- 1School of Management, Zhengzhou University, Zhengzhou, China
- 2Logistics Engineering College, Shanghai Maritime University, Shanghai, China
- 3China Institute of FTZ Supply Chain, Shanghai Maritime University, Shanghai, China
- 4School of Business, Guilin University of Electronic Technology, Guilin, China
- 5Henan University of Economics and Law, Zhengzhou, China
Papers and patents can respectively present the latest progress of scientific research and technological development. Combining the two for correlation analysis has certain reference significance for technology opportunity discovery. Therefore, this paper proposes a method for technology opportunity discovery of proton exchange membrane fuel cell from the perspective of papers-patents correlation analysis. Firstly, papers and patent data are collected from papers and patent databases and the LDA model is applied to extract paper and patent topics. Secondly, the indicators of topic heat and novelty are calculated to select high-value paper topics. After that, the similarity analysis between high-value paper topics and all patent topics is considered to select the patent topics with development potential. Finally, the patent texts with high relevance to these patent topics with development potential are positioned. The technical keywords in them are extracted by text mining tool and classified into innovation dimensions according to their attributes and the TEMPEST model. After that, the innovation rules in the SCAMPER model are introduced to combine with the technical keywords in each innovation dimension to identify specific technology opportunities. An empirical study on the technology of proton exchange membrane fuel cell provides sufficient evidence of the method’s ability to discover technology opportunities. This paper contributes by generating four specific technology opportunities, which can make up for the existing defects of proton exchange membrane fuel cells and provide useful guidance for companies to carry out technological innovation.
1 Introduction
In recent years, the rapid development of the industrial industry makes the problem of global energy shortage more prominent. Besides, rapid urbanization and increased fossil fuel consumption have led to excessive greenhouse gas emissions, which pose a major threat to the global environment (Yu et al., 2022). With the advantages of high energy density and high energy conversion efficiency, the proton exchange membrane fuel cell (PEMFC) is regarded as a promising power source by industry and academia (Ning et al., 2022). Its wide application can help improve the energy shortage problem and contribute to the sustainable development of the environment. However, there are still some defects in its development process, such as poor durability, high operating cost and insufficient power density (Yanyan et al., 2022). For some related enterprises and R&D personnel, the accurate and efficient development of technology innovation activities in the field of PEMFC can quickly improve the product and promote the development of fuel cells. Therefore, more and more experts and scholars are committed to the research of technological innovation in the field of PEMFC and have achieved fruitful results (Park & Geum, 2022).
In the relevant literature, since the rich technological information and cutting-edge information contained in patents, and the advantages of massive data and standardized format (Seo, 2022; Zhao et al., 2022), it is regarded by scholars as a common data source for technology opportunity discovery (TOD) in the field of PEMFC. In recent years, many scholars have conducted extensive research on how to use patent analysis to identify technology opportunities in the field of PEMFC. A framework was proposed that combines the state-of-the-art meta-heuristic algorithm and machine learning method for the performance prediction and parameter optimization of PEMFC (Hong-Wei et al., 2022). The LDA model and LOF algorithm were used to construct a structured TOD framework with a multi-level identification process and then identify technology opportunities for fuel cells (Shi et al., 2019). In addition, SAO network analysis was applied to solve the shortcoming of the keyword-based approach to identify technological trends and analyze the development trends of polymer electrolyte membrane technology in the field of PEMFC (Choi et al., 2011). Patent analysis was integrated with visual tools such as generative topographic mapping (GTM) to identify technology trends and potential technology opportunities in the field of PEMFC (Teng et al., 2021).
However, the current research on TOD methods in the field of PEMFC through patent analysis still has some limitations. Firstly, most of the existing studies focus on the time delay calculation method of multi-source scientific literature. Patents obtained by this method often lag behind papers, which leads to a time difference of 3–4 years between technical research and scientific research (Finardi, 2011). In addition, relying only on patent analysis to identify technology opportunities in the field of PEMFC may lead to the omission of important recent information, which may affect the accuracy of the identification results. Secondly, technical topics or keywords are often used in existing research to represent technology opportunities in the field of PEMFC at a coarse-grained level (Lee et al., 2022), which may make it difficult to provide relevant enterprises with highly operable fine-grained guidance for technological innovation activities.
Therefore, this paper proposes a method for TOD in the field of PEMFC from the perspective of papers-patents correlation analysis. Besides, the TEMPEST model and SCAMPER model are introduced to provide a basis for selecting innovation dimensions and rules to identify specific technology opportunities. Firstly, the LDA model is applied to extract relevant topics from papers and patent datasets. Secondly, quantitative indicators are used to select the high-value paper topics, and then the patent topics with development potential are selected by calculating the cosine similarity between the high-value paper topics and all patent topics. Thirdly, the document-topic probability matrix in the LDA model is combined to locate patent texts with high relevance to patent topics with development potential, and the text mining method is conducted to extract technical keywords from these patent texts and divide them into innovation dimensions. Finally, the innovation rules are introduced to generate technology opportunities and describe them in detail. In summary, the path of TOD constructed in this paper can help make up for the existing defects in the field of PEMFC, and then help related enterprises to carry out technological innovation activities.
