- School of Energy Science and Engineering, Central South University, Changsha, China
Effective carbon dioxide (CO2) capture plays indispensable roles in closing the global carbon cycle, serving the sustainable production of energy, and achieving the grand 1.5 °C goal by 2050. Considering the diversity and complexity of CO2 capture materials, machine learning has stepped into this field years ago and become a powerful tool that promotes the screening and design of involving parameters. From these perspectives, this critical review firstly summarizes the technical backgrounds for the applications of ML-based methods in CO2 capture. Then, through categorizing the materials into two major groups, that is, adsorbents (containing metal organic frameworks, carbonaceous materials, polymers, and zeolites) and absorbents (involving ionic liquids, amine-based absorbents, and deep eutectic solvents), the applications of this effective tool in relevant areas are scrutinized. The major concerns remain to be further addressed are derived based on the above discussions, namely 1) the development of consistent and integrated databases, 2) the wise digitalization of inherent properties of materials, and 3) the validation of the accuracy of ML-derived results under practical scenarios. The main purpose of this critical review is bridging the previous achievements and further developments of ML-assisted design of CO2 capture techniques.
Introduction
Fighting against climate change, with emphasis on the over-accumulated issue of carbon dioxide (CO2) in the air, is one of the most predominant challenges facing carbon-intensive energy industries and the environmental community in the 21st century (Guan et al., 2022). Compared to preindustrial times before the 1750 s, the CO2 concentration in the troposphere has increased from ∼280 ppm to ∼400 ppm, with an annual increase of approximately 1 ppm (Pera-Titus 2014; Oschatz and Antonietti 2018). Notably, this increasing trend is accelerating in the past decades, with the rate boosting from ∼1.1% in the 1990 s to ∼3.0% in the 2000 s. The over-accumulated CO2 in the air is escorted by the rise of Earth’s surface temperature by 0.6–0.7 C (Pera-Titus 2014). Although future trends are difficult to be specified due to unexpected geological activities, the Paris Climate Agreement identifies that, to achieve the grand 1.5°C goal, a net-zero emission of CO2 must be realized by 2050, because even assuming a preferred scenario, the CO2 will exceed 550 ppm until then (Wang et al., 2011). Besides, by converting it back to energy storage materials (Wu et al., 2021), adopting it in extracting residual oils from aquifers (Chen et al., 2022), etc., the centralized control of CO2 also serves the overall benefits of energy production in a depleting era of fossil fuels. From these perspectives, it is of great urgency to develop advanced and effective techniques for CO2 capture and collection from industrial flue gas and ambient environment.
The up-to-date applications of CO2 capture technologies generally involve an absorption process, with the addition of amine-based solution like monoethanolamine (MEA), diethanolamine (DEA), or methyl-diethanolamine (MDEA), to convert CO2 into carbonate solids (Ghanbari et al., 2020). However, the low selectivity of traditional amine-based absorbents towards CO2 capture remains yet a critical issue impeding the closing of carbon-cycle and the achievement of net-zero CO2 emission (Haider and Kumar, 2020). Specifically and firstly, for CO2 capture from industrial flue gas, the limited selectivity makes traditional amine-based absorbents efficient for the capture of components other than CO2, e.g., sulfur dioxide (SO2) and nitrogen oxides (NOx), thus the separation of carbon-laden energy storage products becomes relatively time-consuming and expensive that requires extra steps (Rezaei and Jones 2014). Secondly, for the direct CO2 capture (DAC) from ambient environment, a critical technique to achieve the net-zero emission of CO2, using amine-based absorbents, if not being purposefully functionalized, is generally unpractical (Barzagli et al., 2020). The advancements in CO2 capture with more functional agents exhibiting higher selectivity thus still hinder the world to be on the right track towards forming a batch of available technologies applicable under all possible scenarios.
To realize a highly selective CO2 capture from industrial flue gas or ambient environment, advanced materials including modified amine absorbents (Pakzad et al., 2020; Gaikwad et al., 2021; Li et al., 2021; Mehrabi et al., 2022), MOFs (Ding et al., 2019), metal salts (Hu et al., 2019; Hu et al., 2021), zeolites (Khoramzadeh et al., 2019; Bakhtyari et al., 2020), deep eutectic solvents (Ali et al., 2016; Zarei et al., 2020), etc., have been proposed to be alternatives to traditional CO2 capture agents. The adjustment of physical and chemical properties of absorbents or adsorbents is the most crucial process to make them purposefully functionalized (Rahimi et al., 2021). However, considering the complex composition of the properties that can be designed, experimental methods based on a trial-and-error logic are extremely time-consuming and hardly leverage all key properties. To overcome this research gap and identify more selective agents for CO2 separation, machine learning (ML) has been emerging as an efficient way recently, because it can screen tens of thousands of materials with a variety of physical and chemical properties being adjustable (Fernandez et al., 2014; Anderson et al., 2018; Zhu et al., 2020; Zhang et al., 2021a).
From the abovementioned perspectives, the prediction of the physical and chemical properties of CO2 sorbents is a fundamental towards the design of functionalized CO2 sorbents. Fortunately, using ML-based methods to characterize the inherent properties like density (Abdollahzadeh et al., 2022), viscosity (Bakhtyari et al., 2022), and pore structure (Jablonka et al., 2020) of materials has been in a developed stage. With this advancement, the foreseeable wide application of ML methods in CO2 capture is expected to bring about revolutionary changes that extend the material reservoir, boost the sorption capacity, increase the CO2 selectivity, and reduce the operation cost. While most previous works focused on the CO2 uptake capacity, the CO2 selectivity, another critical factor influencing the applicability of CO2 capture techniques, is much less investigated and clarified, representing the bottleneck at the current stage. Considering the effectiveness of ML in identifying and proposing highly selective CO2 ab-/adsorbents, the use of this method innovatively fuels the break of this bottleneck, but well-constructed framework bridging its previous achievements and further development in this specific field is still lacking (Zhou K. et al., 2019).
Correspondingly, this review aims to prioritize the role of selectivity in CO2 separation from other gas components, if applicable, or discuss the future endeavors towards such a sophisticated end. It firstly and briefly introduces the technical backgrounds of ML methods. The technical backgrounds primarily include four sub-sections, that is, the collection of data, the featurization of descriptors, the evaluation of algorithms, and the training of models and the prediction of performances. Then, the application of ML methods in CO2 capture was summarized based on different kinds of materials, which can be primarily divided into adsorbents and absorbents. Adsorbents take advantage of the abundant pores, large surface area and other properties to capture CO2, including metal organic frameworks (MOF), carbonaceous materials, polymers, and zeolites. Absorbents capture CO2 by chemically reacting with it, including amine-based absorbents and ion-liquid based materials. Finally, the critical research gaps and future development of ML methods in related areas are identified, aiming to provide a dedicatedly constructed framework guiding the effective use of ML techniques in mitigating climate change and saving energy penalty before they step towards losing control.
Technical backgrounds
Since its appearance in the 19th century, ML has been rapidly evolving and widely used in the identification and proposal of novel materials (Lu et al., 2017; Ramprasad et al., 2017; Wang et al., 2022a; Zhang X. et al., 2022). The intelligent nature of ML methods makes it not only an efficient tool to preprocess and analyze data, but also an advanced way to learn and recognize patterns. After the sorption behaviors of CO2 on a designated group of materials are determined using appropriate ML approaches, the CO2 separation performances of ab-/adsorbents that have not been experimentally tested can be predicted based on this recognized pattern. Generally, the following steps represent a typical roadmap to process the ML-based design of CO2 capture materials, that is, the collection of data, the featurization of descriptors, the evaluation of algorithms, the training of models, and the prediction of performances (Chen et al., 2020) (as briefly illustrated by Figure 1).
The collection of data. Firstly, for a ML-based task, sufficient quantity and satisfactory quality of data, which can be collected from published literatures or self-generated through theoretical calculation or experimental test (Butler et al., 2018), are the most critical factors influencing its feasibility. With approximately 40,000 referred results if using ‘CO2 capture’ and ‘CO2 separation’ as keywords for searching on the Web of Science, it is totally possible for ML-based studies in related areas to collect data from previous works. While the consistency of the collected data is difficult to be well-controlled, e.g., different works conducting the CO2 capture experiments under varied conditions that may significantly change the performances, ML may be an effective method to exclude the impacts of these external factors, or even to identify the patterns of how these factors influence the CO2 capture efficiency. In this case, these external factors must be taken as necessary descriptors and participate in the model training process. By processing importance analysis, the model tells users whether these external factors play crucial or marginal roles in the CO2 separation process (Guan et al., 2022). If a crucial role is identified, the specific effects of experimental conditions can be investigated in detail via ML-based approaches, and if a marginal role is found, the experimental conditions can be generally excluded in the subsequent prediction of CO2 capture performances. However, as the primary goal of most ML-based explorations is examining potential materials instead of practical operation conditions, self-generated data may be preferred to ensure their integrity and consistency. The Grand Canonical Monte Carlo (GCMC) method based on molecular dynamics with the assistance of density functional theory (DFT) calculation is a common roadmap to this end. This method is generally applicable in the cases where porous materials that physiosorbed CO2, e.g., MOF, are adopted (Dureckova et al., 2019). Besides, databases like Materials Project (Jain et al., 2013), Cambridge Structural Database (Groom and Allen 2014), Crystal Open Database (Gražulis et al., 2009), etc., that contain tens of thousands of known materials with necessary properties specified, which is still unceasingly updating, also build an indispensable bridge for the self-generation of data.
The featurization of descriptors. The featurization of descriptors refers to a process where essential properties involved in CO2 capture are transferred into numerical values (called descriptors) that are readable for computer. Generally, the essential properties can be categorized into two subsets, that is, external properties of experiments (e.g., the operation temperature/pressure, the concentration of CO2, the dosage of ab-/adsorbents, etc.) and intrinsic properties of materials including their apparent compositions (e.g., elemental composition, the ratio between active adsorbents and inert supporters, etc.), physical characteristics (e.g., pore size, surface area, etc.), and chemical characteristics (e.g., the interaction pattern among different atoms, the charge of active sites, the d-band center of active sites, etc.) (Ghiasi et al., 2019; Burns et al., 2020; Shi et al., 2020; Gupta and Li 2022; Situ et al., 2022). The selection of properties is a subtle but critical task that directly determines the performance of modeling. The involved properties need to fully represent a group of possible influential factors affecting the CO2 capture performance, while redundant inclusion of properties may cause deviation and overfitting. For example, the surface area and pore volume of a material is generally interconnected, thus the inclusion of both these two properties contain redundant information that may result in overfitting. Although this overfitting concern may be properly addressed with the use of advanced algorithms, it is better to exclude excessively interconnective data when featuring descriptors. Besides, it should be noted that, while part of the abovementioned features are numerical values in nature (e.g., the operation temperature/pressure, the pore size, and surface area), other features like the interactive pattern among different atoms may be unreadable characteristics for computers in their initial forms. For these properties, how to efficiently transfer them into numerical value is still an open question nowadays. Common methods include the use of topologically repeating units, matrix, and/or function to represent the complex situation (Behler and Parrinello 2007; Huang and von Lilienfeld 2016; Barnett et al., 2020). In addition, there are numerically-in-nature properties may proportionally correlate with those that are difficult to be digitalized. A typical example is that the interactive pattern among different atoms may be correlated with the electronegativity of these atoms, hence users can replace the hardly-digitalized characteristics by numerically-in-nature features if their interconnective pattern is recognized to improve the efficiency and avoid overfitting.
