- 1Department of Electrical and Electronics Engineering, SRM Institute of Science and Technology, Chennai, Tamil Nadu, India
- 2Department of Computer Science Engineering, SRM Institute of Science and Technology, Chennai, Tamil Nadu, India
- 3Department of Electrical and Electronics Engineering, Kalasaligam Academy of Research and Education, Krishnan Koil, Tamil Nadu, India
Occupancy-based strategies for the control of ventilation systems in buildings are effective for achieving energy savings and user comfort. Savings in energy consumption of more than 50% can be achieved by controlling heat, ventilation, and air conditioning (HVAC) systems with accurate sensory and occupancy information. In this study, the flow through the damper of the variable area valve (VAV) system and the speed of the blower’s variable frequency drive (VFD) are controlled in the HVAC system, on the basis of human occupancy and indoor parameters, namely, temperature and humidity, segment-wise in the building. In the proposed model, the flapper angle of the VAV is estimated using the indoor temperature, external temperature, and number of occupants. The occupancy data are fed to the controller proposed to regulate the flow through the ducts of the system, which is based on the flapper angle of the VAV, in order to maintain human comfort. The proposed scheme makes it possible to detect abnormalities in energy utilization and to trace maximum utilization in the building based on occupancy, with the control parameters of the HVAC adjusted for a comfortable indoor environment. Performance evaluation of the VAV system with its proposed control strategy, temperature, and flow distribution is simulated using Fluent software. A laboratory grade prototype incorporating the proposed control strategy is then developed, tested under three different conditions, and the results are reported. The experimental results show that an energy saving of 18% can be achieved.
Introduction
Increased energy consumption in buildings and the resulting huge emissions of greenhouse gas have led researchers to focus on energy efficient solutions for smart buildings. The buildings of the future should possess features that meet the anticipated challenges of technological, environmental, and societal progress. The U.S. Green Building Council states that buildings in the United States account for 72% of electricity consumption, 39% of energy use, and 38% of carbon dioxide emissions. In buildings, heating, ventilation, and air conditioning (HVAC) systems account for one-third of energy consumption in providing desired indoor comfort for occupants. It is estimated that over its life cycle, a green building can reduce energy consumption by 24%–50% and CO2 (Lu et al., 2022a; Lu et al., 2022b) emissions by 33%–39%. As a result, the development of green buildings will minimize the impact on human health and the environment. The existing building management systems have limited intelligence and cannot be used for remote operations by end users due to their mechanical logic and the simplicity of their structure and function. To achieve remote control, computing and communication capabilities need to be embedded in all types of structures in the physical environment. Driven by rising environmental concerns, many organizations are adopting smart building networks to reduce the energy consumption and operational costs of commercial buildings. Researchers have become involved in building automated systems in an attempt to find the most effective solutions to meet the high demand for energy conservation while maintaining a comfortable environment inside the building, something that is achieved using HVAC systems.
The main components in HVAC systems are chillers, pumps, control valves, cooling coils, boilers, air handling units, and fans. In existing HVAC systems, the air handling units (AHUs) are controlled by conventional controllers. The main challenge in the HVAC system is to develop a control system that reduces energy consumption and also provides indoor thermal comfort. The literature focuses on the modeling of HVAC systems, the performance of different controllers in HVAC control models, and economic analysis of energy savings. Guo et al. (2007) propose a cascade control strategy for AHU systems involving chilled water flow rate regulation, thereby enhancing the performance of AHUs. A neural network-based controller is developed for the outer control loop to enhance the robustness of the control system. In Stopps et al. (2021), occupant-centric control in residential buildings is exhaustively reviewed and the methods are quantified using results and generalizations. Gholamzadehmir et al. (2020) suggest prediction/horizon control in HVAC systems under various building conditions, thereby ensuring energy and cost savings. Escobar et al. (2020) propose a learning algorithm for multivariable data analysis for control of HVAC systems in buildings. Raad (2018) has developed hybrid layers control for optimization of a HVAC control unit, thereby improving indoor thermal effort and achieving savings in energy. The physical parameters of temperature and humidity are considered for controlling the air velocity to achieve thermal comfort level. In Anuntasethakul and Banjerdpongchai (2021), a supervisory model predictive controller for an HVAC system is considered to minimize operating costs and provide thermal comfort. Occupant-driven demand control to improve the energy efficiency of a building based on stochastic human behavior is achieved in Timilehin et al. (2015).