This study is composed as follows. Section 2 reviews the literature on technology opportunity discovery and papers-patents correlation analysis. Section 3 explains the research framework and process in this study. Section 4 analyzes the identification process and results of PEMFC. Sections 5, 6 provide discussions and conclusions of the research by including contributions and directions for future study.
2 Literature review
2.1 Technology opportunity discovery
TOD refers to the process that collects technical information and transforms it into a form that can be analyzed by companies and R&D personnel to explore new technology opportunities (Russo et al., 2020). As the premise and critical step of technological innovation, technology opportunity identification has increasingly attracted the attention of many scholars (Jinfeng et al., 2022; Teng et al., 2021). In recent years, with the rapid increase of data information, mining the technology opportunities behind massive data through scientific quantitative analysis methods has become a research topic of common concern to academia and industry.
Many scholars have conducted extensive research on the identification of technology opportunities by using quantitative analysis methods. The relative importance of functions in the technical field was analyzed to identify the development trend of technologies in specific fields (Mun et al., 2021). The K-means algorithm was applied to cluster the keyword vectors and visualize them in the generated topographic map, which explored the development direction of technology in specific fields (Teng et al., 2021). Combining technology and services, a technology service opportunity identification method based on business method patent analysis was proposed and applied to the field of mobile services (Kim & Lee, 2020). Therefore, TOD has been widely used and made many breakthroughs.
However, although previous studies focused on extracting and analyzing critical information from patents to discover technology opportunities, there are still some limitations. Firstly, relying only on patent analysis to identify technology opportunities may lead to the omission of important recent information, which may affect the accuracy of the identification results. Secondly, the technology opportunities identified by most previous methods are often represented by technical topics or keywords. It may not be specific enough to provide specific innovation guidance for companies and R&D personnel. Therefore, an innovation path for TOD is constructed in this paper from the perspective of papers-patents correlation analysis. Besides, the TEMPEST model and SCAMPER model are applied to help identify more precise technology opportunities in the field of PEMFC.
2.2 Papers-patents correlation analysis
Papers are the main output form of achievements in scientific research, and patents are the main carrier of the core content of technology research and development (Kim et al., 2017; Choi et al., 2019). The two respectively represent the latest progress in scientific research and technological development (Xu et al., 2020). Analyzing the relationships between them is of great significance for mining and identifying technology opportunities.
Some scholars believe that the scientific research represented by papers has a certain role in promoting technological research and development represented by patents. A knowledge network coupling method was proposed to compare the coupling strength between science and technology networks, and conclude that scientific development is ahead of technological progress (Ba & Liang, 2021). As a direct form of linkage, citations confirmed and illustrated the social character of knowledge diffusion and transfer between science and technology (Li et al., 2017). In addition, many scholars have conducted in-depth research on the identification of cutting-edge topics based on the correlation analysis of papers and patents. Through bibliometric analysis of related topics of science and technology, an identification method of innovation path based on topics correlation was constructed. Papers and Patents were applied together to effectively identify important topics related to science and technology in the evolution process of genetically engineered vaccines (GEVs) field (Xu et al., 2020).
Therefore, based on the perspective of correlation analysis between papers and patents, paper and patent topics are extracted and high-value paper topics are selected in this paper, and then similarity analysis is applied to select out patent topics with development potential. After that, technology opportunities are identified in combination with innovation rules. It can provide guidance for relevant enterprises to accurately identify technology opportunities and efficiently carry out technological innovation activities.
3 Data and methods
The research framework of this paper is proposed, as shown in Figure 1. Firstly, the paper and patent database are used to collect paper and patent data in the target domain, and the LDA model is applied to extract paper and patent topics respectively. Secondly, the indicators of topic novelty and topic heat are constructed to select the high-value paper topics, and the cosine similarity between the high-value paper topics and patent topics is calculated to select the patent topics with development potential. Finally, the document-topic probability matrix of the LDA model is conducted to locate patent texts related to patent topics with development potential, and Natural Language Toolkit (NLTK) algorithm in python is introduced to extract technical keywords from these patent texts, and then identify technology opportunities in the target domain in combination with innovation dimensions and rules.
3.1 Data collection and topic extraction
3.1.1 Collecting and preprocessing paper and patent data
Firstly, the papers and patent databases are determined, and the target domain retrieval schemes are formulated in combination with expert opinions to collect papers and patent data. Secondly, the paper and patent data with low correlation are eliminated by data cleaning and screening. Finally, the paper title, abstract, introduction and conclusion with rich scientific information are extracted as the final paper data set, and the patent title, abstract and claim with rich technical information are extracted as the final patent data set.
3.1.2 Extracting paper topics and patent topics
The obtained papers and patent datasets based on the above steps are usually presented in text form. Since the two datasets contain a large amount of scientific and technological information, it is difficult to obtain critical information directly from them. Therefore, text mining methods need to be applied to extract scientific and technological information and prepare for subsequent data analysis. Besides, the LDA model has the advantages of high speed and good clustering effect when dealing with massive text, and can directly get the text processing results of “document-topic-feature word” (Ligorio et al., 2022; Venugopalan & Gupta, 2022). In this way, the LDA model is conducted in this paper to extract the paper and patent topics in the paper and patent datasets respectively. The specific extraction steps of paper and patent topics are as follows.