The evaluation of algorithms. There are generally three kinds of ML - supervised learning, unsupervised learning, and reinforcement learning (Kargbo et al., 2021). Supervised learning refers to a ML scenario where both the input descriptors and output values in the training set are given, and the purpose of learning is correlating the input and output data with relatively complex laws (Tabor et al., 2018). As for unsupervised learning, unlabeled input descriptors were involved, that is, the output values remaining unclarified in this case, and the primary goal of learning in this case is recognize the patterns of data (Chen et al., 2015). Finally, reinforcement learning is a method that computer mimics the behaviors of human and improves its own ability in the learning process (Podryabinkin and Shapeev 2017). For CO2 capture, supervised learning is the most common technique used in previous studies, and it contains different algorithms, for which the performance is case-dependent. The involved algorithms for exploring the CO2 separation performances of different materials mainly include multiple linear regression (MLR), genetic algorithms (GA), support vector machine (SVM), decision tree (DT), random forest (RF), and neural network (NN) (with the advantages and disadvantages of each algorithm specified in Figure 2).
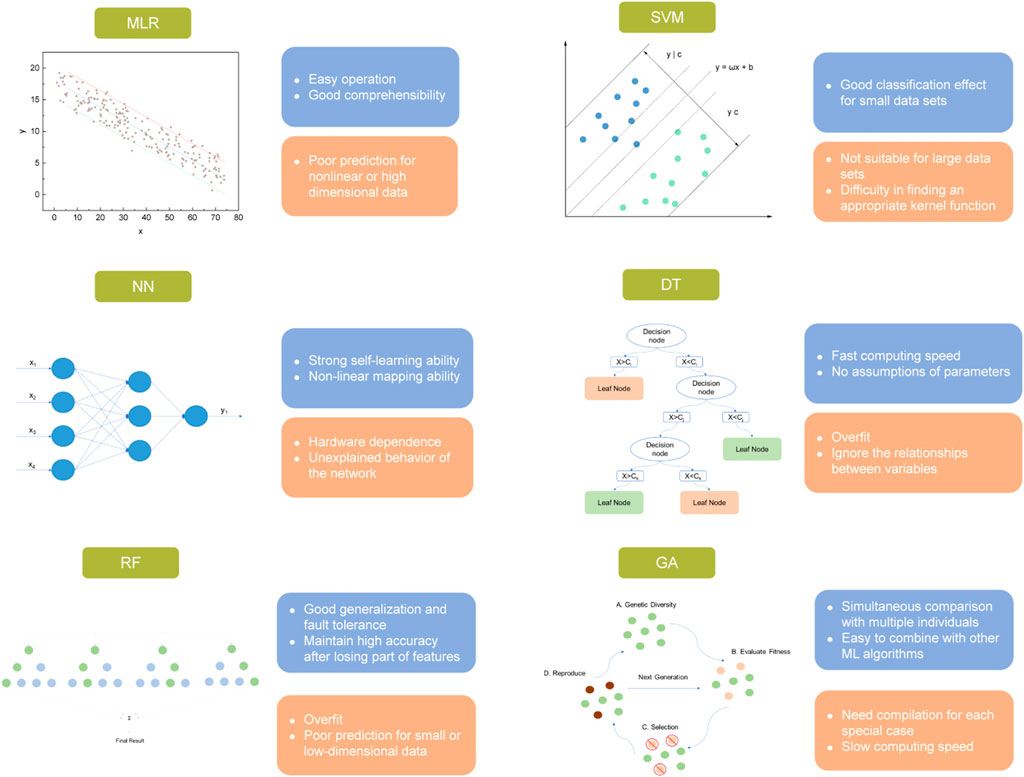
FIGURE 2. Primary ML algorithms used in CO2 separation and their advantages (blue frame) and disadvantages (orange frame).
For effective CO2 separation, the properties of the materials and experiments might be used as the input features, and the output values were CO2 capacity, capture efficiency, and/or selectivity. Although the selectivity is the most concerned issue in this review, the CO2 capacity and capture efficiency are non-neglectable in most cases as they are the foundations for an exceptional selectivity. To judge the accuracy of ML simulations, loss functions are adopted with the assistance of correlation coefficient (R2), mean absolute error (MAE), mean square error (MSE), and root mean square error (RMSE) (Doreswamy et al., 2020). For example, a RMSE value higher than 0.80 is generally taken as a criterion to justify the applicability of a ML algorithm, and that higher than 0.90 indicates an excellent accuracy. Algorithms with higher accuracy was selected for a large-scale simulation, while inherent reasons accounting for such an empirical superiority may be complicated and unknown.
The training of models and prediction of performances. After the algorithms are optimized, it is the time to process ML learning and find the intrinsic correlations between input properties and output values. The collected data are randomly separated into two different groups namely training set and testing set in the training process of models (the size ratio between the training and testing sets generally ranges between 75%:25%–85%:15%), aiming to verify the generalization of the applied algorithms. Multiple cross validation may be in further need if the data size is relatively small (Lu et al., 2017). When, typically, RMSEs of the training and testing processes larger than 0.90 and 0.80 are obtained, it demonstrates that the descriptors adopted and the model used are suitable for the further prediction of CO2 separation performances of untested materials (Barnett et al., 2020). The prediction only requires the input of unlabeled data with specified features and unknown performance. Descriptors that are found to have negligible influence on the performance based on the importance analysis results can be excluded from the input set in the performance prediction, if preferred. It is thus an efficient way to exclude the impact of external factors and investigate the influence of intrinsic characteristics of materials even no consensus is reached among experimenters to provide data generated under a consistent condition. A specific criterion is expected to judge the suitability and applicability of CO2 ab-/adsorbents under practical scenarios, which generally involves the participation of the following parameters, that is, the CO2 capacity, the CO2 selectivity over other gas components, and the plot of CO2 permeability versus its selectivity when a membrane is used (as shown in Figure 3) (Barnett et al., 2020). Tens of thousands of materials that have not been experimentally synthesized and tested can be screened in this process with a relatively high accuracy. Only the preferential materials as indicated by the ML modeling might be synthesized in some cases to further support the validation of the simulation (Zhang Z. et al., 2022), thus significantly saving the time of conducting time- and labor-consuming tests.
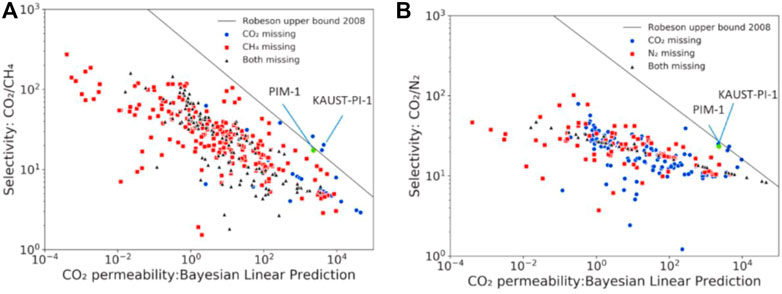
FIGURE 3. The plot of CO2 permeability versus its selectivity over (A) CH4 and (B) N2 of (Yuan Q. et al., 2021).
Application of ML in CO2 separation
The ML based screening and design of CO2 separation ab-/adsorbents leverage its function in the case where complicated material properties or process characteristics remain to be adjusted. According to the function of materials, they are categorized into two major parts namely adsorbents, including metal organic frameworks (MOF), carbonaceous materials, polymers, and zeolites, and absorbents, including ionic liquids (IL), amine-based materials, and deep eutectic solvents (DES) (as shown in Figure 4). It should be noted that the primary purpose of this critical review is to explore the fundamentals and applications of the ML-assisted methods in designing CO2 capture materials, but not to give a comprehensive introduction to all available materials/techniques nowadays.
Adsorbents
Metal organic frameworks (MOF). MOFs are one of the most explored groups for CO2 capture based on ML-based methods. Critical reasons accounting for such popularity include 1) wide interests - the huge reservoir, theoretically infinite, of available MOFs comprised of profoundly adjustable parameters, 2) data availability - there are several databases like Cambridge Crystallographic Data Centre (CCDC) and Computation-Ready, Experimental (CoRE) containing sufficient data of experimentally synthesized and hypothesized MOFs, and 3) time and labor-saving technical roadmap - compared with experimentally testing the CO2 adsorption capacity of MOF, computational methods (e.g., GCMC) generally spend much less time and labor costs to finish the same job. As the key influential factors affecting the physisorption, the pore sizes, surface areas, and void fractions of MOFs, experimentally accessible or derived from theoretical calculation with designated software (e.g., Zeo++), are generally included in the training of ML models. Besides, the chemical properties of MOFs are also important for the CO2 capture, including the heat of adsorption, the atomic weight ratio of different elements, the types of metal nodes, etc. Although the digitalization of the chemical properties of MOFs is generally more complex than that of the physical properties, a recent work employed a GCN method to digitalize MOF structure and obtain its chemical features efficiently (Wang et al., 2022b).
The application of ML in MOF-assisted CO2 capture dates back to 2014 when its use was for the first time reported in related areas (Fernandez et al., 2014). The SVM ML model was adopted to screen and recognize the CO2 adsorption patterns and behaviors over more than 32,000 types of MOFs. Specific criteria were set to select the outperforming MOFs out under different operational pressures, that is, MOFs with capacities higher than 1 mmol g−1 at 0.15 bar and 4 mmol g−1 at 1.0 bar were identified as superior MOFs for CO2 capture. Based on these criteria, a critical interatomic distance in the compact framework ranging between 6 and 9 Å was found to be optimal for CO2 capture. An inferior interatomic distance compromised the adsorption performance probably attributed to the incapability to accommodate CO2, while an excessive distance might allow CO2 to bypass the adsorbents instead. In 2016, the same research group considered more than one kind of gas components and for the first time investigated the selectivity towards CO2 over CH4 of MOFs based on the DT and SVM ML models (Aghaji et al., 2016). It was also found that, due to a similar reason as abovementioned, moderate surface area, void fraction, and pore diameter are both important to enhance the selectivity of MOFs towards CO2 over CH4. A void fraction lower than 0.27 and a pore diameter lower than 6.6 Å were identified to be two critical thresholds to achieve a CO2/CH4 selectivity of >10. However, these two valuable works, despite shedding the initial light on the application of ML in CO2 capture based on MOFs, are deficient in nature due to the omission of their chemical properties.
