- 1College of Urban Construction, Nanjing Tech University, Nanjing, China
- 2Key Laboratory of Aircraft Environment Control and Life Support, MIIT, Nanjing University of Aeronautics and Astronautics, Nanjing, China
- 3Qingdao Hisense Hitachi Air-conditioning Systems Co., Ltd., Qingdao, China
- 4The Second Construction Co., Ltd., China Construction Third Engineering Bureau, Wuhan, China
Building demand-side management is an effective solution for relieving the peak and imbalance problems of electrical grids. How to explore the energy flexibility of buildings and to coordinate a variety of buildings with different energy flexibilities for effective interactions with smart grids are a great challenge. This paper proposes a game theory–based hierarchical demand optimization method for energy flexible buildings for achieving better grid interactions. This method consists of two optimization strategies at the grid and building levels. At the grid level, a demand-price interaction model for buildings and the grid is established to identify the Nash equilibrium solutions based on game theory; these solutions are used to determine the optimized energy demand of buildings and the associated electricity prices by accommodating the interests of all participants involved. At the building level, three types of buildings with different energy flexibilities are investigated to analyze the influence of building management strategies on grid interactions. The effectiveness of the proposed method is verified in a simulated case study. The results show that the optimization method can reduce building operational cost by 3–18%, reduce the fluctuation of the power grid by 30–50%, and ensure that the power grid increases income by 8–20%.
Introduction
Backgrounds
With the continuous increase in power consumption and the high penetration of renewable energy generations, peak load and power imbalance have become two major challenges for an electrical grid; these challenges significantly affect the reliability, quality and energy efficiency of the grid (Arteconi et al., 2019). The peak load usually results from end-user energy behaviors (e.g., the power demands of buildings or industrial production during office hours/peak hours). Power imbalances generally result from a sudden loss of power supply or an increase in power demand. Redundant capacities of power plants and adequate operating reserves are essentially required by power generation sectors. Energy storage devices/systems (e.g., electric vehicles, batteries, and pumped-storage hydroelectricity) can also be employed as operating reserves. However, these methods are usually limited by either geographic conditions or high initial/operation costs and low storage capacities (Yan et al., 2020). In contrast, methods that use incentive benefits (i.e., implement the power demand response programs) to encourage end-users to manage their power usage behaviors are considered a more promising solution for peak load and power imbalance of grids.
Buildings are the largest electricity consumers worldwide and have great potential for power demand management in practice. In the United States, China, and Europe, the proportion of electricity used in construction in total electricity consumption reached 39.1, 20.6, and 29.4%, respectively (Electrical and Mechanical Services Department of Hong Kong, 2012; DoE, 2014). With the wide applications of building automation systems, IT technologies, smart meters and energy storage, buildings have demonstrated their great capabilities and willingness to participate in the demand management of grids through the bidirectional interaction between buildings and smart grids (Reynders et al., 2018). “Energy flexible buildings” (EFBs), which have the capacity to manage their energy demand and production according to local climate conditions, user needs, and grid requirements, have been proposed by the International Energy Agency (IEA) (Jensen et al., 2017). More specifically, Wang proposed the concept of ‘grid-friendly and grid-responsive’ buildings in a research report, where energy flexible buildings are required to work in synergy with (i.e., be responsive to) the grid or avoid additional stresses (i.e., be friendly) on the power grid balance (Wang, 2016). The energy flexibility of buildings can be greatly enhanced with the use of passive/active thermal storage. For instance, building thermal mass is an inherent passive storage of building structures and internal furniture for building load shifting and demand limiting by using precooling and room temperature set-point reset (Liang et al., 2020). Wang summarized three typical categories of active storage used in buildings to enhance energy flexibility: conventional storage (e.g., chilled water storage), small-scale storage (e.g., phase-change material), and innovative use of existing building facilities (e.g., fire water tanks) for load shifting, demand limitation and management. The increasing usage of power generation in buildings is a trend for sustainable building design and significantly affects the demand and flexibility characteristics of buildings (Zhong et al., 2016). Above all, buildings have the potential to be an excellent carrier for demand-side energy consumption management to solve grid imbalance.
Literature Review
How to explore the energy flexibility of buildings and to coordinate a variety of buildings with different energy flexibilities for effective interactions with smart grids are challenging. The key issue is how to accommodate the interests of all participants involved in the interactions between grids and buildings (Xu et al., 2020). In fact, driven by attractive electricity prices and various incentive policies, buildings may change their power demand flexibly and responsively. The altered aggregate power demand of buildings will affect electricity prices once a dynamic pricing mechanism is adopted in a smart grid. In contrast, the adjusted electricity prices will also induce buildings to change their energy behaviors to lower their operation cost. Further adjustments to electricity prices and building demands will continue until optimal operation is approached (Saad et al., 2012; Huang et al., 2019). A dynamic pricing method based on genetic algorithm is proposed by Huang et al.to improve two-way interaction and reduce power imbalance (Huang and Sun, 2019). They also proposed the collaborative demand response of nearly zero energy buildings to respond to the dynamic pricing of cluster-level performance improvement, which provides decision makers with computationally efficient demand response control for almost zero-energy buildings, thereby realizing full collaboration and helping To improve performance (Basar and Olsder, 1999).