Firstly, the relevant literature in the target field should be collected, and then the domain expert knowledge is combined to build a related custom lexicon and a stop word lexicon. Secondly, the confusion curve is used to determine the topic number K of the LDA model, and the hyperparameters α and β of the LDA model are set. Thirdly, run the LDA model and extract the paper topics and patent topics from the paper and patent dataset respectively. Finally, repeat the above steps, remove the interference words in the extraction results of the LDA model to improve the accuracy of the model, and then obtain the paper and patent topics.
3.2 Selection of paper topics and patent topics
3.2.1 Selecting high-value paper topics
Based on the extracted paper topics, it is necessary to further calculate their feature values to guide the selection of high-value paper topics. According to the previous research results, the indicators of topic novelty and topic heat (Rathinasamy & Kandhasamy, 2022) are calculated in this paper to evaluate the value of each paper topic.
3.2.1.1 Topic novelty indicator
It is generally believed that recently published papers have a high degree of novelty. Therefore, the average publication time of related papers containing a topic is used in this paper to measure the novelty of this paper topic. The calculation formula is as follows.
In Eq. 1,
3.2.1.2 Topic heat indicator
It is generally believed that the topics that are studied more frequently in a certain period have higher heat. Therefore, this paper uses the studied number of related papers containing a topic in a certain period to measure the heat of this paper topic. The calculation formula is as follows.
In Eq. 2,
3.2.1.3 Normalized processing
The results obtained by directly calculating the above two indicators may have a large quantitative level gap. Therefore, the positive deviation standardization method is adopted to standardize the two indicator values to improve the accuracy of the selection results of high-value paper topics.
In Eq. 3,
Based on the above analysis, the selection process of high-value paper topics includes the following steps. Firstly, Eqs 1, 2 are combined to calculate the novelty and heat indicator values of each paper topic, and then Eq. 3 is used to normalize the above indicators. Secondly, the CRITIC method is introduced to objectively weigh the novelty and heat indicators of paper topics to comprehensively judge the value of paper topics (Zhang et al., 2022). In addition, the CRITIC method is a weighting method that assigns objective weights to indicators by calculating their contrast strength and conflict (Li & Wang, 2020; Song et al., 2021). It can improve the objectivity of the selection results of high-value paper topics. Finally, based on the obtained objective weights, the composite index of the two indicators is calculated and the high-value paper topics are determined.
3.2.2 Selecting patent topics
It is also necessary to analyze the similarity between high-value paper topics and patent topics. In addition, to improve the accuracy of the selection results of patent topics. The details include the following steps:
Some scholars believe that drawing on the views of existing research, this paper argues that the scientific research represented by papers has a certain role in promoting the technological development represented by patents (Ba & Liang, 2021). Thus, the high-value paper topics are applied in this paper to guide the selection of patent topics with development potential. From the perspective of paper-patent correlation analysis, the patent topics with development potential are screened by calculating the cosine similarity between the high-value paper topics and patent topics. In this way, the accuracy of the selection results of patent topics can be improved. The selection process of patent topics with development potential is as follows.
Firstly, the Word2Vec model is conducted to construct the word vector space of paper and patent data. As a word vector tool, the Word2Vec model can not only map words from high-dimensional space to low-dimensional space, but also retain the existing position between word vectors to solve the problem of vector sparseness and semantic connection (Ma et al., 2023). Secondly, the cosine similarity between the vectors corresponding to the high-value paper topics and the patent topics is calculated to obtain the topic similarity matrix. Finally, domain expert opinions are combined to set similarity thresholds and screen out patent topics with development potential.
3.3 Location of relevant patents and technology opportunities discovery
The patent topics with development potential selected above are considered as innovation-oriented in this paper to identify technology opportunities. Therefore, it is necessary to fully interpret the patent texts related to these patent topics, analyze the existing technical keywords, and combine the innovation dimensions in the TEMPEST model and the innovation rules in the SCAMPER model to identify technology opportunities. The specific process of the location of relevant patents and technology opportunity identification is as follows.
Firstly, the document-topic probability matrix of the LDA model is used to locate patent texts that are highly relevant to patent topics with development potential. It should be noted that this paper mainly focuses on the patent texts with the highest probability value under each patent topic. Secondly, the NLTK algorithm is applied to extract technical keywords in patent texts that are mainly concerned. Finally, the TEMPEST model is conducted to divide the innovation dimensions of technical keywords, and it also needs to combine their attribute features. Specifically, the TEMPEST model is defined as one of the methods that classify patents and qualitatively analyze technological information from various perspectives (Jeong & Yoon, 2015). The combination of various dimensions deeply reflects the mechanism of the operation of the technical system. Besides, how to restructure and innovate technical information represented by keywords to generate specific technology opportunities is an important issue to be answered. The SCAMPER model is an approach to stimulate the generation of new ideas (Jeong & Yoon, 2015). It is an abbreviation of seven different words, namely S (Substitute), C (Combine), A (Adapt), M (Modify), P (Put to other use), E (Eliminate), and R (Rearrange, Reverse). This paper makes coupling innovation of keywords in each innovation dimension based on the combination of the SCAMPER model and expert experience to generate a series of technology opportunities. It can guide enterprises to use limited resources to accurately and efficiently carry out technological innovation.