Many recent works have concentrated on occupancy detection inside the smart buildings. In Chen et al. (2018), occupancy estimation and detection are performed using multiple sensors based on evaluation metrics. Cali et al. (2015) deploy CO2-based occupancy detection founded on real occupancy profiles for dynamic simulation of buildings. The details of window and door positions are used as inputs, which improves the accurate detection of the model. Pedersen et al. (2017) develop occupancy detection based on multiple sensory information and validate their model against the truth on the ground. Their model does not take into account dynamic events and the constraints of different locations, however. Occupancy detection based on CO2 (Lu et al., 2022b; Lu et al., 2022a) concentration at the air return and supply vents using spatial and temporal features is discussed in Jin et al. (2016). In Karavarsamis and Huggins (2019), a two-stage approach is proposed for a homogenous occupancy model that has advantages in model selection. Zhao et al. (2015) develop virtual occupancy sensors that provide occupancy status in the working zone. An image-based depth sensor and camera is deployed for occupancy detection, and a tracking algorithm for use under all lighting conditions is discussed in Shih (2014).
Due to the advancements in IoT in the thermal regulation of buildings, many studies concentrate on implementing IoT in HVAC control. In Vosughi et al. (2020), an occupant-centered control strategy, the Markov linear system, is developed in IoT enabled systems, only the model is not validated in real-time systems. Jeona et al. (2018) discuss IoT-based occupancy-detection in residential apartments based on changing patterns of dust concentration. This work focuses on human activities, such as indoor ventilation time and use of dust masks, with respect to outdoor particulate matter (PM) concentration. A neural network-based smart controller on the IoT platform, integrated with cloud processing for HVAC control, is implemented in Javed et al. (2017). Zhang et al. (2020) propose a framework to identify the thermal model of each zone without manual intervention. The thermal model is learned using temperature readings from IoT smart thermostats. An IoT based intelligent agent learning about the comfort requirements of occupants and control environmental conditions inside a building is discussed in Ashrant Aryal (2021). In Anjali Rajith (2018), an IoT framework is implemented to solve the HVAC control problem by using mixed integer linear programming in a distributed cloud environment. In the works surveyed, it is observed that control of HVAC systems is conducted using only physical sensory information, and does not take account of occupancy information from different segments of the building, thus representing a lack in the control systems of HVACs. Also, the works consulted do not concentrate on damper control in HVACs based on occupancy and environmental conditions. In this present work, the control of HVACs is implemented taking into account both human occupancy and the indoor parameters. The damper of the VAV system and the speed of the VFD drive are controlled in an intelligent manner in light of the system parameters, both in the simulation and in the hardware environment.
The novelty and contribution of this work are as follows:
• The proposed model is an intelligent HVAC control system, which satisfies the occupant-centered temperature regulation requirement in buildings.
• Thermal analysis with Fluent software is conducted to determine the flow and thereby the temperature distribution in the confined space of the room.
• A novel flapper angle equation is developed for the VAV system, employing fusion-based occupancy detection which considers human occupants, temperature, and humidity in every segment of the building.
• A human detection module is developed using adaptive RCNN to control the airflow in human occupancy segments.
Proposed HVAC control framework
This study proposes a novel approach to controlling HVACs in a more intelligent manner by considering room occupancy and indoor parameters. The proposed control algorithm in the HVAC system will provide information that guides decisions about adjusting the control parameters of the drive and flapper angle in the system. The proposed model is developed to produce the flapper point and CFM of the drive framework to fulfil the ISHRAE (standard 55, 2020), by considering the occupancy and segments in each portion of the room. The proposed system achieves both energy savings and comfort in the human occupancy area, based on the intelligent decisions made by the proposed algorithm. The schematic diagram of the proposed HVAC unit control system is presented in Figure 1.
