- 1Mechanical System Design and Control Department, Idaho National Laboratory, Idaho Falls, ID, United States
- 2Center for Renewable Carbon, The University of Tennessee, Knoxville, TN, United States
In this study, we evaluated the pelleting characteristics of southern yellow pine (SYP), switchgrass (SG), and their blends for thermochemical conversion processes, such as pyrolysis and gasification. Using a pilot-scale ring-die pellet mill, we specifically assessed the impact of blend moisture, length-to-diameter (L/D) ratio in the pellet die, and ratio of pine to SG on the physico-chemical properties of the resulting pellets. We found that an increase in pine content by 25–50% marginally affected the bulk density; however, it also led to an increase in calorific value by 7% and a decrease in ash content by 72%. A moisture content of 25% (wet basis) and an L/D ratio of 5 resulted in poor pellet durability at <90% and bulk density values of <500 kg/m3, but increasing the L/D ratio to 9 and lowering the moisture content to 20% (w.b.) improved the pellet durability to >90% and the bulk density to >500 kg/m3. Blends with ≥50% pine content resulted in lower energy consumption, while a lower L/D ratio resulted in higher pelleting energy. Based on these findings, we successfully demonstrated the high-moisture pelleting of 2.5 ton of pine top residues blended with SG at 60:40 and 50:50 ratios. The quality of the pellets was monitored off-line and at-line by near infrared (NIR) spectroscopy. Multivariate models constructed by combining the NIR data and the pelleting process variables could successfully predict the pine content (R2 = 0.99), higher heating value (R2 = 0.98), ash (R2 = 0.95), durability (R2 = 0.94), and bulk density (R2 = 0.86) of the pellets. Thus, we established how blending and densification of SYP and SG biomass could improve feedstock specifications and that NIR spectroscopy can effectively monitor the pellet properties during the high-moisture pelleting process.
1 Introduction
Various woody and herbaceous biomass sources—such as sugarcane, corn stover, dedicated bioenergy crops, forest, and agricultural residues—could be used for biofuels production (National Renewable Energy Laboratory, 2008). According to the United States (U.S.) Department of Energy (DOE) and Department of Agriculture (USDA), it is estimated that more than a billion ton of such lignocellulosic biomass could be made available for energy production (U.S. Department of Energy, 2016). The Renewable Fuel Standard (RFS) mandates that cellulosic biofuel should slowly displace transportation fuels and reach 16 billion gallons by 2022 (U.S. Environmental Protection Agency, 2018), which requires the annual processing of approximately 1,000–1,200 million tons of biomass.
Perennial grasses, such as switchgrass (SG), have the potential to be environmentally beneficial, high-yielding sources of cellulosic feedstock. SG is a dedicated bioenergy crop in North America, which is one of the largest temperate biomes on Earth, as well as being a carbon sink (Risser et al., 1981; Suyker and Verma, 2001). The significant advantages of SG are that it can grow on marginal lands that are not being used currently to grow food crops (Hartman et al., 2011; Feng et al., 2017), it mitigates nutrient pollution from fertilizer, provides flood control, increases yield when used in a crop rotation, creates wildlife habitats, prevents soil erosion, and sequesters carbon with its extensive root system (Blanco-Canqui et al., 2017).
Woody feedstock is essential to meet the U.S. national goal of producing 16 billion gallons of cellulosic ethanol by 2022 (U.S. Environmental Protection Agency, 2018). Besides conventional lumber, residues from forestry operations, urban management, paper, and furniture industries—including sawdust, chips, shrubs, limbs, leaves, tree trimmings, and forest thinning, as well as trees grown for energy—are excellent sources of woody biomass. Southern pine species are an essential component of the forest resources of the U.S. southern region, which is one of the crucial timber-producing regions globally (Prestemon, and Abe, 2002). Out of over 74 million ha of total timberland that are currently available in 11 southern states, excluding Oklahoma and Kentucky, 27.5 million ha are classified as softwood types, while another 8.5 million ha are classified as oak-pine types (Zhang and Polyakov, 2010). Pine plantations account for 15 million ha, more than half of the softwood forest area.
Blending of various biomass feedstocks for biofuel and bioproduct production is gaining momentum. According to Chescheir and Nettles (2017) and Chauhan et al. (2011), there is great interest in planting herbaceous biomass such as SG and other straws with woody biomass, which can make blending of the biomass feasbile in the field. Feedstock cost modeling studies by Idaho National Laboratory (INL) have showed that the blending of woody, herbaceous, and municipal solid waste reduces the grower payment by about 46% (Lane, 2018). Blending or formulation are commonly used in other industries, such as food and feed, to achieve a consistent product with desired quality attributes. In the power industry, coals of different grades are blended to control the SOx and NOx emissions (Moroń and Rybak, 2015). Lignocellulosic formulations can be similarly developed by blending multiple woody and herbaceous biomasses to meet the desired chemical composition and specifications for thermochemical and biochemical conversion processes, such as calorific value, volatiles, cellulose, lignin, hemicellulose, carbon, hydrogen, nitrogen, alkaline, and alkali-earth metals contents (Edmunds et al., 2018; Ou et al., 2018). Woody biomasses have a higher lignin and lower ash content, whereas herbaceous biomasses have a lower lignin and higher ash content (Williams et al., 2017). Blending of these biomasses, therefore, has the potential to homogenize their overall chemical composition, as well as improve downstream conversion.
According to Ray et al. (2017), blending helps to overcome the cost and quality limitations of lignocellulosic biomass for biofuels production. Edmunds et al. (2018) indicated that the blending of different biomass sources helps to improve their overall feedstock specifications for thermochemical conversion. Yancey et al. (2013) showed that blending reduces the variability of the physical properties and the chemical compositions in various biomass sources while producing a consistent feedstock. The advantages of biomass blending are increased feedstock availability for biorefineries at a reduced cost and improved quality and preprocessing characteristics. According to Mahadevan et al. (2016), the blending of SG and southern pinewood produced bio-oils with low acidity and viscosity. Despite these advantages, there are significant challenges in blending different biomass types, which could cause issues related to feeding, handling, transportation, and storage (Ray et al., 2017).
The typical challenges in using biomass blends are: 1) variability in density, particle shape and size distribution, and rheological properties, which result in hurdles such as entrainment and classification; 2) segregation of the particles because of variability in density; and 3) low density that creates challenges in feeding and reduces conversion efficiencies (Ray et al., 2017). These challenges can be overcome by densifying the biomass blends. Densification improves the handling and conveyance efficiency of bioenergy in-feed supply systems (Tumuluru et al., 2011). It also provides better control on the particle size distribution of the product stream for improved feedstock uniformity and density, enhancing the deconstruction of biomass structural components and improving biochemical and thermochemical conversions. Pelleting also reduces transportation costs by 43% for distances exceeding 15 miles (Tumuluru and Mwamufiya, 2021). For densification using conventional methods, the lignocellulosic feedstocks are dried to about 10% moisture content on wet basis (w.b.). Woody biomass after harvesting has a moisture content between 30 and 50% (w.b.) and that of SG between 15 and 25% (w.b.). Hence, drying biomass to <10% (w.b.) moisture content using conventional rotary dryers adds to the energy and capital costs (Tumuluru, 2016). Also, high-temperature drying results in volatile organic emissions forming photo-oxidants that are hazardous to human health if inhaled. A high-moisture pelleting process was therefore developed at INL to eliminate the rotary drying step and to reduce energy and capital costs. In this process, the biomass is pelleted at higher moisture levels >20% (w.b.). We have previously studied the pelleting of corn stover, lodgepole pine, and 2-in. (50.8 mm) pine tops and SG blends in a flat die pellet mill in the moisture range of 20–39% (w.b.) (Tumuluru, 2014, 2016, 2019). The resulting pellets had good quality in terms of density and durability. These results also showed that having a higher pine content (>50%) in the blends resulted in higher quality pellets and lower energy consumption. In their techno-economic analysis (TEA), research conducted by Lamers et al. (2015) indicated that the drying of corn stover to <10% (w.b.) moisture content using conventional rotary dryer added significantly to the pelleting cost, whereas pelleting at high-moisture levels of 30% (w.b.) by eliminating the rotary drying, reduced the pelleting cost by about 40%. Our recent study on municipal solid waste (MSW) also showed that high-moisture pelleting could save about 40–46% of pelleting cost and lower 46% of greenhouse gas emissions (Tumuluru and Mwamufiya, 2021). The resulting MSW pellets exhibited a durability between 90 and 98% and a bulk density of about 450–550 kg/m3 (Tumuluru and Mwamufiya, 2021). Hence, high-moisture pelleting is a good alternative in lieu of conventional pelleting for the densification of pine and SG blends.
In recent years, near infrared (NIR)-based high-throughput spectroscopic techniques have been developed to monitor the output of pelleting and other downstream processing of lignocellulosic biomass (Edmunds et al., 2018; Feng et al., 2018; Li et al., 2018; Li et al., 2021). NIR spectroscopy works based on the absorption, emission, reflection, and diffuse-reflection of light in the region of 800–2,500 nm (e.g., 12,500–4,000 cm−1) (Ozaki et al., 2017). Multivariate prediction models could be built on the basis of on-, at-, or off-line NIR spectroscopic analysis and used for rapid, inexpensive characterization of changes in lignocellulosic feedstocks during thermochemical or physico-chemical conversion processes (Li et al., 2018; Hwang et al., 2021). Principal component analysis of online NIR spectra has been recently used to screen the seasonal variability of cellulose crystallinity and lignin content in sugarcane bagasse (Li et al., 2021). Similarly, off-line NIR spectroscopy was used to monitor regional differences in the composition of cellulose, hemicellulose, lignin, and ash of Jerusalem artichokes (Li et al., 2018). The studies on high-moisture pelleting of southern yellow pine (SYP), switchgrass (SG), and their blends using a pilot-scale pellet mill and data on understanding the quality changes during pelleting using on-, at-, or off-line NIR spectroscopy are not available in the literature. To our knowledge, this will be the first study that tests high moisture pelleting of SYP, SG and their blends using pilot scale pellet mill and uses of at-line and off-line NIR spectroscopy to characterize and predict the properties of blended biomass pellets that are of notable relevance for bioenergy production in the U.S.
The overall objective of this work was to investigate the influence of pellet mill die compression (length to diameter ratio) ratio and moisture content on the pelleting characteristics of different blend ratios of SG and SYP residues. A ring-die pellet mill with one ton/h throughput was used for the pelleting studies. According to Tumuluru et al. (2011), the pelleting process variables—such as die diameter and die compression ratio—and feedstock properties—such as moisture and particle size—impact the quality of the pellet produced. Therefore, the specific objectives of our work were to evaluate the impact of: 1) pellet mill die compression (L/D) ratio between 5 and 9; 2) blend ratios of 6.35 mm ground SG and SYP residues (e.g., 0:100, 25:75, 50:50, 75:25, 100:0); and 3) two different moisture levels of 20 and 25% on resulting pellet properties in terms of moisture, unit, bulk and tapped density, durability, ultimate composition, ash contents, higher heating value (HHV), and specific energy consumption (SEC) of the process. Based on the preliminary studies, we selected the combination of parameters [e.g., blend mositure cotnent of about 20% (w.b.) and a L/D ratio of 9] and demonstrated the pelleting of 2.5 ton of 2-inch (50.8 mm) and 6-inch (152.4 mm) SYP residues blended with SG in the ratio of 60:40 and 50:50. We also demonstrated how off- and at-line NIR spectroscopy could be applied for high-throughput characterization of the pellets and for developing multivariate models to predict physico-chemical properties of blended pellets.