4 Results
As a clean, efficient and renewable power generation device, the fuel cell can directly convert the chemical energy of fuel into electricity. Thus, it is called the ultimate solution to achieve zero emissions. In recent years, fuel cell has been widely applied in aerospace (Baroutaji et al., 2019), transportation (Dash et al., 2022), emergency (Sun & Li, 2021) or uninterruptible power supply (Cheng et al., 2022), and other fields (Wang et al., 2022).
Among different types of fuel cells, PEMFC not only has high energy density and high energy conversion efficiency, but also has the advantages of green, low noise, low thermal radiation and zero pollution (Li et al., 2022; Sanzi López et al., 2022). Therefore, it is considered to be an important path to completely solve the problem of environmental pollution and energy shortage in China. However, the existing products of PEMFC have some defects such as poor durability, high operating cost, and insufficient power density (Yanyan et al., 2022), which makes it difficult to meet the needs of energy storage application scenarios. In this way, it is necessary to carry out technological innovation activities in the field of PEMFC.
Therefore, the constructed path of TOD based on paper-patent correlation analysis is systematically applied in this paper to explore the technology opportunities in the field of PEMFC. It can not only verify the feasibility of the path constructed, but also guide relevant enterprises to carry out technological innovation in the field of PEMFC accurately and efficiently to promote the breakthrough development of China’s new fuel cell energy storage industry.
4.1 Data collection and topic extraction in the field of PEMFC
4.1.1 Collecting and preprocessing paper and patent data in the field of PEMFC
The CNKI (https://www.cnki.net) and Baiteng (https://www.baiten.cn) are used in this paper to respectively collect paper and patent data in the field of PEMFC. In addition, the collected data are cleaned and preprocessed to obtain paper dataset and patent dataset that can be applied for further data analysis. The specific process of the collection and preprocessing of paper and patent data in the field of PEMFC is as follows.
Firstly, literature research and expert opinions in the field of PEMFC are combined to determine the paper retrieval scheme in this field, as shown in Table 1. After searching, 2,748 related papers were collected. In addition, through preprocessing such as data cleaning and screening, data with low correlation with topics in the field of PEMFC were eliminated, and finally 316 papers were obtained. The information extracted from the paper title, abstract, introduction and conclusion can be used as the final paper dataset in the field of PEMFC.
Secondly, literature research and expert opinions in the field of PEMFC are combined to determine the patent retrieval scheme in this field, as shown in Table 2. After searching, 4,695 related patents were collected. In addition, through preprocessing such as data cleaning and screening, data with low correlation with topics in the field of PEMFC were eliminated, and finally 991 patents were obtained. The information extracted from the patent title, abstract and claim can be used as the final patent dataset in the field of PEMFC.
4.1.2 Extracting paper topics and patent topics in the field of PEMFC
The LDA model is applied to extract paper and patent topics in the field of PEMFC from the final paper dataset and patent dataset. However, several important parameter values need to be set before running the LDA model. Among them, the hyperparameters can be set to α = 50/K, β = 0.01 (Sakshi & Vinay, 2023), and the number of topics K is determined by calculating the confusion value of different topics (Wahid et al., 2022).
Firstly, the perplexity values corresponding to the number of different paper topics in the field of PEMFC are calculated. The calculation results are shown in Figure 2.
It is not difficult to be seen from Figure 2 that when the number of paper topics is 14, the corresponding perplexity value is the smallest. Therefore, the number of paper topics is determined as 14 in this paper and the corresponding paper topics and feature words list are extracted. The extraction results of paper topics and feature words list in the field of PEMFC are shown in Table 3.
Secondly, the confusion value corresponding to the number of different patent topics in the field of PEMFC is calculated. The calculation results are as shown in Figure 3.
It is not difficult to be seen from Figure 3 that when the number of patent topics is 14, the corresponding perplexity value is the smallest. Therefore, the number of patent topics is determined as 14 in this paper and the corresponding patent topics and feature words list are extracted. The extraction results of patent topics and feature words list in the field of PEMFC are as shown in Table 4.
4.2 Selection of paper topics and patent topics in the field of PEMFC
4.2.1 Selecting high-value paper topics in the field of PEMFC
Based on the above analysis, the two indicators of topic novelty and topic heat are combined to comprehensively measure the value of paper topics in the field of PEMFC, and then select high-value paper topics. The specific selection process of high-value paper topics is shown as follows.
Firstly, Eqs 1, 2 are used to respectively calculate the novelty and heat values of the paper topics in the field of PEMFC, and then Eq. 3 is applied to normalize the obtained two indicator values. Secondly, the CRITIC method is introduced to objectively assign certain weights to the two indicators. After calculation, the weight of the novelty indicator of paper topics in the field of PEMFC is 46.16%, and the weight of the heat indicator of paper topics in the field of PEMFC is 53.84%. Therefore, the ranking results of the paper topics’ value in the field of PEMFC are obtained. The calculation results are shown in Table 5.