At the same time, as an iterative and interactive power demand management strategy, mutual matching of supply-demand between EFBs and smart grid can be analyzed by game theory. Game theory is a mathematical theory and method for studying interactive phenomena whose nature is characterized by struggle or competition (Huang et al., 2017). Previous studies have investigated the feasibility of using game theory to solve the supply-demand interaction problem. Huang et al. reviewed the related research on cluster energy consumption planning when EFB buildings are connected to the grid. The authors pointed out the importance of consumer participation in the interaction of supply and demand and proposed an information interaction platform structure based on game theory, thereby aiming to improve the reliability of energy planning strategies for building clusters (Chen et al., 2019). Chen et al. used game theory as a tool to define the strategies of participants in the Israeli construction market; the authors combined these strategies with various incentives in the interaction of supply and demand and analyzed the strategy combination that optimally improved the market to help the expansion of the Israeli EFB market (Najafi-Ghalelou et al., 2018). Afshin et al. conducted game modeling on the internal energy interaction problem of a building complex, ensured the global optimal solution through mixed integer programming, and determined the individual strategies in equilibrium through general algebraic modeling system optimization software. The results of this case study showed that the internal energy interaction strategy of the building complex can improve the power exchange capacity and reduce the power cost (Lv and Ai, 2016). Lv et al. proposed a new dynamic energy management strategy that interacts with an active power distribution system based on multigrid-connected microgrids; the strategy was aimed at a higher-level benefit distribution network and multiple smart grid units participating in the interaction. A high-level interaction between smart grid units and benefit distribution networks is described by two-level programming, and the smart grid units are modeled by using an innovative interactive energy game matrix (Li and Wang, 2020). In a study by Li et al., an online multi-objective coordinated control strategy composed of two control optimization schemes was proposed for predictive scheduling and real-time optimal control of the energy system of zero-energy buildings. The strategy is based on a cooperative game model and is tested and evaluated by simulating energy storage system scheduling in a typical period in a zero-energy building. The control variables and target weights are finally optimized, and energy costs and grid fluctuations are minimized (Zhao, 2001). The abovementioned studies regard the smart grid and buildings as two participants simultaneously engaged in decision-making and thereby provide a guiding idea for analyzing supply-demand interaction from the perspective of game theory. The traditional game model regards the building and the power grid as equal participants in the buying and selling relationship; however, this view differs from reality, and consequently, the final Nash equilibrium is inaccurate.
The economic competition relationship between the power grid and buildings is usually defined as a hierarchical planning problem in which one party is dominant and the other party is subordinate. Specifically, the power grid precedes buildings in making decisions on electricity prices, and the buildings then respond to the electricity price plans formulated by the grid and determine the power purchase demand to maximize the buildings’ own benefits. Additionally, because the power grid needs to consider the response of buildings to electricity prices when making decisions (excessive prices will cause buildings to be more self-produced and self-sold), the energy demand of buildings can also counteract the power grid’s pricing plan. This game model of interaction between different levels of decision-making is called the Stackelberg model (Yang et al., 2013). In the production and operation of some commodities with poor price elasticity, the company that decides the strategy first in the Stackelberg model does not have the first-mover advantage, but the company that determines the output later has the latter-mover advantage. Therefore, traditional electricity sales models adopt monopoly or national production and operation methods. However, when renewable energy and energy storage systems are connected, the price demand elasticity of electricity usage in buildings increases. Under the Stackelberg model, the grid side can ensure its own first-mover advantage while incentivizing the buildings to reduce their consumption, and the revenue of electricity is also higher than that under the traditional Cournot model, so it is beneficial to use the Stackelberg model to solve the game equilibrium in the game analysis.
In a study by Peng et al., the Stackelberg model was used to optimize the time-of-use (TOU) pricing strategy of the grid. The model satisfied equilibrium user demand and reduced the potential cost of the utility by encouraging buildings to purchase electricity through low trough and low pricing and by encouraging the self-production of buildings through high peak pricing (Yang et al., 2014). On this basis, Jie et al. optimized the utility problem of building user comfort based on the new effect function for determining the optimal power consumption and grid pricing under real-time electricity prices (RTPs) (Srinivasan et al., 2017). Srinivasan et al. selected dynamic pricing strategies based on game theory for the Singapore electricity market and compared the economic benefits of three pricing strategies: half-hour pricing, time-of-use electricity pricing, and day-and-night electricity pricing (DN). The results show that RTP can reduce the peak load of the residential and commercial sectors by 10 and 5%, respectively; and increase profits by 15.5 and 18.7%, respectively (Tang et al., 2019). Tang et al. used the Stackelberg model to maximize the profits of buildings and power grids by considering the impact of uncertainties (Zhang et al., 2019).
However, the game model used in the literature cited above does not classify the types of buildings that the game applies to and ignores the impact of different building system conditions on the interaction of supply and demand.
Research Gaps and Main Contributions
In previous studies, when considering grid interactions or games between EFBs and smart grids, buildings were often considered to have the same energy “flexibility”. In fact, buildings with different energy systems and different control strategies have different energy flexibilities and energy consumption patterns, which affect the results of the supply-demand interaction of the game with the power grid. To obtain better demand response optimization results with more realistic performances, this paper constructs three typical flexible buildings with different energy systems and energy control strategies and proposes a hierarchical demand optimization method based on game theory to coordinate these buildings with different energy flexibilities for effective grid interaction with smart grids. The remainder of this paper is arranged as follows. In Principle of the Hierarchical Optimization Method, the principle of hierarchical optimization methods will be explained in detail, and the specific interactive game model and internal strategy selection algorithm will be established in Hierarchical Optimization Implementation Based on Game Theory. Finally, in Case Study, the rationality of the two-tier optimization scheme will be analyzed by using case analysis.