To overcome such deficiency, the chemical features of MOFs were originally considered in 2018 with the assistance of a topological method (Anderson et al., 2018). The Coulomb charge of MOFs, the most positive and most negative charges in functional groups, the highest bond dipole moment of MOFs, and the topology of functional groups are involved in the model training (as shown in Figure 5A). Before introducing a crucial chemical descriptor, i.e., the topology of functional group, into the system, the authors first validated the applicability of such descriptor. Specifically, the authors adopted DFT calculation to obtain the binding energy of CO2 on different functional groups. Then, it was found that the GCMC-derived CO2 adsorption capacity of different MOFs was correlated with the binding energy of CO2 on the functional groups these MOFs contain. The result demonstrated that the features of functional groups significantly influenced the CO2 adsorption capacity of MOFs, thus they must be properly included, after digitalized, in the input set to conduct the ML study. Gradient boosted machine and NN are found to be the most accurate models to correlate these descriptors, the abovementioned chemical together with physical ones, with the CO2 capture performances of MOFs. The simulation results showed that, although the chemical features had been included in this study, the physical characteristics like the pore size, void fraction, surface area, and density are still the dominant influential factors affecting the working capacity of MOFs towards CO2 capture in most cases (as shown in Figure 5B). However, for the selectivity of CO2 over other gas components like N2 or H2, the topology of functional groups in MOFs did matter, indicating that the selectivity of MOFs might be more sensitive to the chemical features of MOFs than the capacity. Desired topological structures derived from the simulation results were bcs, bcu, and fcu (as shown in Figure 5A), while the inherent mechanisms accounting for such superiority remains to be further explored.
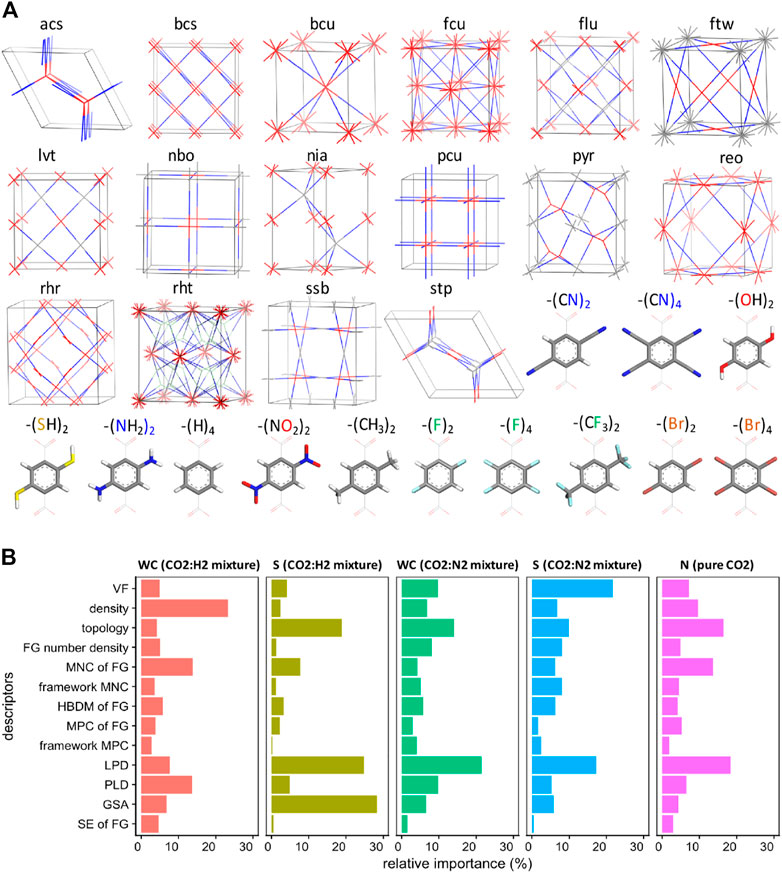
FIGURE 5. (A) Topological blueprints and connecting molecular building blocks used in the construction of the over 400 MOF designs, and (B) Relative importance of material descriptors obtained from GBM training (S = selectivity, WC = working capacity, N = adsorption loading. FG = functional group, VF = void fraction, HDBM = highest dipole moment, MPC = most positive charge, MNC = most negative charge, LPD = largest pore diameter, PLD = limiting pore diameter, SE = sum of epsilons, GSA = gravimetric surface area) (Anderson et al., 2018).
With the physical and chemical descriptors for the MOF-based CO2 capture being proposed, from then on, studies started to investigate the performance of MOF under practical scenarios. The performance of various MOFs for CO2 separation in the presence of water vapor was examined using a NN ML model (Zhang et al., 2021b). Both the physical and chemical features were considered in this case. The simulation results suggested that MOFs with moderate surface area (1750 m2 g−1) and pore diameter (14.2 Å) exhibited an optimized CO2 separation performance if water vapor exists. The applicability of MOFs in direct CO2 capture from air was explored based on RF, NN, DT, and SVM ML models by co-considering the influence of N2 and O2 (Deng et al., 2020). The heat of adsorption was found to be the most important parameter changing the CO2 capture performances of MOFs. It should be noted that the heat of adsorption is a systematical reflection of the chemical properties of MOFs, and the specific chemical features influencing the capture performance remains unspecified. The best-performing MOF with a CSD code of NORGOS possessed a selectivity of CO2 over N2 + O2 of 4712, much superior to the optimal MOFs as identified by experimental pathways. This significant outperformance further supports the unique advantage of ML-based methods in screening and designing CO2 capture agents, while the accuracy of the adsorption capacity and CO2 selectivity are not necessarily in accordance with their experimentally-determined ones. To further consider the real-world conditions, an industrial vacuum swing adsorption (VSA) system was adopted to evaluate the CO2 separation performances of different MOFs from industrial flue gas (Burns et al., 2020), aiming to well-bridge the gap between material optimizations and process designs. The MOF namely ppn-6-CH2DETA was found to possess the highest selectivity towards CO2, which exceeded 50,000 according to the GCMC calculation results. However, IISERP-MOF2, UTSA-16, and zeolite NaA, rather than ppn-6-CH2DETA, were identified to be preferred in this case with parasitic energy of lower than 250 kWhe per MT CO2 and productivities greater than those of pilot-scaled zeolite-13X. These results implied that, in real-world practices, in addition to the adsorption capacity and CO2 selectivity, there are more complicated factors to be included to justify the applicability of MOFs for CO2 separation, which remains yet to be fully clarified.
Carbonaceous materials. Activated carbons, attributed to its porous structure and diversified active ligands, are used for the capture of various environmental pollutants like CO2 (Li and Xiao 2019; Sreńscek-Nazzal and Kielbasa 2019), volatile organic compounds (VOCs) (An et al., 2019; Zhou T. et al., 2019; Li et al., 2020), hazardous metals (Karnjanakom and Maneechakr 2019; Yang et al., 2019; Zhang et al., 2021c), etc. The relatively low unit price, the easy accessibility, the adjustable structure of pores, and the potential to be regenerated make activated carbons promising alternatives to amines for CO2 capture under real-world conditions. Using carbonaceous materials to capture carbon becomes a popular topic nowadays through synthesizing activated adsorbents from biomass wastes. How to prioritizing the effects of different influential factors, that is, the external factors, the physical features of materials, and the chemical characteristics of adsorbents, has become a debating topic recently, and this was the time ML-based methods stepped into this specific area years ago.
To elucidate the influential importance of different types of factors on the CO2 capture performance of activated carbons, several representative descriptors belonging to external factors (temperature and pressure), the physical features of materials (surface area, micropore volume, mesopore volume, and ultra-micropore volume), and the chemical characteristics of adsorbents (the mass percentages of hydrogen, oxygen, nitrogen, and carbon) were featured (Zhu et al., 2020) and processed based on RF model. As shown in Figure 6, the authors found that, within a low-pressure range (0–0.6 bar), pressure primarily affected the CO2 capture performances of activated carbons, while within a high-pressure range (0.6–1 bar), the physical properties of activated carbons dominated. This phenomenon is probably attributed to that 1) microporous and ultra-microporous structures were dominated in these porous carbon materials, and 2) at high pressure, CO2 adsorption on porous carbons proceeded through pore-filling mechanism rather than layer adsorption (Sevilla et al., 2013; Boyjoo et al., 2017). Besides, the authors also found that under a relatively low but unchanged pressure (<0.2 bar), the mesopore volume significantly influenced the CO2 adsorption capability of activated carbons, whereas the ultra-micropore volume took this role over if the pressure increased to >0.6 bar. Although the limited sample size of this study might induce the deviation in the judgement of the role of different features, this work still offered valuable references that inspired further studies in related areas.
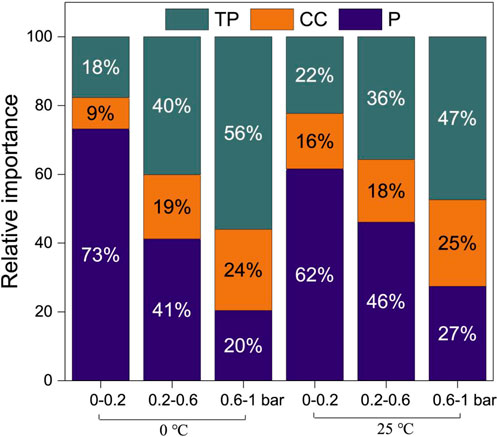
FIGURE 6. The relative importance of integrated influential factors at different pressure ranges (TP, CC and p represented textural properties, chemical compositions and pressure, respectively) (Zhu et al., 2020).
In 2021, the CO2 capture performances of activated carbons were simulated and studied by different tree-based ML algorithms including gradient boosting decision tree (GBDT), light gradient boosting machines (LGB), and extreme gradient boost (XGB) (Yuan X. et al., 2021). GBDT was proven to marginally outperform other 2 ML algorithms in the simulations, and the importance of different types of descriptors follow the order external factors > physical features > chemical characteristics, which was generally in line with previous studies. The CO2 adsorption behaviors of bio-derived N/O-enriched activated carbons were also simulated by a NN-based algorithm, and density functional theory (DFT) calculations were adopted as a supportive method to identify the adsorption mechanism of CO2 (Rahimi et al., 2022). The role of different characteristics, that is, pyridinic nitrogen (N-6), pyrolytic nitrogen (N-5), oxidized nitrogen (N-X), graphitic nitrogen (N-Q), and the fraction of N-6/N-X, of N-containing functional groups was identified, evidencing that N-6, N-5, and N-X considerably functioned in the CO2 capture. The CO2 capture by rice husk derived activated carbons was studied by a NN-based algorithm, while only physical properties like pore volumes and surface areas of activated carbons were considered in this work due to the limited accessibility of data (Palle et al., 2022). It can be clearly found that, for carbonaceous materials, of which the derivation of CO2 capture performance from theoretical calculation is relatively difficult due to the structural complexity of activated carbons, the high-throughput screening on their CO2 selectivity is unpractical at the current stage. This limitation urges the further development of available database that homogeneously describes the structural details of activated carbons in the future.
Polymers. Polymer-based membranes have been widely used in the selective separations of mixed gases like N2/O2 (Himma et al., 2019), N2/H2 (Ockwig and Nenoff 2007), CO2/N2 (Liu et al., 2016), and CO2/CH4 (Liu et al., 2018). Generally, permeability and selectivity are two critical criteria that determines the CO2 separation performances of membranes, whereas these two criteria go against each other under real-world conditions, that is, higher the permeability is, generally lower the selectivity is achieved, and vice versa. However, it should be noted that, although these two parameters negatively correlate, and a ‘Robeson plot’ can be drawn based on this correlation, the upper limit of this plot continuously evolves with the dedications of scientists. The primary purpose of the involvement of ML-based studies in this area is further optimizing the upper limit of such ‘Robeson plot’. It requires to screen and test the CO2 separation performances of a large amount of polymer membranes, which significantly challenges the time-consuming experimental pathways.