2 Materials and Methods
2.1 Feedstock Preparation
Switchgrass (SG) (Panicum virgatum L.) cv. Alamo stands were cultivated and harvested in 2017 in Vonore, TN. The SG with leaves and stalks was processed via two-stage grinding; at stage-1, the hammer mill (Bliss Industries LLC, Ponca city, OK) was fitted with a 76.2 mm screen, while at stage-2, it was fitted with a 6.35 mm (1/4-in.) screen. The ground SG biomass was shipped to INL in super-sacks for further pelleting. The chemical composition of the received biomass was 38 ± 1% cellulose, 28 ± 1% hemicellulose, 21 ± 0% lignin, 5 ± 0% extractives, and 5 ± 0% acetyl contents.
Clean southern yellow pine (SYP) (Pinus spp.) residues with a 152.4 mm (6-in.) stem diameter were harvested from the mature stands at the School of Forestry and Wildlife Sciences at Auburn University, AL, in 2017. The biomass was chipped, dried to about a 10% (w.b.) moisture content, and then shipped to INL. At INL, the pine chips were further ground using a hammer mill (Bliss Industries LLC, Ponca city, OK) fitted with a 6.35 mm screen. The ground SYP and SG were reconditioned to high moistures (20 and 25%, w.b.) and used to test the high-moisture pelleting process either individually or in blends of 25:75, 50:50, and 75:25, respectively. A pilot-scale ring-die pellet mill was used for conducting the pelleting studies on SG, pine, and their blends. Pioneer pellet mill model number: 35A- 75-80HP, serial number 3193, Bliss Industries, LLC, (Ponca City, OK), was used in the present pelleting studies. According to the manufacturer, the recommended break pressure for operating the pellet mill is 1,000 psi. This mill is designed for overall reliability and maximum efficiency and ease of operation. During the pelleting process, the die and collar assembly were protected by a hydraulic overload system, which stops the main motors when an overload situation occurs.
For the 2.5-ton demonstration of high-moisture pelleting, SYP tops of 50.8 mm (2-in.) and 152.4 mm (6-in.) diameter were screened and selected. About 50 pine trees were harvested in 2018 from the mature stands of the School of Forestry and Wildlife Sciences at Auburn University, AL, to gather sufficient biomass between 2,000 and 3,000 kg and further dried to about 10% (w.b.) before shipping to INL. These pine residues were ground at INL using a Bliss hammer mill fitted with a 6.35 mm screen size. The ground material was then blended with either 40% or 50% of the previously obtained SG. The chemical composition of the 2-in. (50.8 mm) SYP was 29 ± 0% cellulose, 20 ± 0% hemicellulose, 38 ± 1% lignin, 10 ± 0% extractives, and 1.5 ± 0% acetyl contents, whereas the chemical composition of the 6-in. (152.4 mm) SYP was 36 ± 0% cellulose, 22 ± 0% hemicellulose, 36 ± 1% lignin, 6 ± 0% extractives, and 2 ± 0% acetyl contents.
2.2 High-Moisture Pelleting
A pilot-scale ring-die pellet mill at INL with a throughput capacity of 1 ton/h, as shown in Figure 1, was used for both the preliminary testing of SG-SYP blends and for the 2.5-ton high pelleting demonstration. The pellet mill is set-up with a ribbon blender, which pre-conditions the biomass moisture, a steam conditioner that adds moisture and heat during pelleting, and data loggers for recording the pelleting parameters, including conveyor speed, amperage, power, pressure, and steam usage. It also has various pellet dies with different compression (L/D) ratios. During the preliminary pelleting of SYP, SG, and their blends, three L/D ratios (e.g., 5, 7, 9) and two moisture levels (20 and 25% w.b.) were tested for five different biomass blends, as shown in Table 1. Based on the preliminary results, we selected an L/D ratio of 9 and a moisture content of 20% (w.b.) for the 2.5-ton pelleting demonstration of 2-in. (50.8 mm) and 6-in. (152.4 mm) SYP blended with SG. During the demonstration, about 1,000 kg of 6-in. (152.4 mm) SYP-50, 227 kg of 6-in. (152.4 mm) SYP-60, 1,000 kg of 2-in. (50.8 mm) SYP-60, and 227 kg of 2-in. (50.8 mm) SYP-50 blends were pelleted using the parameters listed in Table 1. These tests were conducted to understand the impact of blend ratios, L/D ratios, and moisture content on pellet physico-chemical properties, namely ash content, higher heating value (HHV), density (e.g., unit, bulk and tapped), and durability, as well as pelleting energy consumption.
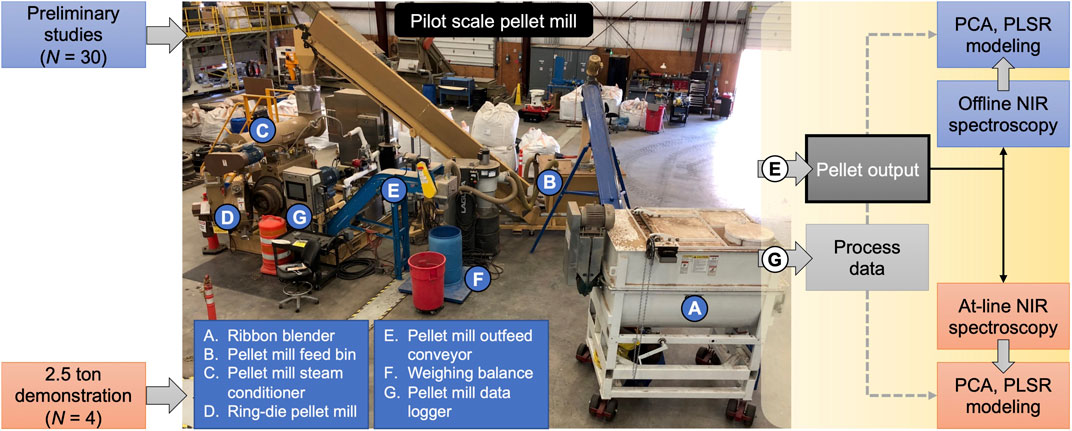
FIGURE 1. Illustration of high-moisture pelleting of southern yellow pine (SYP) and switchgrass (SG) blends at the pilot-scale set-up (Legend: N–number of treatments; NIR–near infrared spectroscopy; PCA–principal component analysis, PLSR–partial least squares regression).
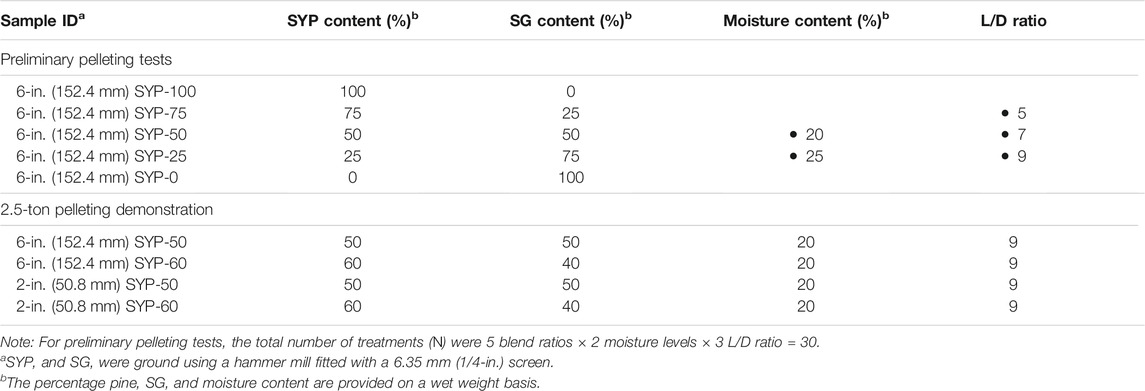
TABLE 1. Experimental plan for preliminary and 2.5-ton high-moisture pelleting tests of southern yellow pine (SYP), switchgrass (SG), and their blends.
Power consumption data during pelleting were logged using LabVIEW (National Instruments, Austin, TX). An APT power monitor meter (Applied Power Technologies, Inc., San Jose, CA) connected to the pellet mill recorded the power consumption during the high-moisture pelleting in kilowatts. The no-load power was recorded by running the pellet mill empty. The specific energy consumption (SEC) was calculated by subtracting the no-load kW from the full-load power using Eq. 1.
2.3 Physico-Chemical Characterization of Pellets
2.3.1 Physical Properties
Pellet moisture content, bulk density, and durability were determined following the American Society of Agricultural and Biological Engineers (ASABE) S269.4 standard (American Society of Agricultural and Biological Engineers (ASABE) Standard S269.4., 2007). Particle size distribution of the ground material was measured using ASABE standard S424.1 (American Society of Agricultural and Biological Engineers (ASABE) Standard S424.1., 1992). The pellets produced were not only analyzed for moisture content; unit, bulk, and tapped density; and durability immediately after pelleting at different moisture levels, but also after drying in an oven at 70°C for 2–3 h to reduce the moisture content to <10% (w.b.). Briefly, moisture content was measured using the oven dry method where the sample was dried at about 105°C for 24 h. The unit density was calculated by measuring the length, diameter, and weight of the individual pellets. The bulk density was measured by pouring the pellets into a cylindrical container and calculated by dividing the weight of the pellets by the container volume. The tapped density was measured by tapping the container on the flat table and filling the container with pellets and measuring the weight again (Tumuluru, 2014; Tumuluru, 2016). A durability tester with four compartments was also used, where 500 g of pellets were placed in each compartment and then rotated at 50 revolutions per minute (RPM) for 10 min (Tumuluru, 2018). Percent durability is given as the ratio of the mass of the intact pellets (after sieving) after tumbling to the total mass of pellets before tumbling. All pellet properties were measured in triplicate.
2.3.2 Ultimate Analysis, Ash, HHV Determination
Biomass materials were ground and screened to a uniform particle size of 0.425 mm (40-mesh) using a Wiley® mini blade mill (Thomas Scientific, Swedesboro, NJ) for ash content and ultimate composition analysis. Total ash content was determined based on the standard laboratory analytical procedure developed by the National Renewable Energy Laboratory (NREL, Golden, CO), where the homogenized biomass was combusted at 575°C, until a constant weight was achieved (Sluiter et al., 2005). The ultimate analysis of carbon (C), hydrogen (H), and nitrogen (N) was performed using a 2,400 Series II CHNS elemental analyzer (PerkinElmer, Shelton, CT), where the oxygen (O) content was calculated by difference [100–(C + H + N)]. For the determination of higher heating value (HHV), about 1.3 g of biomass pellets were loaded into the decomposition vessel of a C 6000 oxygen bomb calorimeter (IKA Works, Inc., Wilmington, NC) and analyzed at 22°C isoperibol mode, with integral oxygen filling/degassing, as well as water recirculation at 3200 RPM. Benzoic acid was used as the calibration standard. All measurements for ash content, CHN, and HHV were conducted in triplicate per sample per biomass blend.