Principle of the Hierarchical Optimization Method
Structure of the Optimization Methods
As shown in Figure 1, the proposed hierarchical game theory-based demand optimization method consists of two optimization strategies at the grid and building levels. At the grid level, a demand-price interaction model between buildings and the grid is established to use game theory to identify the Nash equilibrium solutions, which are used to determine the optimized energy demand of buildings and the associated electricity prices by accommodating the interests of all participants involved. At this level, buildings can adjust their energy consumption patterns in response to the real-time electricity price or other incentive policies to pursue the lowest possible electricity cost. However, the power grid will also provide the corresponding RTP according to the energy consumption of the building at different times to ensure maximum profit for the building. This kind of supply-demand interaction level analysis of building energy strategy is a typical non-cooperative game problem. Building energy demand and grid quotations are bargained at the game level; when the demand and the quotations reach the Nash equilibrium, the mutual benefits of both parties can be maximized.
At the building level, three types of EFBs with different energy flexibilities are investigated to achieve their maximum interests by using appropriate control strategies during the interaction with the grid; the details of the building system conditions are shown in Table 1. Type-1 buildings are “passive storage buildings”, which can adjust their energy usage by changing the indoor air temperature of the air-conditioning system with a certain degree of thermal comfort sacrifice. Considering that the acceptable temperature change range is small (e.g., 2°C), the energy flexibility is limited. Type-2 buildings are “active storage buildings”, which can purchase excess electricity during periods of low electricity prices and use active energy storage systems to transfer the excess electricity to use during periods of high electricity prices. The energy flexibility is generally moderate, and buildings have a certain degree of initiative in the game, which requires the grid to set peak electricity prices with more consideration of the building’s acceptance. Type-3 buildings, which both generate and store energy, have relatively considerable energy flexibility by controlling both their own energy generation and consumption patterns. Such buildings can purchase low-priced or sell high-priced electricity as much as possible and therefore have a strong initiative in the game with the power grid. In summary, the building energy flexibility or the ability of a building to adjust energy consumption in response to electricity prices depends on the system conditions of the building. How to encourage buildings with different energy flexibilities to take part in the grid interaction game and maximize their own interests by using appropriate control strategies is the other key of the proposed optimization method.
Game-Based Interactive Optimization at Grid-Level
The optimization objective of the grid level is to achieve the Nash equilibrium of the EFB-grid game interaction. The three elements that make up game
A strategy vector
In the above definition,
To model the game objective on the building side, the mathematical description of the game objective is as follows:
where
The mathematical description corresponding to the grid strategy is:
where
The premise of the existence of the Nash equilibrium is that the players of the game abide by the basic rules of the transaction, and any conflicting strategy that only considers unilateral interests will lead to the failure of the transaction. Therefore, it is necessary to define the value range of the game strategy set:
In Equation 3,
Internal Optimization at Building-Level
To determine the energy demand scheme that can minimize the overall cost of a building, the cost optimization objective function must be constructed first. The building cost is composed of three main parts: user dissatisfaction, mismatch cost (these two will not be directly reflected in the bill), and the electricity purchase cost of the transaction with the grid.
User Dissatisfaction
User dissatisfaction is an indicator that describes the degree of dissatisfaction of users in the building with the current thermal comfort conditions when the demand is limited to deviate from the normal level. It should be noted that even under the original energy demand, due to differences in the definition of thermal comfort by users, there will still be a small number of users who are dissatisfied with the current thermal environment, so there is a unique positive minimum for user dissatisfaction, and It has the property of monotonically increasing with the degree of deviation from the minimum point, so the quadratic function is used for description in this study. Assuming that there are
where
Mismatch Cost
Mismatch costs refer to the hidden costs, such as energy storage loss and machine loss, incurred when the building demand is shifted or changed. It is determined by the difference between the optimized and original demand at the same time, the minimum value is 0 when the two are equal. It has the same monotonicity and concavity as the user dissatisfaction, so it can also be described by a quadratic function. The building mismatch cost at time
where
Power Purchase Cost
The electricity purchase cost, which is the most intuitive economic cost, refers to the electricity bill paid by the building. The power purchase cost of building
where
Hierarchical Optimization Implementation Based on Game Theory
Grid-Level: The Solution of Nash Equilibrium in the Stackelberg Model
During the interaction between the EFB and the grid, biding of the grid will affect the motivation of the EFB to purchase electricity, while the degree of the EFB’s dependence on electricity will also affect the grid’s biding strategy. The grid’s actions always precede those of EFBs; this accords with the application conditions of the Stackelberg model. The principle of backward induction is used to determine the optimal energy use plan of the buildings under the Nash equilibrium of the game and the optimal price of the power grid.