How to digitalize the features of polymers, which contains complex ligands that may interact with each other in a twisted manner, is a critical challenge facing the application of ML in relevant fields. In 2020, Barnett et al. for the first time addressed this issue by creating fingerprints for polymers based on topological methods (Barnett et al., 2020) (as shown in Figure 7). Specifically, the authors transformed each polymer into a binary fingerprint using the Daylight-like fingerprinting algorithm as implemented in RDKit. This topological-based approach analyzes the various fragments of a molecule containing a certain number of bonds and then hashes each fragment to produce a binary fingerprint that computationally represents the molecule. After a polymer’s repeat unit was read into memory via a molfile, it was broken down into fragments containing between 1 and 7 units (represented for n = 1 to n = 4 in Figure 7), and the structure was hashed into a fingerprint with 2048 bits of information to encode all of the possible connectivity pathways of the monomer. This process is repeated for each group in the molecule to generate the full fingerprint. Each bit was treated as a single feature in our model, which allows us to study the effects of various functional groups and their linkages on gas transport. Each monomer was connected to at least nine other identical repeat units to properly account for longer paths along the polymer backbone. This fingerprinting technique is the simplest representation of the polymer chemistry and structure that is sufficient to capture trends observed in the experimental data.
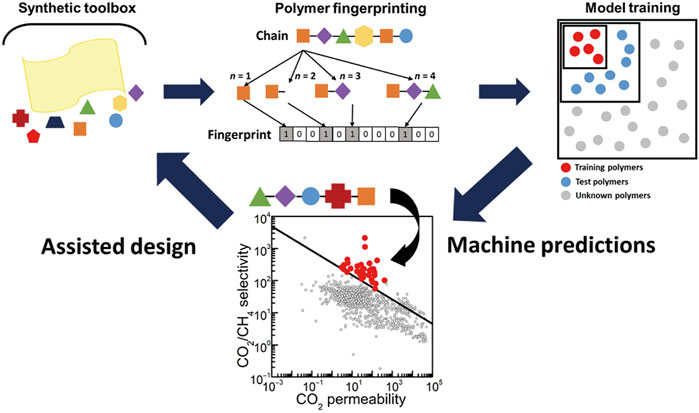
FIGURE 7. Assisted design of high-performance polymer membranes (Barnett et al., 2020).
Based on this novel method, the simulation results showed that two polymers, that is, poly [(1,3-dioxoisoindoline-2,5-diyl) sulfonyl (1,3-dioxoisoindoline-5,2-diyl)-1,4-phenyleneoxy-1,4-phenylene] (ID: P432092) and poly [(1,3-dioxoisoindoline-2,5-diyl) sulfonyl (1,3-dioxoisoindoline-5,2-diyl)-1,4-phenylenemethylene-1,4-phenylene] (ID: P432095) lied well above the upper limit of the ‘Robeson plot’ for CO2/CH4 separation (as shown in Figure 8). Both of these polymers are polyimides containing sulfone groups. Besides, P432092 contains an aromatic ether linkage, and each of these groups is highlighted during the analysis of the ML data as being related to high CO2/CH4 selectivity. In addition to this revolutionary study, another work also explored the permeability of different gas components through polymer membranes (Yuan Q. et al., 2021), and identified that the permeability followed the order O2 > CO2 > N2 > CH4 > He. Two outperforming polymer membranes locating above the Robeson upper limit 2008 were selected out, namely KAUST-PI-1 and PIM-1, for the separation of CO2 and CH4.
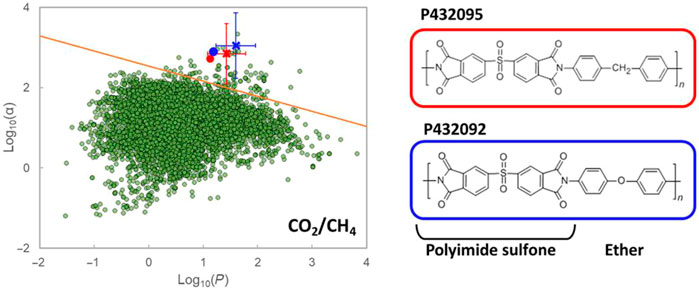
FIGURE 8. Polymer candidates for advanced CO2/CH4 gas transport performance identified through ML and their experimental performance (Barnett et al., 2020).
Zeolites. Zeolites refer to a group of porous materials with the general formula of Mn+1/n (AlO2)-(SiO2)x·yH2O, where Mn+1/n is either a metal ion or H+. Considering the porous and functionalized nature of zeolites, they are widely used as commercial adsorbents for gaseous pollutant removal from industrial flue gas (Ding and Yazaydin 2013; Sun et al., 2014; Bakhtyari et al., 2020; Ma et al., 2021). Several models including the Hybrid-ANFIS, PSO-ANFIS, and LSSVM had been proposed for the prediction of the CO2 adsorption on various zeolites (Raji et al., 2022). The accuracy of the models was assessed based on calculating the MSE AARD, R2, and STD. According to the results, it is shown that the all models are reliable and precise for estimating the CO2 adsorption on various zeolites and applicable methods for the design and analysis of the CO2 adsorption processes. It can be concluded from Figure 9 that pressure increment has a higher effect on the CO2 adsorption performance than temperature. In Zeolite 5A, the effect of temperature was more obvious than other zeolites. For zeolite T type and ssz-13 in the temperature around 330 K, there can be seen an optimal point for CO2 adsorption.
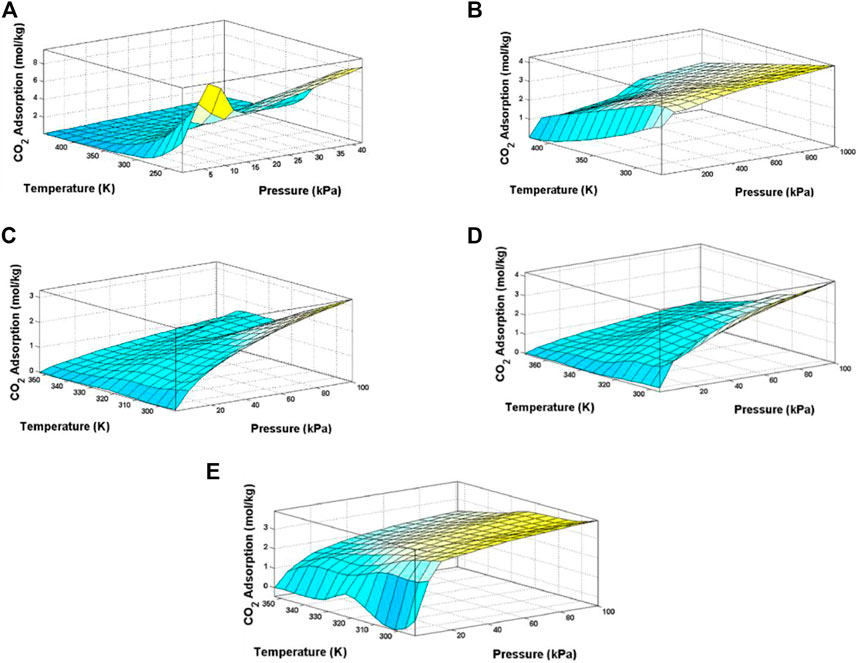
FIGURE 9. Effect of Temperature and pressure on CO2 adsorption (axial Z) on various types of zeolite (A) 13X, (B) 5A (C) SAPO-34 (D) SSZ-13 and (E) T-Type (Raji et al., 2022).
Absorbents
Ionic liquids (IL). IL generally refers to a group of subset examples of molten salts fully comprised of abundant ions (anions and cations) with melting point below 100 °C (Lei et al., 2017; Zhang et al., 2021a; Zhang et al., 2021). Since the last decade when IL was found to be a promising absorbent for CO2 capture (Blanchard et al., 1999), this group of nonvolatile and designable materials has been extensively explored for its functionalized role in related fields (Zeng et al., 2017). Purposefully and pertinently engineering the functional ligands in IL is the key challenge for the further improvement of CO2 selectivity over other gas components and the applicability of IL-based CO2 technologies. The intrinsic complexity of the synthetical and processing optimization makes ML an emerging and effective method to such a sophisticated end. The ways of data collection are categorized into two types, that is, collecting from previous studies and calculating based on theoretical methods. The former one merits for its precision in performing data analysis and processing, while the later one may contain a wider range of material variety for CO2 capture.
In 2017, ML was for the first time used as a tool to investigate the CO2 capture by ILs (Venkatraman and Alsberg, 2017), and the performances of different ILs were collected based on experimentally determined results (Lei et al., 2014). 185 ionic liquids (68 cations, 65 anions) comprised of 10,848 solubility measurements, which is widely inclusive, across different temperatures and pressures were used as the fundamental database for the model training and testing (Figure 10 showed prominent cations (prefixed with “C”) and anions (prefixed with “A”) found in the dataset). Both physical and chemical features, including the HOMO/LUMO energies, polarizabilities, superdelocalizabilities, charge partial surface areas (CPSA), and various geometrical indices as derived from semi-empirical MOPAC, were considered. The main goal of this work was examining the accuracy of different ML algorithms in fitting the CO2 capture capabilities of ILs, and found that random forest was more suitable than decision tree and partial least squares regression in this case, probably attributed to the adaptability of random forest in learning datasets with considerable diversity and deviation. However, the R2 as obtained equaling to 0.71 was still far from satisfactory due to such intrinsic complexity. A limited batch of ILs containing several designated functional groups was hence studied, aiming to attain more precise results within a specific IL scope (Mesbah et al., 2018; Daryayehsalameh et al., 2021). It was identified that, when only [Bmim][BF4] ILs was considered, a NN-based ML model exhibited a much higher accuracy with R2 equaling to 0.99 and 0.98 for the training and testing sets. The effects of pressure and temperature on the CO2 capture performance of [Bmim][BF4] ILs were predicted, indicating that a relatively high pressure and low temperature might be beneficial for CO2 capture by [Bmim][BF4] ILs. Besides, by using a relative sophisticated multi-layer NN-based model with group contribution (GC) methods, a satisfactory precision was also obtained for a wider scope of experimentally collected ILs with more than 10,000 data points (Song et al., 2020).
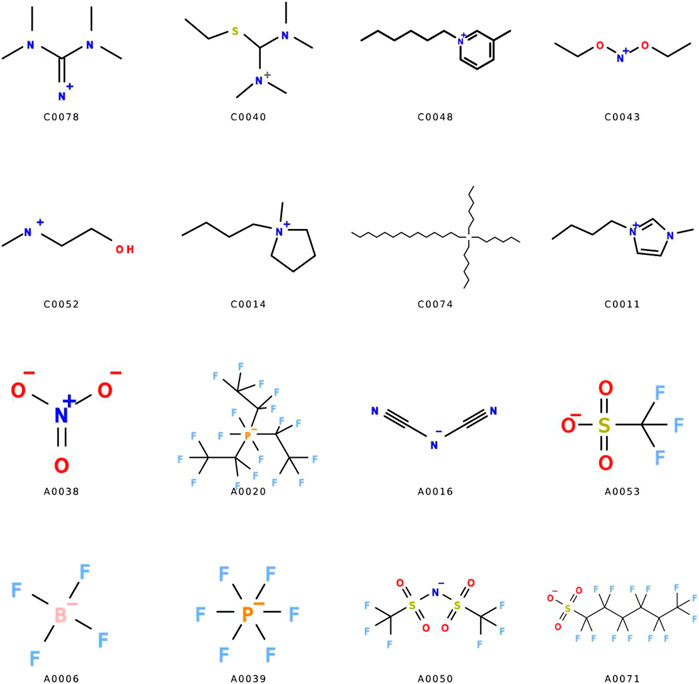
FIGURE 10. Prominent cations (prefixed with “C”) and anions (prefixed with “A”) found in the dataset (Venkatraman and Alsberg, 2017).
