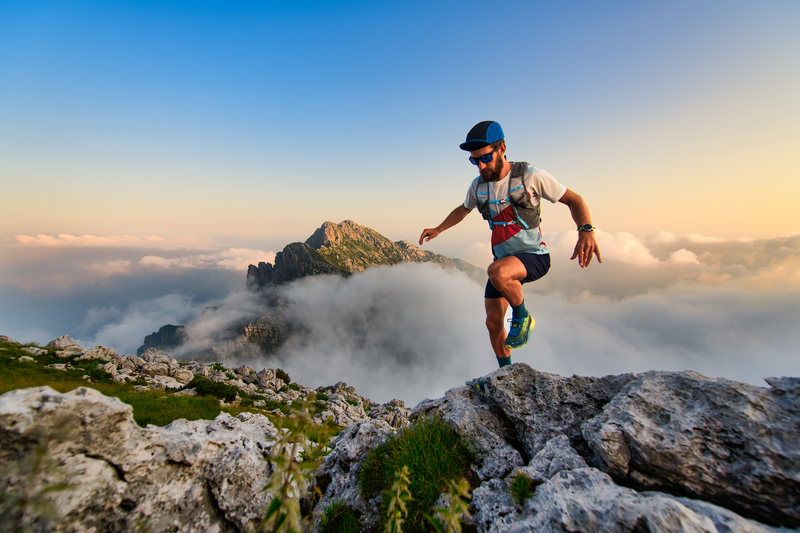
95% of researchers rate our articles as excellent or good
Learn more about the work of our research integrity team to safeguard the quality of each article we publish.
Find out more
HYPOTHESIS AND THEORY article
Front. Energy Res. , 29 October 2021
Sec. Bioenergy and Biofuels
Volume 9 - 2021 | https://doi.org/10.3389/fenrg.2021.760895
This article is part of the Research Topic High-performance Catalytic Processes for Biofuel Production View all 6 articles
In the process of continuously advancing urbanization, the energy efficiency of the construction industry, as a pillar industry of the national economy, has attracted much attention. The imbalance of regional economic development, the difference of regional resource endowment, and the incomplete energy infrastructure in some areas lead to the prominent problem of energy poverty in China. Based on the panel data of 30 provinces and cities from 2008 to 2017, this paper calculates the energy poverty index and the energy efficiency of the construction industry. Using OLS, Tobit, Probit, and other estimation methods, this paper analyzes the internal relationship between energy poverty and the energy efficiency of the construction industry, the mediating role of energy consumption structure, and the moderating effect of technological level and marketization degree. The results show the following: There is a negative correlation between energy poverty and the energy efficiency of the construction industry (Husnain, ENERG POLICY, 2021, 157). The energy consumption structure plays a partial mediating role in the impact of energy poverty on the energy efficiency of the construction industry (Li, CHEM ENG J, 2021, 408). It means that energy poverty inhibits the optimization of the energy consumption structure, and through this process, it also inhibits the improvement of the energy efficiency of the construction industry. The technological level and the degree of marketization play a moderating role in the main effect, which can weaken and strengthen the negative impact of energy poverty on the energy efficiency of the construction industry (Gajdzik, ENERGIES, 2021, 14).
Energy is the material basis for human production activities and the key to high-quality development of a country. As a pillar industry of the national economy, the energy efficiency of the construction industry has also been widely concerned by different aspects of society. The 13th Five-Year Comprehensive Plan for Energy Conservation and Emission Reduction issued by The State Council of China stated that the construction industry should strengthen the binding force of energy conservation and environmental protection, formulate the development plan of the whole industrial chain of green buildings, and stressed that the energy used in the construction and renovation of buildings should meet the energy saving standards, so that the total energy consumption should be controlled under 5 billion tons of standard coal. The targets set in the document demonstrate China's commitment to low-carbon development. Saving energy and reducing consumption and carbon emissions are both important measures for China to fulfill its emission reduction commitments made at the 15th Conference of the Parties to the United Nations Framework Convention on Climate Change (UNFCCC) and the only way to build a beautiful China as outlined in the work report of the 19th CPC National Congress. China’s low utilization of modern clean energy means that it is difficult to obtain and use modern energy, which is also known as energy poverty in the international community. China’s use of advanced clean energy is not high, that is, it is difficult to obtain and use modern energy, which is also known as energy poverty in the world (Husnain et al., 2021). China is actively expanding new energy sectors, including wind, solar, and biomass energy. As an important research object in biomass energy, biodiesel has been studied and improved by many scholars on its preparation technology and methods and is expected to replace diesel as the main clean fuel (Li et al., 2021). At the same time, the energy consumption structure tends to coal and other fossil energy, and the restriction of technology and process also causes energy waste, which has an impact on the energy efficiency of the construction industry to a certain extent (Gajdzik et al., 2021). So how will energy poverty affect the energy efficiency of the construction industry? Will energy poverty lead local businesses to use clean energy? What is the transmission mechanism of the impact of energy poverty on the energy efficiency of the construction industry? These questions are the focus of our research. Therefore, this paper focuses on the imbalance of energy ownership and energy structure and explores its impact on the energy efficiency of the construction industry, from the perspective of energy poverty. Clarifying the logical relationship among energy poverty, energy consumption structure, and energy efficiency of the construction industry is helpful for governments at all levels to formulate relevant energy policies.
Compared with existing studies, the possible contributions of this paper are as follows: At present, energy efficiency research is mainly elaborated from the aspects of energy consumption structure, industrial structure, etc. This paper will start with the logical relationship among energy poverty, energy consumption structure, and energy efficiency and explain that energy poverty inhibits the improvement of energy efficiency and the existence of some mediating effects. The energy consumption structure is affected by regional energy poverty, and the clean energy consumption structure affects the improvement of the energy efficiency of the construction industry (Li et al., 2021). In this paper, the energy consumption structure is taken as an intermediary variable, and the research results show that the weakening of energy poverty will lead to a cleaner and rationalized energy consumption structure in the region, which together will improve the energy efficiency of the regional construction industry and also provide new ideas for the regional government to formulate energy conservation and consumption reduction policies.