Demand Strategy of Energy Flexible Buildings
Determining the energy demand plan that minimizes the overall cost of the building is a cost function conditional extreme value problem with
In Eq. 7,
By expanding the Lagrange function and finding the partial derivatives of the independent variables
In fact, different types of EFBs have different constraints. For passive storage buildings, the energy consumption change caused by temperature resetting is the optimized amount of building energy consumption. According to the law of conservation of energy, the electricity demand before and after optimization meet the following constraints:
In Eq. 9,
For active storage buildings, the excess load during the low electricity consumption period is stored in the energy storage system and transferred to the peak period to reduce the peak electricity consumption. This type of building does not have an independent capacity device, and the total electricity demand after participating in the dynamic electricity price game should be consistent with the benchmark demand, that is, meet the constraint conditions:
For generation and storage buildings, while the load transfer is completed through the energy storage system, photovoltaic and wind power generation systems can be used to assist the power supply to help the building improve its power purchase demand. Due to the building’s own production capacity, the actual demand after the building side participates in the dynamic electricity price game is less than the benchmark demand, and both types of demand satisfy the following numerical relationship:
In Eq. 11,
Due to the backward compatibility of building equipment, it is generally believed that buildings with stronger energy flexibility can also use the energy control strategy of buildings with weaker energy flexibility. If a building uses all three types of energy control strategies at the same time, its demand will meet the constraint conditions:
The optimized power demand of passive storage buildings:
The optimized power demand of active storage buildings:
The optimized power demand of generation & storage buildings:
Therefore, the energy demand plan that minimizes the overall cost of building
The preset parameters
In Equations 12, 14, there are still parameters
Biding Strategy of Grid
The income from grid sales after deducting comprehensive costs is defined as the grid’s revenue. The explicit cost is the direct cost consumed by the power grid, and the hidden cost is the various energy losses caused by the change in the amount of power generated by the power grid in response to user demand fluctuations. The grid’s revenue is defined as the following equation:
where
In Equation 18, a, b, and c are the preset constants, and
Equations 12–14 show that
Substituting
Let
Building-Level: Energy Control Strategy Under Nash Equilibrium
Indoor Temperature Resetting
Changes in air-conditioning temperature can significantly affect building energy consumption. Previous studies have shown that an increase in indoor temperature by 1°C in the southeastern coastal area can bring approximately 3–6% energy savings (Lai et al., 2013). However, increasing the air-conditioning temperature may also lead to an increase in the hidden cost of the building; this cost is reflected mainly in the cost of user dissatisfaction caused by the reduction of thermal comfort in the building and the mismatched cost caused by fluctuations in energy consumption. To balance the benefits produced by the reduction of energy consumption and the losses caused by the increase in hidden costs, the building cost function is traversed in a 0–2°C increase in air-conditioning temperature. The air-conditioning temperature resetting value corresponding to the lowest total cost of the building is taken as the optimal internal energy control strategy of the building under these conditions. The running logic is shown in Figure 3.
1. Input the objective function and parameters.
2. Calculate the total building cost corresponding to the first set of parameters in the traversal domain (e.g., an indoor temperature of 26–28 °C) when the building demand and grid pricing are iterated to the Nash equilibrium, define the cost as
3. Calculate the cost of all parameters in the traversal domain in turn, and compare the size with
4. When all parameters in the traversal domain are involved in the calculation, the final
Energy Generation and Active Storage
The internal control strategy used by generation & storage buildings is to determine the appropriate share of energy produced by the on-site energy generation systems for storage. During periods of low electricity prices, the energy produced by renewable energy in some buildings can be transferred to peak electricity consumption through energy storage systems. The energy produced during the peak period should be used to minimize the purchasing cost. Through the traversal calculation within a 0–100% energy usage rate per hour, the output capacity usage plan corresponding to the lowest construction cost is output as the optimal internal energy control strategy. When the traversal operation is used to determine the optimal share, because the energy storage at the previous moment can theoretically be used at any subsequent time, the traversal domain at every hour will be affected by the previous time except the first hour. The operating logic is shown in Figure 4.
1. Input the objective function and parameters.
2. Read the first parameter in the traversal domain (a generation usage share of 0–100%) at the first hour, and calculate the possible traversal domain at the second hour under this value; then, read the first parameter in the traversal domain at the second moment; then, calculate out the possible traversal domain at the third hour; and so on. Then, record the 24 parameters as a list.
3. Calculate the total construction cost of the parameters in this list when the construction demand and grid pricing are iterated to the Nash equilibrium; the total construction cost is defined as
4. Read all the parameters in the traversal domain at the first moment, repeat step 2, generate the corresponding value list, and calculate the total cost of the building.
5. Compare the calculation result with
6. When all the parameters in the traversal domain are involved in the calculation, the final
Case Study
Building and System Description
In this paper, some typical buildings of Hong Kong Polytechnic University are selected to provide and generate necessary building energy consumption and energy flexibility data. Buildings adopt central air-conditioning systems and providing energy storage capacity for the building complex by installed phase change material (PCM) storage tank in series with chiller, charging during the low power period is used for the joint power supply during the peak period. In the optimization calculation of passive storage buildings, the PCM air-conditioning system is replaced with an ordinary central air-conditioning system with similar system parameters. At this time, the campus buildings will rely on the energy passive storage of building’s thermal mass during the peak period of electricity consumption to regulate demand. In the period of low electricity consumption, considering the marginal cost of sacrificing user dissatisfaction, the demand will be maintained at the baseline level. The renewable power generation system is connected to the building complex to construct a simulated building complex equivalent to generation and storage buildings, and the system parameters refer to the Hong Kong Zero Carbon Building (ZCB) (Jian and Wang, 2010). A distributed energy system that uses building integrated photovoltaics (BIPV), CIGS thin film solar cells and small wind power generation equipment to provide production capacity for buildings, and cooperates with PCM air-conditioning to complete energy output and transfer. The campus buildings can be divided into four areas according to different functions and locations; these areas are equivalent to four large buildings. The division of each area is shown in Table 2. The data sources, such as building benchmark energy consumption requirements, local meteorological parameters, and time-of-use electricity prices, are based on field observations created on July 3, 2017.