While extensive ML-guided studies have been conducted to study the laws of CO2 solubility in ILs, there are still two critical issues remaining to be well-addressed, that is, 1) the predicament between the scale of IL database and the prediction accuracy due to the influence of diversified variables, and 2) the insufficient understanding of the selectivity of CO2 over other gas components because of the limited amounts of relevant experimental results. To obtain an improved data homogeneity, the theoretically derived mass-based Absorption-Selectivity-Desorption index (ASDI) was adopted to characterize the selectivity of approximately 1000 IL systems towards CO2/N2 separation (Wang K et al., 2021). Cyano-based ILs were found to be excellent solvents for separating CO2 and N2, and Aspen Plus [EMIM][TCM] was selected as best IL, the process of which led to 12.9% savings on total annualized cost (TAC) compared to that of [EMIM][Tf2N]. Following this effective method, a subsequent study extended the database of screened ILs to more than 400,000 by adopting the Simplified Molecular Input Line Entry System (SMILES) that sequences strings of symbols in ILs based on their graph theory (Zhang et al., 2021) (a brief illustration on the technical roadmap is shown in Figure 11). The ILs with sequencing number of CCCc1n (CCOc2ccccc2)cc [n + ]1CCOC and CCCc1n (CCC)cc [n + ]1CCOc1ccccc1 were found to exhibit superior performances towards the CO2 separation from H2 or N2. However, as the authors did not consider whether the involved computationally derived structures can be successfully synthesized via experiments, further validation on the feasibility and applicability of these ILs needs to be conducted in the future.
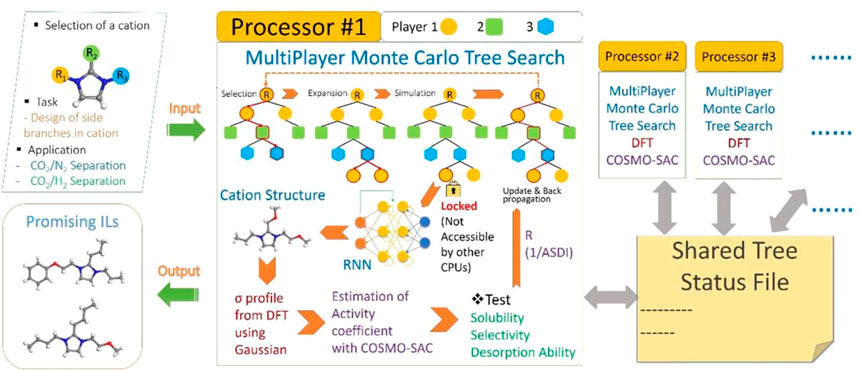
FIGURE 11. A schematic of our algorithm to design an application-specific ionic liquid (Zhang et al., 2021).
To leverage both the structural motif of MOFs and the absorption ability of ILs, the CO2 capture performances of IL-MOF composites were also scrutinized based on ML models (Zhang Z. et al., 2022). The configurational-bias Monte Carlo (CBMC) method was adopted to insert 1-aminopropyl imidazolium bis(trifluoromethylsulfonyl) imine ([NH2-Pmim][Tf2N]) into different MOFs. GCMC was used to calculate the adsorption capacity and CO2 selectivity of IL-MOF composites, and the RF algorithm was taken to perform the ML process. It was identified that the accessible volume (AV), the density (ρ), and free volume (Vfree) are three major influential factors affecting the CO2/N2 selectivity of IL-MOF composites. Structure-performance relationship revealed that IL-MOF with optimal AV (0.176–0.444 cm3 g−1), ρ (1.295–2.046 g⋅cm−3), and Vfree (1724–4785 Å3) result in decent CO2/N2 selectivity. [NH2-Pmim][Tf2N]@ZIF-67, an IL-MOF composite exhibiting moderate CO2 separation performance, was synthesized to successfully verify the accuracy of the ML simulation. However, it should be noted that, in this study, the authors primarily investigated how the change of MOF characteristics influenced the CO2 separation performances of IL-MOF composites, while the features of ILs were skipped. Further scrutinization on the synergetic effects between ILs and MOFs are of great importance to make the performance prediction more practical and precise.
Amine-based absorbents. Amine-based materials have been widely commercialized for CO2 capture from large-scale industrial processes, MEA, piperazine (PZ), and N-methyldiethanolamine (MDEA) are the most studied absorbents in previous works (Dutcher et al., 2015; Yamada 2021). The mechanism of amine-based CO2 capture technologies generally involves the reaction between amine and CO2 to form carbamate anion (NCOO−) and protonated amine (BH+). The predominant merit of amine-based CO2 capture technologies is its reversibility, that is, the feasible regeneration of spent absorbents via relatively facile and cost-effective methods, which significantly reduces the operation cost and makes the commercialization preferred. Due to the lack of applicable computational tools to obtain the absorption capacities of amine-based materials, previous studies all relied on experimental results to conduct ML studies in this field. Under this circumstance, the complex effects of external influential factors may become a major obstacle that impedes the derivation of a highly consistent conclusion. Thus, ML based methods, as an effective method to generalize parameters, began to assist practitioners in designing the next-generation amine absorbents, which may be applicable for highly selective CO2 capture.
As a widely adopted amine-based absorbents, PZ was taken as the first example in ML screening and prediction for its CO2 capture performance (Yarveicy et al., 2018). More than 1000 data points were collected from previous studies, and the reaction temperature, CO2 partial pressure, and the concentration of PZ were featured as descriptors to explore the influence of external factors on the CO2 separation performance of pZ. Four different algorithms including NN, adaptive neuro-fuzzy inference system (ANFIS), SVM, and DT were tested for their suitability and accuracy in this case. It was found that the DT-based algorithm exhibited the highest accuracy with the R2 reaching ∼0.99. A conclusion was drawn that CO2 partial pressure had the predominant impacts on the CO2 absorption performance of PZ, followed by the concentration of PZ and the reaction temperature. Under the optimal reaction condition, that is, reaction temperature, the concentration of PZ, and CO2 partial pressure equaled to 313.15 K, 1.913 mol L−1, and 7.51 kPa, the absorption capacity of PZ towards CO2 was 0.86 mol CO2 per mol pZ. Besides, the influences of reaction temperature, CO2 partial pressure, and the concentration of amine on the CO2 absorption performance of MEA, diethanolamine (DEA), and triethanolamine (TEA) were also scrutinized (Ghiasi et al., 2019). It was surprisingly found that for different amines, the dominant experimental condition changed. Specifically, for MEA and TEA, the importance of different experimental conditions followed the order reaction temperature > partial pressure > the concentration of amines, while for DEA, the order changed to partial pressure > reaction temperature > the concentration of amines. Although errors may exist due to the insufficiency of experimental data, this work also gives valuable implications on the diversity of amine design.
Subsequent studies further extended the variety of descriptors and amines to provide more generalized guidance for absorbent optimization. In addition to reaction temperature, the pressure of flue gas, the flow rate, the pressure of re-boiler, reboiler duty, and condenser duty were also considered to further approximate the practical scenarios (Shalaby et al., 2021). An optimized operation condition was obtained to be flow rate = 4.01 mol s−1, temperature = 319.7 K, the pressure of flue gas = 103.5 kPa, reboiler pressure = 160.0 kPa, reboiler duty = 153,600 W, and condenser duty = 8600 W. It could be found that the optimal reaction temperature as obtained under a relatively complex condition did not deviate from that obtained under simple conditions. Besides, the CO2 capture performance of a novel group of amine-based absorbents, that is, amino acid salt (AAs), were also recently explored by ML-based methods (Pakzad et al., 2020; Dashti et al., 2021a; Mehrabi et al., 2022). In these studies, the intrinsic features of amines, covering the molecular weight of AAs, hydrogen bond donor count, hydrogen bond acceptor count, rotatable bond count, and heavy atom count, were for the first time co-considered with the external influential factors. Different algorithms including SVM, NN, ANFIS, and particle swarm optimization-ANFIS (PSO-ANFIS) were adopted to perform the simulation, among which the NN-based one exhibited the highest accuracy (as shown in Figure 12). The rotatable bond count and the molecular weight of AAs were identified to be the predominant factors influencing the CO2 capture performance of AAs.
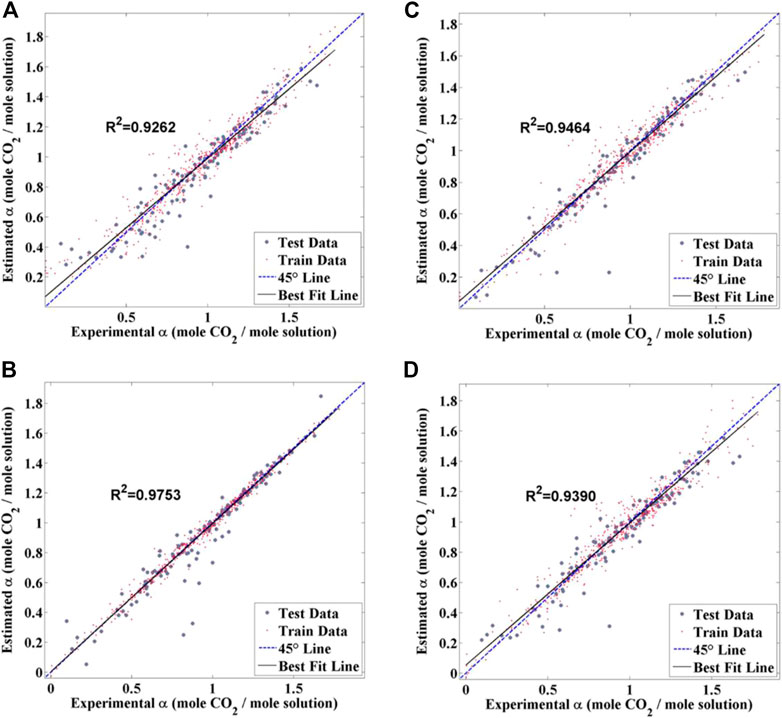
FIGURE 12. Regression plot for the absorption of CO2 prognostication by (A) LSSVM, (B) RBF-NN, (C) PSO-ANFIS, and (D) ANFIS (Dashti et al., 2021a).
Deep eutectic solvent (DES). DES is a new generation of IL analogues sharing similar physicochemical properties as IL, such as negligible vapor pressure, high chemical/thermal stability and easily tunable character. Compared to ILs, DESs offer two advantages: 1) DESs can be prepared easily by mixing a hydrogen bond acceptor (HBA) with a hydrogen bond donor (HBD), avoiding complex synthesis and purification steps for ILs; 2) A large number of cheap and renewable compounds can act as the HBA (e.g., ammonium and phosphonium salts) or HBD (e.g., organic alcohols and acids), making DESs more affordable and sustainable over ILs. Using DES for CO2 capture was extensively investigated in recent years, and ML-based methods were adopted to screen different DESs for their CO2 absorption performances (Dashti et al., 2021b; Wang K et al., 2021).