The structure of this paper is as follows: Domestic and foreign scholars’ studies on energy poverty and energy efficiency are sorted out, and the theoretical basis is determined (Husnain et al., 2021). The energy poverty index and the energy efficiency of the construction industry are calculated for 30 provinces and cities in China, the impact model of the energy efficiency of the construction industry is built, and the impact of energy poverty on the energy efficiency of the construction industry is analyzed with three measurement methods (Li et al., 2021). The empirical results are tested to ensure the accuracy of the results (Gajdzik et al., 2021). Finally, we conclude this paper and provide enlightenment (Nathan and Hari, 2020).
On the research of energy poverty, domestic and foreign scholars mainly focus on the definition of the concept of energy poverty and the measurement of energy poverty. In 1982, energy poverty was first defined in the United Kingdom Fuel Right Movement. At that time, energy poverty mainly meant that people could not afford to use the energy services. With the development of The Times, scholars have paid more attention to the availability of modern energy in developing countries. The International Energy Agency (IEA) regards the lack of access to modern electricity services, or the inability to afford electricity services, as a basic manifestation of energy poverty, resulting in large consumption of traditional biomass energy. Hippu and Nathan (2020) proposed a measure of energy poverty based on the deprivation of modern cooking and lighting fuels and classified energy poverty into transitional, moderate, and extreme levels in terms of depth and severity (Nathan and Hari, 2020). Kahouli and Okushima (2021) made a comparative study of energy poverty and energy inequality in France and Japan and used a direct measurement method to measure energy poverty, that is, determine energy poverty according to the actual household energy services used by each household (Kahouli and Okushima, 2021). Li Shixiang and Li Lijuan (2020) constructed the energy development index (EDI) from three aspects of economic situation, regional natural environment, and farmers themselves and measured the rural energy poverty in 31 provinces, cities, and districts of China (Li and Li, 2020). In this paper, the definition of energy poverty in China is based on the concept of Li et al. (2014): China has difficulties in equitable access to and safe consumption of adequate, affordable, high-quality, and potential energy (Li et al., 2014).
In the 1974 edition of Encyclopaedia Britannica, energy efficiency was defined as “the ratio in economics of output to the energy input required to produce it.” Inspired by the theoretical framework of total factor production, Hu and Wang (2006) proposed the concept of total factor (elements) energy efficiency for the first time (Jin-Li and Shih-Chuan, 2005). Accordingly, this paper defines the energy efficiency of the construction industry as the ratio of the target amount of energy required to be invested to the actual amount of energy in order to achieve a certain output value of the construction industry by comprehensively considering the input factors such as energy, capital, and labor.
Domestic and foreign scholars’ research on energy efficiency mainly focuses on energy efficiency measurement and energy efficiency–influencing factors. Mohsin (2021) took the data of 48 countries in five regions as samples and took the electric power reform as an example to investigate the impact of energy reform on energy efficiency by DEA and DID methods (Mohsin et al., 2021). Reuter et al. (2020) developed a measurement method consisting of 20 indicators to measure the multiple benefits of energy efficiency and explained the data sources and limitations of the method (Reuter et al., 2020). Chang (2020) proposed a new calculation model for the infeasible problem of total factor energy efficiency under the metafrontier framework and summarized the development conditions of renewable energy (Chang, 2020). By studying the connection between energy efficiency and total factor productivity of Indian paper mills, Haider and Bhat (2020) suggested that the government should provide differentiated help for industry characteristics and its own capabilities and promote coordination and cooperation between countries and states to obtain benefits of technological progress through technology diffusion and transfer (Haider and Bhat, 2020). Wang et al. (2021) measured the energy efficiency of the construction industry and explored the key influencing factors including the development degree of the construction industry, industrial concentration, and energy structure under the condition that carbon dioxide emissions were taken as the undesirable output (Wang et al., 2021). Hou and Chen (2020) defined a new total factor energy efficiency formula according to the characteristics of the transportation industry and made an empirical analysis of its influencing factors. The study showed that the technical efficiency and the proportion of electricity in energy consumption were significantly positively correlated with the energy efficiency of the transportation industry (Hou et al., 2020). Hu and Li (2019) established the Super-SBM model to analyze the construction of China’s energy efficiency from the provincial level. It was found that, in recent years, the influence of technology to improve the energy efficiency was limited; especially when there was a big difference between autonomous regional and municipal levels, the means of the future should focus on strengthening market regulation and strengthening regional cooperation (Hu et al., 2019). Zhu et al. (2019) proposed a building construction process model based on Cobb–Douglas production function and estimated the impact of technological progress on energy efficiency. The results showed that technological progress increased energy efficiency by 7.1% per year on average from 1997 to 2014 (Weina et al., 2019).
Existing research suggests that energy poverty has an inhibiting effect on the improvement of the energy efficiency of the construction industry. Sofia et al. (2019) studied the energy efficiency measures adopted by Greek households to overcome the cold during the economic crisis in the form of a questionnaire. The study showed that 37% of the samples faced the problem of energy poverty, and about half of the samples could not afford to improve the energy efficiency of their homes (Sofia-Natalia and Agis, 2019). Qian and Jin (2021) explored the relationship between energy efficiency and resource endowment in 17 provinces along the “One Belt and One Road” policy from the perspective of “resource curse,” concluded that there was a significant “resource curse” phenomenon in areas with high energy reserves, and explored the mitigating effect of environmental regulations (Qian and Jin, 2021). In 2015, Li proposed a new index of ecological total factor energy productivity to comprehensively and systematically analyze the energy efficiency of each region in China. The results show that resource-based cities fall into the “resource curse.” The more energy rich the regions are, the less optimistic the development of ecological total factor energy productivity is, that is, it has a negative impact (Li, 2015).
Based on the above research, the following hypothesis is proposed:
H1: Energy poverty has a negative impact on the energy efficiency of the construction industry.