Finally, combined with the views of experts in the field of PEMFC, the top 3 high-value paper topics are selected, and then the names of these paper topics are summed up by reading the literature corresponding to each topic in the field of PEMFC. The selection results of high-value paper topics are shown in Table 6.
It can be seen from Table 6 that the three paper topics of hydrogen storage technology, stack cooling system and hydrogen-oxygen coupling process are considered as high-value paper topics, which can provide guidance for the selection of paper topics with development potential.
4.2.2 Selecting patent topics in the field of PEMFC
The high-value paper topics and the extracted patent topics are combined and the cosine similarity between them is calculated to screen out the patent topics with high similarity and development potential in the field of PEMFC. The specific selection process of patent topics with development potential is shown as follows.
Firstly, the Word2Vec model is applied to construct a vector space of high-value paper topics and patent topics in the field of PEMFC, and then the cosine similarity between their corresponding vectors is calculated. The calculation results are shown in Table 7.
Secondly, drawing on the existing research, when the cosine similarity is not less than 0.3, it shows that there is a strong correlation between the paper topics and the patent topics (Quanqing et al., 2022). Therefore, the top 10% of patent topics with a cosine similarity greater than 0.3 to the paper topic are determined in this paper as patent topics with development potential in the field of PEMFC. After screening, the patent topics with development potential in the field of PEMFC are T3, T5, T13, and T16. The related patent text information is combined to name these patent topics with development potential. It can be seen from Table 8 that the patent topics with development potential in the field of PEMFC are fuel cell cooling systems, air compressors, safety control system and hydrogen supply systems.
4.3 Location of relevant patents and technology opportunities discovery in the field of PEMFC
4.3.1 Locating relevant patents in the field of PEMFC
Based on the above selected patent topics with development potential, the document-topic probability matrix in the analysis results of the LDA model is conducted to locate the patent texts with high relevance to these selected patent topics in the field of PEMFC. Besides, the patents with the highest probability in the document-topic probability matrix are selected and analyzed as patent texts closely related to these patent topics. The location results of relevant patents in the field of PEMFC are shown in Table 9.
4.3.2 Extracting technical keywords and dividing innovation dimensions in the field of PEMFC
The NLTK algorithm is applied to extract the technical keywords from the patent texts in the field of PEMFC, and the innovation dimension of each technical keyword is divided according to the TEMPEST model and their attribute features. The specific process of technical keywords and dimension division in the field of PEMFC are shown as follows.
Firstly, the jieba toolkit in Python is used to segment the text content of related patent titles, abstracts and claims under each patent topic in the field of PEMFC. Secondly, the quantifiers, modal words and other meaningless words are removed by combining with literature and common stop words list, and then the custom stop words dictionary in the field of PEMFC is constructed.
Thirdly, the NLTK algorithm is run and technical keywords under each patent topics are extracted. Finally, according to the TEMPEST model and the conceptual properties of the technical keywords in the field of PEMFC, the extracted technical keywords are divided into four dimensions, including function dimension, structure dimension, material dimension and mechanism dimension. The extraction results of technical keywords and the division results of innovation dimension in the field of PEMFC are shown in Table 10.
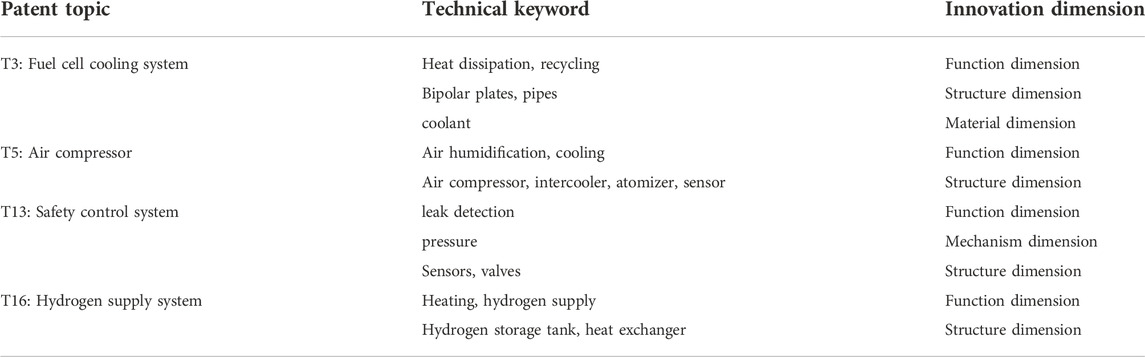
TABLE 10. Extraction results of technical keywords and the division results of innovation dimension in the field of PEMFC.
4.3.3 Identifying technology opportunities in the field of PEMFC
Based on the above analysis, the SCAMPER model is introduced to combine with the technical keywords in the innovation dimensions to generate and specifically describe the four technology opportunities in the field of PEMFC under each patent topic. The identification results of technology opportunities in the field of PEMFC are shown in Table 11.
