi, Agency for New and Renewable Energy Research and Technology, India
Copyright © 2022 Seane, Samikannu and Bader. This is an open-access article distributed under the terms of the Creative Commons Attribution License (CC BY). The use, distribution or reproduction in other forums is permitted, provided the original author(s) and the copyright owner(s) are credited and that the original publication in this journal is cited, in accordance with accepted academic practice. No use, distribution or reproduction is permitted which does not comply with these terms.
*Correspondence: T. B. Seane, dHVtZWxvLnNlYW5lQHN0dWRlbnRtYWlsLmJpdXN0LmFjLmJ3