A typical example to design DESs CO2 absorbents with the assistance of ML-based methods was the application of a random forest derived ML model for performance description (Wang J et al., 2021). Before conducting ML studies, the structures of HBA and HBD of EDSs were first optimized using Gaussian software package (as shown in Figure 13). The red part and the blue part represent positive COSMO charge density and negative charge distribution, respectively. In order to generate the desired COSMO-RS-derived descriptors, the obtained COSMO files are directly imported into the COSMOthermX software to output the σ-profiles of HBAs and HBDs. The qualitative analysis from multiple linear regression shows that the variables, including the descriptors of HBAs and HBDs, molar ratio of HBA to HBD as well as the temperature and pressure, are efficient input parameters for predicting CO2 solubility in DESs. The importance of the involved variables in the QSPR model is ranked as pressure > HBA type > HBD type > HBA:HBD molar ratio > temperature.
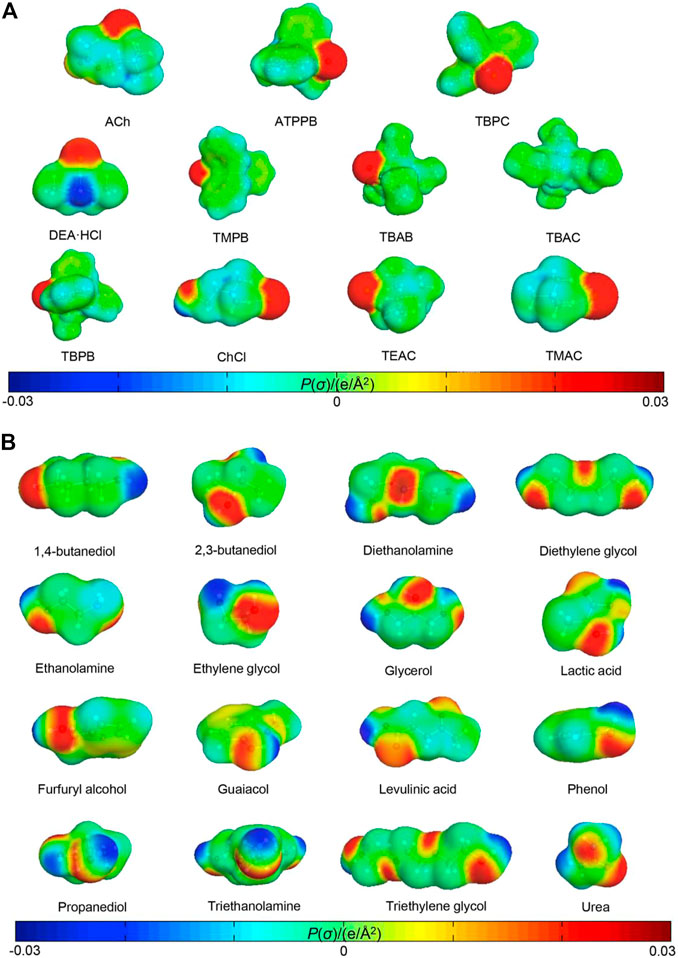
FIGURE 13. Optimized (A) HBA and (B) HBD structures with COSMO surfaces (Wang J et al., 2021).
Outlooks and conclusions
In the past 5 years, due to the rapid development of artificial intelligent and the explosion of material database, ML has stepped into the CO2 separation area as an effective and efficient tool for ab-/adsorbent screening and design. Various ML algorithms including but not limited to MLR, RF, NN, DT, and SVM demonstrated their powers in this process where time-consuming and costly experiments were skipped. The CO2 capture performances of several types of materials were simulated and scrutinized by ML-based methods. Novel materials that might serve the future benefits of carbon capture and sustainable energy conversion were proposed, parts of which proceeded far ahead of the current synthetical techniques. These achievements fully reflect the huge potentials of ML in improving CO2 capture technologies, while the following issues may be the primary concerns to be well-addressed in the future to uncover these potentials.
The first issue is the establishment of consistent and integrated databases that make the influence of microcosmic descriptors more explorable. In previous studies, different scientists generally conducted experiments under varied conditions, and such variety made the effect of the intrinsic properties of materials hard to be identified. In this case, the explorers must firstly take the experimental conditions into consideration to train a reasonable model. However, the inclusion of external factors only contributes marginally to the rationally design of materials. Besides, it is noted that the criteria used to judge the CO2 capture performance was different in different works, and the typical criteria used included the CO2 capacity, the selectivity of CO2 over other gas components, and the CO2 removal efficiency. In a specific work, there were only one to two criteria being adopted, which further impedes the generation of integrated data in ML studies. Thus, it is suggested that a consistent experimental condition will be adopted for a same type of materials, and an integrated testing on its CO2 capture performances involving diverse criteria, especially the CO2 selectivity, is highly preferred.
The second issue is the development of effective methods to digitalize the structural properties of materials. Although the CO2 adsorption over a wide variety of materials has been investigated, it is found that the descriptors used in most works were primarily the textural and compositional properties of materials. A sophisticated method to digitalize and visualize the complex interactions among atoms and ligands in the materials remains yet to be well-developed, while such inherent properties may play predominant roles in CO2 capture, especially in cases where CO2 is chemisorbed by sorbents like solid-state metal salts, which have hardly been investigated by ML-based pathways due to the lack of digitalized methods to feature their properties. To this end, topological method may be an extremely useful tool. However, due to the interdisciplinary nature to achieve the digitalization of such complex features, it was rarely applied in previous studies. Overcoming this critical challenge requires the participation and dedication of more interdisciplinary talents with both computer science and material science backgrounds in the future.
The third issue is the validation of the accuracy of ML-derived results. Despite the effectiveness ML displaying in designing CO2 capture agents, it must be admitted that experimental verifications had rarely been adopted to justify the performances of these agents. The evidence of the accuracy of ML-based studies stuck in a non-rigorous cycle where the CO2 performance was obtained from calculation, and a similar calculation process was conducted to test its validation. While self-consistent results could be generated on this basis, they may be far from the real outcomes obtained under practical scenarios. This is why this review urges the attention to the selectivity of CO2 because no practical condition can ignore the influence of interfering gas components. Besides, this challenge also identifies the importance to conduct experimental verification on the ML-derived results. Otherwise, the application of ML in CO2 will only be a costly activity rather than a beneficial pathway towards the mitigation of climate change in the future.
Author contributions
ZY organized the analysis and wrote the manuscript, BC collected the data and revised the manuscript, HC collected the data, and HL conceived and supervised the research.
Funding
This work is supported by the Science and Technology Innovation Program of Hunan Province (No. 2021RC4005).
Conflict of interest
The authors declare that the research was conducted in the absence of any commercial or financial relationships that could be construed as a potential conflict of interest.
Publisher’s note
All claims expressed in this article are solely those of the authors and do not necessarily represent those of their affiliated organizations, or those of the publisher, the editors and the reviewers. Any product that may be evaluated in this article, or claim that may be made by its manufacturer, is not guaranteed or endorsed by the publisher.
References
Abdollahzadeh, M., Khosravi, M., Hajipour Khire Masjidi, B., Samimi Behbahan, A., Bagherzadeh, A., Shahkar, A., et al. (2022). Estimating the density of deep eutectic solvents applying supervised machine learning techniques. Sci. Rep. 12, 4954. doi:10.1038/s41598-022-08842-5
Aghaji, M., Fernandez, M., Boyd, P., Daff, T., and Woo, T. (2016). Quantitative structure-property relationship models for recognizing metal organic frameworks (MOFs) with high CO2 working capacity and CO2/CH4 selectivity for methane purification. Eur. J. Inorg. Chem. 2016, 4505–4511. doi:10.1002/ejic.201600365
Ali, E., Hadj-Kali, M., Mulyono, S., and Alnashef, I. (2016). Analysis of operating conditions for CO2 capturing process using deep eutectic solvents. Int. J. Greenh. Gas Control 47, 342–350. doi:10.1016/j.ijggc.2016.02.006
An, Y., Fu, Q., Zhang, D., Wang, Y., and Tang, Z. (2019). Performance evaluation of activated carbon with different pore sizes and functional groups for voc adsorption by molecular simulation. Chemosphere 227, 9–16. doi:10.1016/j.chemosphere.2019.04.011
Anderson, R., Rodgers, J., Argueta, E., Biong, A., and Gómez-Gualdrón, D. (2018). Role of pore chemistry and topology in the CO2 capture capabilities of MOFs: From molecular simulation to machine learning. Chem. Mat. 30, 6325–6337. doi:10.1021/acs.chemmater.8b02257
A. Bakhtyari, M. Mofarahi, C. Lee, M. Rahimpour, M. Farsi, and M. Makarem (Editors) (2020). “Chapter 9 - CO2 adsorption by conventional and nanosized zeolites,” Advances in carbon capture (Netherland: Elsevier), 193–228. doi:10.1016/B978-0-12-819657-1.00009-8
A. Bakhtyari, M. Mofarahi, M. Rahimpour, M. Makarem, and M. Kiani (Editors) (2022). “Chapter 3 - thermophysical properties of nanofluids,” Nanofluids and mass transfer (Netherland: Elsevier), 39–96. doi:10.1016/B978-0-12-823996-4.00003-3
Barnett, J., Bilchak, C., Wang, Y., Benicewicz, B., Murdock, L., Bereau, T., et al. (2020). Designing exceptional gas-separation polymer membranes using machine learning. Sci. Adv. 6, eaaz4301. doi:10.1126/sciadv.aaz4301
Barzagli, F., Giorgi, C., Mani, F., and Peruzzini, M. (2020). Screening study of different amine-based solutions as sorbents for direct CO2 capture from air. ACS Sustain. Chem. Eng. 8, 14013–14021. doi:10.1021/acssuschemeng.0c03800
Behler, J., and Parrinello, M. (2007). Generalized neural-network representation of high-dimensional potential-energy surfaces. Phys. Rev. Lett. 98, 146401. doi:10.1103/PhysRevLett.98.146401
Blanchard, L., Hancu, D., Beckman, E., and Brennecke, J. (1999). Green processing using ionic liquids and CO2. Nature 399, 28–29. doi:10.1038/19887
Boyjoo, Y., Cheng, Y., Zhong, H., Tian, H., Pan, J., Pareek, V., et al. (2017). From waste Coca Cola® to activated carbons with impressive capabilities for CO2 adsorption and supercapacitors. Carbon 116, 490–499. doi:10.1016/j.carbon.2017.02.030
Burns, T., Pai, K., Subraveti, S., Collins, S., Krykunov, M., Rajendran, A., et al. (2020). Prediction of MOF performance in vacuum swing adsorption systems for postcombustion CO2 capture based on integrated molecular simulations, process optimizations, and machine learning models. Environ. Sci. Technol. 54, 4536–4544. doi:10.1021/acs.est.9b07407