The occupants are identified using a camera module, which is integrated with a deep learning network to detect the presence of humans in every segment of the area. The proposed detection system is accurate in detecting human presence under both static and dynamic conditions in different segments of the room area. The results from the human recognition module, alongside details of temperature and humidity, are fed to the expert control framework, which gives control signals to the damper unit and drive system. The inflow and surge of the duct are constrained by variable air volume (VAV) control damper units, whose point of opening and shutting are acquired from the numerical model created and fed to the damper control unit.
Proposed intelligent control strategy for flapper and CFM
Control of the flapper angle in the inlet and outlet of the duct plays a vital role in controlling the flow of air in a closed area. This feature is integrated with the human detection module in considering the input: namely, the number of occupants, and the internal and external temperature of the area. The proposed HVAC master control system is tested under a simulation platform and then implemented in a real-time environment to provide the outputs for the flapper angle and the CFM of the HVAC (ASHARE Guideline 36-2018). In this work, the angle of the VAV flapper is calculated using the indoor temperature, external temperature, and number of occupants as follows:
where TR is room temperature, Tr is setpoint temperature, n is number of occupants, t is time required, and “0.319” Kwh is multiplied with the temperature change required for unit degree change in temperature in a room with total volume 155 m3, and where 146.536 W h is required by each person. The value of the CFM depends on the following factors: total room volume, duct sizing, blower fan rating, and losses that occur due to the friction factor in the ducts. Once the room volume is known, the duct size plays an important role in carrying the air flow into the room area. The total CFM required in the closed room area is calculated as follows:
The speed of the motor, which is connected to the blower fan, has direct control in transferring the air into the duct regions, which are branched through the areas of ventilation. The velocity of air in the duct is provided the blower and fan speeds are controlled by a variable frequency drive adjustment-based RPM controller, thereby ensuring that the desired CFM remains within ASHRAE standards.
Adaptive RCNN-based deep-learning model for occupancy detection
The human occupancy detection system identifies the number of occupants in the given segmented area, information that is then directly fed into the HVAC master control that regulates the flow through the duct systems in the room area. The image data sets are sent from the camera module to the processing unit, which aggregates them into different human profiles based on gender, lighting conditions, and clothing color. After processing, the collected data sets in the real-time environment are fed as inputs into the RCNN-based deep-learning model for training the algorithm. The proposed RCNN is comprised of an augmented original Faster R-CNN model with the bottom convolution layers shared between all components. The modification introduced in the adaptive Faster R-CNN is the image-level domain classifier, which is added after the last convolution layer, while the instance level domain classifier is added to the end of the ROI wise features to enhance the level of object detection. The two classifiers are linked with a consistency loss to encourage the RPN to be domain-invariant. First, the input image is represented as a convolution feature map, produced by the shared bottom convolution layers. A Region Proposal Network, which generates candidate objects in the region of interest, predicts the category label from the feature vector label using ROI pooling. The object detection problem in this case is viewed as a learning posterior function P(C,B|I), where I is the image representation and B is the bounding box of the human detection. To C belong all the number of categories from {1,….., K}, where k is the total number of categories. The proposed human detection model uses the data set as the source domain, which is developed by segregation of images of different datasets that utilize the workspace on a regular basis, which is Ș, and the test data is T. The domain adaptation components are as follows: image level adaptation and instance level adaptation. In image level adaptation, and to avoid domain distribution mismatch, a patch-based domain classifier is trained by a domain classifier on each activation. The activation function located at (u, v) of the feature map in the ith image in the base convolutional layers is denoted as φ u,v (Ii). By denoting the output of the domain classifier as pi(u,v) and using the cross entropy loss, the image-level adaptation loss can be written as
where
In the instance level adaptation, ROI-based feature vectors help reduce the aspects of appearance, color, viewpoints, and angles of the humans through the camera before feeding to the final category. The instance level domain classifier for the jth region proposal in the ith image as
Results and discussion
The proposed model is implemented in the Research Centre of SRMIST, India, where live occupancy-detection is performed to control the segment-wise operations. The simulation of the temperature distribution for the study area is carried out in the Ansys test bench using Fluent CFD analysis, with the temperature distribution at various flow rates of the inlet and outlet achieved by varying the RPM of the blower. The room volume is 292.96 m3, which is equivalent to 969 CFM, and requires a blower of 1000 CFM. Taking losses into account, a standard 1200 CFM blower is connected to the duct inputs in the simulation setup and the air flow is varied by controlling the speed of the blower through the VFD.