In recent years, China’s energy dependence on the outside world is high. On the one hand, China itself is short of energy resources, and the storage of oil, natural gas, and other resources is far below the world’s average level. On the other hand, it is an extensive energy economy based on coal, with an unreasonable energy consumption structure and extremely serious energy waste in production and consumption, which also seriously aggravates the contradiction between supply and demand. To predict whether carbon dioxide emissions will peak by 2050, Xu and Schwarz (2020) divided energy consumption into energy consumption and energy consumption structure. The dynamic changes of energy consumption and carbon emissions were explored under three hypothetical scenarios of business-as-usual, planned energy structure, and low-carbon energy structure. It could be found that coal consumption would gradually decline, while the consumption of natural gas and non-fossil energy would increase year by year, and the whole energy consumption structure would tend to a cleaner and modernized balance, thus affecting energy efficiency (Xu et al., 2020). Li Ying and Li Zilian (2020) analyzed the relationship between energy consumption and GDP in Shanxi Province from 2000 to 2016, and found that coal was highly correlated with GDP. Such energy consumption structure highly dependent on coal inhibited the transformation of Shanxi Province to green and low-carbon economic development and affected regional energy efficiency (Li and Li, 2020). Coal and other traditional energy sources with high energy consumption, high pollution, and low efficiency account for a large proportion of energy consumption, so the energy utilization efficiency is relatively low. Energy efficiency will be improved if clean energy sources such as natural gas and electricity make up the bulk of the energy consumption mix. In conclusion, this paper believes that energy poverty will inhibit the cleaning and modernization of the energy consumption structure in the region and thus the improvement of the energy efficiency of the construction industry.
Based on the above research, the following hypothesis is proposed:
H2: The energy consumption structure plays an intermediary role in the impact of energy poverty on the energy efficiency of the construction industry.
According to the theory of economic development and market freedom in western economics, the market plays an important role in the allocation of resources. Theoretically, the higher the degree of industrial marketization is, the more efficient the allocation of resources can be, and the optimal one can be achieved by reducing waste. Wei and Zheng et al. (2020), starting from the new perspective of market segmentation, believed that the market system is the key factor affecting the improvement of energy efficiency, put forward the hypothesis and verified it, and finally came to the conclusion that the existence of market segmentation significantly inhibits the impact of scale efficiency, technical efficiency, and allocation efficiency on energy efficiency (Chu and Xinye, 2020). In the study of industrial energy efficiency, Li and Shi (2018) put forward two aspects of marketization: the degree of economic marketization and the external market environment, which are the main obstacles to the gap (Li and Shi, 2018).
So let us propose Hypothesis H3 as follows:
H3: The degree of marketization plays a moderating role in the impact of energy poverty on the energy efficiency of the construction industry.
Nowadays, it is not enough to improve energy efficiency only by optimizing the energy structure and optimizing the allocation of resources in the market. Technology is always the primary productive force. Advanced processes and technologies can be introduced to improve the working efficiency of equipment and directly reduce the energy consumption per unit product, so as to improve energy efficiency. Wu and Ge et al. (2020) studied the influence of industrial agglomeration degree on agricultural energy efficiency and proposed to strengthen technological innovation and sharing of green agricultural development to promote the optimization of agricultural and rural energy structure (Wu et al., 2020). Liao and Ren et al. (2020) found that there is a threshold for energy bias technologies, below which the progress of energy bias technologies will have a positive impact on energy efficiency. Beyond that, the effect will be negative. Since this effect is not one-way, they define it as the “double-edged sword effect” (Liao and Ren, 2020). At the same time, Zhou and Kong (2018) also have similar conclusions that technological progress has different effects on energy efficiency in regions with different energy efficiency performance, especially “rebound” in regions with low energy efficiency (Zhou and Kong, 2018). Peng et al. (2019) found through research that the average level of total factor energy efficiency in China is on the decline and the technical level is also on the decline (Peng and Zhang, 2019). However, as an effective means to reduce energy consumption, technological progress often does not play a role alone. For example, the shift of the energy consumption structure to more efficient energy requires certain technical support, in which the technological level plays a regulating role. Hypothesis H4 is proposed as follows.
H4: The technical level plays a moderating role in the impact of energy poverty on the energy efficiency of the construction industry.
The original data of energy efficiency and energy consumption structure of the construction industry are from China Energy Statistical Yearbook. The technical level, the added value of the construction industry, and the degree of opening to the outside world are from China Construction Statistical Yearbook. The industrial development degree, the auxiliary industry development of the construction industry, and the number of construction employees are from China Statistical Yearbook. The degree of marketization comes from the report of China’s marketization index compiled by Fan et al. Due to the availability of data, the above data range covers the period 2008–2017 and covers 30 provinces in China.
1) Dependent variable. The dependent variable in this paper is the energy efficiency of the construction industry. Considering the limitation of the energy efficiency of the construction industry, the input-oriented CCR model of data envelopment analysis is selected for calculation. According to Wilson et al. (1994), Chen et al. (2021), Yang et al. (2021), and Yu and Qu (2021), labor force, energy, and capital were selected as input vectors and the total output value of the construction industry in each region was selected as the output vector. The formula is as follows (Husnain et al., 2021):
Here, θ represents the energy efficiency of construction enterprises in the k province, Xj represents the input factor vector of the construction industry in the j province, Yj represents the output vector of the construction industry in the j province, λj is the weight of the j province, S− is the slack variable of the input, and S+ is the slack variable of the output. The energy efficiency of the construction industry is calculated by the following formula (Li et al., 2021):
where EE(i,t) is the total factor energy efficiency of the construction industry in the i province in the period of t, EImb(i,t) is the energy input of the construction industry in the i province at the target point of the most productive frontier in the period of t, and EIsj(i,t) is the actual energy input of the construction industry in the i province in the period t. The calculation results of the energy efficiency of the construction industry in China’s provinces during 2008–2017 are shown in Table 1.