Demand Optimization Results and Strategy Analysis
Passive Storage Buildings
Figure 5 shows the energy interaction strategy of each building when the building’s renewable energy system and energy storage system are not connected. In the interactive optimization of the game built on the power grid, during the low electricity consumption period, the energy consumption demand is consistent with the benchmark energy demand, and the user passively accepts the electricity price set by the grid; during the peak period, the user will consider the economic stimulus of the high price. The preset temperature of indoor air conditioners can be adjusted to reduce electricity demand. The peak power consumption reduced in this way can reduce the cost of direct power consumption while also reducing the peak-to-valley power consumption difference of the building and alleviating grid imbalance.
The gray, red and blue curves in Figure 5 represent the original building load (e.g., indoor temperature of 26°C), the building load when the indoor temperature is fixed at 28°C, and the optimized demand when the indoor temperature of different areas changes in different periods, respectively. Due to the impact of unsatisfactory and mismatched costs, the excessive increase in building air-conditioning temperature increases the hidden cost of the building. To balance the reduction in power purchase costs and the increase in hidden costs, the internal optimization method described in Indoor Temperature Resetting is used as the building internal strategy to determine the optimal indoor temperature, as shown in Figure 6, thus resulting in the optimized hourly energy demand for each area, as shown by the blue curve in Figure 5. The load affected by the temperature fluctuation of the air conditioner is calculated using Hongye load calculation software (Lüth et al., 2018). For the convenience of calculation, the relationship between load and temperature is assumed to be linear.
Active Storage Buildings
Figure 7 shows the energy requirements of buildings after the energy storage system is connected. During low electricity consumption periods, electricity charges are relatively low, and users purchase excessive electricity, thereby transferring low-cost electricity to the peak electricity consumption through the energy storage system, reducing high-cost electricity purchases and thereby meeting economic needs. As a result, the peak-to-valley difference in building power consumption is significantly reduced, and grid imbalance is alleviated.
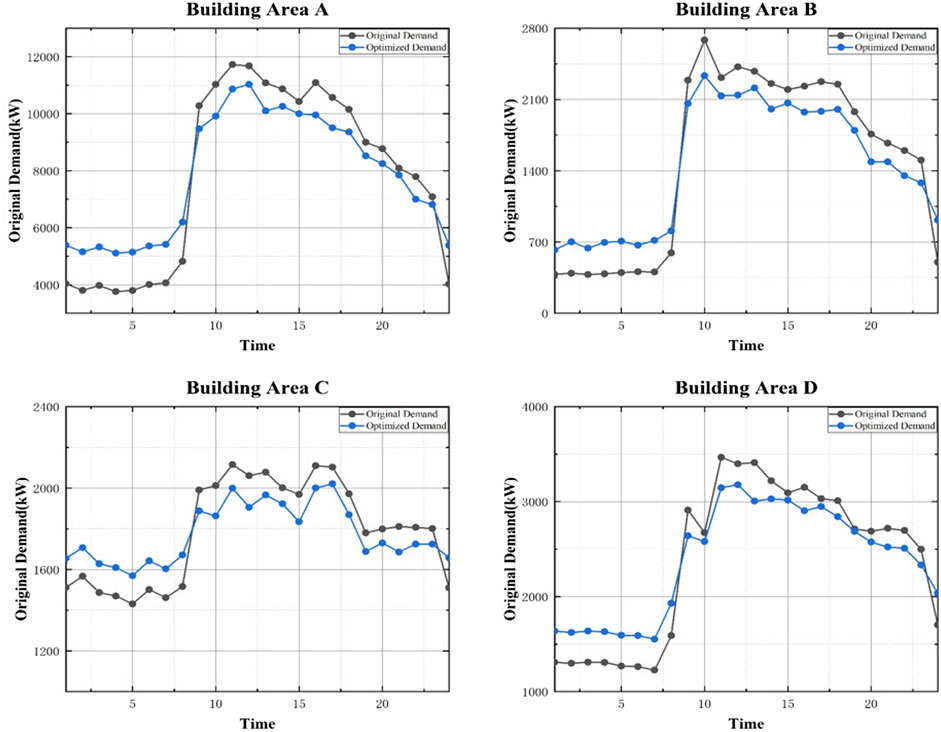
FIGURE 7. Optimized demand profiles of four campus accounts on the test day of active storage buildings.
As described in Demand Strategy of Energy Flexible Buildings, because the energy storage system will not affect the total energy consumption of the building throughout the test day, the interaction strategy under this system condition can be determined before the internal strategy. Furthermore, there is no need to separately filter the internal strategy, and the energy storage at each time can be determined by the difference between the energy consumption before and after optimization. The result is shown in Figure 8.
Generation and Storage Buildings
Figure 9 shows the energy demand of buildings when the renewable capacity system and the energy storage system are connected as the building interaction strategy. While the energy storage system reduces the high-cost power purchase during peak periods through load shifting, the system can also reduce the excessive power purchases of users during the low period through the self-production and self-sale of renewable energy; on this basis, the renewable energy system can also reduce the power purchases by users during peak periods. This article is based on the relevant measurement data of Hong Kong’s ZCB renewable energy power generation. Using the output calculation model given in the literature (Kusakana and Vermaak, 2014; Guo et al., 2019) for data processing, the simulated distributed energy system is transplanted to the campus building of Hong Kong Polytechnic University for data analysis of generation & storage buildings.