2.4 At- and Off-Line NIR Spectroscopy
Samples from preliminary pelleting tests (N = 30) were characterized off-line using a desktop DA 7250 SD NIR spectrometer (Perten Instruments AB, Hägersten, Sweden). During the 2.5-ton pelleting demonstration, samples of approximately 25 g were manually collected every 2.5 min from the outfeed pellet conveyor and subjected to at-line NIR spectroscopy using the same DA 7250 SD instrument. The at-line NIR scans were meticulously time-stamped and the corresponding pellet temperature was recorded by using a hand-held infrared thermometer. The pellet moisture content was also determined in duplicate by drying at 105°C until constant weight. The pellet samples for both the at- and off-line NIR analysis were packed twice. For each repack, the samples were scanned twice in the range of 950–1,650 nm. The sample temperature and moisture content at the time of NIR analysis are provided in Supplementary Table S1.
2.4.1 Multivariate Prediction Modeling
The collected NIR spectra were subjected to detrending and normalization via a standard normal variate (SNV) method prior to mulitivariate analysis using the Unscrambler X v10.4 software (Aspen Technology, Inc., Bedford, MA). Principal component analysis (PCA) was carried out on the preprocessed NIR spectra to identify clusters and/or trends caused by varying the percent of pine and SG, SYP tops diameter, pellet L/D ratio, and moisture content. The non-linear iterative partial least squares (NIPALS) algorithm was used to build the PCA models and the analysis of NIR spectral variability was limited to five principal components. The PCA scores extracted from PC-1, 2, 3, 4, and 5 were then merged with the pellet mill process data to build partial least squares regression (PLSR) models in order to predict a specific pellet property (e.g., moisture, ash, and carbon contents). Process data obtained from the pellet mill data loggers included steam mass flow, steam process pressure, conditioner water flow rate, current, speed, and temperature. Pellet properties, including durability, bulk density, carbon, moisture, ash, HHV, and the amount of pine biomass (%SYP) were used as Y-response factors. The process variables were averaged and the standard deviations for each run were also correlated to the pellet properties. Cross-validation was applied for both the PCA and PLSR models, and up to eight predicting factors were used in the PLSR models. During PLS regression of the preliminary test samples, variables with auto-correlation (r > 0.9) and lower coefficient of variation (CV < 1%) were removed from the data set.
3 Results
3.1 Physical and Chemical Properties of Raw Material
The bulk density and geometric mean particle length of switchgrass (SG) after passing through a 6.35 mm screen were 104 kg/m3 and 1.25 mm, respectively. Determination of particle size distribution indicated that milling of SG in two stages led to more fines in the grind. In the case of 6-in. (152.4 mm) SYP residues used for the preliminary tests, the geometric mean particle length and bulk density were determined to be 0.91 mm and 190 kg/m3, respectively, at a moisture content of about 10% (w.b.).
The ultimate analysis, ash content, and HHV of the raw material are provided in Table 2. Both the 2-in. (50.8 mm) SYP and SG biomass contained twice the amount of ash when compared to the 6-in. (152.4 mm) SYP biomass. Our previous work has also shown that the 2-in. (50.8 mm) SYP biomass (4,595 mg/kg) contained significantly higher amounts of combined alkali and alkaline earth metals (Ca, K, Mg, Na) when compared to SG (2,198 mg/kg) and 6-in. (152.4 mm) SYP (2,472 mg/kg) biomasses (Edmunds et al., 2018). The carbon content of SYP was also appreciably higher than the SG, but the HHV differed by only 5% between these raw materials. Ash could reduce the performance of biomass conversion reactors, whereas alkali and alkaline earth metals can specifically affect the composition and yield of downstream products during thermochemical conversion of lignocellulosic feedstocks (Edmunds et al., 2018; Kim et al., 2018). Hence, blending these raw materials could potentially reduce heterogeneity and improve their downstream conversion properties.
3.2 Preliminary Pelleting of Southern Yellow Pine (SYP) and Switchgrass (SG): Effect of moisture content, L/D, and Blend ratio
In the case of the 100% pine pellets, 5–10% (w.b.) moisture loss was observed during the pelleting process. A higher moisture loss of 10% (w.b.) was observed at a lower L/D ratio of 5 compared to L/D ratios of 7 and 9. The unit, bulk, and tapped densities at different moisture levels and L/D ratios were in the range of 1,096–1,169, 471–569, and 518–616 kg/m3, respectively. The durability was in the range of 89–95%, where a higher L/D ratio and lower moisture content were found to be beneficial. The physico-chemical properties of all pellet blends are provided in Table 3, Supplementary Tables S2 and S3, and the appearance of the pellets is depicted in Figure 2.
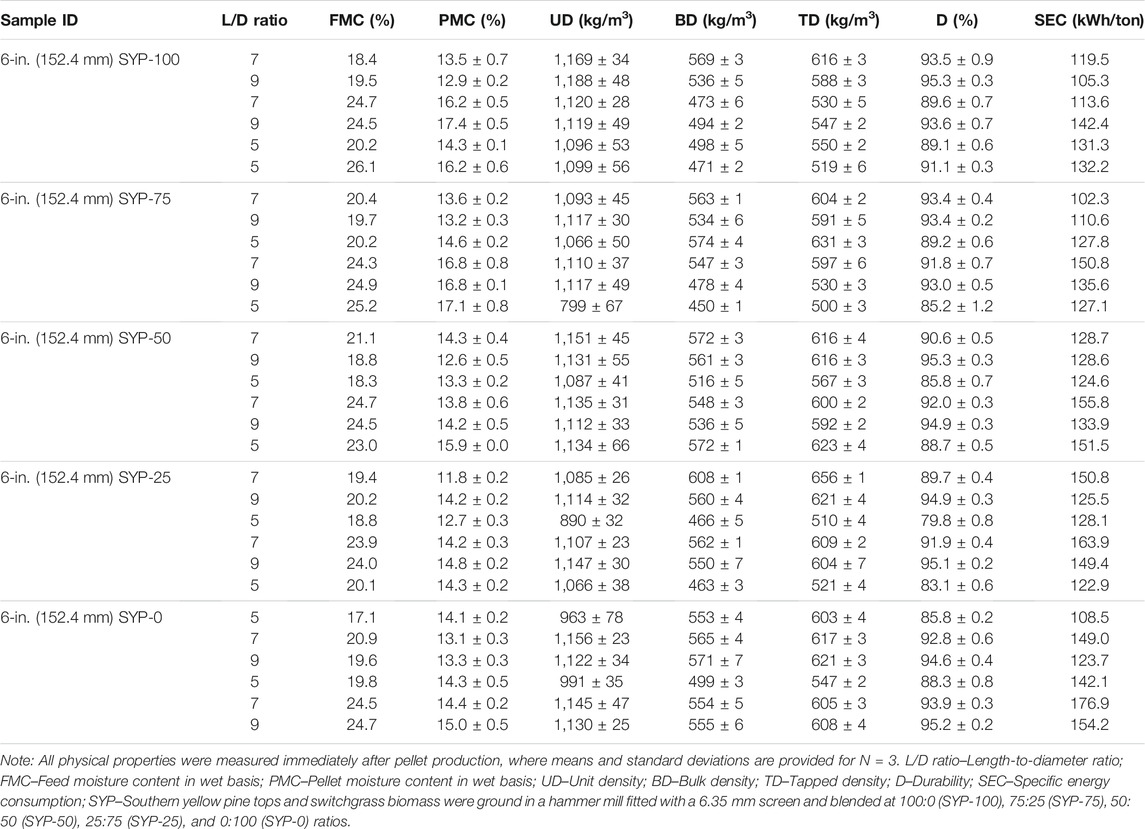
TABLE 3. Average physical properties of high-moisture pellets produced immediately after pelleting during preliminary tests.
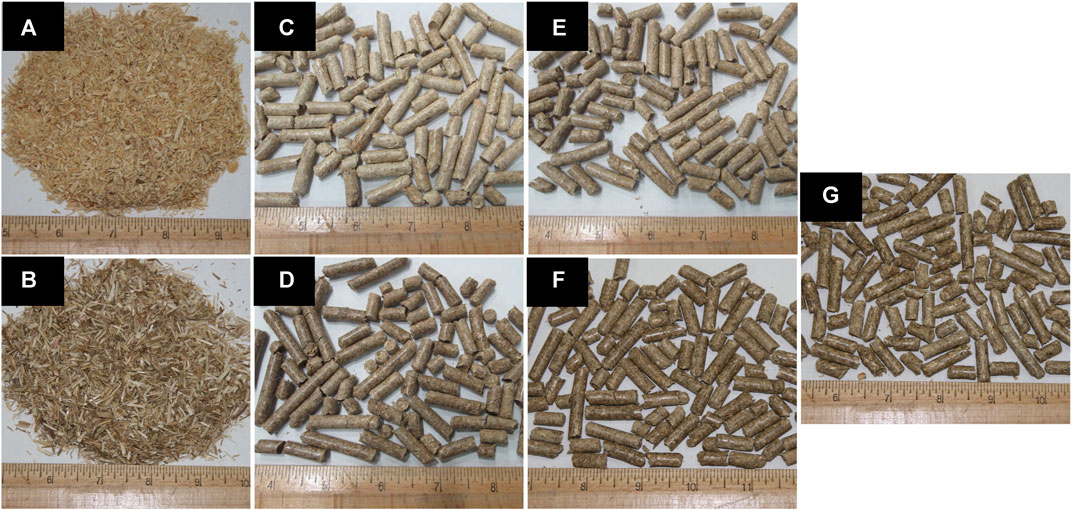
FIGURE 2. Raw biomass and pellets made of 6-in. (152.4 mm) southern yellow pine (SYP) tops, switchgrass (SG), and their blends. All pellets were produced at high-moisture content (20% w.b.) and at two different length-to-diameter ratios (7 or 9). (A) SYP tops ground to 6.35 mm screen size in a hammer mill; (B) SG biomass ground to 6.35 mm screen size in a hammer mill; (C) 100% SYP pellets; (D) 100% SG pellets; (E) 75% SYP and 25% SG pellets; (F) 75% SG and 25% SYP pellets; and (G) 50% SYP and 50% SG pellets.
In the case of the 75% pine pellets, there was a moisture loss of 6–8% (w.b.). A higher moisture loss was observed at a higher L/D ratio of 9 and higher blend moisture content of 25% (w.b.). The unit, bulk, and tapped densities were in the range of 799–1,117, 450–573, 499–631 kg/m3, respectively, and the durability was 85–93%. A higher moisture content and a lower L/D ratio reduced the density and durability of 75% pine pellets.
In the case of the 50% pine pellets, a moisture loss of 6–10% (w.b.) was observed during pelleting and a higher L/D ratio of 9 as well as a higher blend moisture content of 25% (w.b.) resulted in greater losses. The unit, bulk, and tapped densities were in the range of 1,087–1,115, 516–572, 569–623 kg/m3, respectively, and the maximum durability was observed for a lower blend moisture content and L/D ratio of 9. Overall, the durability values were in the range of 85–95% for the different pelleting parameters.