2) Independent variable. The independent variable in this paper is energy poverty. Based on the literature review and theoretical analysis mentioned above, this paper establishes the evaluation index of energy poverty. It includes refrigerators per 100 urban households, electricity consumption per capita, natural gas consumption per capita, air conditioning per 100 urban households, urban gas penetration rate, solar water heater per capita coverage area in rural areas, methane production per capita in rural areas, and range hood ownership per 100 rural areas. The weight of the energy poverty index was determined according to the method of determining the weight of the energy development index, and the index was calculated. The higher the energy poverty index, the more serious the energy poverty in the region. The formula is as follows (Gajdzik et al., 2021):
Here, EP is the energy poverty index of the i province, wj is the weight of the j index, and yij is the standardized value of the j index of province i. The calculation results of the energy poverty index of China’s provinces from 2008 to 2017 are shown in Table 2.
3) Mediating variables and moderating variables. The intermediate variable in this paper is the energy consumption structure. China has been in the production of coal consumption economy for a long time, and the impact of coal consumption structure has always been the focus of research. In recent years, with China’s increasing attention on resource and environmental protection, as well as its remarkable achievements in the development of renewable resources such as hydropower, biogas facilities, wind power, and nuclear power, the proportion of coal consumption in China has been declining, followed by more people choosing clean energy such as electricity and natural gas. In recent years, in the study of the energy consumption structure, scholars mostly use the proportion of electricity consumption in the total energy consumption or the proportion of coal consumption in the total energy consumption to represent the energy consumption structure (Gao et al., 2020; Xu et al., 2020; Zhang et al., 2020; Chen et al., 2021). Considering the availability of data and the characteristics of energy consumption structure in various provinces in recent years, the ratio of electricity consumption in the construction industry to total energy consumption in the construction industry is chosen to represent the energy consumption structure.
The adjusting variables are the marketization degree and technology level. The index of marketization degree can be expressed by the ratio of the total output value of non-state-owned construction enterprises in the region to the total output value of the construction industry in the region (Wang and Huang, 2021). It can also be expressed by Fan Gang et al.’s “China Marketization Index: Report on the Relative Process of Marketization in Different Regions in 2009.” Considering the authoritativeness and universality of application, the latter was chosen. The technical level index is represented by the technical equipment rate or R&D/GDP (Feng and Wang, 2015; Li et al., 2020), and R&D/GDP is chosen in this study to represent the technical level.
4) Control variables. Drawing on the research of Yu (Gao et al., 2020; Xu et al., 2020; Zhang et al., 2020; Chen et al., 2021), Yang (Gao et al., 2020; Xu et al., 2020; Zhang et al., 2020; Chen et al., 2021), and Li (Gao et al., 2020; Xu et al., 2020; Zhang et al., 2020; Chen et al., 2021), factors such as the industrial development degree, opening degree, auxiliary industry development of the construction industry, added value of the construction industry, and number of construction industry employees are selected as control variables.
Through the foregoing analysis, it is found that energy poverty affects the energy efficiency of the construction industry by affecting the energy consumption structure. The model is constructed as follows: (Nathan and Hari, 2020) - (Jin-Li and Shih-Chuan, 2005). The model of Nathan and Hari (2020) is the regression of the impact of energy poverty on the energy efficiency of the construction industry. The model of Kahouli and Okushima (2021) is the regression of energy poverty to the energy consumption structure. The model of Li and Li (2020) is the regression of energy poverty, energy consumption structure, and energy efficiency of the construction industry. The model of Li et al. (2014) and Jin-Li and Shih-Chuan (2005) is based on the model of Gajdzik et al. (2021) to add the moderating variables and the interaction term between the moderating variables and the core explanatory variables for regression:
Here, EEit is the observed energy efficiency of the construction industry in the i province in the t year and is the explained variable; EPit is the energy poverty index of the i province in the t year and is the explanatory variable; α0, β0, χ0, φ0, and ψ0 are intercept terms; α1-αj, β1-βj, χ1-χj, φ1-φj, and ψ1-ψj are regression coefficients; ECSit is the energy consumption structure of the i province in the t year, MDit is the marketization degree of the i province in the t year, and TLit is the technology level of the i province in the t year; Controlijt is the j control variable in year t and province i; Year is the dummy variable, and δt is its regression coefficient; εit is the residual; EPit*MDit is the cross product of energy poverty and marketization degree; and EPit*TLit is the cross product of energy poverty and technology level.
In this study, the energy efficiency of the construction industry is taken as the explained variable, and the OLS (ordinary least square) model, Tobit model, and Probit model are, respectively, used to study the impact of energy poverty on the energy efficiency of the construction industry. When Probit regression is carried out, because it is a binary selection model, the following processing is needed for the energy efficiency and energy consumption structure of the construction industry when conducting the mediation effect test. The value is turned into a binary selection model to meet the regression conditions, where the boundary value is the mean value, as shown in the model of Mohsin et al. (2021).
In order to more intuitively understand the impact of energy poverty, energy consumption structure, technological level, and marketization degree on the energy efficiency of the construction industry, this paper conducted a descriptive statistical analysis of variables on 300 effective samples from 30 provinces and cities from 2008 to 2017. The specific results are shown in Table 3.
As can be seen from Table 3, the average value of energy efficiency of the sample construction industry is 0.83, the maximum value is 1, and the minimum value is 0.51, indicating that the energy efficiency of the construction industry varies greatly. The maximum value of the energy poverty index is 0.935, the minimum value is 0.280, and the average value is 0.634, which indicates that there are great differences in energy poverty among different regions. Whether there is a relationship between these two differences is the research content of this paper. The mean value of the energy consumption structure is 0.24, the maximum value is 0.88, and the minimum value is 0.03. Such inter-provincial differences in the energy consumption structure are also the object of exploration for the relationship between energy poverty and the energy efficiency of the construction industry. The standard deviations of each variable are all less than the mean, indicating that the data have no extreme values to affect the results, and the standard deviation is not much different from the mean. The industrial development degree, external development degree, construction industry employees, construction industry’s auxiliary industry development degree, and construction industry’s added value reflect the development status of the construction industry, indicating that the sample is reliable.