Butler, K., Davies, D., Cartwright, H., Isayev, O., and Walsh, A. (2018). Machine learning for molecular and materials science. Nature 559, 547–555. doi:10.1038/s41586-018-0337-2
Chen, C., Baiyee, Z., and Ciucci, F. (2015). Unraveling the effect of la a-site substitution on oxygen ion diffusion and oxygen catalysis in perovskite BaFeO3 by data-mining molecular dynamics and density functional theory. Phys. Chem. Chem. Phys. 17, 24011–24019. doi:10.1039/C5CP03973H
Chen, C., Zuo, Y., Ye, W., Li, X., Deng, Z., and Ong, S. (2020). A critical review of machine learning of energy materials. Adv. Energy Mat. 10, 1903242. doi:10.1002/aenm.201903242
Chen, Z., Zhou, Y., and Li, H. (2022). A review of phase behavior mechanisms of CO2 EOR and storage in subsurface formations. Ind. Eng. Chem. Res. 61, 10298–10318. doi:10.1021/acs.iecr.2c00204
Daryayehsalameh, B., Nabavi, M., and Vaferi, B. (2021). Modeling of CO2 capture ability of [Bmim] [BF4] ionic liquid using connectionist smart paradigms. Environ. Technol. Innovation 22, 101484. doi:10.1016/j.eti.2021.101484
Dashti, A., Amirkhani, F., Hamedi, A., and Mohammadi, A. (2021a). Evaluation of CO2 absorption by amino acid salt aqueous solution using hybrid soft computing methods. ACS Omega 6, 12459–12469. doi:10.1021/acsomega.0c06158
Dashti, A., Raji, M., Amani, P., Baghban, A., and Mohammadi, A. (2021b). Insight into the estimation of equilibrium CO2 absorption by deep eutectic solvents using computational approaches. Sep. Sci. Technol. 56, 2351–2368. doi:10.1080/01496395.2020.1828460
Deng, X., Yang, W., Li, S., Liang, H., Shi, Z., and Qiao, Z. (2020). Large-scale screening and machine learning to predict the computation-ready, experimental metal-organic frameworks for CO2 capture from air. Appl. Sci. 10, 569. doi:10.3390/app10020569
Ding, L., and Yazaydin, A. (2013). The effect of SO2 on CO2 capture in zeolitic imidazolate frameworks. Phys. Chem. Chem. Phys. 15, 11856–11861. doi:10.1039/C3CP51003D
Ding, M., Flaig, R., Jiang, H., and Yaghi, O. (2019). Carbon capture and conversion using metal-organic frameworks and MOF-based materials. Chem. Soc. Rev. 48, 2783–2828. doi:10.1039/C8CS00829A
Doreswamy, K. S. H., Km, Y., and Gad, I. (2020). Forecasting air pollution particulate matter (PM2.5) using machine learning regression models. Procedia Comput. Sci. 171, 2057–2066. doi:10.1016/j.procs.2020.04.221
Dureckova, H., Krykunov, M., Aghaji, M., and Woo, T. (2019). Robust machine learning models for predicting high CO2 working capacity and CO2/H2 selectivity of gas adsorption in metal organic frameworks for precombustion carbon capture. J. Phys. Chem. C 123, 4133–4139. doi:10.1021/acs.jpcc.8b10644
Dutcher, B., Fan, M., and Russell, A. (2015). Amine-based CO2 capture technology development from the beginning of 2013—a review. ACS Appl. Mat. Interfaces 7, 2137–2148. doi:10.1021/am507465f
Fernandez, M., Boyd, P., Daff, T., Aghaji, M., and Woo, T. (2014). Rapid and accurate machine learning recognition of high performing metal organic frameworks for CO2 capture. J. Phys. Chem. Lett. 5, 3056–3060. doi:10.1021/jz501331m
Gaikwad, S., Kim, Y., Gaikwad, R., and Han, S. (2021). Enhanced CO2 capture capacity of amine-functionalized MOF-177 metal organic framework. J. Environ. Chem. Eng. 9, 105523. doi:10.1016/j.jece.2021.105523
Ghanbari, T., Abnisa, F., and Wan Daud, W. (2020). A review on production of metal organic frameworks (MOF) for CO2 adsorption. Sci. Total Environ. 707, 135090. doi:10.1016/j.scitotenv.2019.135090
Ghiasi, M., Abedi-Farizhendi, S., and Mohammadi, A. (2019). Modeling equilibrium systems of amine-based CO2 capture by implementing machine learning approaches. Environ. Prog. Sustain. Energy 38, 13160. doi:10.1002/ep.13160
Gražulis, S., Chateigner, D., Downs, R., Yokochi, A., Quirós, M., Lutterotti, L., et al. (2009). Crystallography open database-an open-access collection of crystal structures. J. Appl. Crystallogr. 42, 726–729. doi:10.1107/S0021889809016690
Groom, C., and Allen, F. (2014). The cambridge structural database in retrospect and prospect. Angew. Chem. Int. Ed. 53, 662–671. doi:10.1002/anie.201306438
Guan, J., Huang, T., Liu, W., Feng, F., Japip, S., Li, J., et al. (2022). Design and prediction of metal organic framework-based mixed matrix membranes for CO2 capture via machine learning. Cell Rep. Phys. Sci. 3, 100864. doi:10.1016/j.xcrp.2022.100864
Gupta, S., and Li, L. (2022). The potential of machine learning for enhancing CO2 sequestration, storage, transportation, and utilization-based processes: A brief perspective. JOM 74, 414–428. doi:10.1007/s11837-021-05079-x
Haider, M. B., and Kumar, R. (2020). Solubility of CO2 and CH4 in sterically hindered amine-based deep eutectic solvents. Sep. Purif. Technol. 248, 117055. doi:10.1016/j.seppur.2020.117055
Himma, N., Wardani, A., Prasetya, N., Aryanti, P., and Wenten, I. (2019). Recent progress and challenges in membrane-based O2/N2 separation. Rev. Chem. Eng. 35, 591–625. doi:10.1515/revce-2017-0094
Hu, Y., Guo, Y., Sun, J., Li, H., and Liu, W. (2019). Progress in mgo sorbents for cyclic CO2 capture: A comprehensive review. J. Mat. Chem. A Mat. 7, 20103–20120. doi:10.1039/C9TA06930E
Hu, Y., Lu, H., and Li, H. (2021). Single step fabrication of spherical cao pellets via novel agar-assisted moulding technique for high-temperature CO2 capture. Chem. Eng. J. 404, 127137. doi:10.1016/j.cej.2020.127137
Huang, B., and von Lilienfeld, O. (2016). Communication: Understanding molecular representations in machine learning: The role of uniqueness and target similarity. J. Chem. Phys. 145, 161102. doi:10.1063/1.4964627
Jablonka, K., Ongari, D., Moosavi, S., and Smit, B. (2020). Big-data science in porous materials: Materials genomics and machine learning. Chem. Rev. 120, 8066–8129. doi:10.1021/acs.chemrev.0c00004
Jain, A., Ong, S., Hautier, G., Chen, W., Richards, W., Dacek, S., et al. (2013). Commentary: The materials project: a materials genome approach to accelerating materials innovation. Apl. Mater. 1, 011002. doi:10.1063/1.4812323
Kargbo, H., Zhang, J., and Phan, A. (2021). Optimisation of two-stage biomass gasification for hydrogen production via artificial neural network. Appl. Energy 302, 117567. doi:10.1016/j.apenergy.2021.117567
Karnjanakom, S., and Maneechakr, P. (2019). Adsorption behaviors and capacities of cr(vi) onto environmentally activated carbon modified by cationic (hdtma and ddab) surfactants. J. Mol. Struct. 1186, 80–90. doi:10.1016/j.molstruc.2019.03.022
Khoramzadeh, E., Mofarahi, M., and Lee, C. (2019). Equilibrium adsorption study of CO2 and N2 on synthesized zeolites 13x, 4a, 5a, and beta. J. Chem. Eng. Data 64, 5648–5664. doi:10.1021/acs.jced.9b00690
Lei, Z., Chen, B., Koo, Y., and MacFarlane, D. (2017). Introduction: Ionic liquids. Chem. Rev. 117, 6633–6635. doi:10.1021/acs.chemrev.7b00246
Lei, Z., Dai, C., and Chen, B. (2014). Gas solubility in ionic liquids. Chem. Rev. 114, 1289–1326. doi:10.1021/cr300497a
Li, M., and Xiao, R. (2019). Preparation of a dual pore structure activated carbon from rice husk char as an adsorbent for CO2 capture. Fuel Process. Technol. 186, 35–39. doi:10.1016/j.fuproc.2018.12.015
Li, X., Liu, J., Jiang, W., Gao, G., Wu, F., Luo, C., et al. (2021). Low energy-consuming CO2 capture by phase change absorbents of amine/alcohol/H2O. Sep. Purif. Technol. 275, 119181. doi:10.1016/j.seppur.2021.119181
Li, X., Zhang, L., Yang, Z., He, Z., Wang, P., Yan, Y., et al. (2020). Hydrophobic modified activated carbon using pdms for the adsorption of vocs in humid condition. Sep. Purif. Technol. 239, 116517. doi:10.1016/j.seppur.2020.116517
Liu, J., Hou, X., Park, H. B., and Lin, H. (2016). High-performance polymers for membrane CO2/N2 separation. Chem. Eur. J. 22, 15980–15990. doi:10.1002/chem.201603002
Liu, Y., Liu, G., Zhang, C., Qiu, W., Yi, S., Chernikova, V., et al. (2018). Enhanced CO2/CH4 separation performance of a mixed matrix membrane based on tailored MOF-polymer formulations. Adv. Sci. (Weinh). 5, 1800982. doi:10.1002/advs.201800982
Lu, W., Xiao, R., Yang, J., Li, H., and Zhang, W. (2017). Data mining-aided materials discovery and optimization. J. Materiomics 3, 191–201. doi:10.1016/j.jmat.2017.08.003
Ma, Y., Xu, T., Wang, J., Shi, Y., Wang, H., Xiong, F., et al. (2021). Superior Hg0 capture performance and SO2 resistance ofCo-Mn binary metal oxide-modified layered MCM-22 zeolite for SO2-containing flue gas. Environ. Sci. Pollut. Res. 28, 16447–16457. doi:10.1007/s11356-020-12214-9
Mehrabi, K., Bakhtyari, A., Mofarahi, M., and Lee, C. (2022). Facile and accurate calculation of the density of amino acid salt solutions: a simple and general correlation vs artificial neural networks. Energy fuels 36, 7661–7675. doi:10.1021/acs.energyfuels.2c01705
Mesbah, M., Shahsavari, S., Soroush, E., Rahaei, N., and Rezakazemi, M. (2018). Accurate prediction of miscibility of CO2 and supercritical CO2 in ionic liquids using machine learning. J. CO2 Util. 25, 99–107. doi:10.1016/j.jcou.2018.03.004
Ockwig, N., and Nenoff, T. (2007). Membranes for hydrogen separation. Chem. Rev. 107, 4078–4110. doi:10.1021/cr0501792
Oschatz, M., and Antonietti, M. (2018). A search for selectivity to enable CO2 capture with porous adsorbents. Energy Environ. Sci. 11, 57–70. doi:10.1039/C7EE02110K
Pakzad, P., Mofarahi, M., Izadpanah, A., and Afkhamipour, M. (2020). Experimental data, thermodynamic and neural network modeling of CO2 absorption capacity for 2-amino-2-methyl-1-propanol (AMP) + methanol (MeOH) + H2O system. J. Nat. Gas Sci. Eng. 73, 103060. doi:10.1016/j.jngse.2019.103060