For the 25% pine pellets, a moisture loss in the range of 5–9% (w.b.) was observed, and a higher blend moisture content of 24% (w.b.) and a L/D ratio of 9 resulted in greater losses. The unit, bulk, and tapped densities were the lowest at an L/D ratio of 5 and a blend moisture content of 19% (w.b.). Overall, the unit, bulk, and tapped densities were in the range of 889–1,146, 465–607, 510–654 kg/m3, respectively. The lowest durability of 83% was observed at an L/D ratio of 5 and a blend moisture content of 20% (w.b.), whereas a maximum durability of 95% was observed at a L/D ratio of 9 and a moisture content of about 20% (w.b.).
In the case of the 100% SG pellets, the moisture loss was similar to 100% pine pellets (about 5–10%, w. b.), but interestingly, higher moisture losses were recorded at higher L/D ratios of 7 and 9. The unit, bulk, and tapped densities were between 991 and 1,156, 499–552, and 546–621 kg/m3, respectively, and durability was between 85 and 95%. Overall, higher L/D ratios of 7 and 9 and a lower moisture content of about 20% (w.b.) maximized the durability of 100% SG pellets, as can be seen in Table 3.
Drying the pellets at 70°C for 2–3 h was aimed at reducing the moisture content to <10% (w.b.); however, it also affected pellet density and durability. In the case of unit density, a loss of about 40–50 kg/m3 was observed, whereas the bulk and tapped densities decreased by 20–25 kg/m3. The pellet durability changed between −4.7% and +1.9%, and most pellet treatments experienced a decrease in durability because of low temperature drying, as observed in Supplementary Table S2. The trends observed for blended SYP and SG pellets in terms of bulk density and durability matched our earlier studies with corn stover, lodgepole pine, and municipal solid waste (MSW) (Tumuluru, 2014; Tumuluru et al., 2015; Tumuluru, 2016; Tumuluru and Mwamufiya, 2021), where higher moisture in the biomass reduced the density and higher L/D ratio increased the durability.
Specific energy consumption (SEC) of the pelleting process was determined as per Eq. 1 and provided in Table 3. Based on the results, a higher pelleting moisture and L/D ratio led to an increase in pelleting energy. In the case of 100% pine pellets, the lowest energy consumption was observed at 20% (w.b.) pelleting moisture and an L/D ratio of 9, as can be seen in Table 3. Increasing the moisture content increased the pelleting energy to 130–140 kWh/ton for different L/D ratios. Compared to 100% pine, the 100% SG pellets consumed more energy. For example, at an L/D ratio of 9 and 20% (w.b.) moisture content, energy consumption of 100% SG pellet was 123 kWh/ton, whereas the 100% pine only consumed 105 kWh/ton of energy. Further increasing the moisture content and lowering the L/D ratio to 5 increased the pelleting energy to about 176 kWh/ton. In the blended feedstocks, higher amounts of pine (75%) reduced the energy consumption to about 102–110 kWh/ton for the L/D ratios of 7 and 9. In the case of the 50% pine biomass, energy consumption increased to a range of 124–155 kWh/ton for the different L/D ratios (5–9) and moisture contents (20–25%, w. b.). In the case of 75% SG blends, the lowest energy consumption (125 kWh/ton) was observed at a pelleting moisture content of 20% (w.b.) and an L/D ratio of 9.
Multivariate models constructed by combining the NIR data and the pelleting process variables indicated that the L/D ratio (R2 = 0.72) and bulk density (R2 = 0.50) had positive correlations with pellet durability, as shown in Supplementary Figure S1 (Supplementary data), whereas the pine content (R2 = 0.26) exhibited a mild negative correlation to the bulk density, and the pelleting moisture content had no correlation to the pellet bulk density (R2 = 0.09) or durability (R2 = 0.002). Hence, we determined that an L/D ratio of 9 is desirable to improve pellet durability, whereas the blending of pine residues with SG would improve pelleting energy consumption.
3.2.1 Effect of Pelleting Paramters on Ultimate Composition, Ash, and HHV
No significant differences were observed in the carbon (C), hydrogen (H), and oxygen (O) content of the preliminary test pellets as a function of pine blend ratio, blend moisture content, or L/D ratio, as observed in Supplementary Table S3. Only the nitrogen (N) content displayed minor variations depending on the pine blend ratio; pellets of 100% SG contained 86% more N than those with 100% SYP, whereas all blended pellets contained even quantities of N. Hence, blending of pine and SG homogenizes the ultimate composition of the resulting pellets. Other physico-chemical properties, namely the ash content and the HHV, were affected by the pelleting parameters. Pine content had a significant positive correlation to the calorific value of the pellets, which can be clearly observed in Figure 3A. Similarly, higher pine content and heating values were negatively correlated with the ash content, as shown in Figure 3B. However, factors such as L/D ratio (R2 = 0.02–0.05) and pelleting moisture [(R2 = 0.05–0.09) did not have any significant impact on pellet ash content or HHV, as observed in Supplementary Figure S2]. Hence, modulating the pine content would be essential for controlling the downstream conversion performance of the blended pellets.
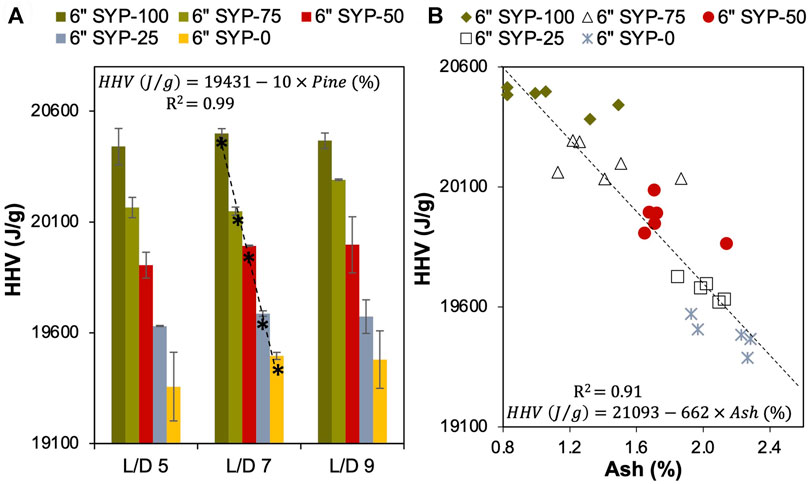
FIGURE 3. (A) Variations in the higher heating value (HHV) of pellets as a function of biomass blend and length-to-diameter (L/D) ratios. The dotted line represents linear regression between pellet pine content (%) and HHV (J/g) for all L/D ratios, while the corresponding regression equation is provided as an inset; (B) Distribution of pellet HHV as a function of ash content. Southern yellow pine (SYP) tops of 6-in. (152.4 mm) stem diameter were ground in a hammer mill fitted with 6.35 mm screen and was blended with similarly ground switchgrass (SG) at 100:0 (SYP-100), 75:25 (SYP-75), 50:50 (SYP-50), 25:75 (SYP-25), and 0:100 (SYP-0) ratios. The linear regression of pellet HHV and ash content is represented as a dotted line, with the corresponding equation provided as an inset.
3.2.2 Prediction Modeling: Off-Line NIR Spectroscopy Combined With Pelleting Process Data
Partial least squares regression (PLSR) models developed based on off-line NIR spectroscopic data of the 30 preliminary pellet samples showed that the ash content, HHV, and pine ratio could be predicted to a high accuracy, as observed in Table 4. We also combined the scores extracted from principal component analysis (PCA) of the pellet NIR spectra with that of the pelleting process information and investigated the effects of PLSR model predictability. As shown in Table 4, integration of pelleting process data significantly improved the predictability of pellet bulk density and durability. Input variables of significance to a specific pellet property were obtained from the PLS regression coefficients and provided in Table 4 as well. Both the NIR PCA scores and process parameters, including in-feed speed, steam mass flow, drive current, and conditioner outlet temperature, were of significance to the pellet properties. We thus showed how PLSR prediction models for lignocellulosic feedstocks could be improved by integrating process data.
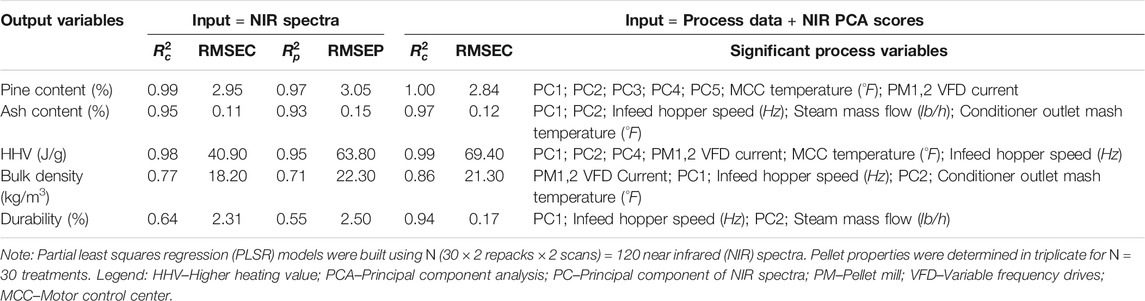
TABLE 4. Performance of PLSR models for predicting pellet properties based on NIR PCA scores (in 950–1,650 nm region) and pelleting process data.
3.3 2.5-ton Demonstration of High-Moisture Pelleting
Following the preliminary pilot-scale studies on SYP, SG, and their blends, subsequent demonstration on a 2.5-ton scale were conducted. The L/D ratio of 9 and moisture content of about 20% (w.b.) were selected since these pelleting conditions resulted in higher pellet quality, in terms of bulk density and durability, at lower energy consumption. Pine top residues of 2-in. (50.8 mm) and 6-in. (152.4 mm) diameter were selected to investigate the impact of lower quality feedstock (i.e., 50.8 mm pine tops) on final pellet properties. The grind properties, namely geometric mean particle length and D50 (median particle size) of the 2-in. (50.8 mm) pine residues were 1.22 and 1.30 mm, respectively, and those of 6-in. (152.4 mm) pine residues were 0.99 and 1.06 mm, respectively. The grinding energies for 2-in. (50.8 mm) and 6-in. (152.4 mm) pine residues were 24.3 and 23.3 kWh/ton, respectively. Hence, the pelleting properties were not significantly different for the two types of pine residues tested.
On the other hand, differences in physical properties of the pellets were observed due to the pine blend ratio and pine residue size, as shown in Table 5. In the case of 6-in. (152.4 mm) pine residues, the 60% blend resulted in higher unit density, but the bulk and tapped density, as well as the durability, were not significantly different compared to the 50% blend. The energy consumption was slightly higher for the 50% blend of 6-in. (152.4 mm) SYP at 98 kWh/ton when compared to the 60% blend (89 kWh/ton). In the case of 2-in. (50.8 mm) SYP residues, a 60% blend produced pellets with lower unit, bulk, and tapped densities, as well as durability, but the SEC was lower at 87 kWh/ton than that of the 50% blend (95 kWh/ton). Among the four tested blends, 2-in. (50.8 mm) SYP-60 produced lower quality pellets, whereas 6-in. (152.4 mm) SYP-50 produced comparably higher quality pellets. Higher SYP content led to lower pelleting energy consumption. Changes in pine blend ratio and stem diameter had no significant effect on the elemental composition, ash content, and HHV of the pellets, as shown in Table 5, which shows that blending of 6-in. (152.4 mm) and 2-in. (50.8 mm) pine tops with SG led to homogenization of chemical properties.