In order to preliminarily verify the basic assumptions of this paper, this paper carries out correlation coefficient tests on energy efficiency, energy poverty, energy consumption structure, technology level, marketization degree, and control variables of the construction industry. The specific variables are shown in Table 4.
As shown in Table 4, the energy efficiency of the construction industry is negatively correlated with energy poverty (coefficient = −0.545, p < 0.01) and technology level (coefficient = −0.107, p < 0.1), and it is negatively correlated with the energy consumption structure (coefficient = 0.257, p < 0.01) and marketization degree (coefficient = 0.547, p < 0.01), which was in line with the theoretical expectation and preliminarily tested the hypotheses in this paper.
Stata 15 software is used to analyze the impact of energy efficiency of the construction industry, and Wen et al.’s (2004) improved intermediate and regulatory step-by-step inspection method based on Baron and Kenny is used to test the intermediate effect of energy consumption structure and the regulatory effect of marketization degree and technological level.
As an explained variable, the energy efficiency of the construction industry is studied on the impact of energy poverty on the energy efficiency of the construction industry. The OLS model, Tobit model, and Probit model are adopted, respectively. The specific results are shown in Table 5.
The column “OLS, Husnain et al. (2021)” is the result of regression using OLS. There is a negative correlation between energy poverty and the energy efficiency of the construction industry. The energy efficiency of the construction industry decreases by 0.315 units for each unit increase of energy poverty. The R-squared value of 0.424 indicates that the interpretation degree of the model is 42.4%, and the F statistic is 13.961, which is significant at 1%, indicating that the overall significance of the model is good. Therefore, when the industrial development degree, the degree of opening to the outside world, the auxiliary industry development degree of the construction industry, the added value of the construction industry, the number of construction employees, and other variables are controlled, the main effect of energy poverty on energy efficiency is significant. The column “Tobit, Li et al. (2021)” is the result of regression using the Tobit model. Because energy efficiency is a constrained continuous variable and its value follows a normal distribution roughly, this model is adopted. Lr is 183.28, and the corresponding p value is 0.000, indicating that the model as a whole is significant at 1%.
The column “Probit, Gajdzik et al. (2021)” gives the regression results by using the Probit model. Since the energy efficiency of the construction industry is a continuous variable of 0–1, considering that the energy efficiency obeys the basic normal distribution, the energy efficiency that is greater than or equal to 0.82 is classified as 1, and the energy efficiency that is less than 0.82 is classified as 0, and it is converted into a binary selection problem in line with Probit’s thought. The regression results of this model are consistent with those of OLS and Tobit models. The Wald value of 110.958 indicates a good model fitting degree, and the corresponding p value is 0.000, indicating that the overall model is significant at 1%.
In the three regression models, energy poverty is negatively correlated with the energy efficiency of the construction industry. Based on the above theoretical research, energy storage can further stimulate the technical inefficiency in the process of energy input, eventually lead to the decrease of energy utilization efficiency, and have a negative impact on regional economic development. Therefore, it shows that the regional energy poverty level will inhibit the improvement of the energy efficiency of the construction industry, which verifies Hypothesis 1.
Table 6 shows the regression results of the mediating effect of energy consumption structure. The column “OLS, Husnain et al. (2021)” is the impact of energy poverty on the energy consumption structure, and the column “OLS, Nathan and Hari (2020)” is the impact of energy poverty on the energy efficiency of the construction industry after adding the energy consumption structure.
The column “OLS, Husnain et al. (2021)” shows the significant negative impact of energy poverty on the energy consumption structure (coefficient = −0.136, t = −1.72, p < 0.1). In addition, the other two measurement methods, i.e., the columns “Tobit, Li et al. (2021)” and “Probit, Gajdzik et al. (2021),” also show consistent results with those of the column “OLS, Husnain et al. (2021).” In the column “OLS, Nathan and Hari (2020),” after adding the energy consumption structure, the energy poverty has a significant negative impact on the energy efficiency of the construction industry (coefficient = −0.278, t = −5.08, p < 0.01), and the energy consumption structure has a significant positive impact on the energy efficiency of the construction industry (coefficient = 0.274, t = 6.68, p < 0.01). The coefficient of energy poverty on the energy efficiency of the construction industry in the column “OLS, Nathan and Hari (2020)” of Table 6 is −0.278***, which is slightly higher than the regression coefficient of −0.315*** in the column “OLS, Husnain et al. (2021)” of Table 5, which can prove the existence of mediating effect. Hypothesis 2 is valid. In columns “OLS, Husnain et al. (2021)” and “Probit, Gajdzik et al. (2021),” the coefficient β1 of energy poverty (EP) is negative, and in columns “OLS, Nathan and Hari (2020)” and “Probit, Li and Li (2020),” the sign of the energy consumption structure (ECS) coefficient χ2 is positive. It can be seen that the sign of β1*χ2 is negative, which is in the same direction as the χ2 sign of the energy poverty (EP) coefficient in columns “OLS, Nathan and Hari (2020)” and “Probit, Li and Li (2020),” and there is a partial mediating effect. Combined with theoretical analysis, it can be seen that energy poverty has a negative impact on the energy consumption structure, that is to say, the more serious the energy poverty in the province is, the more dependent the energy consumption structure will be on traditional energy instead of adjusting to clean and modern energy. The energy consumption structure has a positive impact on the energy efficiency of the construction industry. The more the energy consumption structure shifts to modern energy, the higher the energy utilization efficiency of the construction industry will be. Therefore, in order to improve energy efficiency, it is necessary to reduce energy poverty.