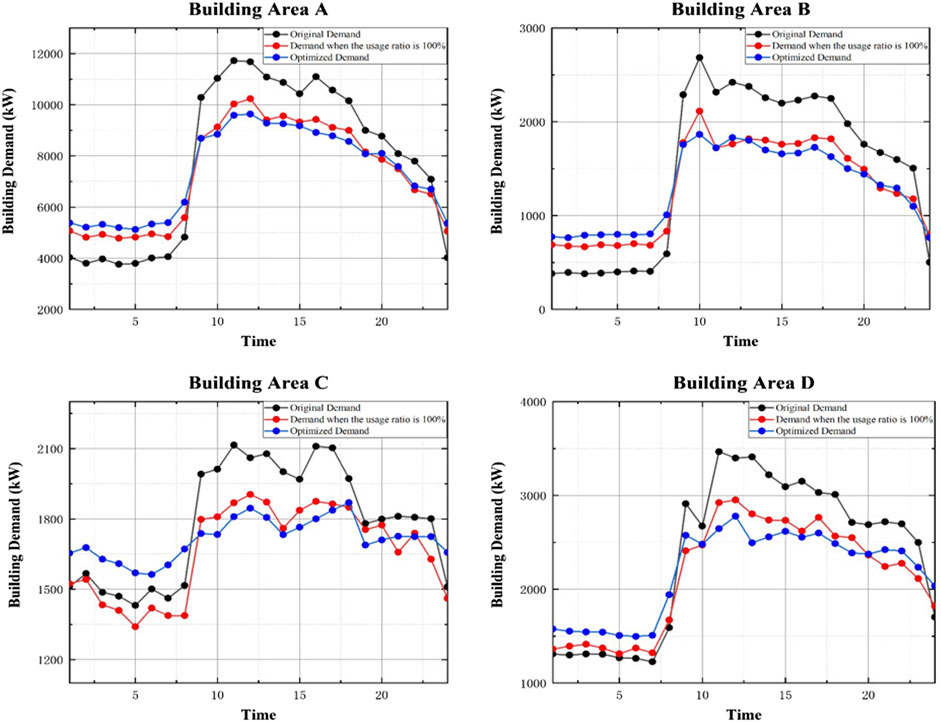
FIGURE 9. Optimized demand profiles of four campus accounts on the test day of generation and storage buildings.
The red curve in Figure 9 is the building energy consumption curve when all renewable energy production capacity is fully used in real time. Compared with the blue curve representing the final optimization result, after using a reasonable internal energy control strategy optimization, the peak energy consumption of the building is significantly reduced, the fluctuation of energy consumption is reduced, and the hidden cost of the grid can be significantly controlled. The specific output storage value is shown in Figure 10 as the building’s internal strategy.
Economic Analysis
Figure 11 shows the electricity purchase cost of each building area under three different system conditions. For passive storage buildings, by sacrificing a certain degree of comfort, the peak energy consumption is reduced, and consequently, the electricity purchase cost is directly reduced by 3–4.4%. For active storage buildings, since the use of stored energy during peak periods reduces the demand for electricity, the cost of electricity purchases can be reduced, consider the increase in cost caused by excessive power purchase during the low electricity consumption period, the electricity bills of different area have been reduced by 5.5–8.8%. For generation & storage buildings, With the further improvement of building energy flexibility, peak electricity consumption has been greatly reduced, the total daily electricity purchase bill of the building decreased by 13.7–18%.
After adopting the hierarchical optimization method, although the direct sales revenue of smart grid will decrease because of the reduction of the building electricity purchase cost, the peak-to-valley difference is also significantly reduced due to building energy demand optimization, thereby effectively reducing the hidden costs caused by grid imbalance. As shown in Figure 12, the grid’s total income has increased by 8, 12.3, and 20%.
Discussion
The effectiveness and advantages of the hierarchical optimization method proposed in this paper are discussed by comparing with several optimization methods that have been used in previous studies. References (Yang et al., 2014; Zhang et al., 2019) are selected for comparison of optimization methods based on game theory, and references (Wang et al., 2018; Huang et al., 2019) are introduced for comparison of two-stage optimization methods. The analysis details are shown in Table 3.
Conclusion
A hierarchical demand optimization method based on game theory is proposed for achieving the optimal grid interaction between smart grids and energy flexible buildings. Considering the impact of the diversity of the EFB system on the outcome of the game, a case study of three types of buildings with different energy flexibilities is conducted. The following remarkable conclusions can be drawn.
1. Game theory can be used as an efficient mathematical tool to coordinate buildings with different energy flexibilities for effective interactions with smart grids. The Stackelberg model can be effectively used to identify the hierarchical game equilibrium in the economic competition between the power grid and buildings.
2. The effectiveness of the proposed method is quantitatively verified in a case study that consists of three typical energy flexible buildings in Hong Kong. Results show that the proposed hierarchical demand optimization method can consecutively reduce grid fluctuations by 30, 44, and 50% while increasing the comprehensive income of the grid by 8–20%; for the demand side (the EFB), peak demand is reduced by 6–22%, and electricity costs are reduced by 3–18%.
3. Building energy flexibility conditions have a significant impact on the decision-making and ultimate benefits in the game. During the demand side energy consumption optimization and result analysis, full consideration of the building system diversity can make the forecast results more accurate. Buildings with larger energy flexibility can have lower peak power demand, which will bring smaller fluctuations to the grid and occupy a more active position in the game with the grid. Compared with active storage and passive storage buildings, the electricity price of the generation & storage buildings with the strongest energy flexibility is reduced by 10–11.8% and 12.6–14% during the peak electricity consumption period, the whole-day electricity cost is reduced by 13 and 16% respectively.