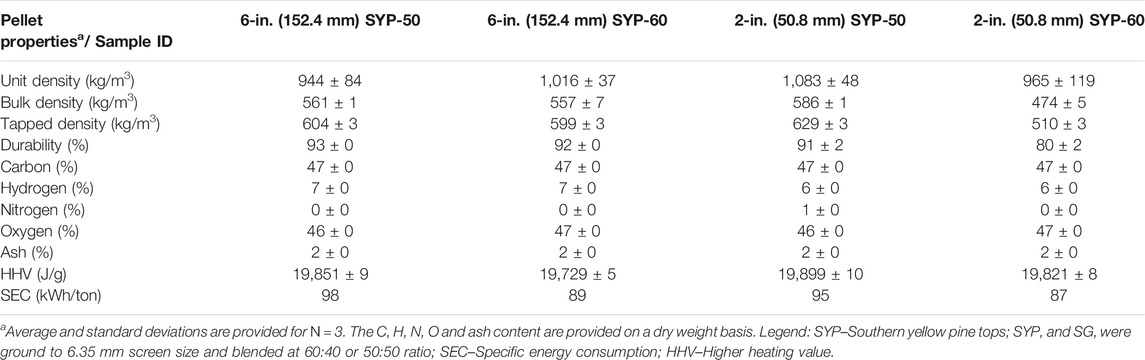
TABLE 5. Average physico-chemical properties of switchgrass (SG) and southern yellow pine (SYP) blended pellets immediately after pelleting produced during 2.5-ton demonstration.
3.3.1 At-Line NIR Spectroscopy of Pellet Outfeed and Multivariate Analysis
The NIR spectra collected at-line on the blended pellets were subjected to PCA and the resulting scores plot showed discernable differences depending on the percentage of SYP content, as well as the size of the SYP tops. As given in Figure 4A, principal component 2 (PC-2) accounted for differences in the percentage of pine content, whereas PC-3 accounted for variations due to the pine tops size. Sample spectra belonging to the 60% pine blends, whether 2-in. (50.8 mm) or 6-in. (152.4 mm) in size, were mainly aggregated in the negative quadrant of PC-2, and the 6-in. (152.4 mm) SYP-60 blend displayed significant grouping compared to the other blends. Based on the PC-1 loadings shown in Figure 4B, the 6-in. (152.4 mm) SYP-60 blend contained higher O–H stretch (first overtone) for cellulose and hemicellulose corresponding to 1,400 nm (Li et al., 2015). This observation is justified by the fact that 6-in. (152.4 mm) SYP contains more cellulose (36%) than the 2-in. (50.8 mm) SYP (29%). Based on the PC-2 loadings, pellets containing 60% pine registered higher–CH3 deformation and C–H stretching in lignin corresponding to 1,375 nm and 1,200 nm (Jin et al., 2017). The pine biomass contained 36–38% of lignin, as opposed to SG with only 21% of lignin, hence, it is plausible that the 60% pine blends contained detectable lignin signature. The PC-3 accounted for minor (3%) variations between the 2-in. (50.8 mm) and 6-in. (152.4 mm) pine tops content, as observed in Figure 4A. According to the PC-3 loadings, shown in Figure 4B, these variations could be attributed to higher C–H stretching in cellulose and hemicellulose corresponding to 1,585 nm and 1,365 nm, respectively, and a higher O–H stretch (second overtone in bound forms) in hemicellulose corresponding to 1,080 nm in the 6-in. (152.4 mm) SYP blends (Jin et al., 2017). Thus, at-line NIR spectroscopy was successfully employed to monitor the changes in chemical signatures as a result of changes in pine blend ratio.
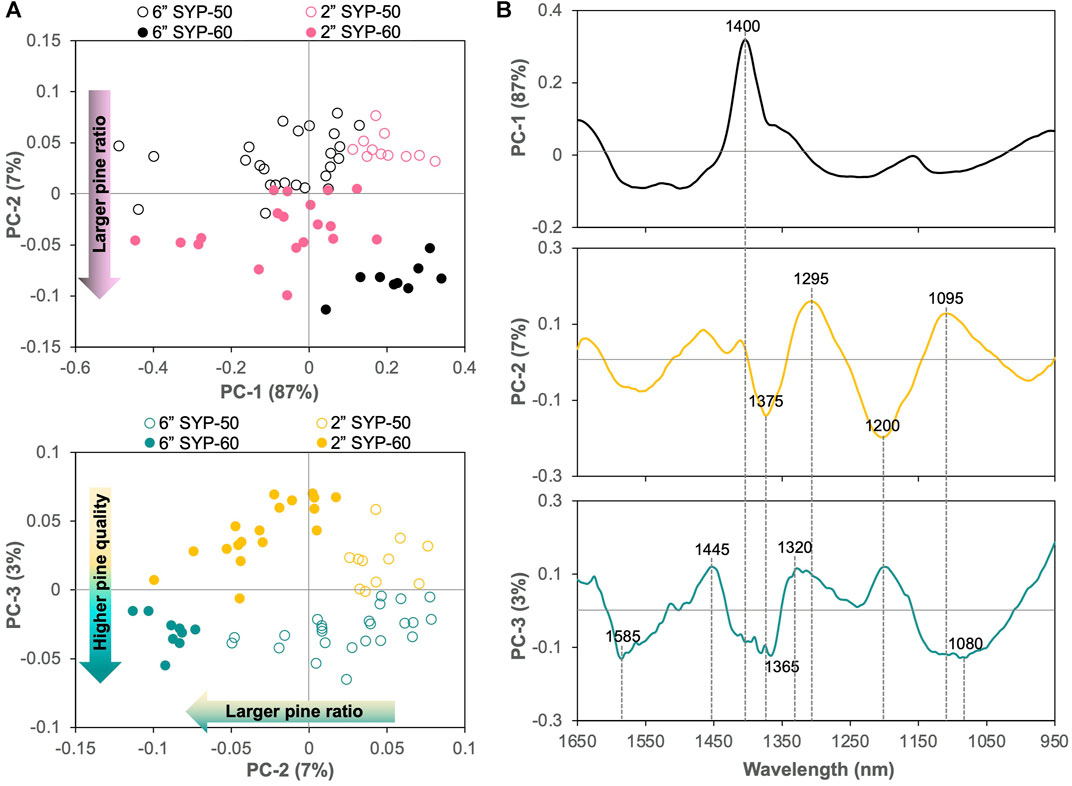
FIGURE 4. (A) Scatter plots comparing the scores of principal components (PC-) 1, 2, and 3. Principal component analysis (PCA) was conducted with NIR spectra of blended southern yellow pine (SYP) and switchgrass (SG) pellets in the 950–1,650 nm region; (B) Line plots of principal component loadings corresponding to PC-1, PC-2, and PC-3. The pellet blends were composed of 6-in. (152.4 mm) or 2-in. (50.8 mm) SYP tops and SG, ground in a hammer mill fitted with a 6.35 mm screen, and mixed at either 60:40 or 50:50 ratio.
PLSR models obtained by combining the pelleting process data with pellet NIR PCA scores showed that, properties, such as ash content, could be predicted to a high coefficient of determination (R2) of 0.96, as observed in Table 6. Other pellet properties namely HHV, moisture content, and carbon content could be predicted to R2c values of 0.85, 0.84, and 0.81, respectively, as shown in Table 6. Figure 5A depicts the scatter plots of prediction results for pellet ash content using a PLSR model trained with NIR PCA scores. Input variables namely feed hopper current, outfeed current, pellet mill speed, and the NIR bands of 1,030, 1,150, and 1,610 nm were determined to be sensitive to predicting the ash content, as per the regression coefficients given in Figure 5B. The signal at 1,610 nm is attributed to the overtone of O–H stretching in bound water (Ma et al., 2020). The regression coefficients for the pellet HHV, moisture, and carbon content are provided in Supplementary Figures S3–S5, respectively. Pelleting process parameters namely steam mass flow, in-feed hopper current, pellet mill speed, and conditioner outlet mash temperature had a strong correlation to predicting the pellet HHV, moisture, and carbon content. Similarly, the NIR band at 1,030 nm had a correlation to pellet moisture and carbon content, whereas the bands at 1,310, 1,460, and 1,620 nm were correlated to pellet HHV. Other input variables, including outfeed speed, steam boiler pressure, and the NIR bands at 1,025, 1,155, 1,375, 1,435 and 1,605 nm, were also identified as major contributors to these pellet properties. Thus, fairly robust PLSR models were built for estimating the ash, moisture, carbon, and HHV content of the blended pellets based on at-line NIR spectroscopy combined with the pellet mill process data.

TABLE 6. Performance of PLSR models for predicting pellet properties during 2.5-ton demonstration using at-line NIR spectroscopy (950–1,650 nm region) and pelleting process data.
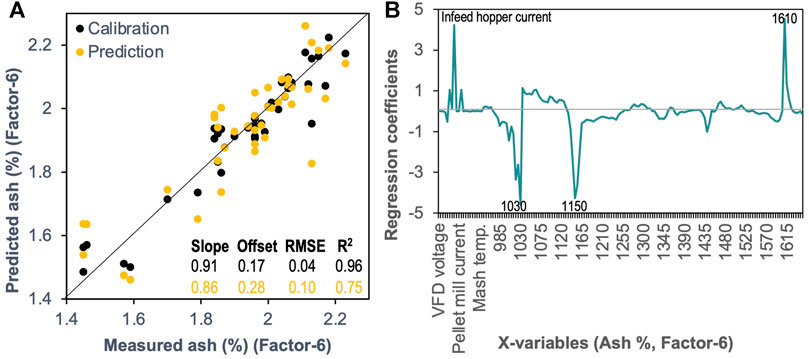
FIGURE 5. (A) Scatter plots of prediction results for ash content (%) of the blended pellets by a PLSR model trained with NIR principal component scores (in 950–1,650 nm region) and pelleting process data; (B) Regression coefficients associated with the PLSR model. The pellet blends were composed of 6-in. (152.4 mm) or 2-in. (50.8 mm) southern yellow pine (SYP) tops and switchgrass (SG), ground in a hammer mill fitted with a 6.35 mm screen and mixed at either 60:40 or 50:50 ratio.