Temperature and flow distribution
The temperature distribution is simulated using Fluent version (3.2.26) through internal grids created in the 3D modeling. A turbulence model is used in the CFD calculation to determine the flow, and in this way the temperature distribution is analyzed in the confined space of the room. The flow, temperature, and velocity simulation in the Ansys Fluent workbench is shown in Figure 2. The velocities at the wall regions are considered to be zero because the no-slip condition and the boundary conditions are applied on interior objects, such as seating benches and other heat sources. The room layout is modelled in SolidWorks, with the dimensions of 12 × 8 × 5 mts, using the geometry input of the Fluent work bench, while the entire room volume is meshed using the tetrahedron mesh. An algebraic multigrid solver is used to identify the flow pattern at various RPMs of the blower. The model is validated by providing the inputs as required room temperature and occupancy-count in every segment of the workspace.
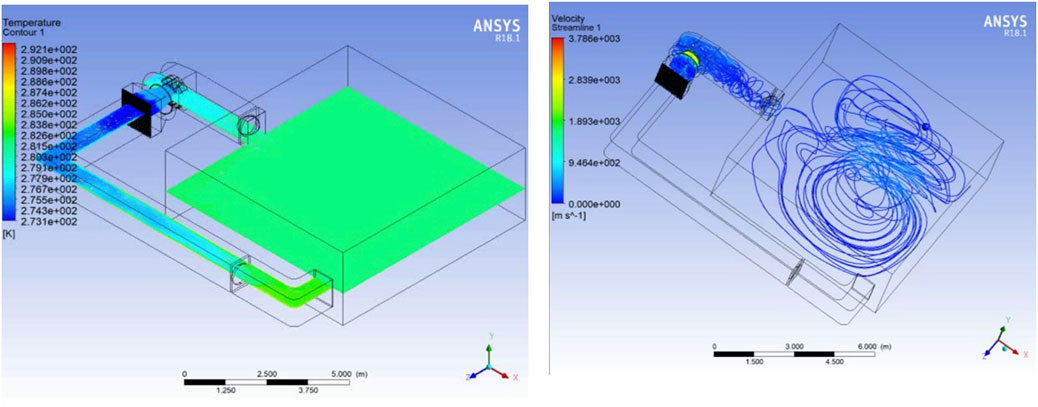
FIGURE 2. Temperature and flow velocity distribution of the room area through ANSYS Fluent workbench.
Experimental results
Occupancy-detection using the proposed adaptive RCNN-based deep-learning model has been carried out in the Research Lab with different occupancies. The occupancy-detection is carried out using higher as well as lower frame rates to validate the detection results. The occupancy count is given to the HVAC as a parameter to regulate the flow control. The experiment is repeated with different numbers of occupants at different frame rates, and the average detection accuracies of the proposed system are 93% and 95% for low and high frame rates. Figure 3 shows the real-time occupancy-detection in the research lab under various lighting conditions and frame rates, along with the experimental setup of the proposed system. In the experimental setup, two cameras (Hikvision 1080p) are mounted at a height of 7 feet for acquiring the live data of human occupancy in the object detection module. The performance of the system is evaluated through a developed test bed, which comprises a MyRio controller interfaced to local control and measurement units. The master controller is integrated with the blower and flapper control to control the air velocity through the flapper angle, and the blower speed through a variable frequency drive.
Table 1 shows the time required to attain the temperature, the angle, and the RPM of the blower unit for various air volume and flapper angles in the experimental setup. The flapper angle is controlled based on the set point temperature and number of occupants. Hence, these two parameters influence the RPM of the blower unit, which in turn provides energy-efficient comfort. It is found that an average of 18% in energy savings is achieved at various air velocities.