The regression results of the column “OLS, Husnain et al. (2021)” in Table 7 show that energy poverty has a negative impact on the energy efficiency of the construction industry, and the regression coefficient (coefficient = −0.560, t = −3.29, p < 0.01) after the marketization degree is added. Compared with the regression coefficient (coefficient = −0.315, t = −5.38, p < 0.01) in the column “OLS, Husnain et al. (2021)” in Table 5, the negative impact of energy poverty on the energy efficiency of the construction industry is intensified. The results generated in columns “Tobit, Li et al. (2021)” and “Probit, Gajdzik et al. (2021)” are consistent with those in the column “OLS, Husnain et al. (2021),” which verifies Hypothesis 3. The reason for this is that the higher the degree of marketization, the more intense the market competition, the more difficult the survival of construction enterprises, and the more reluctant it is to choose modern clean energy, resulting in low energy efficiency.
The columns “OLS, Nathan and Hari (2020)” and “Probit, Li and Li (2020)” in Table 7 show that the technological level weakens the negative impact of energy poverty on the energy efficiency of the construction industry, which verifies Hypothesis 4. At this time, the impact of energy poverty on the energy efficiency of the construction industry changed from the negative coefficient in Table 5 (coefficient = −0.315, t = −5.38, p < 0.01) to the positive coefficient in Table 7 (coefficient = 2.545, t = 2.37, p < 0.05). The results show that the regions with high technical level will weaken the negative impact of energy poverty on the energy efficiency of the construction industry. That is to say, although the energy poverty in this region leads to the increase of energy prices and production costs, the construction industry in this region has a high technical level. The construction industry will rely on high technology and mechanized equipment to reduce the energy waste in the production process and thus improve the energy use efficiency.
In order to ensure the stability of the above regression results, the alternative energy poverty index was used to test the robustness of the regression model. The above energy poverty index considers the difference between urban and rural areas and selects indicators from the perspectives of energy input and energy service. The replacement energy poverty index will select indicators from four aspects of energy supply, demand, investment, and cleanliness, calculate the new energy poverty index, and make a regression. The main effect and mediating effect still existed after replacing the main variables, which proved that the result was robust. The detailed results are shown in Table 8.
This paper analyzes the influence mechanism of the energy efficiency of the construction industry, uses DEA to measure the energy efficiency of the construction industry, and uses the regression model to test the mediating effect of energy poverty on the energy efficiency of the construction industry through the energy consumption structure, as well as the moderating effect of marketization degree and technological level on it. The main conclusions are as follows: The influence model of energy efficiency of the construction industry is constructed, and OLS, Tobit, and Probit estimation methods are used to analyze the impact of energy poverty on the energy efficiency of the construction industry and the mediating role of energy consumption structure in it. It is found that energy poverty has a negative impact on the energy efficiency of the construction industry. That is to say, the more serious the energy poverty in the region is, the more it will restrain the adjustment of the energy consumption structure in the region to a more stable and efficient energy direction, leading to the decrease of the energy efficiency of the construction industry (Husnain et al., 2021). The improvement of marketization will strengthen the negative impact of energy poverty on the energy efficiency of the construction industry. In other words, the higher the marketization degree of the construction industry in this region, the more the negative impact of energy poverty on the energy efficiency of the construction industry will be intensified. The technology level will reduce the negative impact of energy poverty on the energy efficiency of the construction industry. That is to say, areas with high technical level of construction industry can reduce part of energy consumption through technological progress, thus improving energy utilization efficiency (Li et al., 2021).
The effective use of energy is related to the production and development of all walks of life and is related to the smooth realization of energy conservation and emission reduction targets in China’s construction industry. Through the analysis of the research results, the following policy recommendations are put forward: In view of the significant differences in energy efficiency and energy poverty in the construction industry of various provinces, differentiated energy policies can be formulated (Husnain et al., 2021). For example, in some resource-rich areas, according to the “resource curse” theory, enterprises have weak awareness of conservation when carrying out production operations due to abundant resources, low cost, and other reasons, resulting in a large amount of energy waste and energy efficiency reduction. The local government could then regulate the project by asking the tenderee to specify the best energy use for the project, and the government would provide a tiered energy price plan for the project. In the enterprise bidding, this requirement is written into the tender and the construction unit to carry out accordingly. At the time of completion and acceptance, the government charges for exceeding the standard, so as to urge enterprises to improve energy efficiency and reduce waste. In resource-deficient areas, the policy focus should be on changing the way energy is used, improving the economic scale of the construction industry, and strengthening regional energy cooperation. The local government promotes the combination of technological progress and the adjustment of the energy consumption structure to promote the adjustment of the energy consumption structure to cleaner and modern energy, so that energy consumption is not only dependent on a certain kind of energy but also diversified and balanced, so as to improve energy efficiency (Li et al., 2021). Local governments should reasonably increase the investment in green technology innovation in the construction industry, provide human support, guide the strong construction enterprises to research and develop green technology, use energy-saving materials, and improve the energy utilization level of construction enterprises (Gajdzik et al., 2021). Improved technology can help reduce energy poverty and increase energy consumption, thereby increasing the energy efficiency of the construction industry. The improvement of technical level and technical equipment rate is conducive to the improvement of the energy efficiency of various industries. High-technology areas have relatively high energy efficiency, so technology diffusion and sharing should be carried out to drive less-developed areas. By optimizing resource allocation and actively carrying out market-oriented reform, local governments can promote free and orderly competition among construction enterprises, and benign competition enables construction enterprises to reduce energy consumption costs by improving the energy efficiency of the construction industry, so as to gain more favorable cost advantages (Nathan and Hari, 2020).
The raw data supporting the conclusions of this article will be made available by the authors, without undue reservation.
JZ was responsible for the theme of energy poverty and energy efficiency of the construction industry. YL was responsible for data collection, cleaning, and analysis and drafting of the thesis. XL was responsible for the study design and final revision of the paper. WZ was responsible for reviewing the revised draft at all stages of the paper and putting forward suggestions, and was responsible for the paper.