4 Discussion
4.1 Blending Bioenergy Feedstocks
Edmunds et al. (2018) observed that the major advantage of blending woody and herbaceous biomass, such as pine and SG, is the improvement in chemical composition. The limitations of herbaceous biomass for bioenergy production are their lower energy density, issues related to storage, handling, and transportation (Sahoo and Mani, 2016; Sahoo and Mani, 2017). According to Edmunds et al. (2018), Tumuluru et al. (2015), and Sahoo and Mani (2017), herbaceous biomasses typically have a bulk density of 150–160 kg/m3. Ray et al. (2017) concluded that low density biomasses require more resources for transportation and shipping, which increases cost. These limitations pose a severe challenge to the utilization of herbaceous biomass on a commercial scale. In addition, woody biomasses have higher carbon and lower ash contents, whereas herbaceous biomasses have lower carbon and higher ash contents. Blending with woody biomass could therefore help to overcome herbaceous feedstock limitations regarding carbon and ash content and improve their specifications for different conversion scenarios. In thermochemical conversion, desired specifications are high calorific value, and carbon content, but low volatiles, nitrogen, and ash contents. Most herbaceous biomasses, such as corn stover and SG, have higher ash content, lower calorific value, and lower carbon content. Hence, Tumuluru and Fillerup (2020) concluded that blending SG and corn stover with lodgepole pine helped to improve the proximate and ultimate composition and calorific value and made the feedstocks more suitable for preprocessing, such as densification, and for further downstream conversion.
4.2 High-Moisture Pelleting
A major challenge of blending woody and herbaceous biomass is particle segregation during storage, feeding, and handling. One approach to address this challenge is to densify the blended biomass. Tumuluru et al. (2011) suggested that pellet mills, briquette presses, cubers, agglomerators, and tablet presses help to improve the bulk density of biomass feedstocks. Densification not only improves the density, but also avoids particle segregation. Among the various densification systems, pelleting is the most used method for densifying the biomass. But the major challenge in using pellets for biorefinery operations is the cost. Drying biomass to less than 10% (w.b.) moisture content for future pelleting is the major energy consumer in conventional pelleting processes (Lamers et al., 2015). At Idaho National Laboratory we have developed a high-moisture pelleting process that eliminates the high-temperature drying step in pellet production, thus reducing the cost and making pelleting an environment-friendly process. Eliminating high-temperature drying also reduces volatile organic emissions during pelleting. In a techno-economic analysis, Lamers et al. (2015) indicated that a 40% reduction in pellet production costs could be achieved by switching to low temperature dryers, such as grain or belt dryers that operate at a significantly lower cost. In addition, the major advantage of high-moisture pelleting is the versatility of designing pellets with different densities, whereas the conventional method would produce pellets with very high densities (>700 kg/m3) that are more suitable for long-distance transportation. According to Tumuluru (2016), if pellets are transported by truck, very high bulk densities are not needed to fill the truck to its full capacity. If the pellets are to be transported shorter distances, such as 200–300 miles, they do not need to meet the durability standards set for long-distance transportation. Tumuluru (2016) also suggested that the cost of pellet production using conventional methods cannot be completely offset by saving on transportation costs, especially if the transportation distances are less than 200–300 miles. A recent study conducted by Tumuluru and Mwamufiya (2021) found that transportation costs decreased by about 43% for MSW pellets as compared to non-pelleted material if the transportation distance was >15 miles. Therefore, the high-moisture pelleting tested in this study can make pelleting more cost-effective and environmentally friendly for biorefineries, as it avoids the expensive drying methods commonly used by the industry.
4.3 Impact of Blend Composition on Pelleting Process
Biomass physical and chemical properties significantly affected pellet quality. The lignin component of plant biomass is considered as a natural binding agent and plays an essential role in the densification process in terms of pellet quality and energy consumption. In the present study, SYP residues containing higher lignin required less pelleting energy as compared to SG. Also, blending SYP with SG helped to reduce the SEC and improved pellet properties, such as durability. Studies conducted by Tumuluru and Fillerup (2020) on briquetting blends of lodgepole pine, SG, and corn stover showed that the addition of pine decreased energy consumption and increased the durability of SG briquettes. Tumuluru et al. (2012) also found that grasses have lower lignin content, require higher pelleting energy, and produce pellets with lower density and durability. This limitation can be overcome by blending grasses with woody biomass. The current study also indicated that, blending SYP with SG improved the chemical composition—especially the lignin and ash contents—and particle size distribution, which helped to produce good quality pellets at lower energy consumption. In addition, blending improved the pelleting characteristics due to better interlocking ability and flowability of the biomass in the pellet die.
4.4 Impact of Process Parameters on Pellet Properties
Tumuluru and Fillerup (2020) studied the briquetting characteristics of woody and herbaceous biomass blends and concluded that the blend moisture content and screen size of the grind affected briquette quality. A smaller hammer mill screen (4.8 mm size) and a lower blend moisture content of 12% (w.b.) resulted in the maximum unit and bulk density values. Our current results indicated that both moisture content and compression ratio of the die (L/D ratio) affected pellet quality. An L/D ratio of 9 and a lower moisture content of 20% maximized the pellet unit, bulk, and tapped densities. Said et al. (2015) studied the pelleting of rice straw in a flat die mill and showed that the bulk density decreased with an increase in moisture content, as observed in the present study. Studies conducted by Jackson et al. (2016) on corn stover at about 20–26% (w.b.) moisture content showed that pellets with bulk densities between 500 and 600 kg/m3 could be produced. Serrano et al. (2011) used barley straw and came to a similar conclusion that pellet bulk density decreases with an increase in the moisture content of the feedstock. Rhén et al. (2005) investigated the pelleting of Norway spruce at different preheating temperatures and pressures and reported that preheating temperature and moisture content significantly affected the bulk density of the pellets. These observations have been corroborated by the present research findings, whereas higher moisture reduced the bulk density of the SG-SYP pellets.
Studies on rice straw pelleting in a flat die mill by Said et al. (2015) concluded that increasing the moisture content to 17% (w.b.) increased pellet durability. Studies on briquetting the blends of woody and herbaceous biomass by Tumuluru and Fillerup (2020) indicated that durability increased with an increase in hammer mill screen size to 12.7 mm and increasing the moisture content to 15–18% (w.b.). These authors reasoned that increases in briquette durability could be because of the interlocking of larger particles during the compression and extrusion processes. Harun and Afzal (2015) found that higher percentages of woody biomass in the blend of pine and SG increased the pellet strength and durability values. The present research corroborates this observation and supports that the blending SYP with SG produces a good quality pellet in terms of density and durability. Pelleting studies conducted by Tumuluru (2018) using high-moisture woody and herbaceous biomass also determined that woody biomass with higher lignin content produced pellets with higher bulk density and durability. In the present study, pellets produced using pure SYP had higher durability values of >95% at a lower moisture content and higher L/D ratio of the pellet die. For SG, the maximum durability was 95% for a higher L/D ratio of 9 and feedstock moisture content of 20 and 25% (w.b.). The lower durability values of pure SG pellets could be attributed to the lower lignin content. But blending SG with SYP improved the pellet durability at a lower L/D ratio and higher moisture content. Our study also showed that a higher L/D ratio resulted in higher durability of the pellets, which may be because higher residence times of pine and SG particles in the die could induce glass transition of the lignocellulosic components and promote particle binding.
Moisture loss of about 5–10% (w.b.) was observed when SYP and SG blends were pelleted at higher moisture content of 20 and 25% (w.b.). This observation corroborates our earlier works (Tumuluru, 2014; Tumuluru, 2016; Tumuluru, 2019) on corn stover, SG, and lodgepole pine blends, where the loss of moisture depended on the initial moisture content of the feedstock. Higher initial moisture content and larger L/D ratios led to greater moisture losses during pelleting. Moisture losses may occur during pelleting due to flash-off when the pellets are compressed and extruded out of the die. Tumuluru (2016) reasoned that losing moisture during pelleting can be attributed both to frictional heat developed in the die and to further cooling, which can dry most of the pellet surface moisture, resulting in partially dried pellets.
4.5 Application of At-Line and Off-Line NIR Spectroscopy for High-Throughput Prediction of Pellet Properties
Multivariate predictive models constructed based on at-line or in-line NIR spectroscopic analysis could be a valuable resource for high-throughput and inexpensive characterization of chemical and physical changes in lignocellulosic feedstocks during thermochemical or physico-chemical conversion processes (Li et al., 2018; Hwang et al., 2021). Previous research has shown that NIR analysis in the 900–1700 nm region could be coupled with chemometric approaches to predict the cellulose (R2 = 0.92), hemicellulose (R2 = 0.84), and lignin (R2 = 0.71) contents of various types of lignocellulosic pellets like corn stover, rice straw, pine, mahogany, and rubber wood (Feng et al., 2018). PLSR models developed based on NIR spectra have been used to predict HHV (R2 = 0.92), carbon (R2 = 0.85), and ash (R2 = 0.51) content of bamboo culms (Posom and Sirisomboon, 2017). The moisture content (R2 = 0.99) and calorific value (R2 = 0.99) of dedicated bioenergy crops like Miscanthus and short rotation coppice willow have also been predicted with higher precision using the NIR-PLSR models (Fagan et al., 2011). However, prediction of ash content (R2 = 0.58) was poor for these lignocellulosic feedstocks (Fagan et al., 2011).
Our previous study has shown that PLSR models developed based on Fourier transform infrared spectra could predict the ash content of hybrid poplar wood and bark, as well as SG biomass, with a higher accuracy (R2 = 0.98; RMSE = 0.38–0.40%) (Edmunds et al., 2017). In this study, we employed NIR spectroscopy such that we could collect the pellet samples from the outfeed conveyor and perform rapid “at-line” or “off-line” characterizations. Despite the higher moisture content (11–16%) and temperature (50–55°C) of the pellet samples, at-line NIR spectroscopy provided comparable information to that of off-line NIR spectroscopy. Through the integration of pelleting process parameters, we could build a very good PLSR model (R2c = 0.96; RMSE = 0.04–0.1%) for predicting the pellet ash content based on the at-line NIR spectra. However, the prediction accuracy of moisture, HHV, and carbon content was not comparably significant (R2c = 0.81–0.85). Since PLSR modeling of lignocellulosic feedstocks is affected by the number of samples and the chemical composition range of the calibration and validation set (Kline et al., 2016), there is room for future improvement. Thus, at-line NIR spectroscopy was successfully deployed during the pelleting demonstration and multivariate models were developed for determining the chemical composition of blended feedstocks.
The robustness of PLSR prediction models improved significantly with the use of off-line NIR spectroscopy. Despite the smaller sample size (N = 30), wider variability of the pine and SG blends enhanced the prediction capability. Integration of pelleting process information with the NIR PCA scores improved the predictability of pellet pine ratio, ash content, HHV, bulk density, and durability (R2c improved from 0.64 to 0.94) when compared to only using the NIR spectra. In previous research, integration of process temperature with online NIR spectroscopy enabled the PLS prediction of transesterification efficiency and bio-diesel production from soybean oil (Killner et al., 2011). In the near future, NIR-based sensing and multivariate modeling tools could be applied to develop machine learning techniques that can integrate in-/on-line data directly with process controllers to achieve real-time management of bioproduct manufacturing (Gargalo et al., 2020). Our work also provides the incentive for adapting NIR sensing to monitor industrial scale processing of lignocellulosic feedstocks.