Conclusion
In this study, a heat ventilation and air conditioning system is operated in a more intelligent manner by controlling the airflow through the damper of the variable area valve system and the speed of the variable frequency drive. The control module is provided with inputs, namely human occupancy, temperature, and humidity, which are acquired, segment-wise, in the building area. The flapper angle of the VAV flapper and the speed of the variable frequency drive, which directly controls the HVAC system, are estimated by the proposed model using the indoor temperature, external temperature, and the number of occupants. The development of the novel equation derivation of the flapper angle of the damper enables more intelligent control of the system, and thereby provides human comfort in every segment of the building. Thermal analysis using Fluent is conducted to determine the flow and thereby the temperature distribution in the confined space of the room. This proposed model can be retrofitted into commercial buildings to produce future understanding of the building operation area, together with the real-time utilization behavior of flow through ducts of HVAC systems and flow control through VAV units.
Data availability statement
The raw data supporting the conclusion of this article will be made available by the authors, without undue reservation.
Author contributions
The authors confirm contributions to the study as follows: CV: study conception, design, and simulation; JR and RU: data collection; CV, JR, and DD: analysis and interpretation of results; CV and JR: draft manuscript preparation. All authors reviewed the results and approved the final version of the manuscript. The authors confirm sole responsibility for the following: study conception and design, data collection, analysis and interpretation of results, and manuscript preparation.
Conflict of interest
The authors declare that the research was conducted in the absence of any commercial or financial relationships that could be construed as a potential conflict of interest.
Publisher’s note
All claims expressed in this article are solely those of the authors and do not necessarily represent those of their affiliated organizations, or those of the publisher, the editors, and the reviewers. Any product that may be evaluated in this article, or claim that may be made by its manufacturer, is not guaranteed or endorsed by the publisher.
Supplementary material
The Supplementary Material for this article can be found online at: https://www.frontiersin.org/articles/10.3389/fenrg.2022.1017785/full#supplementary-material
References
Anuntasethakul, C., and Banjerdpongchai, D. (2021). Design of supervisory model predictive control for building HVAC system with consideration of peak-load shaving and thermal comfort, 9. IEEE access, 41066–41081. doi:10.1109/ACCESS.2021.3065083
Aryal, A., Burcin, B.-G., Lucas, G. M., and Roll, S. C. (2021). Intelligent agents to improve thermal satisfaction by controlling personal comfort systems under different levels of automation. IEEE Internet Things J. 8 (8), 7089–7100. doi:10.1109/jiot.2020.3038378
Cali, D., Matthes, P., Huchtemann, K., Streblow, R., and Müller, D. (2015). CO2 based occupancy-detection algorithm: Experimental analysis and validation for office and residential buildings. Build. Environ. 86, 39–49. doi:10.1016/j.buildenv.2014.12.011
Chen, Z., Jiang, C., and Xie, L. (2018). Building occupancy estimation and detection: A review. Energy and Buildings, 169, 260–270. doi:10.1016/j.enbuild.2018.03.084
Escobar, M., Aguila, J., Garces-Jimenez, A., De Mesa, J. A. G., and Gomez-Pulido, J. M. (2020), Advanced fuzzy logic based context driven control for HVAC management systems in buildings. IEEE access. 8, 16111–16126. doi:10.1109/ACCESS.2020.2966545
Gholamzadehmir, M., Del Pero, C., Buffa, S., Fedrizzi, R., and Aste, N. (2020). Adaptive-predictive control strategy for HVAC systems in smart buildings – a review. Sustain. Cities Soc. 63, 102480. doi:10.1016/j.scs.2020.102480
Guo, C., Song, Q., and Cai, W. (2007). A neural network assisted cascade control system for air handling unit. IEEE Trans. Ind. Electron. 54 (1), 620–628. doi:10.1109/tie.2006.888809