5 Discussion
It should be noted that the technology opportunities identified in this paper combined the knowledge and experience of experts in the field of technological innovation and new energy, which can improve the operability of technological innovation in the field of PEMFC.
Taking technology opportunity 1 in Table 11 as an example, the specific description of this technology opportunity is as follows. First of all, the heat dissipation material is coated on the bipolar plate to cover the closed cavity injected with the coolant. It can not only improve the heat dissipation efficiency of PEMFC, but also quickly discharge the internal heat of the fuel cell to efficiently improve the durability of PEMFC. Secondly, recycling the coolant in the process of heat dissipation can not only reduce the consumption of the coolant, but also avoid frequent usage of coolant injection in the subsequent process to greatly reduce the operating cost of PEMFC. Thirdly, the heat dissipation pipeline of the intercooler is connected to the fuel cell exhaust pipeline. In other words, a heat dissipation water vapor discharge path is designed. It can not only quickly discharge the generated water vapor to improve the safety of the battery operation, but also improve the operation efficiency of PEMFC to effectively avoid defects such as insufficient power density.
Based on the above analysis, the technology opportunities identified in this paper can accurately and efficiently solve the existing problems of poor durability, high operating cost and insufficient power density in the development of the PEMFC field, which has certain innovation and feasibility. In addition, it can provide the useful reference for enterprises to avoid the risk of blind innovation and provide new ideas for the rapid development of the PEMFC industry.
6 Conclusion
This paper proposes an innovation path for TOD from the perspective of paper-patent correlation analysis, and takes the field of PEMFC as an empirical study to verify the feasibility of the identification path of technology opportunities. It can provide new ideas for enterprises to carry out technological innovation efficiently, and has certain theoretical and practical significance.
The contributions of this study are as follows. From the aspect of methodology, firstly, the similarity between high-value paper topics and patent topics is calculated in this paper to filter the patent topics with development potential and identify technological opportunities in the field of PEMFC. It can not only break through the limitations of identifying technology opportunities based on a single source of patent data, but also improve the accuracy of the identification results. Secondly, the innovation dimensions and rules are applied in this paper to identify more concrete technology opportunities in the field of PEMFC. It can not only solve the problems of vagueness and coarse-grained that may be caused by explaining technology opportunities mainly by technology topics or keywords, but also improve the operability of the identification results of technology opportunities. From the perspective of application, the method proposed in this paper can provide an objective and comprehensive reference for new energy enterprises to analyze technology opportunities. In addition, the identified technology opportunities in this paper can provide relevant enterprises with innovative improvement directions for the PEMFC field, thus contributing to global energy efficiency and environmental protection.
Despite the contribution, this study has some limitations, and further study is required. Firstly, in this paper, only a single product of PEMFC is selected for empirical study, which may lead to the lack of comprehensive conclusion. In the future, the proposed path of TOD should be applied to study technological innovation in more fields. Secondly, in the process of extracting technical keywords from patents, this paper does not fully consider the different importance of technical keywords that may be caused by the different funds and R&D time of each patent. In the future, more factors affecting the importance of technical keywords should be considered in order to extract keywords more accurately.
Data availability statement
The original contributions presented in the study are included in the article/Supplementary Material, further inquiries can be directed to the corresponding author.
Author contributions
LF: Conceptualization, Methodology, Resources. KL: Data Curation, Writing—Original Draft. JW: Supervision, Funding acquisition. K-YL: Methodology, Writing—Review and Editing. KZ: Formal analysis, Investigation. LZ: Software, Validation.
Funding
This research was funded by Innovation Method Fund of China, grant numbers 2018IM020300, 2019IM020200; Joint Funds of the National Natural Science Foundation of China, grant number U1904210-4; Shanghai Science and Technology Program, grant number 20040501300; National Key Research and Development Program, grant number 2022YFF0608700; Research Subject of Federation of Social Sciences for Henan Province, grant number SKL-2022–2312 and National Natural Science Foundation of China, grant number 62173253.
Conflict of Interest
The authors declare that the research was conducted in the absence of any commercial or financial relationships that could be construed as a potential conflict of interest.
Publisher’s note
All claims expressed in this article are solely those of the authors and do not necessarily represent those of their affiliated organizations, or those of the publisher, the editors and the reviewers. Any product that may be evaluated in this article, or claim that may be made by its manufacturer, is not guaranteed or endorsed by the publisher.