Another important aspect of this study is the utilization of at-line NIR spectroscopy to classify the pellets based on the pine quality and blend ratio. In recent times, blending of feedstocks like that of pine and SG are often recommended to offset the uncertainty of continuous feedstock supply, and to improve the economic feasibility of biorefineries (Ray et al., 2017; Lan et al., 2020). Blending of lignocellulosic feedstocks has the advantage of homogenizing the conversion attributes of pellets, namely the moisture and ash contents (Tumuluru et al., 2012; Edmunds et al., 2018; Lan et al., 2020). Hence, developing a rapid NIR-based screening tool for the “quality control” attributes of blended lignocellulosic feedstocks will ensure successful implementation and operations of biomass conversion facilities. We periodically scanned a 2.5-ton feed of blended pine and SG pellets and developed at-line NIR spectroscopy-based PCA models. Results of PCA showed NIR signals from blended pellets containing higher quality pine residues (152.4 mm tops) and larger percentage of pine (60%) could be isolated based on their chemical signature for cellulose, hemicellulose, and lignin. To our knowledge, this will be the first study that utilizes at-line NIR spectroscopy to classify blended lignocellulosic feedstocks that are of notable relevance for bioenergy production in the U.S.
5 Summary and Conclusion
Based on the high-moisture pelleting studies of southern yellow pine (SYP), switchgrass (SG), and their blends, as well as the subsequent large-scale pelleting demonstration, we could draw the following conclusions:
• High-moisture pelleting resulted in 5–10% (w.b.) moisture loss of the blended pellets. Moreover, a higher initial moisture content resulted in greater moisture loss during pelleting. A higher L/D ratio of 7 and 9 also resulted in higher moisture losses.
• Based on our preliminary pelleting tests, an L/D ratio of 9 and blend moisture content of 20% (w.b.) was determined to produce pellets with >95% durability and >500 kg/m3 bulk density.
• In the blended pellets, higher SYP content led to an increase in HHV and a reduction in ash content, which is favorable for further thermochemical conversions.
• Drying the pellets at a lower temperature of 70°C reduced the final moisture content to <10% (w.b.), but also adversely affected the pellet durability and density.
• Specific energy consumption of the high-moisture pelleting process was influenced by the type of feedstock; SYP required the lowest and SG required the highest pelleting energy. Blending SYP with SG moderated the energy consumption.
• Demonstration of high-moisture pelleting using 2.5 ton of 2-in. (50.8 mm) and 6-in. (152.4 mm) SYP tops blended with SG at 50:50 and 60:40 ratio successfully produced pellets with a bulk density between 473 and 586 kg/m3 and durability between 91 and 93%. Energy consumption for the four test blends was between 87 and 98 kWh/ton.
• Partial least square regression models (PLSR), obtained by integrating the pelleting process data with at-line and off-line NIR principal component scores, were successfully developed to predict the pellet pine ratio, ash content, HHV, durability, and bulk density with coefficients of determination (R2) between 0.86 and 0.997.
Data Availability Statement
The original contributions presented in the study are included in the article/Supplementary Material, further inquiries can be directed to the corresponding author.
Author Contributions
JST conceived and conducted the preliminary pelleting studies on southern yellow pine, switchgrass, and the blends of the same. JST, TR, NL, JM, and NA conceived and planned the pelleting demonstration using 2- and 6-inch southern yellow pine top blends with switchgrass. JST, KR, CP, CH, JM, and NA conducted the pilot scale pelleting and NIR spectroscopy experiments, as well as the data analysis. All authors contributed to the preparation of the manuscript.
Funding
Funding for this research was provided by the U.S. Department of Energy’s Logistics for Enhanced-Attribute Feedstocks (LEAF) Project under the Office of Energy Efficiency and Renewable Energy (EERE) Award (#DE-EE0006639).
Author Disclaimer
The views and opinions of the authors expressed herein do not necessarily state or reflect those of the United States government or any agency thereof. Accordingly, the publisher, by accepting the article for publication, acknowledges that the U.S. government retains a nonexclusive, paid-up, irrevocable, worldwide license to publish or reproduce the published form of this manuscript or allow others to do so for U.S. government purposes.
Conflict of Interest
The authors declare that the research was conducted in the absence of any commercial or financial relationships that could be construed as a potential conflict of interest.
Publisher’s Note
All claims expressed in this article are solely those of the authors and do not necessarily represent those of their affiliated organizations, or those of the publisher, the editors and the reviewers. Any product that may be evaluated in this article, or claim that may be made by its manufacturer, is not guaranteed or endorsed by the publisher.
Acknowledgments
The authors thank Genera, LLC (Vonore, TN) for providing the switchgrass biomass used in this study.
Supplementary Material
The Supplementary Material for this article can be found online at: https://www.frontiersin.org/articles/10.3389/fenrg.2021.788284/full#supplementary-material
References
American Society of Agricultural and Biological Engineers (ASABE) Standard S424.1. (1992). Method of Determining and Expressing Particle Size of Chopped Forage Materials by Screening. MI, USA: American Society of Agricultural and Biological Engineers, St. Joseph.
American Society of Agricultural and Biological Engineers (ASABE) Standard S269.4. (2007). Cubes, Pellets, and Crumbles – Definitions and Methods for Determining Density, Durability, and Moisture Content. MI, USA: ASABE, St. Joseph.
Blanco‐Canqui, H., Mitchell, R. B., Jin, V. L., Schmer, M. R., and Eskridge, K. M. (2017). Perennial Warm‐season Grasses for Producing Biofuel and Enhancing Soil Properties: an Alternative to Corn Residue Removal. GCB Bioenergy. 9, 1510–1521. doi:10.1111/gcbb.12436
Chauhan, S. K., Gupta, N., Walia, R., Yadav, S., Chauhan, R., and Mangat, P. S. (2011). Biomass and Carbon Sequestration Potential of Poplar-What Intercropping System in Irrigated Agro-Ecosystem in India. J. Agric. Sci. Technol. A. 1, 575–586.
Chescheir, G., and Nettles, J. (2017). Optimization of Southeastern Forest Biomass Crop Production: Watershed Scale Evaluation of the Sustainability and Productivity of Dedicated Energy Crop and Woody Biomass Operations. Denver, Colorado: The US, DOE Bioenergy Technologies Office (BETO) Project Peer Review. Available at: https://www.energy.gov/sites/prod/files/2017/05/f34/analysis_and_sustainability_chescheir_1.1.1.101.pdf (Accessed August 15, 2021).
Edmunds, C. W., Hamilton, C., Kim, K., André, N., and Labbé, N. (2017). Rapid Detection of Ash and Inorganics in Bioenergy Feedstocks Using Fourier Transform Infrared Spectroscopy Coupled With Partial Least-Squares Regression. Energy Fuels. 31, 6080–6088. doi:10.1021/acs.energyfuels.7b00249
Edmunds, C. W., Reyes Molina, E. A., André, N., Hamilton, C., Park, S., Fasina, O., et al. (2018). Blended Feedstocks for Thermochemical Conversion: Biomass Characterization and Bio-Oil Production From Switchgrass-Pine Residues Blends. Front. Energ. Res. 6, 79. doi:10.3389/fenrg.2018.00079
Fagan, C. C., Everard, C. D., and Mcdonnell, K. (2011). Prediction of Moisture, Calorific Value, Ash and Carbon Content of Two Dedicated Bioenergy Crops Using Near-Infrared Spectroscopy. Bioresour. Technology. 102, 5200–5206. doi:10.1016/j.biortech.2011.01.087
Feng, Q., Chaubey, I., Engel, B., Cibin, R., Sudheer, K. P., and Volenec, J. (2017). Marginal Land Suitability for Switchgrass, Miscanthus and Hybrid poplar in the Upper Mississippi River Basin (UMRB). Environ. Model. Softw. 93, 356–365. doi:10.1016/j.envsoft.2017.03.027
Feng, X., Yu, C., Liu, X., Chen, Y., Zhen, H., Sheng, K., et al. (2018). Nondestructive and Rapid Determination of Lignocellulose Components of Biofuel Pellet Using Online Hyperspectral Imaging System. Biotechnol. Biofuels. 11, 88. doi:10.1186/s13068-018-1090-3
Gargalo, C. L., Udugama, I., Pontius, K., Lopez, P. C., Nielsen, R. F., Hasanzadeh, A., et al. (2020). Towards Smart Biomanufacturing: A Perspective on Recent Developments in Industrial Measurement and Monitoring Technologies for Bio-Based Production Processes. J. Ind. Microbiol. Biotechnol. 47, 947–964. doi:10.1007/s10295-020-02308-1
Hartman, J. C., Nippert, J. B., Orozco, R. A., and Springer, C. J. (2011). Potential Ecological Impacts of Switchgrass (Panicum Virgatum L.) Biofuel Cultivation in the Central Great plains, USA. Biomass and Bioenergy. 35, 3415–3421. doi:10.1016/j.biombioe.2011.04.055
Harun, N. Y., and Afzal, M. T. (2015). Chemical and Mechanical Properties of Pellets Made. Trans. ASABE. 58, 921–930. doi:10.13031/trans.58.11027
Hwang, S.-W., Hwang, U. T., Jo, K., Lee, T., Park, J., Kim, J.-C., et al. (2021). NIR-Chemometric Approaches for Evaluating Carbonization Characteristics of Hydrothermally Carbonized Lignin. Sci. Rep. 11, 16979. doi:10.1038/s41598-021-96461-x
Jackson, J., Turner, A., Mark, T., and Montross, M. (2016). Densification of Biomass Using a Pilot Scale Flat Ring Roller Pellet Mill. Fuel Process. Technology. 148, 43–49. doi:10.1016/j.fuproc.2016.02.024
Jin, X., Chen, X., Shi, C., Li, M., Guan, Y., Yu, C. Y., et al. (2017). Determination of Hemicellulose, Cellulose and Lignin Content Using Visible and Near Infrared Spectroscopy in Miscanthus Sinensis. Bioresour. Technology. 241, 603–609. doi:10.1016/j.biortech.2017.05.047
Killner, M. H. M., Rohwedder, J. J. R., and Pasquini, C. (2011). A PLS Regression Model Using NIR Spectroscopy for On-Line Monitoring of the Biodiesel Production Reaction. Fuel. 90, 3268–3273. doi:10.1016/j.fuel.2011.06.025
Kim, P., Hamilton, C., Elder, T., and Labbé, N. (2018). Effect of Non-Structural Organics and Inorganics Constituents of Switchgrass During Pyrolysis. Front. Energ. Res. 6, 96. doi:10.3389/fenrg.2018.00096
Lamers, P., Roni, M. S., Tumuluru, J. S., Jacobson, J. J., Cafferty, K. G., Hansen, J. K., et al. (2015). Techno-Economic Analysis of Decentralized Biomass Processing Depots. Bioresour. Technology. 194, 205–213. doi:10.1016/j.biortech.2015.07.009
Lan, K., Park, S., Kelley, S. S., English, B. C., Yu, T. H. E., Larson, J., et al. (2020). Impacts of Uncertain Feedstock Quality on the Economic Feasibility of Fast Pyrolysis Biorefineries With Blended Feedstocks and Decentralized Preprocessing Sites in the Southeastern United States. GCB Bioenergy. 12, 1014–1029. doi:10.1111/gcbb.12752
Lane, J. (2018). The Idaho Team that Looped $1 a Gallon of the Cost of the Fuel and How They Did it. Available at: http://www.biofuelsdigest.com/bdigest/2018/09/09/the-idaho-team-that-lopped-1-a-gallon-off-the-cost-of-fuel-and-how-they-did-it/ (Accessed March 15, 2018).