Javed, A., Larijani, H., Ahmadinia, A., Emmanuel, R., Mannion, M., Gibson, D., et al. (2017). Design and implementation of a cloud enabled random neural network-based decentralized smart controller with intelligent sensor nodes for HVAC. IEEE Internet Things J. 4 (2), 393–403. doi:10.1109/jiot.2016.2627403
Jeona, Y., Choa, C., Seoa, J., Kwona, K., Parkb, H., Ohc, S., et al. (2018). IoT-based occupancy-detection system in indoor residential environments. Build. Environ. 132, 181–204. doi:10.1016/j.buildenv.2018.01.043
Jin, M., Bekiaris-Liberis, N., Kevin, W., Spanos, C. J., and Bayen, A. M. (2016). Occupancy-detection via environmental sensing. IEEE Trans. Autom. Sci. Eng. 15 (2), 443–455. doi:10.1109/tase.2016.2619720
Karavarsamis, N., and Huggins, R. M. (2019). Two-stage approaches to the analysis of occupancy data II.The heterogeneous model and conditional likelihood. Comput. Stat. Data Anal. 133, 195–207. doi:10.1016/j.csda.2018.09.009
Lu, X., Pang, Z., Fu, Y., and O'Neill, Z. (2022a). Advances in research and applications of CO2-based demand-controlled ventilation in commercial buildings: A critical review of control strategies and performance evaluation, Build. Environ., 223, 109455. doi:10.1016/j.buildenv.2022.109455
Lu, X., Pang, Z., Fu, Y., and O'Neill, Z. (2022b). The nexus of the indoor CO2 concentration and ventilation demands underlying CO2-based demand-controlled ventilation in commercial buildings: A critical review. Build. Environ. 218, 109116–116. doi:10.1016/j.buildenv.2022.109116
Pedersen, T. H., Nielsen, K. U., and Petersen, S. (2017). Method for room occupancy detection based on trajectory of indoor climate sensor data. Build. Environ. 115, 147–156. doi:10.1016/j.buildenv.2017.01.023
Rajith, A., Soki, S., and Hiroshi, M. (2018). Real-time optimized HVAC control system on top of an IoT framework. Proceedings of the 2018 Third International Conference on Fog and Mobile Edge Computing (FMEC). 23-26 Apr. 2018, Barcelona, Spain.
Raad, Z. (2018). Analysis and optimization of HVAC control systems based on energy and performance considerations for smart buildings. Renew. Energy 126, 49–64. doi:10.1016/j.renene.2018.03.022
Shih, H.-C. (2014). A robust occupancy detection and tracking algorithm for the automatic monitoring and commissioning of a building. Energy Build. 77, 270–280. doi:10.1016/j.enbuild.2014.03.069
Stopps, H., Brent, H., Touchie, M. F., and O’Brien, W. (2021). Is anyone home? A critical review of occupant-centric smart HVAC controls implementations in residential buildings. Build. Environ. 187, 107369. doi:10.1016/j.buildenv.2020.107369
Timilehin, L., Zeiler, W., Boxem, G., and Yang, Z. (2015). Occupancy measurement in commercial office buildings for demand-driven control Applications- A Survey and detection system evaluation. Energy Build. 93, 303–314. doi:10.1016/j.enbuild.2015.02.028
Vosughi, A., Xue, M., and Roy, S. (2020). Occupant-location-catered control of IoT-enabled building HVAC systems. IEEE Trans. Control Syst. Technol. 28 (6), 2572–2580. doi:10.1109/tcst.2019.2936804
Zhang, X., Pipattanasomporn, M., Chen, T., and Rahman, S. (2020). An IoT-based thermal model learning framework for smart buildings. IEEE Internet Things J. 7 (1), 518–527. doi:10.1109/jiot.2019.2951106
Keywords: HVAC system, damper control, occupancy detection, VAV (variable air volume), BMS (building management system)
Citation: Vasanthkumar CH, Roselyn JP, Uthra RA and Devaraj D (2022) Adaptive damper control for HVAC systems based on human occupancy and indoor parameters: A development study. Front. Energy Res. 10:1017785. doi: 10.3389/fenrg.2022.1017785
Received: 12 August 2022; Accepted: 06 October 2022;
Published: 24 October 2022.
Edited by:
Leyla Amiri, Université de Sherbrooke, CanadaReviewed by:
Xing Lu, Texas A&M University, United StatesHangxin Li, Hong Kong Polytechnic University, Hong Kong SAR, China
Copyright © 2022 Vasanthkumar, Roselyn, Uthra and Devaraj. This is an open-access article distributed under the terms of the Creative Commons Attribution License (CC BY). The use, distribution or reproduction in other forums is permitted, provided the original author(s) and the copyright owner(s) are credited and that the original publication in this journal is cited, in accordance with accepted academic practice. No use, distribution or reproduction is permitted which does not comply with these terms.
*Correspondence: J. Preetha Roselyn, cHJlZXRoYWpAc3JtaXN0LmVkdS5pbg==