Data Availability Statement
The raw data supporting the conclusion of this article will be made available by the authors, without undue reservation.
Author Contributions
Conceptualization, CY; Data curation, JX; Investigation, YX; Methodology, CY; Writing-original draft, JX and YX; Writing-review and editing, JS and KS; Writing-review and data provision, XX.
Funding
The authors gratefully acknowledge the support of the National Key Research and Development Program of China (2018YFE0116300), the National Natural Science Foundation of China (No. 51708287), the Priority Academic Program Development of Jiangsu Higher Education Institutions, and a grant of the Qingdao Hisense Hitachi Air-conditioning Systems Co., Ltd., and Science and Technology Research and Development Project of CSCEC (No. CSCEC-2019-Z-3).
Conflict of Interest
JS and KS were employed by Qingdao Hisense Hitachi Air-conditioning Systems Co., Ltd. Contributed to the writing-review, editing of the paper, and provided funding for this research. XX is employed by the Second Construction Co., Ltd., China Construction Third Engineering Bureau. Contributed to the review of the paper, and provided data for this research.
The remaining authors declare that the research was conducted in the absence of any commercial or financial relationships that could be construed as a potential conflict of interest.
Publisher’s Note
All claims expressed in this article are solely those of the authors and do not necessarily represent those of their affiliated organizations, or those of the publisher, the editors and the reviewers. Any product that may be evaluated in this article, or claim that may be made by its manufacturer, is not guaranteed or endorsed by the publisher.
References
Arteconi, A., Mugnini, A., and Polonara, F. (2019). Energy Flexible Buildings: A Methodology for Rating the Flexibility Performance of Buildings with Electric Heating and Cooling Systems. Appl. Energ. 251, 113387. doi:10.1016/j.apenergy.2019.113387
Basar, T., and Olsder, G. J. (1999). Dynamic Noncooperative Game Theory. London: Accademic Press. 30. doi:10.1016/0377-2217(95)90173-6
Chen, C., Pearlmutter, D., and Schwartz, M. (2019). Promoting green Building in Israel: A Game Theory-Based Analysis. Building Environ. 163, 106277. doi:10.1016/j.buildenv.2019.106227
DoE (2014). Buildings Energy Databook. Washington, D.C.: Energy Efficiency & Renewable Energy Department.
Electrical and Mechanical Services Department of Hong Kong (2012). Hong Kong Energy End-Use Data. Available at: www.emsd.gov.hk/emsd/e_download/pee/HKEEUD 2012.pdf.
Guo, S., Yang, H., Li, Y., Zhang, Y., and Long, E. (2019). Energy Saving Effect and Mechanism of Cooling Setting Temperature Increased by 1 °C for Residential Buildings in Different Cities. Energy and Buildings 202, 109335. doi:10.1016/j.enbuild.2019.109335
Huang, P., and Sun, Y. (2019). A Collaborative Demand Control of Nearly Zero Energy Buildings in Response to Dynamic Pricing for Performance Improvements at Cluster Level. Energy 174, 911–921. doi:10.1016/j.energy.2019.02.192
Huang, P., Xu, T., and Sun, Y. (2019). A Genetic Algorithm Based Dynamic Pricing for Improving Bi-directional Interactions with Reduced Power Imbalance. Energy and Buildings 199, 275–286. doi:10.1016/j.enbuild.2019.07.003
Huang, Z., Yu, H., Peng, Z., and Feng, Y. (2017). Planning Community Energy System in the Industry 4.0 Era: Achievements, Challenges and a Potential Solution. Renew. Sustain. Energ. Rev. 78, 710–721. doi:10.1016/j.rser.2017.04.004
Jensen, S. Ø., Marszal-Pomianowska, A., Lollini, R., Pasut, W., Knotzer, A., Engelmann, P., et al. (2017). IEA EBC Annex 67 Energy Flexible Buildings. Energy and Buildings 155, 25–34. doi:10.1016/j.enbuild.2017.08.044
Jian, Y., and Wang, R. (2010). Comparative Study of Software thermal Model Affecting Load Calculation Results[J]. J. Beijing Univ. Technol. (12), 53–58.
Kusakana, K., and Vermaak, H. J. (2014). Hybrid Diesel Generator/renewable Energy System Performance Modeling. Renew. Energ. 67, 97–102. doi:10.1016/j.renene.2013.11.025
Lai, A., Leung, M. K., Yau, R., and Ng, E. (2013). Hong Kong First Zero Carbon Building: Design Principles, Features and Technologies. In: PLEA2013 - 29th Conference, Sustainable Architecture for a Renewable Future, Munich, Germany 10-12 September 2013.