Li, M., He, S., Wang, J., Liu, Z., and Xie, G. H. (2018). An NIRS-Based Assay of Chemical Composition and Biomass Digestibility for Rapid Selection of Jerusalem Artichoke Clones. Biotechnol. Biofuels. 11, 334. doi:10.1186/s13068-018-1335-1
Li, X., Ma, F., Liang, C., Wang, M., Zhang, Y., Shen, Y., et al. (2021). Precise High-Throughput Online Near-Infrared Spectroscopy Assay to Determine Key Cell wall Features Associated With Sugarcane Bagasse Digestibility. Biotechnol. Biofuels. 14, 123. doi:10.1186/s13068-021-01979-x
Li, X., Sun, C., Zhou, B., and He, Y. (2015). Determination of Hemicellulose, Cellulose and Lignin in Moso Bamboo by Near Infrared Spectroscopy. Sci. Rep. 5, 17210. doi:10.1038/srep17210
Ma, T., Inagaki, T., and Tsuchikawa, S. (2020). Rapidly Visualizing the Dynamic State of Free, Weakly, and Strongly Hydrogen-Bonded Water with Lignocellulosic Material During Drying by Near-Infrared Hyperspectral Imaging. Cellulose. 27, 4857–4869. doi:10.1007/s10570-020-03117-6
Mahadevan, R., Adhikari, S., Shakya, R., Wang, K., Dayton, D., Lehrich, M., et al. (2016). Effect of Alkali and Alkaline Earth Metals on Iin-Ssitu Catalytic Fast Pyrolysis of Lignocellulosic Biomass: A Microreactor Study. Energy Fuels. 30, 3045–3056. doi:10.1021/acs.energyfuels.5b02984
M. Kline, L., Labbé, N., Labbé, N., Boyer, C., Edward Yu, T., C. English, B., et al. (2015). Investigating the Impact of Biomass Quality on Near-Infrared Models for Switchgrass Feedstocks. AIMS Bioeng. 3, 1–22. doi:10.3934/bioeng.2016.1.1
Moroń, W., and Rybak, W. (2015). NOx and SO2 Emissions of Coals, Biomass and Their Blends Under Different Oxy-Fuel Atmospheres. Atmos. Environ. 116, 65–71. doi:10.1016/j.atmosenv.2015.06.013
National Renewable Energy Laboratory (2008). Learning about Renewables. Available at: http://www.nrel.gov/learning (Accessed May 7, 2008).
Ou, L., Luo, G., Ray, A., Li, C., Hu, H., Kelley, S., et al. (2018). Understanding the Impacts of Biomass Blending on the Uncertainty of Hydrolyzed Sugar Yield From a Stochastic Perspective. ACS Sustainable Chem. Eng. 6, 10851–10860. doi:10.1021/acssuschemeng.8b02150
Ozaki, Y., Genkawa, T., and Futami, Y. (2017). “Near-infrared Spectroscopy,” in Encyclopedia of Spectroscopy and Spectrometry. Editors J. Lindon, G. E. Tranter, and D. Koppenall (Cambridge, MA: Academic Press), 40–49. doi:10.1016/b978-0-12-409547-2.12164-x
Posom, J., and Sirisomboon, P. (2017). Evaluation of the Higher Heating Value, Volatile Matter, Fixed Carbon and Ash Content of Ground Bamboo Using Near Infrared Spectroscopy. J. Near Infrared Spectrosc. 25, 301–310. doi:10.1177/0967033517728733
Prestemon, J. P., and Abe, R. (2002). “Timber Products Supply and Demand,” in ” in Southern Forest Resource Assessment. Editors D. Wear, and J. G. Greis (Asheville, NC: USDA-Forest Service, Southern Research Station), 299–325.
Ray, A. E., Li, C., Thompson, V. S., Daubaras, D. L., Nagle, N., and Hartley, D. S. (2017). “Biomass Blending and Densification: Impacts on Feedstock Supply and Biochemical Conversion Performance,” in Biomass Volume Estimation and Valorization for Energy. Editor J. S. Tumuluru (Idaho Falls, ID: InTech), 1–22. doi:10.5772/67207
Rhén, C., Gref, R., Sjöström, M., and Wästerlund, I. (2005). Effects of Raw Material Moisture Content, Densification Pressure and Temperature on Some Properties of Norway spruce Pellets. Fuel Process. Technology 87, 11–16. doi:10.1016/j.fuproc.2005.03.003
Risser, P. G., Birney, E. C., Blocker, H. D., May, S. W., Parton, W. J., and Wiens, J. A. (1981). The True Prairie Ecosystem. Stroudsburg, PA: Hutchinson Ross Publications.
Sahoo, K., and Mani, S. (2016). Engineering Economics of Cotton Stalk Supply Logistics Systems for Bioenergy Applications. Trans. ASABE. 59, 737–747. doi:10.13031/trans.59.11533
Sahoo, K., and Mani, S. (2017). Techno‐economic Assessment of Biomass Bales Storage Systems for a Large‐Scale Biorefinery. Biofuels, Bioprod. Bioref. 11, 417–429. doi:10.1002/bbb.1751
Said, N., Abdel Daiem, M. M., García-Maraver, A., and Zamorano, M. (2015). Influence of Densification Parameters on Quality Properties of rice Straw Pellets. Fuel Process. Technology. 138, 56–64. doi:10.1016/j.fuproc.2015.05.011
Serrano, C., Monedero, E., Lapuerta, M., and Portero, H. (2011). Effect of Moisture Content, Particle Size and Pine Addition on Quality Parameters of Barley Straw Pellets. Fuel Process. Technology. 92, 699–706. doi:10.1016/j.fuproc.2010.11.031
Sluiter, A., Hames, B., Ruiz, R., Scarlata, C., Sluiter, J., and Templeton, D. (2005). “Determination of Ash in Biomass,” in NREL Laboratory Analytical Procedure (Golden, CO: National Renewable Energy Laboratory), 1–12.
Suyker, A. E., and Verma, S. B. (2001). Year-round Observations of the Net Ecosystem Exchange of Carbon Dioxide in a Native Tallgrass Prairie. Glob. Change Biol. Bioenergy. 7, 279–289. doi:10.1046/j.1365-2486.2001.00407.x
Tumuluru, J., Lim, C., Bi, X., Kuang, X., Melin, S., Yazdanpanah, F., et al. (2015). Analysis on Storage Off-Gas Emissions From Woody, Herbaceous, and Torrefied Biomass. Energies. 8, 1745–1759. doi:10.3390/en8031745
Tumuluru, J. (2019). Pelleting of pine and Switchgrass Blends: Effect of Process Variables and Blend Ratio on the Pellet Quality and Energy Consumption. Energies. 12, 1198. doi:10.3390/en12071198
Tumuluru, J. S. (2018). Effect of Pellet die Diameter on Density and Durability of Pellets Made From High Moisture Woody and Herbaceous Biomass. Carbon Resour. Convers. 1, 44–54. doi:10.1016/j.crcon.2018.06.002
Tumuluru, J. S. (2014). Effect of Process Variables on the Density and Durability of the Pellets Made From High Moisture Corn Stover. Biosyst. Eng. 119, 44–57. doi:10.1016/j.biosystemseng.2013.11.012
Tumuluru, J. S., and Fillerup, E. (2020). Briquetting Characteristics of Woody and Herbaceous Biomass Blends: Impact on Physical Properties, Chemical Composition, and Calorific Value. Biofuels, Bioprod. Bioref. 14, 1105–1124. doi:10.1002/bbb.2121
Tumuluru, J. S., Hess, J. R., Boardman, R. D., Wright, C. T., and Westover, T. L. (2012). Formulation, Pretreatment, and Densification Options to Improve Biomass Specifications for Co-Firing High Percentages With Coal. Ind. Biotechnol. 8, 113–132. doi:10.1089/ind.2012.0004
Tumuluru, J. S., and Mwamufiya, M. (2021). FCIC DFO – Moisture Management and Optimization in Municipal Solid Waste Feedstock Through Mechanical Processing. Available at: https://www.energy.gov/sites/default/files/2021-04/beto-12-peer-review-2021-fcic-tumuluru.pdf (Accessed August 15, 2021).
Tumuluru, J. S. (2016). Specific Energy Consumption and Quality of Wood Pellets Produced Using High-Moisture Lodgepole Pine Grind in a Flat die Pellet Mill. Chem. Eng. Res. Des. 110, 82–97. doi:10.1016/j.cherd.2016.04.007
Tumuluru, J. S., Wright, C. T., Hess, J. R., and Kenney, K. L. (2011). A Review of Biomass Densification Systems to Develop Uniform Feedstock Commodities for Bioenergy Application. Biofuels, Bioprod. Bioref. 5, 683–707. doi:10.1002/bbb.324
U.S. Department of Energy (DOE) (2016). Billion-ton Report: Advancing Domestic Resources for a Thriving Bioeconomy—Volume 1: Economic Availability of Feedstocks. ORNL/TM‐2016/160. Oak Ridge, TN, USA: Oak Ridge National Laboratory.
U.S. Environmental Protection Agency (EPA) (2018). Overview for Renewable Fuel Standard. Available at: https://www.epa.gov/renewable-fuel-standard-program/overview-renewable-fuel-standard (Accessed October 9, 2018).
Williams, C. L., Emerson, R., and Tumuluru, J. S. (2017). “Biomass Compositional Analysis for Conversion to Renewable Fuels and Chemicals,” in Biomass Volume Estimation and Valorization for Energy. Editor J. S. Tumuluru, IntechOpen, 251–270. doi:10.5772/65777
Yancey, N. A., Tumuluru, J. S., and Wright, C. T. (2013). Drying, Grinding and Pelletization Studies on Raw and Formulated Biomass Feedstock's for Bioenergy Applications. J. Biobased Mat Bioenergy. 7, 549–558. doi:10.1166/jbmb.2013.1390
Keywords: southern yellow pine, switchgrass, blends, high moisture pelleting, physical properties, chemical composition, near infrared spectroscopy, multivariate modeling
Citation: Tumuluru JS, Rajan K, Hamilton C, Pope C, Rials TG, McCord J, Labbé N and André NO (2022) Pilot-Scale Pelleting Tests on High-Moisture Pine, Switchgrass, and Their Blends: Impact on Pellet Physical Properties, Chemical Composition, and Heating Values. Front. Energy Res. 9:788284. doi: 10.3389/fenrg.2021.788284
Received: 01 October 2021; Accepted: 09 November 2021;
Published: 17 January 2022.
Edited by:
Zia Haq, United States Department of Energy (DOE), United StatesReviewed by:
Macmanus Ndukwu, Michael Okpara University of Agriculture, NigeriaHui Zhou, Tsinghua University, China
Copyright © 2022 Tumuluru, Rajan, Hamilton, Pope, Rials, McCord, Labbé and André. This is an open-access article distributed under the terms of the Creative Commons Attribution License (CC BY). The use, distribution or reproduction in other forums is permitted, provided the original author(s) and the copyright owner(s) are credited and that the original publication in this journal is cited, in accordance with accepted academic practice. No use, distribution or reproduction is permitted which does not comply with these terms.
*Correspondence: Jaya Shankar Tumuluru, JayaShankar.Tumuluru@inl.gov