This research was supported by the National Natural Science Foundation of China project “Research on the influence mechanism of local official turnover on green technology innovation of High energy Consuming Enterprises” (Grant No. 72002029); Heilongjiang Postdoctoral Research Foundation project “The Impact of international Oil price Fluctuations on Heilongjiang Industrial Industry” (Grant No. 16190026); the open fund project of the liberal arts base of Northeast Petroleum University “Research on the Influence Mechanism of Official Turnover on Green Technology Innovation of Petrochemical Enterprises” (Grant No. WKJD202001); And the Heilongjiang Provincial Philosophy and Social Science Research Planning Project “Research on Carbon Decoupling Effect of Carbon Emission Trading on Energy-intensive Enterprises in Heilongjiang Province” (Grant No. 20JYE280).
The authors declare that the research was conducted in the absence of any commercial or financial relationships that could be construed as a potential conflict of interest.
All claims expressed in this article are solely those of the authors and do not necessarily represent those of their affiliated organizations, or those of the publisher, the editors, and the reviewers. Any product that may be evaluated in this article, or claim that may be made by its manufacturer, is not guaranteed or endorsed by the publisher.
Chang, M. (2020). An Application of Total-Factor Energy Efficiency under the Metafrontier Framework. ENERG POLICY 142, 111498. doi:10.1016/j.enpol.2020.111498
Chen, J. Q., Liu, N., and Ma, X. J. (2021). Energy Eco-Efficiency Measurement and its Driving Factors of Eight Comprehensive Economic Zones in China Based on Stochastic Frontier Model. Chin. Environ. Sci. 41, 1–11. doi:10.19674/j.cnki.issn1000-6923.20210223.018
Chu, W., and Xinye, Z. (2020). A New Perspective on Raising Energy Efficiency: A Test Based on Market Segmentation. Social Sci. China 41, 59–78. doi:10.1080/02529203.2020.1719736
Feng, B., and Wang, X. Q. (2015). Research on Energy Economic Efficiency and Energy Environmental Efficiency of Chinese Construction Industry -- Two-Stage Analysis Based on SBM Model and Panel Tobit Model. J. Beijing Inst. Tech. (Social Sci. Edition) 17, 14–22. doi:10.15918/j.jbitss1009-3370.2015.0103
Gajdzik, B., Sroka, W., and Vveinhardt, J. (2021). Energy Intensity of Steel Manufactured Utilising EAF Technology as a Function of Investments Made: The Case of the Steel Industry in Poland. ENERGIES 14, 5152. doi:10.3390/EN14165152
Gao, Y., Yang, G. S., and Xie, Q. H. (2020). The Impact Mechanism of Heterogeneous Environmental Regulation on green Total Factor Productivity: Based on the Moderating Effect of Energy Consumption Structure. Resour. Industries 22, 1–10. doi:10.13776/j.cnki.resourcesindustries.20200529.001
Haider, S., and Bhat, J. A. (2020). Does Total Factor Productivity Affect the Energy Efficiency. Int. J. Energ. Sector Manag. 14, 108–125. doi:10.1108/IJESM-11-2018-0010
Hou, J. C., Chen, Q. M., and Sun, F. H. (2020). Total Factor Energy Efficiency and its Influencing Factors in China's Transportation Industry. Stat. Decis. making 36, 103–108. doi:10.13546/j.cnki.tjyjc.2020.03.022
Hu, M. M., Li, Z. Y., Xie, Y. X., and Xiang, P. C. (2019). Research on Energy Efficiency of Provincial Construction Industry in China Based on Super-SBM Model: A Case Study from 2006 to 2015. Building economy 40, 100–105. doi:10.14181/j.cnki.1002-851x.201907100
Husnain, M. I. U., Nasrullah, N., Khan, M. A., and Banerjee, S. (2021). Scrutiny of Income Related Drivers of Energy Poverty: A Global Perspective. ENERG POLICY, 157. doi:10.1016/J.ENPOL.2021.112517
Jin-Li, H., and Shih-Chuan, W. (2005). Total-factor Energy Efficiency of Regions in China. ENERG POLICY 34. doi:10.1016/j.enpol.2005.06.015
Kahouli, S., and Okushima, S. (2021). Regional Energy Poverty Reevaluated: A Direct Measurement Approach Applied to France and Japan. ENERG ECON., 102. doi:10.1016/J.ENECO.2021.105491
Li, H., Chu, H., Ma, X., Wang, G., Liu, F., Guo, M., et al. (2021). Efficient Heterogeneous Acid Synthesis and Stability Enhancement of UiO-66 Impregnated with Ammonium Sulfate for Biodiesel Production. CHEM. ENG. J., 408. doi:10.1016/J.CEJ.2020.127277
Li, J. K., Ma, J-J., and Wei, W. (2020). Regional Differences of Energy Carbon Emission Efficiency in Eight Comprehensive Economic Zones in China. Quantitative Tech. Econ. Res. 37, 109–129. doi:10.13653/j.cnki.jqte.2020.06.006
Li, K., Wang, K., and Wang, Y. X. (2014). Comprehensive Evaluation of Regional Energy Poverty in China. J. Beijing Inst. Tech. (Social Sci. Edition) 16, 1–12. doi:10.15918/j.jbitss1009-3370.2014.02.015
Li, L. B. (2015).Dynamic Evolution, Regional gap and Cause Identification of Energy Performance in China: Based on a New Total Factor Energy Productivity Change Indicator. Management of the world (11), 40–52. doi:10.19744/j.cnki.11-1235/f.2015.11.008
Li, S. X., and Li, L. J. (2020). Regional Differences and Influencing Factors of Rural Energy Poverty in China. J. Agric. For. Econ. Manag. 19, 210–217. doi:10.16195/j.cnki.cn36-1328/f.2020.02.22
Li, Y. T., and Shi, D. (2018). The Formation Mechanism and Empirical Study of China's Industrial Energy Efficiency gap. J. Shanxi Univ. Finance Econ. 40, 61–73. doi:10.13781/j.cnki.1007-9556.2018.06.005
Li, Y., and Li, Z. L. (2020). Grey Correlation Analysis of Energy Consumption and Economic Growth in Shanxi Province -- Based on the Perspective of Energy Structure Constraint. Pract. understanding Math. 50, 60–66.