Palle, K., Vunguturi, S., Gayatri, S., Rao, K., Babu, P., and Vijay, R. (2022). The prediction of CO2 adsorption on rice husk activated carbons via deep learning neural network. MRS Commun. 12, 434–440. doi:10.1557/s43579-022-00197-2
Pera-Titus, M. (2014). Porous inorganic membranes for CO2 capture: present and prospects. Chem. Rev. 114, 1413–1492. doi:10.1021/cr400237k
Podryabinkin, E., and Shapeev, A. (2017). Active learning of linearly parametrized interatomic potentials. Comput. Mater. Sci. 140, 171–180. doi:10.1016/j.commatsci.2017.08.031
Rahimi, M., Abbaspour-Fard, M., Rohani, A., Yuksel Orhan, O., and Li, X. (2022). Modeling and optimizing N/O-enriched bio-derived adsorbents for CO2 capture: Machine learning and DFT Calculation Approaches. Ind. Eng. Chem. Res. 61, 10670–10688. doi:10.1021/acs.iecr.2c01887
Rahimi, M., Moosavi, S., Smit, B., and Hatton, T. (2021). Toward smart carbon capture with machine learning. Cell Rep. Phys. Sci. 2, 100396–103864. doi:10.1016/j.xcrp.2021.100396
Raji, M., Dashti, A., Alivand, M., and Asghari, M. (2022). Novel prosperous computational estimations for greenhouse gas adsorptive control by zeolites using machine learning methods. J. Environ. Manag. 307, 114478. doi:10.1016/j.jenvman.2022.114478
Ramprasad, R., Batra, R., Pilania, G., Mannodi-Kanakkithodi, A., and Kim, C. (2017). Machine learning in materials informatics: Recent applications and prospects. npj Comput. Mat. 3, 54. doi:10.1038/s41524-017-0056-5
Rezaei, F., and Jones, C. (2014). Stability of supported amine adsorbents to SO2 and NOx in postcombustion CO2 capture. 2. Multicomponent adsorption. Ind. Eng. Chem. Res. 53, 12103–12110. doi:10.1021/ie502024z
Sevilla, M., Parra, J., and Fuertes, A. (2013). Assessment of the role of micropore size and N-doping in CO2 capture by porous carbons. ACS Appl. Mat. Interfaces 5, 6360–6368. doi:10.1021/am401423b
Shalaby, A., Elkamel, A., Douglas, P., Zhu, Q., and Zheng, Q. (2021). A machine learning approach for modeling and optimization of a CO2 post-combustion capture unit. Energy 215, 119113. doi:10.1016/j.energy.2020.119113
Shi, Z., Yang, W., Deng, X., Cai, C., Yan, Y., Liang, H., et al. (2020). Machine-learning-assisted high-throughput computational screening of high performance metal-organic frameworks. Mol. Syst. Des. Eng. 5, 725–742. doi:10.1039/D0ME00005A
Situ, Y., Yuan, X., Bai, X., Li, S., Liang, H., Zhu, X., et al. (2022). Large-scale screening and machine learning for metal-organic framework membranes to capture CO2 from flue gas. Membranes 12, 700. doi:10.3390/membranes12070700
Song, Z., Shi, H., Zhang, X., and Zhou, T. (2020). Prediction of CO2 solubility in ionic liquids using machine learning methods. Chem. Eng. Sci. 223, 115752. doi:10.1016/j.ces.2020.115752
Sreńscek-Nazzal, J., and Kiełbasa, K. (2019). Advances in modification of commercial activated carbon for enhancement of CO2 capture. Appl. Surf. Sci. 494, 137–151. doi:10.1016/j.apsusc.2019.07.108
Sun, W., Lin, L., Peng, X., and Smit, B. (2014). Computational screening of porous metal-organic frameworks and zeolites for the removal of SO2 and NOx from flue gases. AIChE J. 60, 2314–2323. doi:10.1002/aic.14467
Tabor, D., Roch, L., Saikin, S., Kreisbeck, C., Sheberla, D., Montoya, J., et al. (2018). Accelerating the discovery of materials for clean energy in the era of smart automation. Nat. Rev. Mat. 3, 5–20. doi:10.1038/s41578-018-0005-z
Venkatraman, V., and Alsberg, B. (2017). Predicting CO2 capture of ionic liquids using machine learning. J. CO2 Util. 21, 162–168. doi:10.1016/j.jcou.2017.06.012
Wang, J., Song, Z., Chen, L., Xu, T., Deng, L., and Qi, Z. (2021). Prediction of CO2 solubility in deep eutectic solvents using random forest model based on COSMO-RS-derived descriptors. Green Chem. Eng. 2, 431–440. doi:10.1016/j.gce.2021.08.002
Wang, K., Xu, H., Yang, C., and Qiu, T. (2021). Machine learning-based ionic liquids design and process simulation for CO2 separation from flue gas. Green Energy & Environ. 6, 432–443. doi:10.1016/j.gee.2020.12.019
Wang, Q., Luo, J., Zhong, Z., and Borgna, A. (2011). CO2 capture by solid adsorbents and their applications: Current status and new trends. Energy Environ. Sci. 4, 42–55. doi:10.1039/C0EE00064G
Wang, Z., Zhou, T., and Sundmacher, K. (2022a). Interpretable machine learning for accelerating the discovery of metal-organic frameworks for ethane/ethylene separation. Chem. Eng. J. 444, 136651. doi:10.1016/j.cej.2022.136651
Wang, Z., Zhou, Y., Zhou, T., and Sundmacher, K. (2022b). Identification of optimal metal-organic frameworks by machine learning: structure decomposition, feature integration, and predictive modeling. Comput. Chem. Eng. 160, 107739. doi:10.1016/j.compchemeng.2022.107739
Wu, Y., Liang, Y., and Wang, H. (2021). Heterogeneous molecular catalysts of metal phthalocyanines for electrochemical CO2 reduction reactions. Acc. Chem. Res. 54, 3149–3159. doi:10.1021/acs.accounts.1c00200
Yamada, H. (2021). Amine-based capture of CO2 for utilization and storage. Polym. J. 53, 93–102. doi:10.1038/s41428-020-00400-y
Yang, Z., Li, H., Yang, J., Feng, S., Liu, X., Zhao, J., et al. (2019). Nanosized copper selenide functionalized zeolitic imidazolate framework-8 (CuSe/ZIF-8) for efficient immobilization of gas-phase elemental mercury. Adv. Funct. Mat. 29, 1807191. doi:10.1002/adfm.201807191
Yarveicy, H., Ghiasi, M., and Mohammadi, A. (2018). Performance evaluation of the machine learning approaches in modeling of CO2 equilibrium absorption in piperazine aqueous solution. J. Mol. Liq. 255, 375–383. doi:10.1016/j.molliq.2017.11.156
Yuan, Q., Longo, M., Thornton, A., McKeown, N., Comesaña-Gándara, B., Jansen, J., et al. (2021). Imputation of missing gas permeability data for polymer membranes using machine learning. J. Membr. Sci. 627, 119207. doi:10.1016/j.memsci.2021.119207
Yuan, X., Suvarna, M., Low, S., Dissanayake, P., Lee, K., Li, J., et al. (2021). Applied machine learning for prediction of CO2 adsorption on biomass waste-derived porous carbons. Environ. Sci. Technol. 55, 11925–11936. doi:10.1021/acs.est.1c01849
Zarei, A., Hafizi, A., Rahimpour, M., and Raeissi, S. (2020). Carbon dioxide absorption into aqueous potassium salt solutions of glutamine amino acid. J. Mol. Liq. 301, 111743. doi:10.1016/j.molliq.2019.111743
Zeng, S., Zhang, X., Bai, L., Zhang, X., Wang, H., Wang, J., et al. (2017). Ionic-liquid-based CO2 capture systems: Structure, interaction and process. Chem. Rev. 117, 9625–9673. doi:10.1021/acs.chemrev.7b00072
Zhang, K., Wu, J., Yoo, H., and Lee, Y. (2021). Machine learning-based approach for tailor-made design of ionic liquids: Application to CO2 capture. Sep. Purif. Technol. 275, 119117. doi:10.1016/j.seppur.2021.119117
Zhang, X., Ding, X., Song, Z., Zhou, T., and Sundmacher, K. (2021a). Integrated ionic liquid and rate-based absorption process design for gas separation: Global optimization using hybrid models. AIChE J. 67, e17340. doi:10.1002/aic.17340
Zhang, X., Wang, J., Song, Z., and Zhou, T. (2021b). Data-driven ionic liquid design for CO2 capture: Molecular structure optimization and DFT verification. Ind. Eng. Chem. Res. 60, 9992–10000. doi:10.1021/acs.iecr.1c01384
Zhang, X., Zhang, K., Yoo, H., and Lee, Y. (2021c). Machine learning-driven discovery of metal-organic frameworks for efficient CO2 capture in humid condition. ACS Sustain. Chem. Eng. 9, 2872–2879. doi:10.1021/acssuschemeng.0c08806
Zhang, X., Zhou, T., and Sundmacher, K. (2022). Integrated metal-organic framework and pressure/vacuum swing adsorption process design: Descriptor optimization. AIChE J. 68, e17524. doi:10.1002/aic.17524
Zhang, Z., Cao, X., Geng, C., Sun, Y., He, Y., Qiao, Z., et al. (2022). Machine learning aided high-throughput prediction of ionic liquid@MOF composites for membrane-based CO2 capture. J. Membr. Sci. 650, 120399. doi:10.1016/j.memsci.2022.120399
Zhou, K., Ma, W., Zeng, Z., Ma, X., Xu, X., Guo, Y., et al. (2019). Experimental and DFT study on the adsorption of VOCs on activated carbon/metal oxides composites. Chem. Eng. J. 372, 1122–1133. doi:10.1016/j.cej.2019.04.218
Zhou, T., Song, Z., and Sundmacher, K. (2019). Big data creates new opportunities for materials research: A review on methods and applications of machine learning for materials design. Engineering 5, 1017–1026. doi:10.1016/j.eng.2019.02.011
Keywords: CO2, carbon capture, machine learning, metal organic framework, flue gas
Citation: Yang Z, Chen B, Chen H and Li H (2023) A critical review on machine-learning-assisted screening and design of effective sorbents for carbon dioxide (CO2) capture. Front. Energy Res. 10:1043064. doi: 10.3389/fenrg.2022.1043064
Received: 13 September 2022; Accepted: 17 November 2022;
Published: 06 January 2023.
Edited by:
Uwe Schröder, University of Greifswald, GermanyReviewed by:
Ali Bakhtyari, Shiraz University, IranTeng Zhou, Hong Kong University of Science and Technology, Hong Kong SAR, China
Copyright © 2023 Yang, Chen, Chen and Li. This is an open-access article distributed under the terms of the Creative Commons Attribution License (CC BY). The use, distribution or reproduction in other forums is permitted, provided the original author(s) and the copyright owner(s) are credited and that the original publication in this journal is cited, in accordance with accepted academic practice. No use, distribution or reproduction is permitted which does not comply with these terms.
*Correspondence: Hailong Li, hailongli18@gmail.com