Li, H., and Wang, S. (2020). Model-based Multi-Objective Predictive Scheduling and Real-Time Optimal Control of Energy Systems in Zero/low Energy Buildings Using a Game Theory Approach. Automation in Construction 113, 103139. doi:10.1016/j.autcon.2020.103139
Liang, P., Zhang, S., Xu, L., and Li, Y. (2020). Thermal Performance Optimization and Evaluation of a Redial Finned Shell-And-Tube Latent Heat thermal Energy Storage Unit. Appl. Therm. Eng. 166, 114753. doi:10.1016/j.applthermaleng.2019.114753
Lüth, A., Zepter, J. M., Crespo del Granado, P., and Egging, R. (2018). Local Electricity Market Designs for Peer-To-Peer Trading: The Role of Battery Flexibility. Appl. Energ. 229, 1233–1243. doi:10.1016/j.apenergy.2018.08.004
Lv, T., and Ai, Q. (2016). Interactive Energy Management of Networked Microgrids-Based Active Distribution System Considering Large-Scale Integration of Renewable Energy Resources. Appl. Energ. 163, 408–422. doi:10.1016/j.apenergy.2015.10.179
Najafi-Ghalelou, A., Zare, K., and Nojavan, S. (2018). Optimal Scheduling of Multi-Smart Buildings Energy Consumption Considering Power Exchange Capability. Sustain. Cities Soc. 41, 73–85. doi:10.1016/j.scs.2018.05.029
Reynders, G., Amaral Lopes, R., Marszal-Pomianowska, A., Aelenei, D., Martins, J., and Saelens, D. (2018). Energy Flexible Buildings: An Evaluation of Definitions and Quantification Methodologies Applied to thermal Storage. Energy and Buildings 166, 372–390. doi:10.1016/j.enbuild.2018.02.040
Saad, W., Han, Z., Poor, H., and Basar, T. (2012). Game-theoretic Methods for the Smart Grid: an Overview of Microgrid Systems, Demand-Side Management, and Smart Grid Communications. IEEE Signal. Process. Mag. 29, 86–105. doi:10.1109/msp.2012.2186410
Srinivasan, D., Rajgarhia, S., Radhakrishnan, B. M., Sharma, A., and Khincha, H. P. (2017). Game-Theory Based Dynamic Pricing Strategies for Demand Side Management in Smart Grids. Energy 126, 132–143. doi:10.1016/j.energy.2016.11.142
Tang, R., Wang, S., and Li, H. (2019). Game Theory Based Interactive Demand Side Management Responding to Dynamic Pricing in price-based Demand Response of Smart Grids. Appl. Energ. 250, 118–130. doi:10.1016/j.apenergy.2019.04.177
Wang, D., Qiu, J., Reedman, L., Meng, K., and Lai, L. L. (2018). Two-stage Energy Management for Networked Microgrids with High Renewable Penetration. Appl. Energ. 226, 39–48. doi:10.1016/j.apenergy.2018.05.112
Wang, S. (2016). Making Buildings Smarter, Grid-Friendly, and Responsive to Smart Grids. Sci. Technol. Bulid. Environ. 22 (6), 629e632. doi:10.1080/23744731.2016.1200888
Xu, Y., Yan, C., Liu, H., Wang, J., Yang, Z., and Jiang, Y. (2020). Smart Energy Systems: A Critical Review on Design and Operation Optimization. Sustain. Cities Soc. 62, 102369. doi:10.1016/j.scs.2020.102369
Yan, C., Wang, F., Pan, Y., Shan, K., and Kosonen, R. (2020). A Multi-Timescale Cold Storage System within Energy Flexible Buildings for Power Balance Management of Smart Grids. Renew. Energ. 161, 626–634. doi:10.1016/j.renene.2020.07.079
Yang, J., Zhang, G., and Ma, K. (2014). Matching Supply with Demand: A Power Control and Real Time Pricing Approach. Int. J. Electr. Power Energ. Syst. 61, 111–117. doi:10.1016/j.ijepes.2014.03.014
Yang, P., Tang, G., and Nehorai, A. (2013). A Game-Theoretic Approach for Optimal Time-Of-Use Electricity Pricing. EEE Trans. Power Syst. 28 (2), 884. doi:10.1109/tpwrs.2012.2207134
Zhang, X., Gao, G., Zhang, Z., and Yang, L. (2019). Demand Response-Based Optimal Dispatching of Building Integrated Energy System. Power Demand Side Manage. 4, P28–P34.
Zhao, X. (2001). Nature of Nonlinear Cournot Model and Stackelberg Model. CNKI:SUN:JSDN.0. 2001-03-001. J. Jishou Univ (Nat. Scie. Ed.).
Zhong, J., Ju, X., and Lu, Y. (2016). Distributed Renewable Energy Building Application Development Path and Policy Guidance. Bull Chin Acad Sci. 31, 2. doi:10.16418/j.issn.1000-3045.2016.02.008
Glossary
a, b, c Preset constants that affect the cost of power generation
∅ value range of building strategy
EFB Energy Flexible Building
IEA International Energy Agency
DN Day and Night Price
TOU Time-of-use Price
RTP Real-time Prices.
Keywords: energy flexible building, demand optimization, game theory, smart grid, grid interaction
Citation: Xu J, Yan C, Xu Y, Shi J, Sheng K and Xu X (2021) A Hierarchical Game Theory Based Demand Optimization Method for Grid-Interaction of Energy Flexible Buildings. Front. Energy Res. 9:736439. doi: 10.3389/fenrg.2021.736439
Received: 05 July 2021; Accepted: 16 August 2021;
Published: 31 August 2021.
Edited by:
Yongjun Sun, City University of Hong Kong, Hong Kong, SAR ChinaCopyright © 2021 Xu, Yan, Xu, Shi, Sheng and Xu. This is an open-access article distributed under the terms of the Creative Commons Attribution License (CC BY). The use, distribution or reproduction in other forums is permitted, provided the original author(s) and the copyright owner(s) are credited and that the original publication in this journal is cited, in accordance with accepted academic practice. No use, distribution or reproduction is permitted which does not comply with these terms.
*Correspondence: Chengchu Yan, Y2hlbmdjaHUueWFuQG5qdGVjaC5lZHUuY24=