Liao, M., and Ren, Y. (2020). The ‘double-Edged Effect’ of Progress in Energy-Biased Technology on Energy Efficiency: A Comparison between the Manufacturing Sector of China and Japan. J. ENVIRON. MANAGE 270, 110794. doi:10.1016/j.jenvman.2020.110794
Mohsin, M., Hanif, I., Taghizadeh-Hesary, F., Abbas, Q., and Iqbal, W. (2021). Nexus between Energy Efficiency and Electricity Reforms: A DEA-Based Way Forward for Clean Power Development. ENERG POLICY 149, 149. doi:10.1016/J.ENPOL.2020.112052
Nathan, H. S. K., and Hari, L. (2020). Towards a New Approach in Measuring Energy Poverty: Household Level Analysis of Urban India. ENERG POLICY 140, 111397. doi:10.1016/j.enpol.2020.111397
Peng, D. Y., and Zhang, J. (2019). Impact of Environmental Regulation on Total Factor Energy Efficiency in China: an Empirical Test Based on Inter-provincial Panel Data. Ind. Technol. economy 38, 59–67.
Qian, M., and Jin, X. L. (2021). The Impact of Environmental Regulation and Energy Endowment on Energy Efficiency from the Perspective of "resource Curse" : Based on 17 Provinces along the belt and Road. Res. coal economy 41, 4–9. doi:10.13202/j.cnki.cer.2021.03.002
Reuter, M., Patel, M. K., Eichhammer, W., Lapillonne, B., and Pollier, K. (2020). A Comprehensive Indicator Set for Measuring Multiple Benefits of Energy Efficiency. ENERG POLICY 139, 111284. doi:10.1016/j.enpol.2020.111284
Sofia-Natalia, B., and Agis, M. P. (2019).Energy Poverty and Energy Efficiency Improvements: A Longitudinal Approach of the Hellenic Households. Energy & Buildings, 197. doi:10.1016/j.enbuild.2019.05.027
Wang, X. B., and Huang, L. X. (2021). State-owned Economy, Marketization Degree and Steady Growth of Chinese Economy. Ind. Econ. Rev. (4), 52–72. doi:10.19313/j.cnki.cn10-1223/f.2021.04.003
Wang, Y. S., Zeng, F. S., and Zhang, Y. B. (2021). Research on Energy Efficiency of Chinese Construction Industry Based on Carbon Emission Measurement. J. Eng. Manag. 35, 1–6. doi:10.13991/j.cnki.jem.2021.04.003
Weina, Z., Zhihui, Z., Xiaodong, L., Wei, F., and Jifeng, L. (2019). Assessing the Effects of Technological Progress on Energy Efficiency in the Construction Industry: A Case of China. J. CLEAN. PROD. 238, 238. doi:10.1016/j.jclepro.2019.117908
Wen, Z. L., Zhang, L., Hou, J. T., and Liu, H. Y. (2004). Mediation Effect Test Program and its Application. J. Psychol. 36, 614–620.
Wilson, B., Trieu, L. H., and Bowen, B. (1994). Energy Efficiency Trends in Australia. ENERG POLICY 22, 287–295. doi:10.1016/0301-4215(94)90003-5
Wu, J., Ge, Z., Han, S., Xing, L., Zhu, M., Zhang, J., et al. (2020). Impacts of Agricultural Industrial Agglomeration on China’s Agricultural Energy Efficiency: A Spatial Econometrics Analysis. J. CLEAN. PROD. 260, 121011. doi:10.1016/j.jclepro.2020.121011
Xu, G., Schwarz, P., and Yang, H. (2020). Adjusting Energy Consumption Structure to Achieve China's CO2 Emissions Peak. Renew. Sust. Energ. Rev. 122, 109737. doi:10.1016/j.rser.2020.109737
Yang, H. C., Li, L. S., and Zhang, F. M. (2021). The Impact of Manufacturing Agglomeration on Energy Performance and Regional Comparison. Scientific Res. Manag. 42, 102–115. doi:10.19571/j.cnki.1000-2995.2021.05.012
Yu, J., and Qu, G. Q. (2021). Regional Ecological Energy Efficiency and Potential for Energy Conservation and Emission Reduction in China. Stat. Decis. making 37, 66–69. doi:10.13546/j.cnki.tjyjc.2021.12.014
Zhang, X. F., Song, G., and Yan, Y. (2020). The Impact of Urban Low-Carbon Transportation System on Energy Consumption Structure: Panel Data from 14 Cities in China. Chin. Manag. Sci. 28, 173–183. doi:10.16381/j.cnki.issn1003-207x.2020.12.017
Keywords: energy poverty, energy consumption structure, technical level, marketization degree, energy efficiency
Citation: Zhang J, Liu Y, Zhang W and Lin X (2021) Study on the Impact of Energy Poverty on Energy Efficiency of Construction Industry: Mediating Role of Energy Consumption Structure. Front. Energy Res. 9:760895. doi: 10.3389/fenrg.2021.760895
Received: 19 August 2021; Accepted: 22 September 2021;
Published: 29 October 2021.
Edited by:
Hui Li, Shandong Jianzhu University, ChinaCopyright © 2021 Zhang, Liu, Zhang and Lin. This is an open-access article distributed under the terms of the Creative Commons Attribution License (CC BY). The use, distribution or reproduction in other forums is permitted, provided the original author(s) and the copyright owner(s) are credited and that the original publication in this journal is cited, in accordance with accepted academic practice. No use, distribution or reproduction is permitted which does not comply with these terms.
*Correspondence: Xiangyi Lin, ZHFsaW54aWFuZ3lpQDE2My5jb20=
Disclaimer: All claims expressed in this article are solely those of the authors and do not necessarily represent those of their affiliated organizations, or those of the publisher, the editors and the reviewers. Any product that may be evaluated in this article or claim that may be made by its manufacturer is not guaranteed or endorsed by the publisher.
Research integrity at Frontiers
Learn more about the work of our research integrity team to safeguard the quality of each article we publish.