References
Ba, Z., and Liang, Z. (2021). A novel approach to measuring science-technology linkage: From the perspective of knowledge network coupling. J. Inf. 15 (3), 101167. doi:10.1016/j.joi.2021.101167
Baroutaji, A., Wilberforce, T., Ramadan, M., and Olabi, A. G. (2019). Comprehensive investigation on hydrogen and fuel cell technology in the aviation and aerospace sectors. Renew. Sustain. Energy Rev. 106, 31–40. doi:10.1016/j.rser.2019.02.022
Cheng, Y., Zhang, Y., and Chen, Q. (2022). Energy management strategy of fuel-cell Backup power supply systems based on Whale optimization Fuzzy control. Electronics 11 (15), 2325. doi:10.3390/electronics11152325
Choi, J., Jeong, B., and Yoon, J. (2019). Technology opportunity discovery under the dynamic change of focus technology fields: Application of sequential pattern mining to patent classifications. Technol. Forecast. Soc. Change 148, 119737. doi:10.1016/j.techfore.2019.119737
Choi, S., Yoon, J., Kim, K., Jae, Y. L., and Kim, C.-Han (2011). SAO network analysis of patents for technology trends identification: A case study of polymer electrolyte membrane technology in proton exchange membrane fuel cells. Scientometrics 88 (3), 863–883. doi:10.1007/s11192-011-0420-z
Dash, S. K., Chakraborty, S., Roccotelli, M., and Sahu, U. K. (2022). Hydrogen fuel for future Mobility: Challenges and future aspects. Sustainability 14 (14), 8285. doi:10.3390/su14148285
Finardi, U. (2011). Time relations between scientific production and patenting of knowledge: The case of nanotechnologies. Scientometrics 89 (1), 37–50. doi:10.1007/s11192-011-0443-5
Hong-Wei, L., Bin-Xin, Q., Jun-Nan, L., Yue, Y., Wenxuan, F., and Guo-Long, L. (2022). A data-driven framework for performance prediction and parameter optimization of a proton exchange membrane fuel cell. Energy Convers. Manag., 271. 116338. doi:10.1016/j.enconman.2022
Jeong, Y., and Yoon, B. (2015). Development of patent roadmap based on technology roadmap by analyzing patterns of patent development. Technovation 39-40, 37–52. doi:10.1016/j.technovation.2014.03.001
Jinfeng, W., Kang, L., and Lijie, F. (2022). Tracing the technological trajectory of coal slurry pipeline transportation technology: An HMM-based topic modeling approach. Frontiers in Energy Research. doi:10.3389/FENRG.2022.974747
Kim, B., Gazzola, G., Yang, J., Lee, J.-M., Coh, B.-Y., Jeong, M. K., et al. (2017). Two-phase edge outlier detection method for technology opportunity discovery. Scientometrics 113 (1), 1–16. doi:10.1007/s11192-017-2472-1
Kim, C., and Lee, H. (2020). A patent-based approach for the identification of technology-based service opportunities. Comput. Industrial Eng. 144, 106464. doi:10.1016/j.cie.2020.106464
Lee, M., Kim, S., Kim, H., and Lee, J. (2022). Technology opportunity discovery using deep learning-based text mining and a knowledge Graph. Technol. Forecast. Soc. Change 180, 121718. doi:10.1016/j.techfore.2022.121718
Li, D., Azoulay, P., and Sampat, B. N. (2017). The applied value of public investments in biomedical research. Science 356 (6333), 78–81. doi:10.1126/science.aal0010
Li, H.-W., Qiao, B.-X., Liu, J.-N., Yang, Y., Fan, W., and Lu, G.-L. (2022). A data-driven framework for performance prediction and parameter optimization of a proton exchange membrane fuel cell. Energy Convers. Manag. 271, 116338. doi:10.1016/j.enconman.2022.116338
Li, S., and Wang, B. (2020). Research on evaluating algorithms for the service quality of Wireless sensor networks based on Interval-Valued Intuitionistic Fuzzy EDAS and CRITIC methods. Math. Problems Eng. 2020, 1–12. doi:10.1155/2020/5391940
Ligorio, L., Venturelli, A., and Caputo, F. (2022). Tracing the boundaries between sustainable cities and cities for sustainable development. An LDA analysis of management studies. Technol. Forecast. Soc. Change 176, 121447. doi:10.1016/j.techfore.2021.121447
Ma, J., Wang, L., Zhang, Y.-R., Yuan, W., and Guo, W. (2023). An integrated latent Dirichlet allocation and Word2vec method for generating the topic evolution of mental models from global to local. Expert Syst. Appl. 212, 118695. doi:10.1016/j.eswa.2022.118695
Mun, C., Yoon, S., Raghavan, N., Hwang, D., Basnet, S., and Park, H. (2021). Function score-based technological trend analysis. Technovation 101, 102199. doi:10.1016/j.technovation.2020.102199
Ning, L., Guo, Z., Yongquan, Z., Wu, D., and Qifang, L. (2022). Extracting Unknown parameters of proton exchange membrane fuel cells using Quantum Encoded Pathfinder algorithm. Front. Energy Res. 10. doi:10.3389/fenrg.2022.964042
Park, M., and Geum, Y. (2022). Two-stage technology opportunity discovery for firm-level decision making: GCN-based link-prediction approach. Technol. Forecast. Soc. Change 183, 121934. doi:10.1016/j.techfore.2022.121934
Quanqing, Y., Jianming, L., Zeyu, C., and Michael, P. (2022). Multi-Fault Diagnosis of Lithium-Ion battery systems based on correlation Coefficient and similarity Approaches. Front. Energy Res. 10. doi:10.3389/fenrg.2022.891637
Rathinasamy, M., and Kandhasamy, S. (2022). A novelty analysis about an Impact of Tweets and Twitter Bios on topic quality discovery using the topic modeling. J. Inst. Eng. India. Ser. B 103 (5), 1431–1441. doi:10.1007/s40031-022-00776-w
Russo, D., Spreafico, M., and Precorvi, A. (2020). Discovering new business opportunities with dependent semantic parsers. Comput. Industry 123, 103330. doi:10.1016/j.compind.2020.103330
Sakshi, , and Vinay, K. (2023). Recent trends in mathematical expressions recognition: An LDA-based analysis. Expert Syst. Appl. 213, 119028. doi:10.1016/j.eswa.2022.119028
Sanzi López, V., Costa-Castelló, R., and Batlle, C. (2022). Literature review of energy management in combined heat and power systems based on high-Temperature proton exchange membrane fuel cells for Residential Comfort applications. Energies 15 (17), 6423. doi:10.3390/en15176423
Seo, W. (2022). A patent-based approach to identifying potential technology opportunities realizable from a firm’s internal capabilities. Comput. Industrial Eng. 171, 108395. doi:10.1016/j.cie.2022.108395
Shi, X., Cai, L., and Song, H. (2019). Discovering potential technology opportunities for fuel cell Vehicle Firms: A multi-level patent Portfolio-based approach. Sustainability 11 (22), 6381. doi:10.3390/su11226381
Song, W., Niu, Z., and Zheng, P. (2021). Design concept evaluation of smart product-service systems considering sustainability: An integrated method. Comput. Industrial Eng. 159, 107485. doi:10.1016/j.cie.2021.107485
Sun, K., and Li, Z. (2021). Development of emergency response strategies for typical accidents of hydrogen fuel cell electric vehicles. Int. J. Hydrogen Energy 46 (75), 37679–37696. doi:10.1016/j.ijhydene.2021.02.130
Teng, F., Sun, Y., Chen, F., Qin, A., and Zhang, Q. (2021). Technology opportunity discovery of proton exchange membrane fuel cells based on generative topographic mapping. Technol. Forecast. Soc. Change 169, 120859. doi:10.1016/j.techfore.2021.120859
Venugopalan, M., and Gupta, D. (2022). An enhanced guided LDA model augmented with BERT based semantic strength for aspect term extraction in sentiment analysis. Knowledge-Based Syst. 246, 108668. doi:10.1016/j.knosys.2022.108668
Wahid, J. A., Shi, L., Gao, Y., Yang, B., Wei, L., Tao, Y., et al. (2022). Topic2Labels: A framework to annotate and classify the social media data through LDA topics and deep learning models for crisis response. Expert Syst. Appl. 195, 116562. doi:10.1016/j.eswa.2022.116562
Wang, J., Zhang, L., Lin, K.-Y., Feng, L., and Zhang, K. (2022). A digital twin modeling approach for smart manufacturing combined with the UNISON framework. Comput. Industrial Eng. 169, 108262. doi:10.1016/j.cie.2022.108262
Xu, H., Winnink, J., Yue, Z., Liu, Z., and Yuan, G. (2020). Topic-linked innovation paths in science and technology. J. Inf. 14 (2), 101014. doi:10.1016/j.joi.2020.101014
Yanyan, C., Yuekun, L., Yingjie, X., Xudong, G., Yang, C., and Wuyi, M. (2022). Review: Modeling and simulation of membrane Electrode material structure for proton exchange membrane fuel cells. Coatings 12 (8), 1145. doi:10.3390/coatings12081145
Yu, S., Du, Z., and Chen, L. (2022). Optimal Regulation strategy of electric Vehicle Charging and discharging based on dynamic Regional Dispatching Price. Front. Energy Res. 10. doi:10.3389/fenrg.2022.873262
Zhang, K., Feng, L., Lin, K.-Y., Wang, J., Liu, K., and Zhang, L. (2022). UNISON framework of model-based innovation for collaborative innovation of smart product-service system design. Comput. Industrial Eng. 171, 108494. doi:10.1016/j.cie.2022.108494
Keywords: papers, patents, technology opportunity discovery, proton exchange membrane fuel cell, correlation analysis
Citation: Feng L, Liu K, Wang J, Lin K-Y, Zhang K and Zhang L (2023) Technology opportunity discovery of proton exchange membrane fuel cell from the perspective of papers-patents correlation analysis. Front. Energy Res. 10:1035657. doi: 10.3389/fenrg.2022.1035657
Received: 03 September 2022; Accepted: 11 November 2022;
Published: 18 January 2023.
Edited by:
Jinlong Liu, Zhejiang University, ChinaCopyright © 2023 Feng, Liu, Wang, Lin, Zhang and Zhang. This is an open-access article distributed under the terms of the Creative Commons Attribution License (CC BY). The use, distribution or reproduction in other forums is permitted, provided the original author(s) and the copyright owner(s) are credited and that the original publication in this journal is cited, in accordance with accepted academic practice. No use, distribution or reproduction is permitted which does not comply with these terms.
*Correspondence: Jinfeng Wang, d2FuZ2ppbmZlbmdAc2htdHUuZWR1LmNu